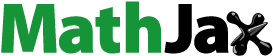
Abstract
It is known that all agents that cause cancer (carcinogens) also cause a change in the DNA sequence. In order to identify such often subtle changes, we attempt to integrate multiple molecular profile data sets released by the International Cancer Genome Consortium (ICGC). The list of data sets includes matched gene and microRNA expression profiles, somatic copy number variation, DNA methylation, and protein expression profiles for lung adenocarcinoma patients receiving treatments. We consider both unsupervised and supervised learning techniques (clustering and penalized regression) to identify interesting molecular markers corresponding to each type of –omics profiles that can differentiate patients. Associations between important markers of 2 types have been studied. An adaptive ensemble binary regression model has been presented that uses the entirety of available –omics profiles leading to a more accurate clinical prognosis for the patients in the given sample. This integrated study provides a more comprehensive picture of lung adenocarcinoma.
Introduction
According to Stratton et al,Citation1 identification of changes in the DNA sequence of a genome is central to the study of all cancers. There are many ways of observing biological data related to the DNA sequence, including measuring gene expressions, microRNA (abbreviated miRNA hereafter) expressions, protein expressions, somatic copy number variation, and DNA methylation profiles for individual subjects. Each type of data can give insights into related disease processes; finding ways to integrate statistical analyses of data sets of multiple types has the potential to identify connections between important genes, miRNAs, proteins, chromosomal segments, and methylation patterns associated with the disease process rather than merely identifying those which are associated with the disease process from each individual molecular profile.
In recent years, there have been some attempts to understand the disease process comprehensively by integrating various molecular profiles of patients (Kristensen et al.Citation2). In this paper, we consider several methods of analyzing matched data on genes, miRNAs, proteins, and copy number variation for the CAMDA 2014 lung adenocarcinoma challenge data provided by ICGC. We also explore the methylation patterns for this data.
We begin with separate analyses of data sets of different molecular types, where we cluster subjects with similar profiles (section- Exploratory Cluster Analysis). Subsequently, the clustering results are compared 2 at a time, along with clinical outcomes, using an overlapping proportion measure. In addition, penalized logistic regression models based on the elastic net regularization (Zou et al.Citation3) are used to detect statistically important variables (such as genes or miRNAs) for each molecular profile type (section- Prediction of Clinical Outcomes) for disease prognosis that is characterized by 2 clinical outcomes following treatment: disease progression and disease remission. An optimal model with integrated data is found in terms of accuracy rates (section- Integrated Penalized Regression) followed by ensembling. Finally, a correlation analysis is performed to identify associations between the significant variables detected by the regression analyses leading to a more complete picture of the disease process. Following the format of this journal, the results are presented in the next section. All the above mentioned underlying procedures are described in the Materials and Methods section of the manuscript. The paper ends with a Conclusion section.
Results
As part of an exploratory analysis, we first report the plots of normalized expression values for genes, miRNAs, and proteins ( respectively) for each data set (molecular profiles) to obtain overall summaries of the data sets and to identify features that stand out (perhaps indicating strong signals). The two genes with the highest mean expression values are SFTPC and SFTPA1 (); SFTPC regulation is known to be associated with lung diseases (see refs. Citation4-6) and SFTPA1 is associated with newborn respiratory distress syndrome and lung sarcoma (see refs. Citation7-13). The miRNA with the highest mean expression value is hsa-mir-21 (); this is well-known as an oncomir associated with a wide variety of cancers (see refs. Citation14-19). The protein with the highest mean expression value is FN1 (). Its expression is up-regulated in non-small cell lung carcinoma (NSCLC) and it may promote lung tumor growth/survival and resistance to therapy (Han et al.Citation20). A plot of the average segment mean by chromosome for the progression and complete remission groups is shown in . In both groups, chromosomes 1, 5, 14, 17, and 20 have higher average segment means while chromosomes 9, 13 and 18 have lower averages. Oxnard et al.Citation21 identified that chromosomes 1 and 17 are the locations of some potential oncogenes for non-small cell lung cancer. More importantly, the average segment means of chromosomes 9, 22 and X are noticeably different in the 2 groups of subjects with 2 different clinical outcomes or 2 different stages of the disease process (disease progression and the remission from the disease). According to Liu et al.,Citation22 chromosome 9 is the location of the LHX6 gene, which acts as a potential tumor-suppressor with epigenetic inactivation in lung cancer. This chromosome has significantly lower average segment mean in the disease progression group compared to the complete remission group. We paid attention to these results for more in depth analysis later. The segment means for Chromosome 22 appear to be positively (negatively) associated with expressions for genes (proteins) that are predictive of the clinical outcome (see, e.g., ).
Figure 1. Plots of various molecular profiles averaged over all available samples used in our analysis. Only some form of normalized molecular profiles were provided in the source data. (A) Plot of average expression values for different genes. (B) Plot of average expression values for different miRNAs. (C) Plot of average expression values for different proteins. (D) Plot of average segment means for various chromosomes.
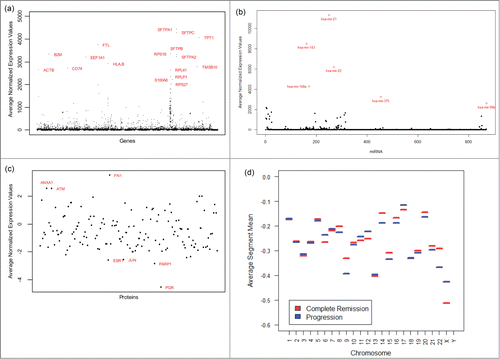
Figure 2. Diagram showing connections between some predictive genes, miRNAs, proteins, and chromosomes. All connected pairs have statistically significant correlations with P values< 0.05, except for one.
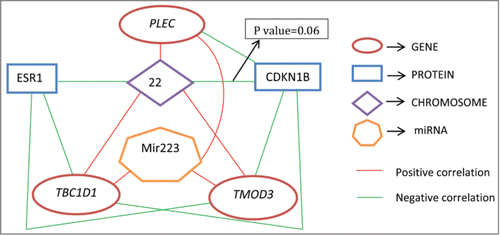
It is now well known that DNA methylation is essential for proper mammalian development and plays a role in many important activities such as X-chromosome inactivation. Hence we attempt to find the average methylation patterns in every chromosome, especially, for chromosomes 9, 22 and X as they seem to have different segment means in the 2 groups of patients. We plot the methylation patterns for several portions of the data (not shown here). Additionally, we find that on chromosomes 9, 22 and X, there is a statistically significant difference between the average methylation patterns in the subjects in complete remission and progression groups, based on a 2-sample t-test (with test statistic values 7.79, 3.80,−18.07 and p-values 6.7e-15, 0.0001 and <2.2e-16, respectively). Tsou et al.Citation23 suggested that chromosomal instability caused by hyper or hypo methylation could potentially become a causal epigenetic factor in cancer. Results of this analysis suggest that there may be a role of methylation in altering the copy number variation in chromosomes 9, 22 and X and may play an important role in NSCLC cancer.
Clustering and co-clustering results
For each data set containing a specific molecular profile, internal validation, described in Brock et al.Citation24 and Datta et al.,Citation25 is used to select the appropriate clustering algorithm and the optimal number of clusters. Plots are made of each internal validation measure (see Eqns. 1-3; ). Although one can visually inspect such plots to identify an optimal clustering algorithm and the number of clusters, such a task becomes increasingly difficult when the number of competing clustering algorithms is large and so is the number of validation measures. Thus we use a stochastic optimization and rank aggregation based method developed earlier by Pihur et al.Citation26 and Pihur et al.Citation27 to find the best clustering algorithm and the optimal size of clusters for this data.
Figure 3. Internal validation measures for 3 different clustering algorithms using proteins. The optimal cluster size is 2 and the k-means clustering optimizes each of the validation measures for the protein data.
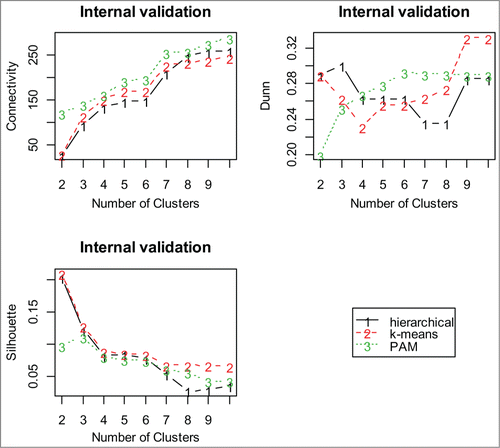
Complete linkage is used for gene and miRNA profiles, but for protein and copy number profiles, Ward's linkage is used for clustering the data. This is done to maintain relative uniformity between the sizes of obtained clusters. We find that hierarchical clustering with 2 clusters for each of the gene, miRNA and copy number profiles and k-means clustering with 2 clusters for the protein profile provide the best clustering of the subjects. A representative dendrogram based on hierarchical clustering is shown in . Interestingly, the optimal cluster number matches with the number of patient groups as characterized by the 2 possible clinical outcomes.
Figure 4. Dendrogram of hierarchical clustering using miRNA profiles. The divisions for the 2 main clusters are indicated by dashed lines; the two main clusters are indicated by 2 different colors.
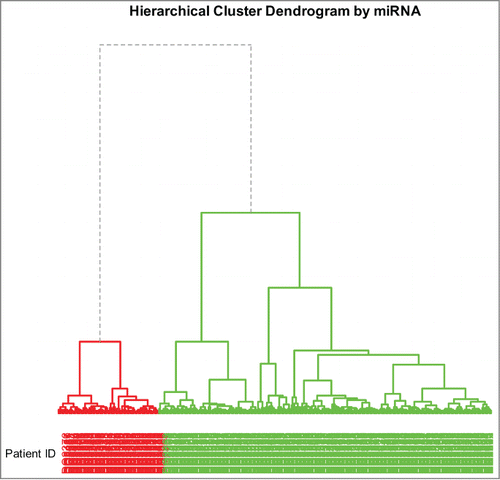
In order to examine the consistency of the subject clustering using pairs of molecular profiles, we calculate the overlap proportions of the cluster profiles. The overlap proportions of subjects between each pair of cluster profiles (EquationEqn. 4(4)
(4) ) are shown in . Empirically (as well as mathematically), it can be seen that the overlap proportion is expected to be roughly 0.33 if the group assignments are made randomly. As all the proportions in are larger than 0.33, there is some amount of consistency in the subject clustering results based on various –omics profiles data. Moreover, the overlap proportions between clustering based on gene and clinical data, as well as miRNA and clinical data, are quite substantial. These indicate that there are noticeable differences in the –omics profiles between the 2 groups of patients who would end up in remission versus those for whom the cancer would progress.
Table 1. Overlap proportions between the subject cluster profiles combining the results of clustering of 2 molcular profiles at a time
Penalized regression results
Next we investigate the predictive performances of the molecular profiles. The elastic net regularization for the penalized logistic regression model (see Eqn. 5) is used to predict the clinical outcomes/disease status (progression or remission) of the subjects. Each data set contains the molecular profiles of the subjects, along with their age and gender. Overall, 19 subjects have missing age and 104 subjects have missing disease status in the clinical data set; these subjects are excluded from regression analysis which adjusts for age and gender.
The optimal values of the elastic net tuning parameters (α, λ) (see equation EquationEqn. 6(6)
(6) ) are determined to be (1, .087), (.7, .102), (.5, .145), and (.3, .145) for individual regression models based on the gene expression, miRNA expression, protein expression, and copy number variation data sets, respectively. These models were used for variable selection for a correlation analysis (see Section 2.3). Next, we ran additional elastic net regressions where we integrate all possible combinations of the individual molecular profiles as covariates for predicting the clinical outcomes and then report the accuracy of prediction of clinical status of the subjects (). The accuracy rates for all models range from 64.3% to 74.3%. Finally, we use an adaptive ensemble binary regression model, described in Datta et al.Citation28 and Shah et al.,Citation29 where all individual penalized logistic regressions based on each molecular profile are considered as its components (see the methods section for details) leading to an overall accuracy rate of 75.7%.
Table 2. Accuracy rates for models with different combinations –omics profiles. Here G, M, P and C stand for gene, miRNA, protein and copy number variation, respectively
Correlation analysis results
The Spearman's rank correlation coefficients (see Eqn. 8) are computed between each pair among the genes, miRNAs, and proteins deemed most important by the penalized logistic regression analyses, and with the segment means for each chromosome. A diagram illustrating all interesting connections corresponding to strong (positive or negative) correlation is given in . Each pair of connected items is statistically significant (P-value <0.05) except for the CDKN1B –chromosome 22 pair, which is borderline significant (P-value = 0.06).
The miRNA hsa-mir-223 has significant positive correlations with 3 genes TMOD3, TBC1D1, and PLEC. Each of these genes has a significant negative correlation with the protein CDKN1B while the genes TMOD3 and TBC1D1 have significant negative correlations with the protein ESR1 (part of estrogen signaling pathway). p27 is an enzyme inhibitor which is encoded by the CDKN1B gene. Past research suggests that p27 is regulated by miRNAs and is associated with the cell cycle pathway. This is associated with multiple carcinomas. According to Blain et al.,Citation30 p27 is highly correlated with the prognosis of patients and hence has been explored as a potential target for cancer therapy. For example, low levels of p27 may indicate that a cancer is not that aggressive. This also has a significant negative association with chromosome 22. Baik et al.Citation31 identified that ESR1 is a part of estrogen signaling pathway which is being considered as a novel therapeutic target for NSCLC in women. The gene TMOD3 has been associated with estrogen excess and transitional cell carcinoma as noted by Pawlak et al.Citation32 It is connected with the protein ESR1 which has been linked to estrogen resistance syndrome and uterine disease (ref. Citation33) and NSCLC as stated before. TMOD3 is also connected with the protein CDKN1B which has been found to be connected with breast and prostate cancer as noted by Canbay et al.Citation34 and Chang et al.Citation35 From Wiesner et al.,Citation36 we know that loss of CDKN1B has also been frequently detected in tumors for blastic plasmacytoid dendritic cell neoplasms. The PLEC gene is also highly correlated with the protein CDKN1B. According to Charlesworth et al.,Citation37 it can have mutations which cause autosomal recessive forms of epidermolysis bullosa simplex with muscular dystrophy or pyloric atresia. Additionally, PLEC is known to be positively correlated with the Epidermal growth factor receptor (EGFR) signaling pathway. Rosell et al.Citation38 found that EGFR mutation related lung cancers are associated with lung cancers in women and never-smokers. Genetic variation in the TBC1D1 gene causes obesity in women. Obesity is typically associated with decreased insulin sensitivity and elevated circulating concentrations of glucose and insulin. Estrogen signaling plays a role in this process.
Materials and Methods
We consider pre-processed and normalized data from the International Cancer Genomic Consortium (ICGC) on gene expression, miRNA expression, protein expression, somatic copy number variation, and DNA methylation profiles for subjects with lung adenocarcinoma. As the number of genes is too large, a variance based filtration of the gene profile is used. Through this technique, we retain the top 75% of the genes based on the variances of their expression values. Two subjects (DO23996 and DO25020) with different sets of genes are dropped leading to a data set with 15916 genes on 131 subjects. Also, there are expression values for 709 miRNAs on 379 subjects (after removing the miRNAs which had all zero values for normalized expression levels) and expression values for 139 proteins on 237 subjects. In addition, there are a few subjects with missing chromosomal segment means in the copy number variation data, and after removing these subjects, segment means for each of the 24 chromosomes (taking X and Y as different chromosomes) are available for 383 subjects. Finally, methylation data is available on all chromosomes, and clinical data for disease status (progression or complete remission), age, and gender are also available. In summary, there are 2 groups of patients, namely, progression and complete remission, with multiple molecular profiles.
Exploratory cluster analysis
In order to find natural groupings in the data we use cluster analysis. Cluster analysis groups subjects in terms of the similarity of their molecular profiles. As different clustering algorithms group the data differently, we use 3 different clustering algorithms, hierarchical (with Ward and/or complete linkage), k-means, and PAM, and obtained the best clustering algorithm for each of these molecular profiles in the following manner. We evaluate the performances of these clustering algorithms using 3 different measures for internal validation, namely, connectivity (see refs. Citation24,39), silhouette width (see refs. Citation24,40), and the Dunn index (see refs. Citation24,41). Then a stochastic optimization technique named rank aggregationCitation26,27 is used to combine the results of above internal validation measures to determine the optimal clustering algorithm as well as an appropriate cluster size. The definitions of the internal validation measures considered by us are given below.
Connectivity: For the ith observation in the data set, let its nearest neighbor be denoted by
and let
be defined as 0 if
and
are in the same cluster and
otherwise. Here, the nearest neighbor to the
observation is that observation (other than the
observation itself) which has the smallest distance from the
observation. This is the interpretation for
. Similarly, the
nearest neighbor to an observation, for
, can also be defined. For a particular clustering partition of N observations into K disjoint clusters, say,
, the connectivity is defined as
(1)
(1) Here, L determines the number of neighbors that contribute to the connectivity measure. Note that, a neighbor to an observation can belong to a cluster different from that of the observation. Connectivity ranges from 0 to
; it should be minimized for obtaining the ‘optimal’ clustering partition.
Silhouette width: For any observation , the silhouette value is defined as
(2)
(2) Here,
is the average distance between
and all other observations in the same cluster,
is the average distance between
and the observations in the “nearest neighboring cluster,” where
is the cluster which contains the
observation,
is a distance (e.g., Euclidean, Manhattan) between the
and the
observations and n(C) is the number of observations in cluster C. The silhouette width is the average of the silhouette values for all observations and ranges from −1 to 1. The silhouette width should be maximized for obtaining the best clustering partition.
Dunn index: The Dunn index is computed as the ratio of the smallest distance between observations not in the same cluster to the largest intra-cluster distance which is given by(3)
(3) Here,
is the maximum distance between observations in cluster
. The Dunn index ranges from 0 to
and needs be maximized for obtaining the ‘best’ clustering partition.
In order to maintain brevity of this paper, we do not include the details of the rank aggregation technique which are available in Pihur et al.Citation26 and Pihur et al.Citation27 For each molecular profile (e.g., gene, miRNA, protein, copy number variation), the rank aggregation technique is used to determine the optimal clustering algorithm among hierarchical, k-means and PAM based on the 3 internal validation measures described above. This technique also determines the optimal cluster size for each profile.
To measure the similarity between the optimal clustering partitions of data sets for different molecular profiles, the overlapping proportion(4)
(4) is computed for each pair of profiles. Here,
is the set of subjects in the cluster containing the ith subject based on the jth profile,
is the number of subjects common to the jth and kth data sets, and
is the set of common subjects.
Prediction of clinical outcomes
For each data set, a penalized logistic regression model for predicting clinical outcomes for the disease status (progression or disease remission) is considered based on each molecular profile data such as expression values or chromosomal segment means of the subjects. Additionally, the ages and gender of the subjects are also used in the model. The model is given by(5)
(5) for
where, for the
th subject based on the
th profile data set,
is the probability for progression of the disease,
is the
th expression value or chromosomal segment mean, and the β's are the corresponding regression coefficients in the model. We fit the elastic net regression model, which estimates the parameter vector by minimizing
(6)
(6) .
The R package glmnet, developed by Friedman et al.,Citation42 is used to select the tuning parameters and
by minimizing the cross validation error. In order to reduce the sensitivity of the model fitting and variable selection procedure, a bootstrap step is added (Efron et al.Citation43). Covariates with non-zero coefficients for each bootstrap sample are recorded. The bootstrap sampling process is repeated 1000 times, and the covariates are ranked based on how often they were selected by the elastic net model for the bootstrap samples. This provides us with a ranked list of variables corresponding to each molecular data type. The variables selected by this algorithm may represent the genes, miRNAs, and proteins that are most useful for prediction of the clinical outcome. We use the top 20 genes, miRNAs, and proteins for a correlation analysis to derive a more complete picture.
Integrated penalized regression
Next, the 4 data sets (gene expression, miRNA expression, protein expression and somatic copy number variation) are merged together and a penalized logistic regression model is fitted for predicting the clinical outcome of disease status based on age, gender, expression values and chromosomal segment means. The accuracy rate (see EquationEqn. 7(7)
(7) ) for this model is computed and compared to that of the models with individual molecular profiles as covariates as described in the Penalized Regression Results section based on each of the 4 individual data sets.
Next, to understand which combinations of integrated molecular profiles provide more accurate prediction of the clinical outcomes of the subjects, we compute the accuracy rates for all the models involving all possible combinations of the individual data sets.(7)
(7) where ‘observed’ and ‘predicted’ in the above display denotes the observed and predicted clinical outcomes, respectively, for every subject, and ‘i’ denotes a typical subject.
Finally, we build a super binary regression model to predict the clinical outcome where we integrate all data types in the following way. Bootstrap resamples of the original data are used as training data to fit penalized regression models based on individual –omics profiles. These models are then evaluated on the “out of bag” sample (this is a term used in the bagging literature to indicate part of the original sample that was not selected in a bootstrap sample) using multiple performance measures. An overall best predictive model is identified for each bootstrap replicate via rank aggregation of the performances of the models on various measures and stored for future prediction. Finally, the clinical outcome for a patient is predicted by running these individual best models and combining the predictions by majority voting.
In our case, 4 penalized logistic regression models with a common set of observations (70 subjects) based on each of the 4 data sets (gene expression, miRNA expression, protein expression and somatic copy number variation) are considered. Also, we consider 4 performance measures which are to be used (sensitivity, specificity, false discovery rate and false non-discovery rate). Data of one subject is removed from each of the 4 data sets and is kept aside for outcome prediction by the ensemble model. A bootstrap sample of size 69 is drawn (from the remaining observations) using simple random sampling with replacement. For each of the 4 data sets, a penalized logistic regression model is fitted using the bootstrap sample which is used to predict the binary outcomes of the out of bag (OOB) samples. Since the true responses (clinical outcome) for the OOB samples are known to us, we can compute the 4 performance measures (sensitivity, specificity, false discovery rate and false non-discovery rate) from the predicted outcomes. The four models are ranked according to their performances under each of the 4 performance measures and 4 ordered lists of models , each of size 4, are obtained. These four ordered lists are aggregated using the weighted rank aggregation procedureCitation26,27 which determines the best performing model
. We repeat the procedure 100 times with new bootstrap samples and to obtain 100 best models. Using these 100 best models, we predict the clinical outcome of the left out observation. Each model cast a vote (either 0 or 1) regarding the clinical outcome and the final prediction is done on the basis of majority voting. The whole algorithm is repeated for each of the 70 observations. A schematic diagram describing the steps of computing the ensemble binary regression model is shown in . The accuracy rate is calculated for the ensemble binary regression using the formula given in Equation(7)
(7)
(7) .
Correlation analysis
Spearman's rank correlation coefficients are computed for each pair of profile data sets using the formula(8)
(8) where
is the difference between the ranks of the ith subject that is common to the jth and kth profile data sets and
denotes the number of such subjects (patients). Using the asymptotic t-distribution, approximate marginal p-values are computed for these correlation coefficients to identify statistically significant associations between important genes, miRNAs, proteins, and chromosomes that are predictive of the clinical outcomes.
Conclusion
Although there has been a vast number of research articles which examine how to connect individual molecular profiles with clinical outcomes, there are relatively few results on the integration of molecular profiles. However, in recent years there have been some attempts to integrate different molecular profiles to get a comprehensive knowledge of the disease process for a complex disease like cancer. In this paper, we use multiple methods of data integration to provide an integrated analysis of matched gene expression, miRNA expression, protein expression, and somatic copy number variation profiles and their effects on the clinical outcomes using NSCLC lung adenocarcinoma, CAMDA 2014 challenge data. It is to be noted that the data available were already normalized and we did not have access to the raw data. In general, due to ICGC policies regarding public disclosure of clinical information, very limited information was provided about the data. Additionally, the data lacks a control group and thus all these subjects have had cancer. So, we expect a lot of similarity in their molecular profiles. In spite of all these limitations, we are able to show that there are subtle but important and biologically meaningful differences between the –omics profiles of patients who would enter remission vs. those for whom the cancer would progress even after the treatment. The results are quite illuminating; however, further investigation is needed to claim the causation of the clinical outcome from such retrospective investigations. We are nevertheless optimistic that this data analysis pipeline can be used for integrating multiple molecular profiles data toward disease status prediction.
We find that the cluster analysis along with the rank aggregation technique is able to identify the number of groups in the data consistently across the molecular profiles which also matched with the number of clinical groups in the sample. Also, the co-clustering results show that not only individually but also collectively, the molecular profiles are somewhat consistent in terms of their ability to group the subjects into their respective clinical outcome groups. Secondly, the comparative results of penalized regression models using different individual molecular profiles, the combinations of multiple molecular profiles, and the ensemble binary regression () show that optimally ensembling different individual regression models may provide the best predictive model for the clinical outcome; in our case it led to an observed accuracy rate of 75.7%. Thirdly, from our correlation analysis of top molecular features that are predictive of the clinical separation, we note that the estrogen signaling pathway and the epidermal growth factor receptor (EGFR) signaling pathways are 2 of the most differentiating pathways between the 2 groups of patients. Estrogen signaling is known to play a significant role in lung cancer, especially for women with estrogen therapy. EGFR pathway has long been established as therapeutic targets of lung cancer. It is also known that estrogen signaling interacts with EGFR in lung cancer. The existing results and the present analysis may mean that both of these pathways may play a role not only in terms of disease causation but also in the treatment outcome for NSCLC.
Overall, it is our hope that this paper makes a convincing case in favor of more integrated studies of –omics molecular profiles in the future since such studies yield a bigger biological picture than a single assay type which may in turn help us make better prognoses and provide better treatment regimes.
Disclosure of Potential Conflicts of Interest
No potential conflicts of interest were disclosed.
Acknowledgments
We thank an anonymous reviewer for many useful comments.
Funding
This research work is partially supported by NIH grant CA 170091−01A1 (Su. Datta).
References
- Stratton MR, Campbell PJ, Futreal PA. The cancer genome. Nature 2009; 458:719-24; PMID:19360079; http://dx.doi.org/10.1038/nature07943.
- Kristensen VN, Vaske CJ, Ursini-Siegel J, Van Loo P, Nordgard SH, Sachidanandam R, Sørlie T, Wärnberg F, Haakensen VD, Helland A, et al. Integrated molecular profiles of invasive breast tumors and ductal carcinoma in situ (DCIS) reveal differential vascular and interleukin signaling. Proc Natl Acad Sci U S A 2012; 109:2802-7; PMID:21908711; http://dx.doi.org/10.1073/pnas.1108781108.
- Zou H, Hastie T. Regularization and variable selection via the elastic net. J Royal Statistical Society B 2005; 67:301-20; http://dx.doi.org/10.1111/j.1467-9868.2005.00503.x.
- Guillot L, Epaud R, Thouvenin G, Jonard L, Mohsni A, Couderc R, Counil F, de Blic J, Taam RA, Le Bourgeois M, et al. New surfactant protein C gene mutations associated with diffuse lung disease. J Med Genet 2009; 46:490-4; http://dx.doi.org/10.1136/jmg.2009.066829.
- Nogee LM, Dunbar AE, Wert SE, Askin F, Hamvas A, Whitsett JA. A mutation in the surfactant protein C gene associated with familial interstitial lung disease. N Engl J Med 2001; 344:573-9; http://dx.doi.org/10.1056/NEJM200102223440805.
- Thomas AQ, Lane K, Phillips III J, Prince M, Markin C, Speer M, Schwartz DA, Gaddipati R, Marney A, Johnson J, et al. Heterozygosity for a surfactant protein C gene mutation associated with usual interstitial pneumonitis and cellular nonspecific interstitial pneumonitis in one kindred. Am J Respir Crit Care Med 2002; 165:1322-8; PMID:11991887; http://dx.doi.org/10.1164/rccm.200112-123OC.
- Jiang F, Caraway NP, Bekele BN, Zhang HZ, Khanna A, Wang H, Li R, Fernandez RL, Zaidi TM, Johnston DA, et al. Surfactant protein A gene deletion and prognostics for patients with stage I non-small cell lung cancer. Clin Cancer Res 2005; 11:5417-24; PMID:16061856; http://dx.doi.org/10.1158/1078-0432.CCR-04-2087.
- Lüfgren J, Rämet M, Renko M, Marttila R, Hallman M. Association between surfactant protein A gene locus and severe respiratory syncytial virus infection in infants. J Infect Dis 2002; 185:283-9.
- Pettigrew MM, Gent JF, Zhu Y, Triche EW, Belanger KD, Holford TR, Bracken MB, Leaderer BP. Association of surfactant protein A polymorphisms with otitis media in infants at risk for asthma. BMC Med Genet 2006; 7:68; http://dx.doi.org/10.1186/1471-2350-7-68.
- Pettigrew MM, Gent JF, Zhu Y, Triche EW, Belanger KD, Holford TR, Bracken MB, Leaderer BP. Respiratory symptoms among infants at risk for asthma: association with surfactant protein A haplotypes. BMC Med Genet 2007; 8:15; PMID:17407567; http://dx.doi.org/10.1186/1471-2350-8-15.
- Seifart C, Lin HM, Seifart U, Plagens A, DiAngelo S, von Wichert P, Floros J. Rare SP-A alleles and the SP-A1-6A(4) allele associate with risk for lung carcinoma. Clin Genet 2005; 68:128-36; PMID:15996209; http://dx.doi.org/10.1111/j.1399-0004.2005.00470.x.
- Stoffers M, Goldmann T, Branscheid D, Galle J, Vollmer E. Transcriptional activity of surfactant-apoproteins A1 and A2 in non-small cell lung carcinomas and tumor-free lung tissues. Pneumologie 2004; 58:395-9; PMID:15216431; http://dx.doi.org/10.1055/s-2004-818506.
- Stray-Pedersen A, Vege A, Opdal SH, Moberg S, Rognum TO. Surfactant protein A and D gene polymorphisms and protein expression in victims of sudden infant death. Acta Paediatr 2009; 98:62-8; PMID:18983439; http://dx.doi.org/10.1111/j.1651-2227.2008.01090.x.
- Asangani IA, Rasheed SA, Nikolova DA, Leupold JH, Colburn NH, Post S, Allgayer H. MiRNA-21 (miR-21) post-transcriptionally downregulates tumor suppressor Pdcd4 and stimulates invasion, intravasation and metastasis in colorectal cancer. Oncogene 2008; 27:2128-36; PMID:17968323; http://dx.doi.org/10.1038/sj.onc.1210856.
- Liu M, Wu H, Liu T, Li Y, Wang F, Wan H, Li X, Tang H. Regulation of the cell cycle gene, BTG2, by miR-21 in human laryngeal carcinoma. Cell Res 2009; 19:828-37; PMID:19546886; http://dx.doi.org/10.1038/cr.2009.72.
- Meng F, Henson R, Wehbe-Janek H, Ghoshal K, Jacob ST, Patel T. MicroRNA-21 regulates expression of the PTEN tumor suppressor gene in human hepatocellular cancer. Gastroenterology 2007; 133:647-58; PMID:17681183; http://dx.doi.org/10.1053/j.gastro.2007.05.022.
- Papagiannakopoulos T, Shapiro A, Kosik KS. MicroRNA-21 targets a network of key tumor-suppressive pathways in glioblastoma cells. Cancer Res 2008; 68:8164-72; PMID:18829576; http://dx.doi.org/10.1158/0008-5472.CAN-08-1305.
- Wickramasinghe NS, Manavalan TT, Dougherty SM, Riggs KA, Li Y, Klinge CM. Estradiol down regulates miR-21 expression and increases miR-21 target gene expression in MCF-7 breast cancer cells. Nucleic Acids Res 2009; 37:2584-95; PMID:19264808; http://dx.doi.org/10.1093/nar/gkp117.
- Zheng J, Xue H, Wang T, Jiang Y, Liu B, Li J, Liu Y, Wang W, Zhang B, Sun M. miR-21 downregulates the tumor suppressor P12 CDK2AP1 and Stimulates Cell Proliferation and Invasion. J Cell Biochem 2011; 112:872-80; PMID:21328460; http://dx.doi.org/10.1002/jcb.22995.
- Han S, Khuri FR, Roman J. Fibronectin stimulates non-small cell lung carcinoma cell growth through activation of Akt/mammalian target of rapamycin/S6 kinase and inactivation of LKB1/AMP-activated protein kinase signal pathways. Cancer Res 2006; 66:315-23; PMID:16397245; http://dx.doi.org/10.1158/0008-5472.CAN-05-2367.
- Oxnard GR, Binder A, Jänne PA. New targetable oncogenes in non-small-cell lung cancer. J Clin Oncol 2013; 31:1097-104; PMID:23401445; http://dx.doi.org/10.1200/JCO.2012.42.9829.
- Liu W-b, Jiang X, Han F, Li Y-h, Chen H-q, Liu Y, Cao J, Liu J-y. LHX6 acts as a novel potential tumor suppressor with epigenetic inactivation in lung cancer. Cell Death Dis 2013; 4:e882; http://dx.doi.org/10.1038/cddis.2013.366.
- Tsou JA, Hagen JA, Carpenter CL, Laird-Offringa IA. DNA methylation analysis: a powerful new tool for lung cancer diagnosis. Oncogene 2002; 21:5450-61; PMID:12154407; http://dx.doi.org/10.1038/sj.onc.1205605.
- Brock G, Pihur V, Datta S, Datta S. clValid: An R package for cluster validation. J Statistical Software 2008; 25:1-22.
- Datta S, Datta S. Comparisons and validation of statistical clustering techniques for microarray gene expression data. Bioinformatics 2003; 19:459-66; PMID:12611800; http://dx.doi.org/10.1093/bioinformatics/btg025.
- Pihur V, Datta S, Datta S. Weighted rank aggregation of cluster validation measures: a Monte Carlo cross-entropy approach. Bioinformatics 2007; 23:1607-15; http://dx.doi.org/10.1093/bioinformatics/btm158.
- Pihur V, Datta S, Datta S. RankAggreg, an R package for weighted rank aggregation. BMC Bioinformatics 2009; 10:62; PMID:19228411; http://dx.doi.org/10.1186/1471-2105-10-62.
- Datta S, Pihur V, Datta S. An adaptive optimal ensemble classifier via bagging and rank aggregation with applications to high dimensional data. BMC Bioinformatics 2010; 11:427; http://dx.doi.org/10.1186/1471-2105-11-427.
- Shah JS, Datta S, Datta S. A multi-loss super regression learner via bagging and rank aggregation with application to survival prediction using proteomics. Computational Statistics 2014; 29:1749-67; PMID:20716381; http://dx.doi.org/10.1007/s00180-014-0516-z.
- Blain SW, Scher HI, Cordon-Cardo C, Koff A. p27 as a target for cancer therapeutics. Cancer Cell 2003; 3:111-5; PMID:12620406; http://dx.doi.org/10.1016/S1535-6108(03)00026-6.
- Baik CS, Eaton KD. Estrogen signaling in lung cancer: an opportunity for novel therapy. Cancers 2012; 4:969-88; PMID:24213497; http://dx.doi.org/10.3390/cancers4040969.
- Pawlak G, McGarvey TW, Nguyen TB, Tomaszewski JE, Puthiyaveettil R, Malkowicz SB, Helfman DM. Alterations in tropomyosin isoform expression in human transitional cell carcinoma of the urinary bladder. Int J Cancer 2004; 110:368-73; http://dx.doi.org/10.1002/ijc.20151.
- www.genecards.org/cgi-bin/carddisp.pl?gene=ESR1.
- Canbay E, Eraltan IY, Cercel A, Isbir T, Gazioglu E, Aydogan F, Cacina C, Cengiz A, Ferahman M, Zengin E, et al. CCND1 and CDKN1B polymorphisms and risk of breast cancer. Anticancer Res 2010; 30:3093-8.
- Chang BL, Zheng SL, Isaacs SD, Wiley KE, Turner A, Li G, Walsh PC, Meyers DA, Isaacs WB, Xu J. A polymorphism in the CDKN1B gene is associated with increased risk of hereditary prostate cancer. Cancer Res 2004; 64:1997-9; http://dx.doi.org/10.1158/0008-5472.CAN-03-2340.
- Wiesner T, Obenauf AC, Cota C, Fried I, Speicher MR, Cerroni L. Alterations of the cell-cycle inhibitors p27(KIP1) and p16(INK4a) are frequent in blastic plasmacytoid dendritic cell neoplasms. J Invest Dermatol 2010; 130:1152-7; http://dx.doi.org/10.1038/jid.2009.369.
- Charlesworth A, Chiaverini C, Chevrant-Breton J, DelRio M, Diociaiuti A, Dupuis RP, El Hachem M, Le Fiblec B, Sankari-Ho AM, Valhquist A, et al. Epidermolysis bullosa simplex with PLEC mutations: new phenotypes and new mutations. Br J Dermatol 2013; 168:808-14; PMID:23289980; http://dx.doi.org/10.1111/bjd.12202.
- Rosell R, Moran T, Queralt C, Porta R, Cardenal F, Camps C, Majen M, Lopez-Vivanco G, Isla D, Provencio M, et al. Screening for epidermal growth factor receptor mutations in lung cancer. N Engl J Med 2009; 361:958-67; PMID:19692684; http://dx.doi.org/10.1056/NEJMoa0904554.
- Handl J, Knowles J, Kell DB. Computational cluster validation in post-genomic data analysis. Bioinformatics 2005; 21:3201-12; PMID:15914541; http://dx.doi.org/10.1093/bioinformatics/bti517.
- Rousseeuw PJ. Silhouettes: a graphical aid to the interpretation and validation of cluster analysis. J Computat Appl Math 1987; 20:53-65; http://dx.doi.org/10.1016/0377-0427(87)90125-7.
- Dunn JC. Well-separated clusters and optimal fuzzy partitions. J Cybernet 1974; 4:95-104; http://dx.doi.org/10.1080/01969727408546059.
- Friedman J, Hastie T, Tibshirani R. Regularization paths for generalized linear models via coordinate descent. J Statist Software 2010; 33:1-22; PMID:20808728.
- Efron B, Tibshirani R. Improvements on cross-validation: the 632+ bootstrap method. J Am Statist Associat 1997; 92:548-60.