ABSTRACT
Cryptococcus neoformans is an environmental yeast that primarily affects immunocompromised individuals, causing respiratory infections and life-threatening meningoencephalitis. Treatment is complicated by limited antifungal options, with concerns such as adverse effects, dose-limiting toxicity, blood–brain barrier permeability, and resistance development, emphasizing the critical need to optimize and expand current treatment options against invasive cryptococcosis. Galleria mellonella larvae have been introduced as an ethical intermediate for in vivo testing, bridging the gap between in vitro antifungal screening and mouse studies. However, current infection readouts in G. mellonella are indirect, insensitive, or invasive, which hampers the full potential of the model. To address the absence of a reliable non-invasive method for tracking infection, we longitudinally quantified the cryptococcal burden in G. mellonella using bioluminescence imaging (BLI). After infection with firefly luciferase-expressing C. neoformans, the resulting bioluminescence signal was quantitatively validated using colony-forming unit analysis. Longitudinal comparison of BLI to health and survival analysis revealed increased sensitivity of BLI in discriminating cryptococcal burden during early infection. Furthermore, BLI improved the detection of treatment efficacy using first-line antifungals, thereby benchmarking this model for antifungal testing. In conclusion, we introduced BLI as a real-time, quantitative readout of cryptococcal burden in G. mellonella over time, enabling more sensitive and reliable antifungal screening.
Introduction
Cryptococcus neoformans (CN) is an opportunistic pathogenic yeast responsible for severe diseases and high mortality among HIV-infected individuals. Upon inhalation, environmental CN cells or spores initially cause pulmonary cryptococcosis; however, under immunosuppressive conditions, they can disseminate to the central nervous system (CNS) and cause life-threatening cryptococcal meningitis [Citation1]. Cryptococcal meningitis is the leading cause of meningitis in patients with HIV and is estimated to account for 19% of AIDS-related deaths globally [Citation2]. The recommended treatment consists of a combination of fluconazole, flucytosine, and amphotericin B, but is limited by BBB permeability, adverse effects, dose-limiting toxicity, and inaccessibility in resource-limited settings [Citation3]. Moreover, reduced susceptibility of CN isolates to fluconazole, the most commonly used antifungal against cryptococcosis, has been described, further limiting treatment options [Citation4,Citation5]. Thus, there is an urgent need to expand and optimize current treatment options for invasive cryptococcosis [Citation3,Citation6]. This was highlighted by a recent publication of the WHO fungal priority pathogen list, in which CN received the highest overall combined rank based on research and development priorities and public health importance [Citation7].
Over the last decades, Galleria mellonella caterpillars have gained popularity as experimental and preclinical infection models, among others, for antifungal (synergy) testing against cryptococcosis [Citation8–13]. G. mellonella has important advantages over more commonly used in vivo models, such as rodents, because they are cost- and time-efficient, easy to handle, and do not require specialized housing equipment or ethical approval because they lack nociceptors and are thus insensitive to pain [Citation14]. Another unique advantage G. mellonella has compared to other popular invertebrate models, is that they can be kept at 37°C, enabling the activation of temperature-dependent virulence factors in human pathogens such as C. neoformans [Citation15]. Therefore, it is a convenient model to test many different antifungal conditions in vivo before potentially moving on to more in-depth investigations in rodents, thereby complying with the 3 R’s (replacement, reduction, and refinement) of ethical animal research [Citation16,Citation17]. In vivo screening in G. mellonella is thus a significant step up from initial screening in in vitro systems, as G. mellonella offers in vivo therapeutic toxicity assessment and antifungal efficacy testing in the presence of an innate immune system, which in addition allows preliminary investigation of host-pathogen responses [Citation18]. Moreover, the virulence and toxicity of fungal and bacterial strains in G. mellonella translate well towards mice, as shown by models of Aspergillus fumigatus, Candida albicans, and multiple bacterial strains [Citation19–23], and antibiotic efficacy and dose in G. mellonella correlated with human data [Citation23]. In a therapeutic study with propolis-loaded nanoparticles, G. mellonella was successfully used for in vivo validation between in vitro and mouse studies [Citation24]. Taken together, these data highlight the added value of this model in preclinical antifungal testing pipelines.
Driven by its numerous advantages, G. mellonella is with good reasons emerging as an infection model. As it is a relatively recent model, the available readouts for fungal burden and antifungal efficacy are limited. On the one hand, there are longitudinal, non-invasive readouts based on survival and descriptive health assessment [Citation25]. While these are the most commonly used readouts in antifungal efficacy studies, survival and health scoring are indirect and observer-dependent measures which are binary or semi-quantitative. Moreover, the widespread lack of commercial research-grade larvae and standardized rearing protocols increases the variability of these health-based readouts, thereby preventing direct comparisons in the literature [Citation17]. On the other hand, there are invasive endpoint readouts such as colony-forming units (CFU), currently the gold standard for fungal burden quantification, and qPCR [Citation26]. While these are quantitative readouts, they have their own limitations and lack the longitudinal aspect, which is crucial for understanding the dynamics of infection and treatment. Therefore, the study of cryptococcosis in G. mellonella would benefit from a novel readout of fungal burden that is quantitatively correct but also non-invasive, allowing direct longitudinal follow-up of cryptococcal infection and treatment effects over time, independent of overall larval health state and off-target toxicity. Recently, we optimized bioluminescence imaging (BLI) for real-time quantitative readout of Aspergillus fumigatus, a filamentous fungus, in G. mellonella [Citation27]. Similarly, BLI has the potential to be implemented as a fungal readout in G. mellonella for yeasts, such as C. neoformans.
In this study, we propose BLI as a non-invasive quantitative readout of cryptococcal burden in G. mellonella over time. We aimed to develop the first BLI-based G. mellonella model of cryptococcosis and compare the sensitivity of in vivo BLI with the current standard longitudinal readouts in terms of cryptococcal load discrimination and antifungal detection, as well as with CFU for quantitative validation. Finally, we benchmarked the model for antifungal screening using first-line antifungal agents against C. neoformans.
Materials and methods
Bioluminescent Cryptococcus neoformans strain and fungal culture
We used a previously validated C. neoformans (CN) KN99α strain expressing codon-optimized red-shifted firefly luciferase (KN99α-CnFLuc) [Citation28]. KN99α-CnFLuc was first grown on Sabouraud agar for 2–4 d and then transferred to liquid Sabouraud medium for 2–3 d at 30°C. Fungal cells were harvested by centrifugation and washed twice with PBS (Dulbecco’s phosphate-buffered saline, Gibco, Paisley, UK). The number of cells was counted using a Neubauer haemocytometer (Mariënfield Superior, Lauda-Köningshofen, Germany) and diluted to the required concentration in PBS [Citation28]. The cell count in the final suspension was confirmed by in vitro bioluminescence imaging (BLI) and colony forming unit (CFU) plating, as described below.
In vitro and ex vivo bioluminescence imaging
In vitro BLI was performed on the fungal inoculum, and ex vivo BLI on larval homogenates to confirm the relative fungal cell count. Larval homogenates were prepared by placing individual larvae in 600 µl of PBS and homogenizing them using a tissue homogenizer. Ten-fold serial dilutions of the fungal inoculum and larval homogenates were then made in a black 96-well plate (CliniplateTM, Thermo Scientific, Denmark) and 10% d-luciferin potassium salt (1.25 mg/ml in PBS, Promega, USA) was added. The BLI signal was read using an IVIS Spectrum (PerkinElmer, USA) imaging system by acquiring five consecutive images with an exposure time of 30 s (open filter, F/stop1, subject height 0.5 cm, medium binning). Living Image Software (version 4.5.4, PerkinElmer, USA) was used to define regions of interest (ROI) covering each individual well and to calculate the total photon flux (p/s) per well, and peak total fluxes were used for analysis and comparison [Citation27].
Colony-forming units
To determine the absolute viable cell count in the inocula (CFU/ml) and larval homogenates of weighted larvae (CFU/g), 10-fold serial dilutions were prepared in a black 96-well plate (CliniplateTM, Thermo Scientific, Denmark). Fifty microliters of each dilution were plated on Sabouraud agar containing chloramphenicol, incubated at 37°C and counted after 24 and 48 h [Citation27].
Galleria mellonella infection model
Healthy 6th week instar larvae weighing 300 ± 50 mg (in-house bred) with normal movement and no melanization were selected for the experiments. The larvae were randomly assigned to experimental groups (n = 10 per group) and housed individually in 12-well plates to provide sufficient space for normal movement. They were kept in the dark without food at 37°C to mimic human host temperature, for activation of relevant fungal virulence factors. Fungal inocula (10 µL) were administered via the last right proleg into the haemocoel, using a Hamilton® syringe (10 µL, 701SN, 31 G, Switzerland). Larval health score (movement, melanization, and survival; adapted from [Citation25]) was assessed daily for three to five days post infection (). The negative controls were sham-infected with PBS. At 24 h post infection, two predefined larvae per infected group were weighed and homogenized individually (Tissue Master Homogenizer, OMNI International, Tulsa, OK, USA) in 600 µL PBS per larva for fungal quantification by CFU plating and ex vivo BLI [Citation27].
Table 1. Health index scoring system for G. mellonella larvae.
Antifungal treatment
Fluconazole solution (2 mg/ml) for perfusion (FLU, Diflucan®, Pfizer, Brussels) was diluted to obtain final doses of 12 mg/kg and 6 mg/kg in 0.9% sterile saline. For amphotericin B (AMB, Fungizone®, Bristol Myers Squibb, Canada), a stock solution of 5 mg/ml was prepared in sterile AD and adjusted to obtain final doses of 10, 5, and 1 mg/kg in 5% glucose. These calculations were based on an injection volume of 10 µL and average larval weight of 300 mg. All treatments were freshly prepared and administered daily by intra-haemocoel injection alternately in the last left and right prolegs to prevent potential injury by repeated injections in the same proleg. The infected control groups were injected with the treatment vehicle, and the non-infected control groups received the highest treatment dose to assess treatment-related health effects.
In vivo bioluminescence imaging
BLI of live larvae was performed daily from baseline (day 0, before infection) until day five post infection, using an IVIS Spectrum imaging system (PerkinElmer). d-Luciferin was administered daily before every imaging session at 40 µg/g in PBS for optimal tolerability and photon flux dynamics, as previously optimized for Aspergillus fumigatus [Citation27]. Ten microlitres of d-luciferin were injected into the larval haemocoel by alternating the last right or left proleg. They were then transferred to a black 12-well plate with a transparent bottom (IBL Baustoff + Labor GmbH, Austria) at 37°C for 10 min. Bioluminescence light emission was then measured by acquiring five consecutive images with the following settings: open filter, F/stop 1, subject height 0.5 cm, medium binning, and 30 s exposure time per image. Using living Image Software (version 4.5.4), the total photon flux (p/s) per larva was defined through a circular ROI of 2.5 cm diameter covering each well. We verified that any variability in peak photon fluxes among individual experiments that used the same anticipated inoculum size was due to variations in the infectious doses as determined by CFU and in vitro BLI [Citation27].
Statistical analysis
All statistical analyses were performed using GraphPad Prism version 8.0.2 (GraphPad Software, USA). The log-rank (Mantel-Cox) test was used for survival analysis. Longitudinal health scores and log10-transformed in vivo BLI data were analysed by repeated measures or mixed effects two-way ANOVA with Tukey’s correction for multiple comparisons to detect significant differences within an experiment and between groups at defined time points. Pairwise repeated measures or mixed-effects two-way ANOVA tests were performed to compare the slopes (interaction time and group) over time. Dead larvae were excluded from the BLI and health score statistical analysis but were retained in the graph with their last values before death to avoid visual survival bias. The correlation coefficient r was computed using Pearson correlation analysis after confirming normality using the Shapiro-Wilk test. Statistical significance was set at p < 0.05 [Citation27].
Results
Longitudinal BLI sensitively discriminates cryptococcal load in vivo in G. mellonella
For antifungal screening in G. mellonella, it is essential to differentiate between different cryptococcal burdens with high sensitivity over the course of infection. Therefore, the sensitivity of fungal load differentiation was compared between in vivo BLI and the currently available longitudinal readouts for infection assessment, namely, health score and survival. To this end, larvae were infected with 103, 104, 105 or 106 firefly luciferase-expressing CN cells and were assessed by all three readouts at 3 days post-infection (p.i.). Survival and health scores were unable to detect any significant differences between the log10-fold differences in fungal load over 3 days or at individual time points (). In vivo BLI allowed a significant distinction between all cryptococcal doses since the first measured time point (day 1 p.i.), showing a clear inoculum-dependent increase in photon fluxes over time (). Even before quantification, the difference between the groups was visually discernible on the raw images (). In conclusion, in vivo BLI is the only available non-invasive longitudinal readout that sensitively differentiates log10-differences in CN load in real time as early as day 1 p.i. Thus, BLI clearly outperforms survival analysis and health scoring.
Figure 1. In vivo BLI sensitively discriminates longitudinal CN load in G. mellonella where survival and health score do not.

Figure 2. Visual representation of in vivo BLI of G. mellonella groups infected with different CN loads.

BLI is a real-time quantitative measure for cryptococcal burden in vitro, in vivo and ex vivo
To confirm the quantitative nature of BLI in different experimental samples, we performed in vitro BLI of cryptococcal inocula, in vivo BLI of infected G. mellonella larvae, and ex vivo BLI of larval homogenates, and correlated these BLI signals with their corresponding colony-forming unit (CFU) counts, which are currently considered the gold standard for fungal load quantification. In vitro BLI of the different cryptococcal inocula over a range of 103-106 CN per 10 µL correlated excellently with CFU and confirmed the linearity of the inocula before infection (). Cross-sectional comparison of in vivo BLI and CFU on day 1 p.i. showed a very good correlation between both readouts, confirming the quantitative nature of in vivo BLI in G. mellonella larvae (). Finally, the ex vivo BLI signal of larval homogenates on day 1 p.i. also correlated very well with the corresponding CFU counts (). Overall, the good correlation of in vitro, in vivo, and ex vivo BLI with CFU counts validates BLI as a quantitative readout of the cryptococcal burden in different experimental samples. More specifically, this shows that in vivo BLI qualifies as a real-time alternative for labour-intensive and endpoint CFU counts, with the advantage of longitudinal in vivo assessment in G. mellonella larvae.
Figure 3. In vitro, in vivo, and ex vivo BLI signals correlate with their respective CFU counts.
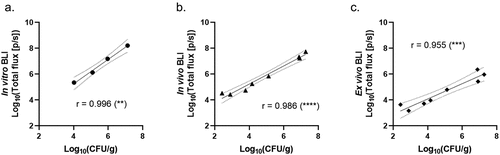
BLI allows improved detection of in vivo antifungal efficacy compared to survival and health readouts
To validate in vivo BLI for antifungal screening and compare its antifungal screening performance with health score and survival readouts, larvae were infected with 105 CN cells and treated daily with different doses of amphotericin B (AMB) or fluconazole (FLU) for 5 days p.i. FLU and AMB were well tolerated in sham-infected larvae with no deterioration in survival or health score compared to vehicle-injected larvae (). Survival and health scores failed to distinguish AMB-treated groups from vehicle-treated controls, nor could they differentiate between different doses of AMB (). With BLI, on the other hand, the in vivo photon fluxes of the infected vehicle-treated group were significantly higher than all treated groups from day 3 p.i. onwards, indicating treatment effect (). Moreover, significant dose-dependent differences in photon fluxes were visible between all treated groups, again from day 3 onwards (). The longitudinal treatment effect of FLU compared to that of vehicle could be detected by survival, health score, and in vivo BLI. However, only in vivo BLI showed significant treatment effects as early as 1 day p.i., while the corresponding health scores showed no significance until the endpoint on day 5 p.i. No dose-dependent treatment differences of FLU were seen in any of the readouts (). Altogether, in vivo BLI outperforms current longitudinal readouts both in detecting dose-dependent treatment effects over time and in sensitive early detection.
Figure 4. In vivo BLI outperforms survival and health score as a method for antifungal screening in G. mellonella.
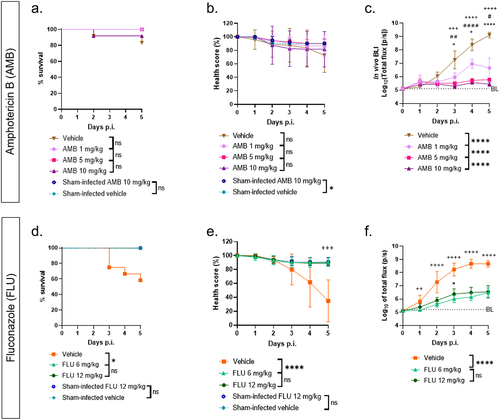
Discussion
In this study, we developed the first Galleria mellonella model of cryptococcosis with real-time quantitative follow-up of the cryptococcal burden within the same larvae over time using in vivo BLI. By benchmarking this tool for first-line clinical antifungals, we unlock the full potential of G. mellonella as an intermediate host and ethical-burden-free alternative to mouse infection models for in vivo antifungal efficacy screening against cryptococcosis. In comparison with the currently used longitudinal readouts of infection in G. mellonella, namely survival and health scoring, in vivo BLI was the only readout capable of detecting (log10) differences in cryptococcal burden over a period of 3 days post-infection (p.i.), with significant differences appearing as soon as 1 day p.i. Therefore, BLI convincingly outperformed the sensitivity of survival and health scores as measures of fungal burden and infection development. Furthermore, good correlations were observed between golden-standard CFU counts and in vitro, in vivo and ex vivo BLI measurements, confirming the quantitative reliability of BLI in this model. Additionally, we validated in vivo BLI as a sensitive readout for preclinical antifungal testing, demonstrating its capacity to detect dose-dependent treatment responses to AMB and FLU more sensitively and earlier than survival and health scoring.
Although survival and health scoring are by far the most popular readouts to report antifungal efficacy, they showed insufficient sensitivity in our model despite using a broad range of fungal inocula and antifungal doses. Even higher fungal inocula or longer follow-up times might be necessary to reveal health differences between the tested conditions, especially for survival readouts that are inherently insensitive to sublethal changes in the fungal load. Moreover, since health readouts are not directly measuring fungal burden but only the subsequent health effect of fungal burden, treatment effects will be detected with a delay in time compared to changes in fungal burden. Health effects are also more prone to inter-larva variation, leading to higher standard deviations and thus lower statistical power to detect treatment effects. In addition, health scores vary greatly depending on the initial quality and health of the larvae before infection, because they are a proxy of non-specific health-compromising effects. As conditions may vary among laboratories, accurately comparing health-based outcomes in the literature is nearly impossible. This is further complicated by the lack of standardized rearing protocols or research-grade suppliers of G. mellonella at this moment. In sharp contrast to these health-based readouts, in vivo BLI performed excellently at distinguishing the different (anti)fungal conditions within the first days p.i., even at non-lethal or non-health-affecting fungal loads, thereby showing a broader application potential for optimizing novel or current antifungal treatments. Also, BLI is regarded as a measure of viable fungal load, as the light-producing interaction between luciferase and luciferin requires ATP and oxygen to be present. As ATP and oxygen deplete fast upon death of the fungal cell, no bioluminescent signal will be generated from dead or metabolically inactive fungi. Moreover, BLI has the advantage of being a real-time quantitative measure of cryptococcal burden with demonstrated capabilities to replace labour-intensive CFU analysis at in vitro, ex vivo and especially in vivo levels, thereby allowing real-time dynamic investigations of fungal load in all larvae without the need for cross-sectional sacrifices. A limitation of BLI is its lower detection limit around 104–5 photons per second, whereas CFU can intrinsically detect even smaller amounts of fungi present. Also, in vivo BLI is only a quantitatively correct proxy for fungal burden in live larvae, possibly due to the limited oxygen supply to the fungi inside a dead larva, thereby preventing luciferase to catalyse luciferin oxidation (and thereby produce photons).
We have confirmed the advantages of in vivo BLI to sensitively monitor the fungal burden in G. mellonella in other fungal pathogens, such as the filamentous fungus Aspergillus fumigatus [Citation27]. In this infection model, we similarly reported a higher sensitivity of in vivo BLI for detecting differences in fungal load and antifungal treatment compared to survival and health scores, as well as good correlations between in vivo BLI and CFU counts within defined dynamic ranges [Citation27]. This underscores the versatility of the model in addressing fungal infections and shows its potential as a valuable tool for studying and optimizing treatments across various fungal pathogens.
We consider BLI-supported fungal burden evaluation in Galleria mellonella to have the potential to become a widely adopted tool for standardized and ethical-burden-free antifungal efficacy screening under in vivo conditions. While the availability of a bioluminescent fungal strain is a prerequisite that can pose a limitation to certain specific applications, such as antifungal testing against specific strains or clinical isolates, an increasing number of bioluminescent fungi are becoming available, and growing expertise in fungal transformation with reporter genes facilitates the development of novel bioluminescent strains [Citation28–34]. Moreover, our methodology is not necessarily restricted to specialized imaging laboratories, as it was previously shown that a standard luminescence plate reader can also be used to detect in vivo photon fluxes in G. mellonella [Citation35]. Alternatively, the imaging protocol for G. mellonella can be adapted to use fluorescent fungal strains, or in the case of bacteria, strains with a lux operon that encodes both the enzyme and the substrate for the light producing reaction. The advantage of those methods is that no luciferin injections are needed. However, we anticipate fluorescence imaging (FLI) to have a lower signal-to-noise ratio, which could decrease the sensitivity in distinguishing cryptococcal burdens compared to BLI [Citation36]. Moreover, BLI has better tissue penetration than FLI, which we consider especially relevant for translation of experiments in G. mellonella towards BLI-compatible mouse models of infection [Citation28]. Indeed, longitudinal in vivo BLI has previously been implemented in a mouse model of cryptococcosis, allowing non-invasive tracking of fungal dissemination from the lung to the brain over time [Citation28]. While preclinical antifungal testing in such imaging-compatible rodent models is still an indispensable step, intermediate testing in G. mellonella larvae can help narrow down and identify the number of relevant testing conditions in rodents, and therefore crucially reduce the ethical and financial impact. By introducing BLI of cryptococcosis in G. mellonella and using the same thoroughly characterized bioluminescent C. neoformans strain used in our BLI-based cryptococcosis mouse model [Citation28], we did not only increase the accuracy of antifungal testing in G. mellonella but also facilitated translation between G. mellonella and mouse models of Cryptococcus infection.
Furthermore, we benchmarked the translational potential of our model by achieving successful antifungal therapeutic effects upon administration of clinically relevant doses of amphotericin B (Fungizone®; 0.5–1 mg/kg per day), and fluconazole (Diflucan®; 6–12 mg/kg per day), based on the recommended paediatric intravenous doses [Citation37]. Our results show the capacity of in vivo BLI to not only detect treatment effects but also define a range of clinically pertinent doses in G. mellonella, thereby proving its value as an intermediate preclinical screening tool that contributes to the 3 R’s in animal research.
In conclusion, we successfully implemented BLI as a superior quantitative readout of cryptococcal burden in G. mellonella over time. We believe that the incorporation of in vivo BLI as a longitudinal readout of fungal burden in G. mellonella models of cryptococcosis will improve the sensitivity of antifungal efficacy detection compared to survival, health score, or CFU analysis, thereby contributing to a more successful and ethical translation of in vitro antifungal hits to animal research in rodents towards ultimately improving patient care.
Author contributions
Conceptualization: E.V., G.V.V.; data curation: E.V., L.V., L.M.; formal analysis: E.V., L.V., L.M.; funding acquisition, G.V.V.; methodology, E.V., A.R.S., G.V.V.; supervision: G.V.V., A.R.S., K.L.; writing – original draft: E.V.; writing – review and editing: E.V., L.V., L.M., A.R.S., K.L., G.V.V. All authors have read and approved the final version of the manuscript.
Acknowledgements
We thank Prof. Rob Lavigne and his team for teaching us how to work with G. mellonella larvae and Prof. Charles Van der Henst, Julien Brillard, Gaetan Clabots, and François Uytterhoeven for their help with starting up our G. mellonella rearing. Bioluminescence imaging was performed at the Molecular Small Animal Imaging Center (MoSAIC, KU Leuven).
Disclosure statement
K.L. received consultancy fees from MRM Health and MSD, speaker fees from Pfizer and Gilead, and a service fee from Thermo Fisher Scientific and TECOmedical, not related to this work. The other authors have no conflicts of interest to declare.
Data Availability statement
Data are available upon request.
Additional information
Funding
References
- Denham ST, Brown JCS. Mechanisms of pulmonary escape and dissemination by Cryptococcus neoformans. J Fungi Basel Switz. 2018 Feb 17;4(1):25. doi: 10.3390/jof4010025
- Rajasingham R, Govender NP, Jordan A, et al. The global burden of HIV-associated cryptococcal infection in adults in 2020: a modelling analysis. Lancet Infect Dis. 2022 Dec;22(12):1748–10. doi: 10.1016/S1473-3099(22)00499-6
- de SC, Wirth C, F LL, et al. New approaches for cryptococcosis treatment. Microorganisms. 2020 Apr 23;8(4):613. doi: 10.3390/microorganisms8040613
- Stone NRH, Rhodes J, Fisher MC, et al. Dynamic ploidy changes drive fluconazole resistance in human cryptococcal meningitis. J Clin Invest. 2019 Jan 28;129(3):999–1014. doi: 10.1172/JCI124516
- Bongomin F, Oladele RO, Gago S, et al. A systematic review of fluconazole resistance in clinical isolates of Cryptococcus species. Mycoses. 2018 May;61(5):290–297. doi: 10.1111/myc.12747
- Stott KE, Loyse A, Jarvis JN, et al. Cryptococcal meningoencephalitis: time for action. Lancet Infect Dis. 2021 Sep;21(9):e259–71. doi: https://doi.org/10.1016/S1473-3099(20)30771-4
- WHO. Fungal priority pathogens list to guide research, development and public health action. Geneva: World Health Organization; 2022. Licence: CC BY-NC-SA 3.0 IGO.
- De Jesus DFF, De Freitas ALD, De Oliveira IM, et al. Organoselenium has a potent fungicidal effect on Cryptococcus neoformans and inhibits the virulence factors. Antimicrob Agents Chemother. 2023 Feb 23.
- Xin C, Wang F, Zhang J, et al. Secretions from Serratia marcescens inhibit the growth and biofilm formation of Candida spp. And Cryptococcus neoformans. J Microbiol. 2023 Feb;61(2):221–232. doi: 10.1007/s12275-023-00037-5
- Wang T, Pan M, Xiao N, et al. In vitro and in vivo analysis of monotherapy and dual therapy with ethyl caffeate and fluconazole on virulence factors of Candida albicans and systemic candidiasis. J Glob Antimicrob Resist. 2021 Dec;27:253–266. doi: 10.1016/j.jgar.2021.10.005
- Andriani GM, Morguette AEB, Spoladori LFA, et al. Antifungal Combination of Ethyl Acetate Extract of Poincianella pluviosa (DC.) L. P. Queiros Stem Bark With Amphotericin B in Cryptococcus neoformans. Front Microbiol. 2021 Jun 10;12:660645. doi: 10.3389/fmicb.2021.660645
- Jemel S, Guillot J, Kallel K, et al. Galleria mellonella for the Evaluation of Antifungal Efficacy against Medically Important Fungi, a Narrative Review. Microorganisms. 2020 Mar 11;8(3):390. doi: 10.3390/microorganisms8030390
- Kong Q, Cao Z, Lv N, et al. Minocycline and Fluconazole Have a Synergistic Effect Against Cryptococcus neoformans Both in vitro and in vivo. Front Microbiol. 2020 May 5;11:836. doi: 10.3389/fmicb.2020.00836
- Eisemann CH, Jorgensen WK, Merritt DJ, et al. Do insects feel pain? — a biological view. Experientia. 1984 Feb;40(2):164–167. doi: 10.3390/jof4010025
- Ménard G, Rouillon A, Cattoir V, et al. Galleria mellonella as a Suitable Model of Bacterial Infection: Past, Present and Future. Front Cell Infect Microbiol. 2021;11:782733. doi: 10.3389/fcimb.2021.782733
- Tsai CJY, Loh JMS, Proft T. Galleria mellonella infection models for the study of bacterial diseases and for antimicrobial drug testing. Virulence. 2016 Apr 2;7(3):214–229. doi: 10.1080/21505594.2015.1135289
- Serrano I, Verdial C, Tavares L, et al. The virtuous galleria mellonella model for scientific experimentation. Antibiotics. 2023 Mar 3;12(3):505. doi: 10.3390/antibiotics12030505
- Curtis A, Binder U, Kavanagh K. Galleria mellonella Larvae as a Model for Investigating Fungal—Host Interactions. Front Fungal Biol. 2022 Apr 26;3:893494. doi: 10.3389/ffunb.2022.893494
- Slater JL, Gregson L, Denning DW, et al. Pathogenicity of aspergillus fumigatus mutants assessed in galleria mellonella matches that in mice. Med Mycol. 2011 Apr;49(S1):S107–13. doi: 10.3109/13693786.2010.523852
- Brennan M, Thomas DY, Whiteway M, et al. Correlation between virulence of Candida albicans mutants in mice and galleria mellonella larvae. FEMS Immunol Med Microbiol. 2002 Oct;34(2):153–157. doi: 10.1111/j.1574-695X.2002.tb00617.x
- Jander G, Rahme LG, Ausubel FM. Positive correlation between virulence of Pseudomonas aeruginosa mutants in mice and insects. J Bacteriol. 2000 Jul;182(13):3843–3845. doi: 10.1128/JB.182.13.3843-3845.2000
- Olsen RJ, Watkins ME, Cantu CC, et al. Virulence of serotype M3 group a streptococcus strains in wax worms (galleria mellonella larvae). Virulence. 2011 Mar;2(2):111–119. doi: 10.4161/viru.2.2.14338
- Ignasiak K, Maxwell A. Galleria mellonella (greater wax moth) larvae as a model for antibiotic susceptibility testing and acute toxicity trials. BMC Res Notes. 2017 Aug 29;10(1):428. doi: 10.1186/s13104-017-2757-8
- Thammasit P, Tharinjaroen CS, Tragoolpua Y, et al. Targeted propolis-loaded poly (butyl) cyanoacrylate nanoparticles: an alternative drug delivery tool for the treatment of cryptococcal meningitis. Front Pharmacol. 2021 Aug 20;12:723727. doi: 10.3389/fphar.2021.723727
- Loh JMS, Adenwalla N, Wiles S, et al. Galleria mellonella larvae as an infection model for group A streptococcus. Virulence. 2013 Jul 1;4(5):419–428. doi: 10.4161/viru.24930
- Resendiz-Sharpe A, Van Holm W, Merckx R, et al. Quantitative PCR Effectively Quantifies Triazole-Susceptible and Triazole-Resistant Aspergillus fumigatus in Mixed Infections. J Fungi. 2022 Oct 25;8(11):1120. doi: 10.3390/jof8111120
- Vanhoffelen E, Michiels L, Brock M, et al. Powerful and Real-Time Quantification of Antifungal Efficacy against Triazole-Resistant and -Susceptible Aspergillus fumigatus Infections in Galleria mellonella by Longitudinal Bioluminescence Imaging. Microbiol spectr. 2023 Aug 17;11(4):e0082523. doi: 10.1128/spectrum.00825-23
- Vanherp L, Ristani A, Poelmans J, et al. Sensitive bioluminescence imaging of fungal dissemination to the brain in mouse models of cryptococcosis. Dis Model Mech. 2019 Jun 1;12(6):dmm039123. doi: 10.1242/dmm.039123
- Papon N, Courdavault V, Lanoue A, et al. Illuminating fungal infections with bioluminescence. PLoS Pathog. 2014 Jul;10(7):e1004179. doi: 10.1371/journal.ppat.1004179
- Cruz-Leite VR M, Tomazett MV, de Curcio J S, et al. Bioluminescence imaging in Paracoccidioides spp.: A tool to monitor the infectious processes. Microbes Infect. 2022 Apr;24(6–7):104975. doi: 10.1016/j.micinf.2022.104975s
- Seldeslachts L, Vanderbeke L, Fremau A, et al. Early oseltamivir reduces risk for influenza-associated aspergillosis in a double-hit murine model. Virulence. 2021 Dec 31;12(1):2493–2508. doi: 10.1080/21505594.2021.1974327
- Reséndiz Sharpe A, da Silva RP, Geib E, et al. Longitudinal multimodal imaging-compatible mouse model of triazole sensitive and resistant invasive pulmonary aspergillosis. Dis Model Mech. 2022;15(3). doi: 10.1242/dmm.049165
- Binder U, Navarro-Mendoza MI, Naschberger V, et al. Generation of a Mucor circinelloides reporter strain-A promising new tool to study antifungal drug efficacy and Mucormycosis. Genes (Basel). 2018 Dec 7;9(12):613. doi: 10.3390/genes9120613
- Jacobsen ID, Lüttich A, Kurzai O, et al. In vivo imaging of disseminated murine Candida albicans infection reveals unexpected host sites of fungal persistence during antifungal therapy. J Antimicrob Chemother. 2014 Oct;69(10):2785–2796. doi: 10.1093/jac/dku198
- Delarze E, Ischer F, Sanglard D, et al. Adaptation of a gaussia princeps luciferase reporter system in candida albicans for in vivo detection in the galleria mellonella infection model. Virulence. 2015;6(7):684–693. doi: 10.1080/21505594.2015.1081330
- Troy T, Jekic-McMullen D, Sambucetti L, et al. Quantitative comparison of the sensitivity of detection of fluorescent and bioluminescent reporters in animal models. Mol Imaging. 2004 Jan;3(1):153535002004031. do i:
- Cohen-Wolkowiez M, Moran C, Benjamin DK, et al. Pediatric antifungal agents. Curr Opin Infect Dis. 2009 Dec;22(6):553–558. doi: 10.1097/QCO.0b013e3283321ccc