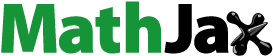
ABSTRACT
Older adults are at disproportionately high risk of severe influenza-related outcomes and represent the main target of the annual influenza vaccination. The protective effect of seasonal influenza vaccination on the observed mortality indicators is controversial. In this ecological study, spatiotemporal patterns of pneumonia- and influenza-related mortality registered in the Italian elderly over seven (2011–2017) consecutive seasons were explored and the epidemiological association between the observed local pneumonia- and influenza-related mortality and influenza vaccination campaign features were modeled by using both fixed- and random-effects panel regression models. The descriptive spatiotemporal analysis showed a clear North–South gradient, where northern regions tended to report more pneumonia- and influenza-related deaths. After adjustment for potential confounders, it was found that each 1% increase in influenza vaccination coverage rate would be associated (P < .001) with a 1.6–1.9% decrease in pneumonia- and influenza-related mortality. Moreover, each 1% increase in the use of MF59®-adjuvanted trivalent influenza vaccine would be associated (P < .05) with a further 0.4% decrease in pneumonia- and influenza-related mortality. This study supports the increase in annual influenza vaccination in Italy and suggests that a higher level of use of the adjuvanted influenza vaccine in the elderly may be beneficial.
Introduction
Worldwide, influenza is one of the leading infectious disease in terms of both incidence and mortality rates.Citation1,Citation2 Seasonal influenza vaccination represents the most effective public health intervention able to reduce the burden of disease.Citation2,Citation3 Indeed, the World Health Organization’s (WHO) most recent position paperCitation2 has listed several priority targets for annual influenza vaccination: pregnant women, children aged 6 months to 5 years, the elderly, subjects with specific chronic conditions, healthcare workers, and international travelers. Among these, the elderly is probably the most recognized target group; indeed, as per the European Center for Disease Prevention and Control (ECDC),Citation4 all European Union (EU) Member States recommend seasonal influenza vaccination for older adults.
Influenza vaccine (IV)-induced immunogenicity and/or protection is often poor in the elderly as a result of immunosenescence.Citation5 In order to circumvent this unmet need, alternative IV formulations have been developed. The first worldwide available IV specifically developed for the elderly was that formulated as a standard-dose egg-based subunit trivalent IV including MF59® (Seqirus UK Ltd.) adjuvant (adjuvanted trivalent influenza vaccine; aTIV).Citation6 Italy was the first country to adopt aTIV in 1997,Citation6 where it was still available during 2020/21 influenza season. Other historically or currently commercialized IVs may also address immunosenescence, including (i) virosomal;Citation7 (ii) intradermalCitation8 and (iii) high-dose IVs.Citation9
The rationale for this study was primarily driven by a gap in the understanding of association between influenza vaccination coverage (IVC) rates and influenza-associated mortality. For instance, a previous Italian ecological studyCitation10 did not find any meaningful association between the IVC rate and influenza excess mortality over time. However, the study by Rizzo et al.Citation10 did not distinguish between different types of available IVs at that time. Indeed, in the paper by Rizzo et al.,Citation10 dating back to 2006, it has been stated that “In Italy, more immunogenic vaccine with novel adjuvants has been introduced since 1997 … but it is too early to evaluate their population impact.” On the other hand, a more recent studyCitation11 conducted in the Province of Treviso (northeastern Italy) found that the risk of all-cause death was significantly lower (by 33–39%) in the vaccinated elderly (as compared with unvaccinated subjects) in three consecutive seasons (2014/15–2016/17). Of note, aTIV was the most frequently administered IV in Treviso.Citation11
In the context of Italian fiscal federalism single regions, the autonomous provinces of South Tyrol and Trento (henceforth referred to as “regions”) are granted a certain level of freedom to achieve their own public health goals.Citation12 Regarding influenza immunization, each year the Italian Ministry of Health issues a circular on the prevention and control of influenza;Citation13 each region may then fully adopt the national recommendations or provide its own circular/recommendations.Citation12,Citation14
Diversity in the adopted policies may result in both (i) the so-called “jeopardization”Citation12,Citation14 of IVC rates [up to double difference reported in the observed 2019/20 season IVC rates among older adults aged ≥65 yearsCitation15 and (ii) different patterns of use for the available types of IV.Citation12,Citation14
The primary aim of this study was to explore spatiotemporal patterns of pneumonia- and influenza (P&I)-related mortality observed in Italian older adults aged 65 years or above. The second goal was to investigate the epidemiological association between the observed local P&I mortality among subjects aged ≥65 years and IVC rates and IV policy patterns.
Materials and methods
Overall study design
This is a typical ecological study: we investigated officially registered P&I-related mortality in the elderly (defined here as subjects aged ≥65 years) at the level (i.e., unit) of Italian provinces (N = 110) and/or regions (N = 21) over seven consecutive post-pandemic seasons (2010/11–2016/17). In other words, we analyzed population groups and not single individuals. Both exploratory and analytical approaches were considered.
Readers interested in both strengths and limitations of the ecological study design are invited to read the paper by Morgenstern;Citation16 moreover, the limitations specific to this exploratory study will be discussed later in the manuscript.
In this paper, we considered only the post-pandemic period (i.e., starting from season 2010/11). This choice was based on the fact that the pandemic 2009 A/H1N1 (A/H1N1pdm09) virus completely replaced the so-called seasonal A/H1N1 (A/H1N1s) that circulated before 2009.Citation17,Citation18 Data for 2018 onwards were not considered since no officially reported P&I-related mortality estimates were available at the time of data extraction (as of December 2020).Citation19,Citation20
Data sources
Most data came from the official Italian data flows publicly available from the Italian Ministry of Health 15, National Institute of Health,Citation18 National Institute of Statistics,Citation19,Citation20 and National Institute for Environmental Protection and Research.Citation21 Data regarding quotas for different types of IV were provided by Seqirus S.r.l., Italy (company database of regional demands for individual types of IV, i.e., data on tender allotments). The variables considered and corresponding data sources are reported in Supplementary Material, Table S1.
Study outcome
The study outcome was the country-/province-/region- and year-specific estimate of P&I-related mortality in older adults aged ≥65 years as per the European Shortlist for Causes of Death (N = 65 causes) compatible with the three most recent International Classification of Diseases (ICD) versions for influenza (ICD-8: 470–474; ICD-9: 487; ICD-10: J10–J11) and pneumonia (ICD-8: 480–486; ICD-9: 480–486; ICD-10: J12–J18) codes.Citation20,Citation22 For this reason, we extracted the readily available datasetCitation20 on the P&I mortality rate (per 10,000 inhabitants) until the last available year of 2017 for the whole country, regions, and provinces.
Spatiotemporal analysis of pneumonia- and influenza-related mortality
Depending on data availability,Citation20 the spatiotemporal analysis could be conducted at the level of single provinces (N = 110). For this reason, first we visually explored the observed province-specific P&I mortality rates separately by year. This was done by plotting choropleth maps.
Moran’s I global spatial autocorrelation coefficientsCitation23 were then computed in order to measure the overall clustering pattern of the observed mortality rates. The interpretation of Moran’s I is similar to that of Pearson’s r correlation coefficient: positive statistically significant I values indicate geographic patterns of spatial clustering, negative significant I estimates show clustering of dissimilar values, while non-significant values at α < 0.05 indicate complete spatial randomness. Considering that Italy has the two Islands of Sicily and Sardinia, for Moran’s I statistics the k nearest neighbor spatial weights matrix was used.Citation24 As a “rule-of-thumb”Citation25 we set the value of k as the square root of the total number of observations (N = 110); this means the k-value used was 10.
Providing that all and year-specific global I coefficients were statistically significant, we then further investigated the local indicators of spatial association (LISA).Citation26 Choropleth maps were created to visualize the four types of clusters/outliers, namely hot-hot (hotspots), i.e., observations that signified provinces with a higher than average mortality rate surrounded by provinces with a higher than average mortality rate, while cold–cold (coldspots) signified provinces with a lower than average mortality rate surrounded by provinces with a lower than average mortality rate. Low–high and high–low outcomes represented outliers: these were provinces with low/high average mortality rates surrounded by provinces with high/low average mortality rates, respectively.
The spatiotemporal analysis was performed in R stats packages, version 2.15.2.Citation27
Spatiotemporal analysis of pneumonia- and influenza-related mortality
The independent variables of interest were regional IVC rates and the proportion of aTIV doses to the total number of IV doses put into tender allotments. During the study period, IVC was recommended and fully reimbursed for all subjects aged ≥65 years, people ≥6 months affected by certain health conditions and some other categories. The Italian Ministry of Health routinely report region- and season-specific IVC rates for both the general population and older adults aged ≥65 years.Citation15 Data on province-specific IVC rates are not publicly available. Therefore, the unit of this analytical part of the analysis was a region (N = 21). The primary predictor of interest was IVC in older adults aged ≥65 years. However, a higher IVC rate in younger age groups may exercise some protective effect on the elderly owing to the phenomenon of herd protection. Indeed, some studies underlined the important role of children and adolescents in spreading influenza virus in their householdsCitation28–30 and therefore to their grandparents. A model by Fumanelli et al.Citation31 has suggested a significant social interaction between Italian elderly and younger individuals. For this reason and in order to account for the possible effects of herd protection, we also included a variable of IVC in subjects aged <65 years.
Another independent variable of interest was the proportion of potential aTIV users to the total number of IV doses put into tender allotments, and the data from the Seqirus Italy tender department. According to the latest Italian official recommendations,Citation13 aTIV may be used only for people aged ≥65 years. Therefore, we hypothesized that the higher local use of aTIV may be associated with better health-related outcomes among the Italian elderly.
To establish an association (or lack of association) between the region- and year-specific P&I mortality rates in the elderly and predictors of interest (i.e., IVC rates and share of aTIV doses) panel regression analysis was undertaken. Briefly, the panel considered 21 spatial units (i.e., regions) followed over seven consecutive post-pandemic years and therefore consisted of 21 × 7 = 147 observations. However, an important assumption has to be highlighted here. P&I mortality data are routinely reported for the whole calendar year,Citation19,Citation20 while the IV campaign usually starts in mid-October/November and almost all IV doses are administered by the end of December32. In Italy, according to the Italian National Institute of Health, most laboratory-confirmed influenza deaths occur between January and March,Citation32 and influenza-like illness (ILI) peaks were usually reached in late January or February (Supplementary Material, Table S2).Citation18 Moreover, considering the time lag of 2–6 weeks between IV administration and the peak of the vaccine-induced immune response,Citation33 it is more likely that IV administered in autumn/winter of a year t-1 will mainly exercise its effect (if any) on bacterial influenza-related complications (that are the most frequentCitation35,Citation36 and require some time to be developed) leading to death in the first months of the following year t.
Both the fixed-effects (FE) and random-effects (RE) methods were applied. The FE approach may be useful in the context of causal inference: while standard regression techniques provide biased estimates of causal effects in case there are unobserved confounders, FE regression may provide unbiased estimates in this situation.Citation32,Citation34 In other words, in our models, region-level FEs were included to absorb unobserved region-level heterogeneity in the observed P&I mortality rates not explained by other covariates in the model.Citation37 Indeed, unobserved effects are typical in ecological and social research.Citation38 By contrast, the RE approach assumes that region-specific effects are not correlated with independent variables.Citation39 In any case, the Hausman’s specification test was appliedCitation40 to formally differentiate between FE and RE models; the null hypothesis of this test is that the RE model estimates are consistent and efficient.
The following socioeconomic, environmental, and virological variables were selected as potential confounders: public health expenditure per capita (€), population density (inhabitants per km2), average winter temperature, and the predominant influenza virus (sub)type(s). The reasons for inclusion of these variables are described below.
Public health expenditure per capita represents a proxy measure of regional welfare and is commonly used in health-related econometric studies.Citation41–44 Indeed, this parameter varies substantially among the Italian regionsCitation20,Citation44 and has been found to be a significant predictor of regional measles, mumps, and rubella (MMR) vaccination uptake in Italy.Citation44
As per environmental factors, we selected two potential confounders, namely: population density and mean winter temperature regimens. The empirical idea for the former variable was that a higher population density would be associated with a higher virus transmission.Citation37,Citation45 In fact, the population density in Italy is highly non-homogeneous.Citation20 Second, single Italian regions lay in different climatological areas with highly different daily temperature paradigms; this fact could have direct implications on the influenza-related outcomes since the so-called “cold waves” usually interfere with the mortality rate.Citation46 Moreover, Lytras et al.Citation47 have concluded that in Greece the winter excess mortality rates attributable to cold temperatures were substantially higher than those attributable to influenza. Therefore, we proxied the cold waves in a given year and region as an average minimum temperature observed in the winter period. In our analysis, the winter period started at week 40 of the previous year and ended at week 20 of the next year, as per the FluMOMO model.Citation48
Finally, circulation patterns of influenza virus (sub)types (A/H1N1pdm09, A/H3N2 and B) may determine the magnitude of influenza-related outcomes. For instance, in Italy the predominance of the A/H3N2 subtype was associated with significantly higher excess mortality in the elderly.Citation10 The predominance of a single virus (sub)type over other (sub)types was a priori set to 50% of the total national detections. This assumption was however, formally proved by performing a single-proportion z-test. Moreover, the adopted classification rule was compared with the previously published Italian studies,Citation49,Citation50 meeting full agreement. Otherwise [i.e., when the most prevalent virus (sub)type was detected in <50% cases], the overall virological picture was dubbed as co-circulation (Supplementary Table S2).
As recommended,Citation37,Citation51 in all panel regression models performed, the continuous variables (i.e., P&I mortality rates, public health expenditure per capita, population density, and average winter temperature) that were not percentages were transformed using natural logarithms (loge). The regression coefficients are therefore interpreted as elasticities. For instance, the model coefficient for IVC rate should be interpreted as the percent change in P&I mortality rate associated with a 1% change in coverage.Citation37,Citation51
The following panel model specification was considered:
for i = 1 … 21 and t = 2011 … 2017, where “P&I_mort_65+” is P&I mortality rate in subjects aged ≥65 years; bs are regression coefficients; α is the unobserved time-invariant regional effect (in FE model) or constant intercept (in RE model); i is a region; t is a year; ε is the error term; “IVC_65+” is IVC in subjects aged ≥65 years; “IVC_<65” is IVC in subjects aged <65 years “PHexp” is public health expenditure per capita; “Dens” is population density; “Temp” is average low winter temperature; “Virus” is a dummy variable indicating the predominant virus (sub)type.
Taking into account a high probability of heteroscedasticity and/or autocorrelation, all models considered also the Arellano’s heteroscedasticity-autocorrelation (HAC) robust standard errors (SEs). We performed the model diagnostics by applying the Breusch–Godfrey test for panel models to detect serial correlation for the errors and Pesaran cross-sectional dependence (CD) and Breusch–Pagan Lagrange multiplier tests for CD in the constructed panel models.Citation52,Citation53
All the modeling was made in R stats packages.Citation27
Results
Exploratory spatiotemporal analysis of pneumonia- and influenza-related mortality in the Italian older adults aged ≥65 years
Over seven years (2011–2017), a total of 71,876 P&I-related deaths were reported in older adults aged ≥65 years. There was some variability in terms of P&I-related mortality rates observed between years. The highest rates were observed in years 2017 (10.05 per 10,000) and 2015 (8.78 per 10,000). In the remaining years the mortality rate was lower and 6.7 < 8 per 10,000 ().
Figure 1. Choropleth maps of pneumonia- and influenza-related mortality rates (per 10,000) in older adults aged ≥65 years, by year.
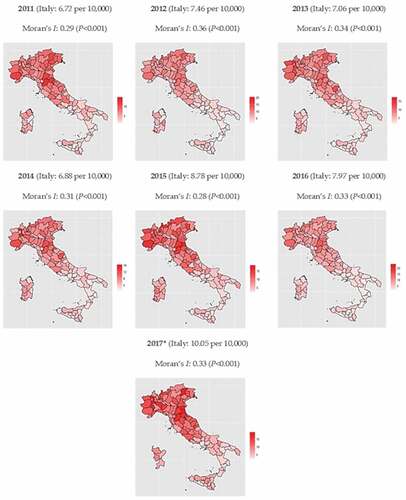
We then explored choropleth charts by mapping province-specific P&I-related death rates. A clear north–south gradient (especially in 2011, 2015, and 2017) was evident: compared with central and southern provinces, those located in the Northern Italy displayed higher P&I mortality rates (). As shown by Moran’s Is, a significant (P < .001) clustered pattern of the observed mortality rates took place in all years with the I–value ranging from 0.28 to 0.36.
The LISA analysis (Supplementary Material, Figure S1) confirmed the north-south gradient: most hotspots and coldspots were located among northern and southern provinces, respectively. The few outliers detected (mainly cold–hot) were located in Sicily.
Association between pneumonia- and influenza-related mortality and influenza vaccination patterns
The total panel was composed of 147 observations and was balanced (i.e., no single space-time observations were missing). reports principal descriptive statistics of the continuous variables of interest. During the study period, an average IVC in the elderly was 55.0%. Two significant drops in IVC were observed: the first occurred in 2012 (from 62.7% to 54.2%), the second in 2014 (from 55.4% to 48.6%). During the study period, only one region reached the recommended target of 75% (Umbria in the 2010/11 season). The proportion of aTIV use was highly non-homogeneous with a range of 0–76% ().
Table 1. Summary statistics of the continuous independent variables considered
For what concerns the seasonal dummy variables, seasons 2011/12, 2013/14 and 2016/17 were dominated by A/H3N2, season 2010/11 by A/H1N1pdm09, seasons 2012/13 and 2015/16 by B type, while the remaining 2014/15 season was ascribed by a co-circulation of A/H1N1pdm09 and A/H3N2 (Supplementary Table S2).
Results of FE and RE panel regression models are reported in . In the FE model, both IVC rate in the elderly, proportion of aTIV use, and average winter temperature were negatively associated with the observed P&I mortality rate in the Italian elderly. In particular, both FE and RE models predicted that each 1% increase in IVC rate in the elderly would be associated (P < .001) with a 1.6–1.9% decrease in P&I mortality. Analogously, each 1% increase in aTIV use would be associated (P < .05) with a 0.4% decrease in P&I mortality. By contrast, the co-circulation of type A virus subtypes was a significant positive predictor. No statistically significant association was observed for other independent variables. The output of the RE model was similar to that of the FE model. However, the Hausman’s test suggested (P < .001) that the FE model should be retained. The model diagnostics justified the use of both HAC robust standard errors ().
Table 2. Panel regression analysis on the association between pneumonia- and influenza-related mortality and potential predictors
Discussion
This study confirms that annual influenza vaccination in the elderly reduces overall P&I mortality rates and therefore that increased immunization rates are desirable. From the ecological and policy-making perspectives this study has also confirmed the usefulness of aTIV in preventing P&I-related mortality in the elderly Italian population. This is in line with a recently proposed concept of the appropriate use of IVs in Italy.Citation54
The protective effect of IV on (excess) mortality is still controversial. For instance, Rizzo et al.Citation10 and Simonsen et al.Citation55 have not documented any meaningful temporal association between IVC rate and winter excess mortality in Italy and the United States, respectively. By contrast, available meta-analyses of observational studiesCitation56,Citation57 suggest a significant reduction in mortality among vaccinated individuals. Contrary to the previous Italian time-trend study by Rizzo et al.,Citation10 we were able to demonstrate a protective effect of IVs on P&I mortality. The reasons for this discrepancy are likely to be multiple. First, two different and non-overlapping time periods with both different circulating viruses and available IVs were assessed. Second, two different proxy outcomes to quantify influenza-related mortality were used. Third, in the present study both time and space were incorporated in the analysis; this may provide additional benefits in countries like Italy with its “jeopardized” pattern of IVCs.Citation58
Our second main finding was that regions using a higher proportion of aTIV showed significantly lower P&I mortality in the elderly independent of IVC, virus circulation pattern, and other potential confounders. In the elderly population aTIV has systematically been shown to be both more immunogenicCitation59 and effectiveCitation60 than standard-dose non-adjuvanted IVs. In particular, meta-analysis by Nicolay et al.Citation59 has shown that the use of aTIV was associated with significantly higher seroconversion rates and geometric mean titers against both vaccine antigen strains and heterologous strains, independently from the (sub)type analyzed. Analogously, the systematic review by Domnich et al.Citation60 concluded that the available observational studies had usually displayed a greater effectiveness of aTIV against various influenza-related outcomes, as compared with non-adjuvanted counterparts. In this regard the ecological study design may confirm findings coming from primary experimental or observational research and may therefore be useful in the decision-making process.
The main strength of this study lies in the methodology adopted. While a limited number of time-space observations does not allow the model to be adjusted for “anything we want to” as well as the fact that some potentially useful data may be not available, the FE panel models provide unbiased estimates in situations when unobserved confounders are present (as in the case of this study).Citation38
Apart from the well-known general limitations of ecological studies (ecological fallacy in primis),Citation16 specific limitations apply to this study that must be considered. First, owing to data availability, the final regression models could be performed at the regional level only. A more space-detailed (e.g., provincial or local health unit level) evaluation is warranted. Second, the data on market share of single IVs come from regional tender allotments and therefore may not exactly correspond to effective IV administration. However, we believe that this limitation had a limited impact on the results since it is unlikely that the wastage/non-utilization rate differs among single IVs. Third, although we have no reason to believe that the automatic coding of causes of death may differ between regions, we could not completely rule out the between-region differences in reporting quality. However, this limitation may have only a small impact on the study results since the Italian National Institute of Statistics performs quality checks on a regular basis. Moreover, the FE panel model adopted may absorb this eventual heterogeneity.
To conclude, our analysis supports the increase in annual influenza vaccination in Italy and suggests that a higher IV uptake in the Italian elderly population would be beneficial. The use of aTIV in older adults is advised to reduce the burden of seasonal influenza disease.
Institutional review board statement
Ethical review and approval were waived for this study, due to the fact that this is based on the publicly available aggregated information sources. No single subject data were available.
Author contributions
Conceptualization, E.F. and A.D.; methodology, E.F. and A.D.; formal analysis, A.D. and A.S.; investigation, E.F. and A.D.; data curation, E.F. A.D. and A.S.; writing—original draft preparation, E.F. and A.D.; writing—review and editing, A.O. and G.I; supervision, A.O. and G.I.; project administration, A.D. All authors have read and approved the submitted manuscript.
Supplemental Material
Download ()Disclosure statement
E.F. is PhD student at Siena University whose program was fully funded by Seqirus, a pharmaceutical company who manufacture and commercialize influenza vaccines. At the time of submission, E.F. became a Seqirus permanent employee. A.D. was a permanent employee of Seqirus at the time study conception and realization. A.S. was remunerated by Seqirus for his statistical analysis. A.O and G.I. declare no conflicts of interest regarding this publication.
Data availability statement
The authors confirm that the data supporting the findings of this study are available within the article and its supplementary materials. In the supplementary materials, Table S1 summarizes all the data sources (and related url) used in the analysis.
Supplementary material
Supplemental data for this article can be accessed on the publisher’s website at https://doi.org/10.1080/21645515.2021.2005381.
Additional information
Funding
References
- Cassini A, Colzani E, Pini A, Mangen MJ, Plass D, McDonald SA, Maringhini G, van Lier A, Haagsma JA, Havelaar AH, et al. Impact of infectious diseases on population health using incidence-based disability-adjusted life years (DALYs): results from the burden of communicable diseases in Europe study, European Union and European economic area countries, 2009 to 2013. Euro Surveill. 2018;23: pii=17–00454. doi:10.2807/1560-7917.ES.2018.23.16.17-00454.
- World Health Organization (WHO). Vaccines against influenza WHO position paper – November 2012. Wkly Epidemiol Rec 2012;87::461–76.
- de Lusignan S, Correa A, Ellis J, Pebody R. Influenza vaccination: in the UK and across Europe. Br J Gen Pract. 2016;66:452–53. doi:10.3399/bjgp16X686677.
- European Centre for Disease Prevention and Control (ECDC). Seasonal influenza vaccination and antiviral use in EU/ EEA Member States. https://www.ecdc.europa.eu/sites/default/files/documents/seasonal-influenza-antiviral-use-2018.pdf .
- Haq K, McElhaney JE. Immunosenescence: influenza vaccination and the elderly. Curr Opin Immunol. 2014;29:38–42. doi:10.1016/j.coi.2014.03.008.
- Tsai TF. Fluad®-MF59®-adjuvanted influenza vaccine in older adults. Infect Chemother. 2013;45:159–74.
- Calcagnile S, Zuccotti GV. The virosomal adjuvanted influenza vaccine. Expert Opin Biol Ther. 2010;10(2):191–200. doi:10.1517/14712590903431014.
- Bragazzi NL, Orsi A, Ansaldi F, Gasparini R, Icardi G. Fluzone® intra-dermal (Intanza®/Istivac® Intra-dermal): an updated overview. Hum Vaccin Immunother. 2016;12(10):2616–27. doi:10.1080/21645515.2016.1187343.
- Wells C, Grobelna A. High dose influenza vaccine for adults: a review of clinical effectiveness, cost-effectiveness, and guidelines. Report No: 1922–8147. Ottawa (ON): Canadian Agency for Drugs and Technologies in Health; 2019 [accessed 2021 April 15]. https://www.cadth.ca/high-dose-influenza-vaccine-adults-review-clinical-effectiveness-cost-effectiveness-and-guidelines .
- Rizzo C, Viboud C, Montomoli E, Simonsen L, Miller MA. Influenza-related mortality in the Italian elderly: no decline associated with increasing vaccination coverage. Vaccine. 2006;24(42–43):6468–75. doi:10.1016/j.vaccine.2006.06.052.
- Bellino S, Piovesan C, Bella A, Rizzo C, Pezzotti P, Ramigni M. Determinants of vaccination uptake, and influenza vaccine effectiveness in preventing deaths and hospital admissions in the elderly population; Treviso, Italy, 2014/2015-2016/2017 seasons. Hum Vaccin Immunother. 2019:1–12. doi:10.1080/21645515.2019.1661754.
- Barbieri M, Capri S, Waure C, Boccalini S, Panatto D. A ge- and risk-related appropriateness of the use of available influenza vaccines in the Italian elderly population is advantageous: results from a budget impact analysis. J Prev Med Hyg. 2017;58(4):E279–E287. doi:10.15167/2421-4248/jpmh2017.58.4.867.
- Italian Ministry of Health. Prevention and control of influenza. Recommendations for 2020–2021 season. https://www.trovanorme.salute.gov.it/norme/renderNormsanPdf?anno=2020&codLeg=74451&parte=1%20&serie=null .
- Boccalini S, Tacconi FM, Lai PL, Bechini A, Bonanni P, Panatto D. Appropriateness and preferential use of different seasonal influenza vaccines: a pilot study on the opinion of vaccinating physicians in Italy. Vaccine. 2019;37(7):915–18. doi:10.1016/j.vaccine.2018.12.057.
- Italian Ministry of Health. Influenza vaccination coverage. http://www.salute.gov.it/portale/influenza/dettaglioContenutiInfluenza.jsp?lingua=italiano&id=679&area=influenza&menu=vuoto .
- Morgenstern H. Ecologic studies in epidemiology: concepts, principles, and methods. Annu Rev Public Health. 1995;16:61–81. doi:10.1146/annurev.pu.16.050195.000425.
- Gasparini R, Bonanni P, Amicizia D, Bella A, Donatelli I, Cristina ML, Panatto D, Lai PL. Influenza epidemiology in Italy two years after the 2009-2010 pandemic: need to improve vaccination coverage. Hum Vaccin Immunother. 2013;9(3):561–67. doi:10.4161/hv.23235.
- Italian National Institute of Health. InfluNet. https://www.epicentro.iss.it/influenza/influnet .
- Italian National Institute of Statistics (ISTAT). Mortality per cause. http://dati.istat.it/Index.aspx?DataSetCode=DCIS_CMORTE1_RES.
- Italian National Institute of Statistics (ISTAT). Health for all. https://www.istat.it/it/archivio/14562 .
- Italian National Institute for the Environmental Protection and Research (ISPRA) 2020. National system for the elaboration and diffusion of climatic data. http://www.scia.isprambiente.it/wwwrootscia/Home_new.html .
- European Commission. European shortlist for causes of death published by Eurostat. https://inspire.ec.europa.eu/document/CoD:1 .
- Moran PA. Notes on continuous stochastic phenomena. Biometrika. 1950;37(1–2):17–23. doi:10.1093/biomet/37.1-2.17.
- Anselin L. Under the hood issues in the specification and interpretation of spatial regression models. Agric Econ. 2002;27:247–67. doi:10.1111/j.1574-0862.2002.tb00120.x.
- Nadkarni P. Core technologies: data mining and “Big Data.” In: Nadkarni P, editor. Clinical research computing: a practitioner’s handbook. Academic Press;2016. p. 187–204. doi:10.1016/B978-0-12-803130-8.00010-5.
- Anselin L. Local indicators of spatial association—LISA. Geogr Anal. 1995;27(2):93–115. doi:10.1111/j.1538-4632.1995.tb00338.x.
- R Core Team. R: a language and environment for statistical computing. Vienna (Austria): R Foundation for Statistical Computing. https://www.R-project.org/ .
- Viboud C, Boëlle PY, Cauchemez S, Lavenu A, Valleron AJ, Flahault A, Carrat F. Risk factors of influenza transmission in households. Br J Gen Pract. 2004;54:684–89.
- Brownstein JS, Kleinman KP, Mandl KD. Identifying pediatric age groups for influenza vaccination using a real-time regional surveillance system. Am J Epidemiol. 2005;162:686–93. doi:10.1093/aje/kwi257.
- Glass LM, Glass RJ. Social contact networks for the spread of pandemic influenza in children and teenagers. BMC Public Health. 2008;8:61. doi:10.1186/1471-2458-8-61.
- Fumanelli L, Ajelli M, Manfredi P, Vespignani A, Merler S. Inferring the structure of social contacts from demographic data in the analysis of infectious diseases spread. PLoS Comput Biol. 2012;8(9):e1002673. doi:10.1371/journal.pcbi.1002673.
- Fabiani M, Volpe E, Faraone M, Bella A, Pezzotti P, Chini F. Effectiveness of influenza vaccine in reducing influenza-associated hospitalizations and deaths among the elderly population; Lazio region, Italy, season 2016-2017. Expert Rev Vaccines. 2020;19(5):479–89. doi:10.1080/14760584.2020.1750380.
- Italian National Institute of Health. FluNews Italia: an integrated influenza surveillance. https://www.epicentro.iss.it/influenza/FluNewsArchivio .
- Rastogi S, Gross PA, Bonelli J, Dran S, Levandowski RA, Russo C, Weksler ME, Kaye D, Levison M, Abrutyn E, et al. Time to peak serum antibody response to influenza vaccine. Clin Diagn Lab Immunol. 1995;2(1):120–21. doi:10.1128/cdli.2.1.120-121.1995.
- Sessa A, Costa B, Bamfi F, Bettoncelli G, D’Ambrosio G. The incidence, natural history and associated outcomes of influenza-like illness and clinical influenza in Italy. Fam Pract. 2001;18(6):629–34. doi:10.1093/fampra/18.6.629.
- Meier CR, Napalkov PN, Wegmüller Y, Jefferson T, Jick H. Population-based study on incidence, risk factors, clinical complications and drug utilization associated with influenza in the United Kingdom. Eur J Clin Microbiol Infect Dis. 2000;19(11):834–42. doi:10.1007/s100960000376.
- Brüderl J, Ludwig V. Fixed-effects panel regression. In: Wolf C, editor. The Sage handbook of regression analysis and causal inference. Los Angeles (CA): SAGE; 2015. p. 327–57.
- Bell A, Fairbrother M, Jones K. Fixed and random effects models: making an informed choice. Qual Quant. 2019;53:1051–74. doi:10.1007/s11135-018-0802-x.
- Goldhaber-Fiebert JD, Lipsitch M, Mahal A, Zaslavsky AM, Salomon JA. Quantifying child mortality reductions related to measles vaccination. PLoS One. 2010;5(11):e13842. doi:10.1371/journal.pone.0013842.
- Hausman J, Taylor W. Panel data and unobservable individual effects. Econometrica 1981;49:1377–98. doi:10.2307/1911406.
- Moreno-Serra R, Anaya-Montes M, Smith PC. Potential determinants of health system efficiency: evidence from Latin America and the Caribbean. PLoS One. 2019;14(5):e0216620. doi:10.1371/journal.pone.0216620.
- Hone T, Mirelman AJ, Rasella D, Paes-Sousa R, Barreto ML, Rocha R, Millett C. Effect of economic recession and impact of health and social protection expenditures on adult mortality: a longitudinal analysis of 5565 Brazilian municipalities. Lancet Glob Health. 2019;7(11):e1575–e1583. doi:10.1016/S2214-109X(19)30409-7.
- Yan I, Korenromp E, Bendavid E. Mortality changes after grants from the Global Fund to Fight AIDS, tuberculosis and malaria: an econometric analysis from 1995 to 2010. BMC Public Health. 2015;15:977. doi:10.1186/s12889-015-2305-1.
- Toffolutti V, McKee M, Melegaro A, Ricciardi W, Stuckler D. Austerity, measles and mandatory vaccination: cross-regional analysis of vaccination in Italy 2000-14. Eur J Public Health. 2019;29(1):123–27. doi:10.1093/eurpub/cky178.
- Chandra S, Kassens-Noor E, Kuljanin G, Vertalka J. A geographic analysis of population density thresholds in the influenza pandemic of 1918-19. Int J Health Geogr. 2013;12:9. doi:10.1186/1476-072X-12-9.
- Rosano A, Bella A, Gesualdo F, Acampora A, Pezzotti P, Marchetti S, Ricciardi W, Rizzo C. Investigating the impact of influenza on excess mortality in all ages in Italy during recent seasons (2013/14-2016/17 seasons). Int J Infect Dis. 2019;88:127–34. doi:10.1016/j.ijid.2019.08.003.
- Lytras T, Pantavou K, Mouratidou E, Tsiodras S. Mortality attributable to seasonal influenza in Greece, 2013 to 2017: variation by type/subtype and age, and a possible harvesting effect. Euro Surveill. 2019;24(14):1800118. doi:10.2807/1560-7917.ES.2019.24.14.1800118.
- Nielsen J, Krause TG, Mølbak K. Influenza-associated mortality determined from all-cause mortality, Denmark 2010/11-2016/17: the FluMOMO model. Influenza Other Respir Viruses. 2018;12(5):591–604. doi:10.1111/irv.12564.
- Puzelli S, Di Martino A, Facchini M, Fabiani C, Calzoletti L, Di Mario G, Palmieri A, Affanni P, Camilloni B, Chironna M, et al. Co-circulation of the two influenza B lineages during 13 consecutive influenza surveillance seasons in Italy, 2004–2017. BMC Infect Dis. 2019;19(1):990. doi:10.1186/s12879-019-4621-z.
- Affanni P, Colucci ME, Bracchi MT, Capobianco E, Zoni R, Caruso L, Castrucci MR, Puzelli S, Cantarelli A, Veronesi L. Virological surveillance of influenza in the eight epidemic seasons after the 2009 pandemic in Emilia-Romagna (Northern Italy). Acta Biomed. 2019;90(9–S):35–44. doi:10.23750/abm.v90i9-S.8722.
- Wooldridge JM. Introductory econometrics: a modern approach. Australia; Cincinnati (OH): South-Western College Pub; 2003.
- Baiocchi G, Distaso W. GRETL: econometric software for the GNU generation. J Appl Econometrics. 2003;18(1):105–10. doi:10.1002/jae.704.
- Croissant Y, Millo G. Panel data econometrics in R: the plm package. J Stat Softw. 2008;27(2):1–43. http://www.jstatsoft.org/v27/i02/ .
- Bonanni P, Boccalini S, Zanobini P, Dakka N, Lorini C, Santomauro F, Bechini A. The appropriateness of the use of influenza vaccines: recommendations from the latest seasons in Italy. Hum Vaccin Immunother. 2018;14(3):699–705. doi:10.1080/21645515.2017.1388480.
- Simonsen L, Reichert TA, Viboud C, Blackwelder WC, Taylor RJ, Miller MA. Impact of influenza vaccination on seasonal mortality in the US elderly population. Arch Intern Med. 2005;165:265–72.
- Yedlapati SH, Khan SU, Talluri S, Lone AN, Khan MZ, Khan MS, Navar AM, Gulati M, Johnson H, Baum S, et al. Effects of influenza vaccine on mortality and cardiovascular outcomes in patients with cardiovascular disease: a systematic review and meta-analysis. J Am Heart Assoc. 2021;10(6):e019636. doi:10.1161/JAHA.120.019636.
- Fukuta H, Goto T, Wakami K, Kamiya T, Ohte N. The effect of influenza vaccination on mortality and hospitalization in patients with heart failure: a systematic review and meta-analysis. Heart Fail Rev. 2019;24(1):109–14. doi:10.1007/s10741-018-9736-6.
- Domnich A, Cambiaggi M, Vasco A, Maraniello L, Ansaldi F, Baldo V, Bonanni P, Calabrò GE, Costantino C, de Waure C, et al. Attitudes and beliefs on influenza vaccination during the COVID-19 pandemic: results from a representative Italian survey. Vaccines (Basel). 2020;8(4):711. doi:10.3390/vaccines8040711.
- Nicolay U, Heijnen E, Nacci P, Patriarca PA, Leav B. Immunogenicity of aIIV3, MF59-adjuvanted seasonal trivalent influenza vaccine, in older adults ≥65 years of age: meta-analysis of cumulative clinical experience. Int J Infect Dis. 2019;85S:S1–S9. doi:10.1016/j.ijid.2019.03.026.
- Domnich A, Arata L, Amicizia D, Puig-Barberà J, Gasparini R, Panatto D. Effectiveness of MF59-adjuvanted seasonal influenza vaccine in the elderly: a systematic review and meta-analysis. Vaccine. 2017;35(4):513–20. doi:10.1016/j.vaccine.2016.12.011.