ABSTRACT
The virulence factors of Staphylococcus aureus are tightly controlled by two-component systems (TCSs) and small RNA (sRNA). TCSs have been well studied over the past several decades, but our understanding of sRNA functions lags far behind that of TCS functions. Here, we studied the biological role of sRNA from 506 S. aureus RNA-seq datasets using independent component analysis (ICA). We found that a previously neglected sRNA, Sau-41, functions in the Agr system. Sau-41 is located within the PSMα operon and controlled by the Agr system. It was predicted to share 22-base complementarity with RNAIII, a major regulator of S. aureus virulence. The EMSA results demonstrated that Sau-41 directly binds to RNAIII. Furthermore, our results found that Sau-41 is capable of repressing S. aureus haemolysin activity by downregulating α-haemolysin and δ-toxin. The repression of α-haemolysin was attributed to the competition between the 5’ UTR of hla and Sau-41 for binding RNAIII. We observed that Sau-41 mitigated S. aureus virulence in an orthopaedic implant infection mouse model and alleviated osteolysis. Together, our results indicate that Sau-41 is a virulence-regulating RNA and suggest that Sau-41 might be involved in a negative feedback mechanism to control the Agr system. This work is a demonstration of using ICA in sRNA identification by mining high-throughput data and could be extended to other organisms as well.
Introduction
S. aureus, a gram-positive bacterium that commonly resides on human skin and mucous membranes as a commensal, is known to cause a wide range of infectious diseases, including skin abscesses, osteomyelitis, endocarditis, bacteraemia, and pneumonia [Citation1–3]. sRNAs serve as post-transcriptional regulators for various mRNAs, either encoded in cis or trans, and has been proven to be significant in bacteria’s environmental adaptation. A recent review [Citation4] suggests that the heightened virulence of S. aureus compared to coagulase-negative Staphylococci (CoNS) may be partly attributable to the presence of sRNAs, which have been frequently identified in S. aureus strains but not in CoNS.
Over the past three decades, there has been a growing focus on the role of sRNAs in regulating virulence genes in S. aureus. By utilizing advanced techniques such as tiling arrays and RNA-Seq, numerous putative sRNAs have been described [Citation5], with the SRD database currently housing 575 unique sRNA sequences [Citation6]. However, the surge in sRNA identification has primarily been limited to descriptive studies concerning sRNA expression, while investigations into their specific molecular targets lagged behind temporarily. Nonetheless, recent developments have yielded various approaches to enhance our understanding of sRNA functions and mechanisms of action in S. aureus. Computational methods (e.g. intaRNA [Citation7], RNApredator [Citation8], TargetRNA2 [Citation9] have emerged as a valuable tool for predicting sRNA targets based on sequence complementarity and homology. These predictions have exhibited high accuracy, underscoring the important role of computational approaches in identifying sRNA targets.
In recent years, researchers have increasingly embraced machine learning techniques for data mining, decomposition, and the prediction of complex biological information [Citation10]. Among these techniques, module detection methods have proven effective in identifying functionally related and co-regulated groups of genes (as well as non-coding RNA), thereby enabling the inference of biological explanations from large gene expression datasets [Citation11]. Independent component analysis (ICA) has emerged as the optimal algorithm for module detection and has been employed in decomposing mRNA transcriptional regulation networks (TRNs) in various organisms, including S. aureus, Pseudomonas aeruginosa, E. coli, and Bacillus subtilis [Citation12–15]. Although these studies have advanced our comprehension of regulator-target relationships, they have not investigated the co-regulation of sRNA and mRNA in the transcriptional regulatory networks of S. aureus. Given the exponential growth of publicly available RNA-seq data, this study recognizes the untapped potential of sRNA analysis as a valuable resource.
In the present study, we collected a dataset comprising 494 high-quality S. aureus RNA-seq samples from public databases supplemented by 12 in-house RNA-seq datasets, to perform ICA analysis on both mRNA and sRNA expression profiles. Through decomposition of the expression profiles, we identified Sau-41 as a component of the Agr quorum-sensing system. Subsequent in vitro experiments confirmed that Sau-41, an uncharacterized sRNA, can repress S. aureus virulence by interacting with RNAIII, a major regulator of the Agr system. Furthermore, we validated the role of Sau-41 in regulating S. aureus virulence in vivo and demonstrated that upregulation of Sau-41 can mitigate infection using an orthopaedic implant infection model.
In conclusion, this study not only sheds light on the prospect of employing ICA for prokaryotic sRNA discovery but also reveals a previously unknown sRNA, Sau-41, which plays a crucial role in regulating S. aureus virulence. The comprehensive validation of the function of Sau-41 in vitro and in vivo strengthens our understanding of its significance.
Material and methods
GEO data acquisition and quality control
By searching the GEO and SRA databases, a total of 854 S. aureus RNA-seq datasets were downloaded from the GEO database. The downloaded data were then subjected to procedures including adapter trimming, quality filtering, and per-read quality pruning using the fastp program. Low-quality data were excluded following the workflow illustrated in Fig. S1. Briefly, data that failed in the FASTQC program were excluded for subsequent analysis as described in a previous study [Citation12]. After transcript quantification with salmon, datasets with mapped reads less than one million or Pearson correlation R2 values within replicates lower than 0.97 were discarded. Finally, outlier samples, samples without technical/biological replicates, samples with no reference group, samples with poor experimental metadata, and datasets from unstranded cDNA libraries were discarded. Detailed information on qualified RNA-seq data is listed in Table S1.
RNA-seq data analysis with the pangenome method
Twenty-five representative S. aureus genomes were selected from the panX database [Citation16] and their coding sequences were predicted using the software prokka [Citation17]. Details of the representative S. aureus genomes are listed in Table S2. S. aureus sRNA sequences from USA300-FPR3757 were obtained from the SRD database [Citation6]. Since most of the read lengths are longer than 100 bp, we decided to solely incorporate sRNAs that are longer than 100 bp. The concept of pangenome-based RNA-seq read mapping was conducted as illustrated in . Briefly, all of the coding sequences of the 25 representative genomes and sRNA longer than 100 bp were collected and merged into a single FASTA file, which was regarded as the reference genome for the subsequent read-mapping procedure. Then, the sequencing reads were quasi-mapped into the reference genome, and transcript expression levels were quantified using Salmon software [Citation18].
Figure 1. Schematic illustration displaying the procedure of this study. (a) RNA-seq data were either downloaded from the GEO database or generated in-house. Samples without detailed experimental information or with no experimental replicates were excluded. (b) RNA-seq reads were mapped to the S. aureus pangenome reference and transcripts abundances were quantified using salmon software. (c) Expression matrix derived from the previous step was used as the input data for the ICA analysis pipeline. Resulting in M and a tables containing iModulon information. M table: Each column represents an independent component (IC), and each row contains the gene weights for each gene across each IC: Each column represents a sample, and each row contains the activity of each iModulon across all samples. (d) The M and a tables together with sample information were used as input for iModulon characterization using python package pymodulon. Components of iModulon containing both sRNA and genes were identified, and their activity under each experimental condition was visualized with bar plots.
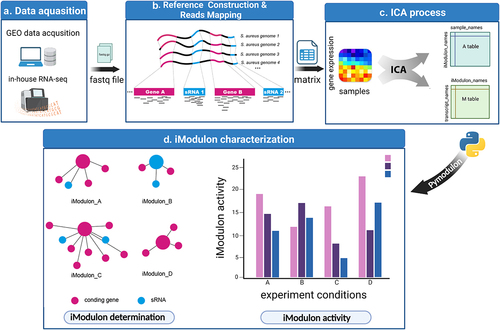
Independent component analysis (ICA) and iModulon inference
The procedure of computing robust independent components with ICA was carried out according to descriptions in previous studies [Citation12] and their GitHub repository (https://github.com/avsastry/modulome-workflow.git), the corresponding scripts were also provided in the supplementary material. Briefly, Log2(TPM +1) centred on the reference conditions was used as input for ICA analysis. FastICA was executed with a convergence tolerance of 10−7. The procedure was repeated several times on the input datasets ranging in dimension from 20 to 350 with a step size of 30. The optimal dimensionality was determined as instructed by McConn et al.[Citation19]
Gene annotation, computing iModulon thresholds and regulator enrichment
The S. aureus gene annotation information was available from the panX database [Citation16]. Additionally, the KEGG information was downloaded from EggNOG-mapper [Citation20]. The TRN file was obtained from the RegPrecise database [Citation21]. The iModulon thresholds were determined, and regulator enrichment was performed with the previously developed python module pymodulon [Citation13].
Oligonucleotide sequences, plasmids, bacterial strains, and growth conditions:
All oligonucleotide sequences used in this study are listed in Table S3. The bacterial strains and plasmids used in this study are listed in Table S4. S. aureus ST1792 is a clinical isolate from a patient with periprosthetic joint infection. For the 12 in-house generated RNA-seq, overnight grown ST1792 was inoculated into 10 mL of fresh TSB supplied with 20% human body fluid (plasma, synovial fluid, drainage fluid, or ddH2O) at 37 ℃ with shaking at 200 rpm for 6 hours until the OD600 reached 0.6. Each condition was subjected to three biological replicates. S. aureus carrying plasmids were grown in TSB (tryptic soy broth supplemented with 10.0 μg/mL chloramphenicol) at 37 °C/200 rpm.
RNA extraction, library construction and sequencing
For in-house transcriptome sequencing, bacterial cells (ST1792) were collected by centrifugation at 4000 rpm for 15 min, and the bacterial wall was physically disrupted by a tissue lyser. Then, the total RNA was purified using the EZ-press microRNA Purification kit plus (EZBioscience, China) according to the manufacturer’s instructions. RNA quality was assessed by an Agilent 2100 bioanalyzer (Agilent, USA). For each sample, after ribosomal RNA depletion, a total of 10 μg of RNA was disrupted into 200 ~ 250 bp segments and reverse transcribed into cDNA with random hexamer primers. All of the constructed cDNA libraries were sequenced using the HiSeq2000 (Illumina, USA) sequencer.
Sau-41 overexpression and knockdown
For Sau-41 overexpression, the plasmid pRMC2 was used as the backbone, and Sau-41 regulated by the well-studied strong promoter cap1A was synthesized chemically and ligated with a PCR-generated linearized pRMC2 fragment using Gibson assembly, resulting in the recombinant pRMC2_Sau-41 plasmid. For Sau-41 mutant overexpression, the pRMC2_Sau-41 plasmid was mutated with a point mutation kit (Vazyme, China), resulting in the pRMC2_Sau-41mt plasmid.
For Sau-41 knockdown, we used a dcas9-based transcriptional repression system, and the previously reported pSD1 [Citation22] was used as the backbone. sgRNA oligos were annealed and inserted into the pSD1 plasmid using golden gate assembly as described by Zhao [Citation22]. The recombinant plasmid was named pSD1_Sau-41.
All recombinant plasmids were transferred into S. aureus strain RN4220 with electroporation as described previously [Citation23].
Hemolysis assay
Overnight-grown S. aureus was diluted 1:50 and grown at 37 °C for 2 hours. The bacterial supernatant was then filter-sterilized (0.22 μm) and collected to investigate haemolysis activity. Peripheral blood was collected from healthy volunteer donors with their informed consent at Shanghai Sixth People’s Hospital Affiliated to Shanghai Jiao Tong University School of Medicine. Human red blood cells were collected by centrifuging 1 mL of human peripheral blood and resuspending it in 10 mL of PBS. The supernatant was mixed with blood samples at a 1:1 ratio and incubated at 37 °C for 1 hour. Sterile H2O was added as the positive control. Incubated blood samples were transferred into 96-well plates, and the OD 540 nm was measured with a microplate reader (BioTek, United States).
Northern blot
S. aureus total RNA was extracted as described above. Ten micrograms of RNA was mixed with 2X RNA loading buffer, and equal volumes were loaded onto a 10% urea-polyacrylamide gel (PAGE). After electrophoresis (100 V for 8 × 8 * 0.15 cm gel), gels were electrotransferred (380 mA, 60 min) onto a positively charged nylon membrane. After prehybridization using hybridization buffer IV (Sangon, Shanghai, China) at 37 °C for 3 hours, the nylon membrane was hybridized with 50 pM biotin-labelled probes at 37 °C overnight. The blotting was detected using chemiluminescence methods after incubation with streptavidin-HRP conjugate (Beyotime). Finally, a Tannon visualization system (Tannon, China) was used for visualization. The resultant image was quantified using ImageJ (1.53c, Fiji).
RNA in vitro transcription, labelling, and electrophoretic mobility shift assay
Sau-41, RNAIII, and the 5’ UTR of hla were transcribed in vitro using a T7 High Yield RNA Transcription Kit (Beyotime, China). The transcription template was generated using PCR. RNA was labelled with a Pierce™ RNA 3” End Biotinylation Kit (Thermo, USA). RNA denaturing, refolding, and binding reactions were performed as described by Huyen et al. [Citation24]. A total of 6 nM labelled Sau-41 was incubated with unlabelled RNAIII at various concentrations. Then, 6 nM labelled hla 5” UTR and 6 nM unlabelled RNAIII were incubated with various concentrations of unlabelled Sau-41. The prepared RNA samples were loaded on a native polyacrylamide gel and underwent electrophoresis in 0.5× TBE until the bromophenol blue dye migrated 3/4 down the length of the gel. (100 V for 8 × 8 * 0.15 cm gel). The gel was then electrotransferred (380 mA, 60 min) onto a positively charged nylon membrane using a standard Bio-Rad transfer apparatus. After UV-crosslinking, the biotin-labelled RNAs were detected using chemiluminescence methods as instructed by the Chemiluminescent Biotin-labelled Nucleic Acid Detection Kit (Beyotime, China) and visualized using a Tannon visualization system (Tannon, China).
S. aureus quantitative PCR
RN4220 cells carrying pRMC2_Sau-41, pSD1_Sau-41 or pRMC2 plasmids were grown in TSB (10 μg/mL chloramphenicol) for 8 hours at 37℃/200 rpm with an initial 1:100 dilution of overnight grown culture. Bacterial cells were collected, and total RNA was extracted as described above. The RNA samples with absorbance ratios of 260 nm/280 nm and 260 nm/230 nm higher than 2.0 were transcribed into cDNA, and the subsequent quantitative PCR analysis was performed as described previously [Citation23]. The relative gene transcription level was quantified with the 2−ΔΔt method using gyrB as the internal reference gene.
In vivo infection model, bacterial burden quantification and μCT analysis
Twenty-four C57BL/6 mice (6 weeks old) were randomly divided into three groups (wild-type, Sau-41 overexpression, and Sau-41 knockdown). The intramedullary femur implant mouse model was conducted as described previously [Citation23]. Approximately 1 × 10[Citation6] CFU S. aureus in a total volume of 50 μL PBS was inoculated into the knee joint capsule on the first day after surgery. All mice were euthanized on the seventh day after infection. Thirty femurs were prepared for bacterial burden quantification, and the remaining 18 femurs underwent μCT scanning using a Sky scan 1172 μCT scanner (Sky Scan, Ltd., Kartuizersweg, Kontich, Belgium). Bone volume/total tissue volume (B.V./T.V.) and bone mineral density (BMD) of the peri-implant region for each specimen was performed using the skyscan CTanalyzer software.
Statistical analysis
Statistical significance between two independent groups was determined using an unpaired two-tailed t test. Multiple comparisons were performed using one-way analysis of variance (ANOVA) with Tukey’s post hoc test. The correlation between RNAIII and Sau-41 transcription levels was analysed using the Pearson test. All analyses were performed using GraphPad Prism 8.3.0 unless otherwise stated. Statistical significance was indicated as a two-sided P < 0.05. All the data are presented as the mean ± standard deviation.
Results
Improving the traditional RNA-seq mapping pipeline
To explore the biological function of sRNA in S. aureus, our study involved four consecutive stages: RNA-seq data acquisition, reference genome construction and read mapping, ICA process, iModulon characterization (). The RNA-seq data were either obtained from the GEO database (n = 854) or generated in-house (n = 12). Due to the diversity of the S. aureus genetic background, reads from strains with different genetic backgrounds should be mapped to a representative reference genome. Thus, we adopted the quasi-mapping software (Salmon) and used the pangenome as a reference genome (as illustrated in ). Pangenome analysis of S. aureus genomes indicated that the number of new genes and conservative genes decreased sharply when the number of involved genomes was less than 20 (). Then, we selected 25 representative genomes from 344 S. aureus genome sequences that were deposited in the panX database and constructed a reference pangenome for reads mapping. The distribution of 25 selected genomes in the S. aureus phylogenetic tree is shown in Figure S2, and their detailed information is listed in Table S2. Our computational results showed that the mapping rate gradually increased along with the increase in the given genome number (). The performance of the pangenome mapping method was then tested in several example datasets and compared with a single reference; the result displayed in shows that the pangenome mapping method could effectively remove the reference bias caused by genome variation.
Figure 2. Pangenome-based read mapping methods outperform single-genome-based methods. (a) The cumulative number of conserved genes and total genes with increasing in pangenome size. (b) Number of new genes and unique genes with increasing in pangenome size. (c) Percentage of mapped reads when aligned to pangenomes of different sizes (number of tested RNA-seq datasets = 5). (d) Mapping rates of RNA-seq data derived from 6 S. aureus strains (BPH2947, LAC, MW2, Newman, TCH1516 and BPH2819) when aligned to the pangenome or single genome.

RNA-seq processing and ICA analysis
After processing the 866 RNA-seq datasets with the quality control workflow illustrated in Figure S1, 360 samples did not meet the quality control standard for any of the following reasons: poor quality reported by FASTQC, inadequate sequencing depth, inadequate metadata information, non-strand-specific library, and low correlation rate with replicates (R ≤ 0.97). The remaining 506 qualified samples, which had an average correlation value of R2 = 0.99 between replicates, were included for subsequent analysis (Figure S3).
Application of ICA to the 506 high-quality expression matrix results in 94 independently regulated modules (iModulons). After excluding iModulons containing only one gene component, 62 iModulons were retained for the following analysis. Most of the iModulons involve in the metabolism pathway (e.g. sugar, amino acids, nucleotide metabolism) (). Those iModulons overlapped with S. aureus regulons previously reported in the literature were named after the regulators (upper right corner of ). Other iModulons, which were enriched for biological pathways or upregulated in specific conditions were named according to their function. For example, iModulons that are exclusively upregulated in biofilm state were named Biofilm-1/2/3. The remaining 17 uncharacterized iModulons represent gene sets with unidentifiable functions based on our current knowledge. Among the functional iModulons, we found a set of well-documented β-lactam resistance genes (blaZ, blaR1, blaI, mecA, mecR1) () enriched in the β-lactam iModulon. The activity of β-lactams iModulon was inducible upon nafcillin treatment in the case of strains TCH1516 and D712 but not in LAC (). These results demonstrated that our computational analysis could well reflect the biological function to some extent.
Figure 3. Characteristics of the S. aureus iModulons. (a) Treemap of the 62 S. aureus iModulons. The size of each box represents the explained variance contributed to S. aureus whole transcriptome. Large explained variance indicates more dynamic changes in iModulon activity across conditions. (b) Association between S. aureus iModulons and previously published regulons. iModulons with high fraction of shared genes with published regulon recall were named after the regulon. (c) β-Lactam iModulon components. Each point represents a gene. The x-axis represents the gene ID, and the y-axis indicates the weight of the gene in iModulon. The genes outside the dashed black lines (threshold) are components of iModulon. (d) Bar plot of the iModulon activities for the β-lactam iModulon under different experimental conditions.

Characteristics of Sau-41, a new member of the Agr quorum-sensing system
We focused on AgrA iModulon because it is a well-studied quorum sensing system regulating the production of many S. aureus virulence factors. Our ICA results showed that AgrA iModulon consists of nine components: PSMα, PSMβ, AgrA-D, Spa, RNAIII, and Sau-41 (). The distribution of the AgrA iModulon in the MW2 genome is shown in . As anticipated, RNAIII, the most studied virulence-regulating RNA, is enriched in AgrA iModulon [Citation27]. Interestingly, a previously unnoticed sRNA named Sau-41 also appeared in AgrA iModulon. We then investigated the Sau-41 locus and found that Sau-41 overlapped with the 3’ part of the PSMα transcript (). Sau-41 shares a common terminator with PSMa and has an experimentally verified independent transcriptional start site (TSS) [Citation25]. It is interesting to note that this TSS was not identified in a recently published study describing the 1861 TSSs in TCH1516 genome [Citation26], indicating that Sau-41 transcription was either dependent on the experiment condition or strain-specific ().
Figure 4. Characteristics of Sau-41. (a) the weight of genes in AgrA iModulon. Genes with prior evidence of Agr regulation are labelled with orange.The genes above the threshold were enriched in AgrA iModulon (b) Distribution of AgrA iModulon components in the MW2 genome. (c) Genomic localization of Sau-41, psmα1–4, and the transcriptional start site (indicated with red arrows) in TCH1516 and MW2 strain. Sau-41 was located within psmα coding sequence and shares a terminator with psmα. TSSs were named after their location in the genome. The TSSs information was derived from previous study [Citation25,Citation26]. (d) Transcription of Sau-41 and RNAIII in USA300 and its isogenic mutant strain ΔagrA at different time points during growth was detected using Northern blot. (e) Quantified transcript level for Sau-41 at various time points was related to RNAIII. The transcript level of each RNA at the first time point was normalized to 100%.
![Figure 4. Characteristics of Sau-41. (a) the weight of genes in AgrA iModulon. Genes with prior evidence of Agr regulation are labelled with orange.The genes above the threshold were enriched in AgrA iModulon (b) Distribution of AgrA iModulon components in the MW2 genome. (c) Genomic localization of Sau-41, psmα1–4, and the transcriptional start site (indicated with red arrows) in TCH1516 and MW2 strain. Sau-41 was located within psmα coding sequence and shares a terminator with psmα. TSSs were named after their location in the genome. The TSSs information was derived from previous study [Citation25,Citation26]. (d) Transcription of Sau-41 and RNAIII in USA300 and its isogenic mutant strain ΔagrA at different time points during growth was detected using Northern blot. (e) Quantified transcript level for Sau-41 at various time points was related to RNAIII. The transcript level of each RNA at the first time point was normalized to 100%.](/cms/asset/8a4ed921-a73b-45bf-9edf-0c2dd6a3de20/kvir_a_2228657_f0004_oc.jpg)
Northern blotting was used to identify Sau-41 transcription, and we observed two bands. The lower band corresponds to the size of Sau-41 (~156 nt), and the upper band was speculated to be the PSMα transcript (~500 nt) (). Northern blot analysis of S. aureus strain USA300 at various growth time points and its isogenic Agr mutant strain (ΔagrA) showed that RNAIII and Sau-41 were positively correlated (R2 = 0.931, p < 0.01) and that both were Agr-dependent ().
Sau-41 represses haemolysin expression by interacting directly with RNAIII
To explore the biological function of Sau-41, we overexpressed (Sau-41+) and knocked down Sau-41 (Sau-41kd) in S. aureus strain RN4220 through plasmid-mediated methods. In addition, to exclude the effect of the PSMα4 peptide, the PSMα4 start codon within the Sau-41 sequence was mutated from ATG into TTG for translation interruption () and overexpressed in RN4220 (Sau-41mt+). A significant difference in haemolysis activity was observed among the wild-type, Sau-41+, and Sau-41kd genotypes, the haemolysis activity decreased significantly in the Sau-41+ strain and increased slightly in the Sau-41kd phenotype (). Furthermore, repressed haemolysis activity was also observed in the Sau-41mt+ strain, thus further validating that this phenomenon was caused by Sau-41 sRNA rather than the PSMα4 peptide.
Figure 5. Sau-41 represses S. aureus haemolytic activity. (a) Schematic illustration of the mutation of the PSMα4 start codon. (b) Hemolytic ring of the RN4220 strain carrying Sau-41/Sau-41mt overexpression, knockdown or empty vector plasmid grown on sheep blood agar overnight. (c) Hemolytic activity of the RN4220 strain carrying Sau-41/Sau-41mt overexpression, knockdown or empty vector plasmid determined by incubating bacterial supernatants with 3% human red blood cell. PBS was used as a negative control, and H2O was used as a positive control. (n = 3/group) (d) Transcript levels of hla, hld, psmα, psmβ, hlgA, hlgB, and hlgC were investigated by qPCR in strain RN4220 carrying pRMC2 or pRmc2_sau-41 (n = 3/group). (e) Volcano plot showing the haemolytic factor transcriptional differences between strain RN4220 carrying pRMC2 or pRmc2_sau-41 and their corresponding adjusted p value (q value). (f) the transcriptional activity of hla in strain RN4220 was assessed by analysing cells that harboured plasmids capable of either overexpressing or knocking down Sau-41 (n = 3/group). *p < 0.05, **p < 0.01, ***p < 0.001, ****p < 0.0001. Sau-41+: RN4220 carrying the pRmc2_sau-41 plasmid, Sau-41mt+: RN4220 carrying the pRmc2_sau-41mt plasmid, Sau-41kd: RN4220 carrying pSd1_sau-41, wild-type: RN4220 carrying the pRMC2 empty vector.

To search for the key genes leading to this haemolytic phenotypic change. The transcription level of all the reported S. aureus haemolytic factors was quantified using qPCR. The results showed decreased expression of α-haemolysin and δ-toxin in Sau-41+ strain (). Interestingly, both haemolysins were regulated by RNAIII. The expression of hla (α-haemolysin) was upregulated by RNAIII and the coding sequence of hld (δ-toxin) was located within RNAIII [Citation27]. In addition, RNA‒RNA interaction analysis predicted that there is a base-pairing region between Sau-41 and RNAIII (). The predicted RNAIII-Sau-41 interaction was then experimentally verified using EMSA with in vitro synthetic RNA. The EMSA results showed that a shifted band appeared as the amount of RNAIII increased gradually (). Furthermore, Sau-41 could not bind to RNAIII when the H1-H2 region of RNAIII was deleted (RNAIII-mutant, ).
Figure 6. Sau-41 interacts directly with RNAIII. (a) the base pairing region of Sau-41 and RNAIII was predicted with IntaRNA software. (b) Complex formations between RNAIII and Sau-41 were analysed by EMSA. Labeled Sau-41 mixed with increasing concentrations of RNAIII and RNAIII-mutant (5, 10, 25 nM and 50 nM; RNAIII-mutant: wild-type RNAIII with H1-H2 region deleted). Blank: Biotin-labelled Sau-41 without RNAIII/RNAIII-mutant addition. (c) Illustration of the speculated biological function of Sau-41. Sau-41 modulates S. aureus haemolytic activity by binding with RNAIII. (d) Competition between Sau-41 and hlaUTR binding with RNAIII was analysed by EMSA. Biotin-labelled hlaUTR interacted with RNAIII, and increasing concentrations of Sau-41 (10, 25, 50 and 125 nM) were added to interrupt the interaction. Ctrl: hlaUTR RNA (6 nM) interacting with RNAIII (6 nM) without the addition of Sau-41.

It has been demonstrated that RNAIII promotes hla transcription by binding to its 5’ UTR [Citation28], and we tested whether Sau-41 could interrupt this process(). The competitive EMSA results showed that the RNAIII-hlaUTR interaction decreased as the concentration of Sau-41 increased (). Overall, these results indicate that Sau-41 modulates S. aureus haemolysin by binding to RNAIII.
Sau-41 knockdown increased S. aureus virulence in vivo
It has been demonstrated that agr mutant strains are frequently isolated from persistent infections during clinical practice [Citation29]. Therefore, we used an orthopaedic implant infection mouse model to investigate the role of Sau-41 during pathogenesis (). μCT scanning of the infected femur showed that the peri-implant region in the Sau-41 overexpression group had a significantly increased trabecular bone mineral density (0.6098 ± 0.087 vs. 0.4862 ± 0.056, p < 0.05) and B.V./T.V. (3.759 ± 0.384 v.s. 2.255 ± 0.411, p < 0.0001) compared with the wild-type group. Conversely, the Sau-41kd group displayed a slight impairment in bone mineral density, although no statistical significance was observed (). In addition, the bacterial burden of the infected femur and implant-associated soft tissue increased in the Sau-41kd group compared with the wild-type and Sau-41+ groups (). The differences in bacterial burden were more significant in the bone tissue than in the soft tissue. Taken together, our findings indicate that Sau-41 could mitigate the virulence of S. aureus in vivo and alleviate the osteolysis caused by infection.
Figure 7. Sau-41 represses S. aureus virulence and alleviates osteolysis in vivo. (a) Illustration of the process of in vivo implant infection model construction. (b) μCT result showing the representative axial, coronal and sagittal views of the implanted femur. (c) 3D reconstruction of μCT data of the infected femur. (d-f) Two hundred sections starting from 30 mm proximal to the femur condyles were quantified for cortical (d) and trabecular (e) bone mineral density and bone volume/total volume (B.V./T.V., F) (n = 6 per group). (g-h): Quantification of the bacterial burden in peri-implant soft tissue (g) and femur (h) (n = 10 per group). *p < 0.05, **p < 0.01, ***p < 0.001, ****p < 0.0001. Sau-41+: RN4220 carrying the pRmc2_sau-41 plasmid, Sau-41kd: RN4220 carrying pSd1_sau-41, wild type: RN4220 carrying the pRMC2 empty vector.

Discussion
This study involves the largest S. aureus transcriptome datasets from the publicly available database as well as in-house generated RNA-seq datasets. Compared with previous studies [Citation12,Citation30], our study addresses not only the USA300 strain but also other commonly seen S. aureus strains. The genome diversity among S. aureus requires a better method to overcome the drawback of conventional single-genome-based read alignment. We proposed a pangenome-based method that could be applied to process RNA-seq data from any S. aureus strain with a universal reference sequence. Other studies also developed methods to address the technical problems that arise from genome variation among the population. For example, the concept of a genome variation graph [Citation31] was produced in 2018 to improve read mapping by compactly representing genetic variations among a population. However, such methods require the development of a panel of new software for read mapping and transcript quantification. Another proposed method is a reference flow pipeline [Citation32] in which reads were aligned to distinct reference genomes sequentially until most of the reads were mapped. Theoretically, the time cost of the reference flow pipeline would increase by several times as the number of genomes increased. In this study, the pangenome method was based on the ultrafast transcript quantification algorithm Salmon [Citation18] thus, the time cost for the whole quantification process for hundreds of RNA-seq data could be greatly reduced.
According to our literature review, the ICA algorithm has not been adopted to identify sRNA targets and biological function inference. We regard sRNA as an important part of S. aureus TRN and therefore should be co-regulated with coding sequences. The success of previous application of ICA in exploring S. aureus co-regulated mRNA has proven ICA to be a feasible solution for sRNA research. Using the ICA method, we identified 62 independently modulated gene sets (iModulons). Compared with the previously published 29 iModulons derived from 108 USA300 RNA-seq datasets [Citation12] and the newly introduced 72 iModulons derived from 385 USA300 datasets [Citation30], our study has taken sRNA into account, and the data were based on all available S. aureus strains. This indicates that the result is more universally applicable, without any preference for specific strains.
In this study, we found that a 156-nt sRNA named Sau-41 is capable of repressing S. aureus virulence by interacting with RNAIII. Sau-41 was first identified in 2010 by Abu-Qatouseh et. al [Citation33]. In their study, Sau-41 together with RNAIII were absent in the small colony variant phenotype when compared with the wild-type strain. It is interesting to note that their study also identified two bands in the Northern blot experiment. However, compared with their results, the upper bands in our study were 500-nt, in contrast to 280-nt in a previous study. In order to clarify the inconsistency, we conducted a literature search and discovered two separate studies that reported different outcomes for the S. aureus TSS (as summarized in ). In the case of strain TCH1516, the study indicated a TSS located between psmα1 and psmα2. Conversely, another study conducted on MW2 identified a TSS upstream of psmα4. The divergent TSS findings among these studies suggest that the S. aureus TSS is influenced by experimental conditions and the specific strains that were examined. This discrepancy may partially account for the disparity in the size of the upper band compared to the findings of the previous study. Nevertheless, their finding combined with ours () demonstrated that the transcription of RNAIII was positively correlated with Sau-41. During clinical practice, agr mutants are often isolated from biofilm-associated infections, including cystic fibrosis lung infection, as well as medical device infection [Citation34–36]. The occurrence of agr dysfunctionality has been explained by the decrease in fitness cost of an agr mutant at the expense of the whole community. In contrast to the on/off mode of agr mutants, we found Sau-41 to be a precise repressor of the Agr system by blocking RNAIII. We regarded it as negative feedback of the agr system for preventing S. aureus from becoming hypervirulent ().
Figure 8 (a) Schematic of the S. aureus Agr system. The AgrD precursor undergoes maturation and export through AgrB, generating the AIP signal. Once the AIP concentration reaches a specific threshold, it triggers the activation of the AgrC-AgrA two-component system. Phosphorylated AgrA then initiates transcription from the P2 promoter, leading to the regulation of auto-feedback. In addition, AgrA facilitates the transcription of RNAIII via the P3 promoter. Furthermore, AgrA enhances the transcription of the psmα and psmβ operons, which encode PSM peptides. Sau-41 is transcribed from the psmα operon. (b-c) the mechanism of Sau-41 in regulating S. aureus virulence. (b) Wild-type: Sau-41 binds with RNAIII thus repressing the expression of α-haemolysin(hla). The Sau-41 and RNAIII complex was probably degraded by RNase III, thus down-regulating the expression of δ-toxin (hld). (c) When Sau-41 was knockdown, RNAIII was preserved and could interact with hla 5’UTR. hla expression was upregulated because its SD sequence was available for ribosome binding.
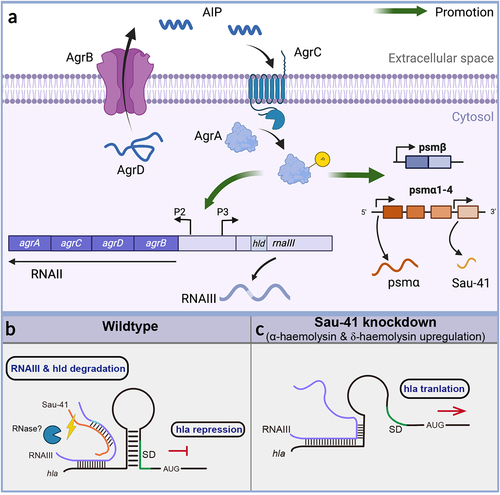
According to our in vivo infection model, the increased bacterial burden in the Sau-41kd group and the decreased bacterial burden in the sau41+ group were more significant in bone tissue than in its surrounding soft tissue (). Combined with the differences in osteolysis that we observed from the μCT data (), we hypothesized that Sau-41 was critical for osteolysis pathogenesis. It is worth mentioning that although our in vivo study demonstrated Sau-41 as a virulence repressor and could alleviate osteolysis, this does not necessarily mean that Sau-41 overexpression is a protective factor for the host. There have been plenty of reports showing that low-virulence strains (e.g. SCV, agr mutants, and CC30 clonal complex [Citation37–39] in clinical practice are always difficult to identify and prone to cause persistent infection. For example, the single-nucleotide polymorphisms (SNPs) that abrogate the production of hla are evolutionary evidence for the CC30 clonal complex favouring niche adaptation [Citation37]. We believe there exists a possibility that Sau-41 provides S. aureus with better adaptability in the host environment with specific tropism in bone tissue rather than soft tissue.
Over the past decades, many studies have focused on PSMs and revealed their roles in immune cell chemotaxis, cytolysis, and biofilm structuring [Citation40,Citation41]. However, among the four amphipathic α helix peptides, PSMα4 is less virulent because of its noncytolytic character compared with its counterparts [Citation41]. In this study, we found an overlapping genomic region of Sau-41 and psmα4. Furthermore, the identification of a TSS between psmα3 and psmα4 in the previous study further strengthens the evidence supporting Sau-41 as a bona fide sRNA [Citation26]. This interesting finding indicates that the long-studied PSMα4 might be functional in its RNA form rather than the translated peptide. It is worth noting that in a recently published work that investigated the S. aureus RNA‒RNA interaction map using the RNaseIII CLASH technique [Citation42], we unexpectedly found Sau-41-RNAIII binding information by exploring their sequencing data. This finding indicated that the Sau-41-RNAIII complex could be processed by endoribonuclease III (RNaseIII). However, this hypothesis needs to be further verified by biological experiments.
Moreover, we acknowledge the following limitations in our study. First, the sRNA annotation information used for read mapping was derived from the SRD database [Citation6], and some entries were annotated with prediction tools. It has been reported that some of the predicted sRNAs are not transcribed, and some are peptide encoding [Citation43]. Thus, the mistaken inclusion of false sRNAs due to technical reasons could impair the performance of the ICA method. Second, although we used northern blotting to quantify Sau-41 transcription, we were not able to determine the sequence of Sau-41 experimentally. Since the sequence of Sau-41 was derived from prediction tools in its first report, more studies are required to verify its sequence in future research.
In summary, we propose a pangenome-based method for prokaryote RNA-seq data analysis. By incorporating sRNA information into the ICA analysis pipeline, we discovered a virulence-regulating sRNA and validated its function experimentally. Our findings also offered the prospect of ICA in sRNA discovery.
Authors’ contributions
J.Y. and M.L. contributed to the concept of the study. M.L. collected the publicly available RNA-seq data. J.Y. performed RNA-seq analysis and ICA. J.W. and M.L performed molecular cloning. M.H., Y.H. and B.W. performed animal studies. J.Y. and F.J. contributed to μCT analysis. J.Y. and M.L contributed to Northern blot and EMSA. Y.H. performed qPCR experiment. P.H. contributed to data interpretation. J.L. wrote the manuscript. J.T and H.S. contributed to study design, manuscript editing and revision. J. Y., M.L. and J.W. contributed equally to the study. All authors contributed to the article and approved the submitted version.
Availability of data and materials
All of the accession IDs of the RNA-seq data involved in this study are listed in Table S1.
Ethics approval
All animal procedures were approved by the Ethics Committees of Shanghai Jiao Tong University Affiliated Sixth People’s. Human body fluid collection was approved by the Ethics Committee of Sixth Peoples Hospital affiliated with Shanghai Jiao Tong University.
Supplemental Material
Download Zip (4.2 MB)Disclosure statement
No potential conflict of interest was reported by the authors.
Supplemental data
Supplemental data for this article can be accessed online at https://doi.org/10.1080/21505594.2023.2228657.
Additional information
Funding
References
- Kwiecinski JM, Horswill AR. Staphylococcus aureus bloodstream infections: pathogenesis and regulatory mechanisms. Curr Opin Microbiol. 2020;53:51–15. doi: 10.1016/j.mib.2020.02.005
- Lee AS, de Lencastre H, Garau J, et al. Methicillin-resistant Staphylococcus aureus. Nat Rev Dis Primers. 2018;4(1):18033. doi: 10.1038/nrdp.2018.33
- Urish KL, Cassat JE, Ottemann KM. Staphylococcus aureus osteomyelitis: bone, bugs, and surgery. Infect Immun. 2020;88:88. doi: 10.1128/IAI.00932-19
- Menard G, Silard C, Suriray M, et al. Thirty years of Srna-mediated regulation in Staphylococcus aureus: from initial discoveries to in vivo biological implications. Int J Mol Sci. 2022;23(13):7346. doi: 10.3390/ijms23137346
- Sorensen HM, Keogh RA, Wittekind MA, et al. Reading between the lines: utilizing RNA-Seq data for global analysis of sRnas in Staphylococcus aureus. mSphere. 2020;5(4):e00439–20. doi: 10.1128/mSphere.00439-20
- S M, A Y, M T, et al. SRD: a Staphylococcus regulatory RNA database. RNA. 2015;21(5):1005–1017. doi: 10.1261/rna.049346.114
- Busch A, Richter AS, Backofen R. IntaRNA: efficient prediction of bacterial sRNA targets incorporating target site accessibility and seed regions. Bioinformatics. 2008;24(24):2849–2856. doi: 10.1093/bioinformatics/btn544
- Eggenhofer F, Tafer H, Stadler PF, et al. Rnapredator: fast accessibility-based prediction of sRNA targets. Nucleic Acids Res. 2011;39:W149–154. doi: 10.1093/nar/gkr467
- Kery MB, Feldman M, Livny J, et al. TargetRNA2: identifying targets of small regulatory RNAs in bacteria. Nucleic Acids Res. 2014;42:W124–129. doi: 10.1093/nar/gku317
- Greener JG, Kandathil SM, Moffat L, et al. A guide to machine learning for biologists. Nat Rev Mol Cell Biol. 2022;23(1):40–55. doi: 10.1038/s41580-021-00407-0
- Lin K, Li L, Dai Y, et al. A comprehensive evaluation of connectivity methods for L1000 data. Brief Bioinform. 2020;21(6):2194–2205. doi: 10.1093/bib/bbz129
- Poudel S, Tsunemoto H, Seif Y, et al. Revealing 29 sets of independently modulated genes in Staphylococcus aureus, their regulators, and role in key physiological response. Proc Natl Acad Sci, USA. 2020;117(29):17228–17239. doi: 10.1073/pnas.2008413117
- Rychel K, Decker K, Sastry AV, et al. iModulondb: a knowledgebase of microbial transcriptional regulation derived from machine learning. Nucleic Acids Res. 2021;49(D1):D112–D120. doi: 10.1093/nar/gkaa810
- Rajput A, Tsunemoto H, Sastry AV, et al. Machine learning from Pseudomonas aeruginosa transcriptomes identifies independently modulated sets of genes associated with known transcriptional regulators. Nucleic Acids Res. 2022;50(7):3658–3672. doi: 10.1093/nar/gkac187
- Sastry AV, Gao Y, Szubin R, et al. The Escherichia coli transcriptome mostly consists of independently regulated modules. Nat Commun. 2019;10(1):5536. doi: 10.1038/s41467-019-13483-w
- D W, B F, Ra N. panX: pan-genome analysis and exploration. Nucleic Acids Res. 2018;46:e5–e5. doi: 10.1093/nar/gkx977
- Seemann T. Prokka: rapid prokaryotic genome annotation. Bioinformatics. 2014;30(14):2068–2069. doi: 10.1093/bioinformatics/btu153
- Patro R, Duggal G, Love MI, et al. Salmon provides fast and bias-aware quantification of transcript expression. Nat Methods. 2017;14(4):417–419. doi: 10.1038/nmeth.4197
- McConn JL, Lamoureux CR, Poudel S, et al. Optimal dimensionality selection for independent component analysis of transcriptomic data. BMC Bioinf. 2021;22(1):584. doi: 10.1186/s12859-021-04497-7
- Huerta-Cepas J, Forslund K, Coelho LP, et al. Fast genome-wide functional annotation through orthology assignment by eggNOG-Mapper. Mol Biol Evol. 2017;34:2115–2122. doi: 10.1093/molbev/msx148
- Novichkov PS, Laikova ON, Novichkova ES, et al. RegPrecise: a database of curated genomic inferences of transcriptional regulatory interactions in prokaryotes. Nucleic Acids Res. 2010;38:D111–118. doi: 10.1093/nar/gkp894
- Zhao C, Shu X, Sun B, et al. Construction of a gene knockdown system based on catalytically inactive (“Dead”) Cas9 (dCas9) in Staphylococcus aureus. Appl environ microbiol. 2017;83(12):e00291–17. doi: 10.1128/AEM.00291-17
- Yu J, Jiang F, Zhang F, et al. Thermonucleases contribute to staphylococcus aureus biofilm formation in implant-associated infections-a redundant and complementary story. Front Microbiol. 2021;12:687888. doi: 10.3389/fmicb.2021.687888
- Le Huyen KB, Gonzalez CD, Pascreau G, et al. A small regulatory RNA alters Staphylococcus aureus virulence by titrating RNAIII activity. Nucleic Acids Res. 2021;49(18):10644–10656. doi: 10.1093/nar/gkab782
- Prados J, Linder P, Redder P. TSS-EMOTE, a refined protocol for a more complete and less biased global mapping of transcription start sites in bacterial pathogens. BMC Genomics. 2016;17(1):849. doi: 10.1186/s12864-016-3211-3
- Choe D, Szubin R, Dahesh S, et al. Genome-scale analysis of Methicillin-resistant Staphylococcus aureus USA300 reveals a tradeoff between pathogenesis and drug resistance. Sci Rep. 2018;8(1):2215. doi: 10.1038/s41598-018-20661-1
- Bronesky D, Wu Z, Marzi S, et al. Staphylococcus aureus RNAIII and its regulon link quorum sensing, stress responses, metabolic adaptation, and regulation of virulence gene expression. Annu Rev Microbiol. 2016;70:299–316. doi: 10.1146/annurev-micro-102215-095708
- Morfeldt E, Taylor D, von Gabain A, et al. Activation of alpha-toxin translation in Staphylococcus aureus by the trans-encoded antisense RNA, RNAIII. Embo J. 1995;14(18):4569–4577. doi: 10.1002/j.1460-2075.1995.tb00136.x
- He L, Zhang F, Jian Y, et al. Key role of quorum-sensing mutations in the development of Staphylococcus aureus clinical device-associated infection. Clin Transl Med. 2022;12(4):e801. doi: 10.1002/ctm2.801
- Poudel S, Hefner Y, Szubin R, et al. Coordination of CcpA and CodY regulators in Staphylococcus aureus USA300strains. mSystems. 2022;7:e00480–22.
- Garrison E, Sirén J, Novak AM, et al. Variation graph toolkit improves read mapping by representing genetic variation in the reference. Nat Biotechnol. 2018;36(9):875–879. doi: 10.1038/nbt.4227
- Chen N-C, Solomon B, Mun T, et al. Reference flow: reducing reference bias using multiple population genomes. Genome Biol. 2021;22(1):8. doi: 10.1186/s13059-020-02229-3
- Abu-Qatouseh LF, Chinni SV, Seggewiß J, et al. Identification of differentially expressed small non-protein-coding RNAs in Staphylococcus aureus displaying both the normal and the small-colony variant phenotype. J Mol Med. 2010;88(6):565–575. doi: 10.1007/s00109-010-0597-2
- He L, Le KY, Khan BA, et al. Resistance to leukocytes ties benefits of quorum sensing dysfunctionality to biofilm infection. Nat Microbiol. 2019;4(7):1114–1119. doi: 10.1038/s41564-019-0413-x
- Vuong C, Kocianova S, Yao Y, et al. Increased colonization of indwelling medical devices by quorum-sensing mutants of Staphylococcus epidermidis in vivo. J Infect Dis. 2004;190(8):1498–1505. doi: 10.1086/424487
- Fowler, Jr. VG, Sakoulas G, McIntyre LM, et al. Persistent bacteremia due to methicillin-resistant Staphylococcus aureus infection is associated with agr dysfunction and low-level in vitro resistance to thrombin-induced platelet microbicidal protein. J Infect Dis. 2004;190(6):1140–1149. doi: 10.1086/423145
- McGavin MJ, Arsic B, Nickerson NN. Evolutionary blueprint for host- and niche-adaptation in Staphylococcus aureus clonal complex CC30. Front Cell Infect Microbiol. 2012;2:48. doi: 10.3389/fcimb.2012.00048
- George SE, Hrubesch J, Breuing I, et al. Oxidative stress drives the selection of quorum sensing mutants in the Staphylococcus aureus population. Proc Natl Acad Sci U S A. 2019;116(38):19145–19154. doi: 10.1073/pnas.1902752116
- Zhou S, Rao Y, Li J, et al. Staphylococcus aureus small-colony variants: formation, infection, and treatment. Microbiol Res. 2022;260:127040. doi: 10.1016/j.micres.2022.127040
- Cheung GYC, Joo H-S, Chatterjee SS, et al. Phenol-soluble modulins–critical determinants of staphylococcal virulence. FEMS Microbiol Rev. 2014;38(4):698–719. doi: 10.1111/1574-6976.12057
- Otto M. Phenol-soluble modulins. Int J Med Microbiol. 2014;304(2):164–169. doi: 10.1016/j.ijmm.2013.11.019
- McKellar SW, Ivanova I, Arede P, et al. RNase III CLASH in MRSA uncovers sRNA regulatory networks coupling metabolism to toxin expression. Nat Commun. 2022;13:3560. doi: 10.1038/s41467-022-31173-y
- Liu W, Rochat T, Toffano-Nioche C, et al. Assessment of Bona Fide sRnas in Staphylococcus aureus. Front Microbiol. 2018;9. doi: 10.3389/fmicb.2018.00228