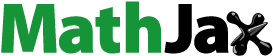
ABSTRACT
A mature developed urban area like London has various policy tools to trigger economic development and leverage poverty. The scale of economic inequality in London has called for more effective policy tools to leverage poverty and close the gaps of economic inequality. Opportunity Areas is one of the tools in urban regeneration to alleviate poverty and develop communities. After over a decade of the policy rollout we have little knowledge how effective this type of policy tool is. The project investigates how effective opportunity areas for economic growth and societal development in London. The key economic indicators including unemployment rate and house price were assessed at MSOA/ward levels in London to understand their spatial variation through GIS mapping. Subsequently, socio-economic factors of commercial land use, culture infrastructure and public transport accessibility were discussed and selected to explore whether they have impacts on the difference of economic performance within opportunity areas. The spatial impacts of these factors among wards/MSOA were evaluated by Geographically Weighted Regression. The research discussed these factors’ variations in Lee Valley, Park Royal and Croydon. Finally, this paper argued that local geographical context has limitations for small area growth corresponding to general policy and strategic management plan.
Introduction
Backgrounds of opportunity areas
Opportunity area was introduced in the London Plan around 2011, representing several chosen key locations with potential for new homes, jobs and infrastructure. It is a form of urban regeneration because the selected sites were previously brownfield lands. The government aims to revive such areas to revitalize the local economy and social activities by investing in constructing new properties and infrastructures (Mayor of London, Citation2011b). In the London Plan, it has been stated that each opportunity area should accommodate about 5000 new jobs and 2500 new homes (Mayor of London, Citation2011a).
As an urban regeneration policy, the opportunity areas have been designated to reduce the severe deprivation situations in many areas of London. illustrates the Index of Multiple Deprivation (IMD), which officially measures relative deprivation in England and is produced by the Ministry of Housing, Communities, and Local Government. The IMD considers a wide range of factors like income, employment, education, and health, reflecting actual local people’s economic activities and living qualities (Elvery, Citation2019). The map shows significant spatial variations of most deprived areas in London. also effectively reflects inequalities in each London borough. Some regions in Barking and Dagenham, Hackney, Haringey, and Tower Halmets would be significantly more deprived than areas like the City of London, Richmond upon Thames and Kingston upon Thames. Therefore, the opportunity areas are initiated in those more deprived areas to revive local economies and reduce spatial inequalities (Donnelly, Citation2020).
Figure 2. Number of most and least deprived areas in London boroughs (Elvery, Citation2019).

As shown in , opportunity areas are widely allocated across different London boroughs and boundaries, covering most deprived areas in London. Some of the main opportunity areas and their names are displayed in . The Mayor may work with boroughs to prepare Opportunity Area Planning Frameworks (OAPFs), specifying how an ‘opportunity area’ should be planned (Drozdz, Citation2020). For example, OAPFs will set the overall objectives for the redevelopment including new housing and jobs, better infrastructure access and high-quality urban built form and facilities to facilitate cultural activities (Mayor of London, Citation2011b). OAPFs will also identify factors and challenges affecting the development of Opportunities Areas, such as land uses, infrastructure provision and public realms.
Figure 3. Opportunity areas allocation in London (Drozdz, Citation2020).

Research questions and research methods justification
As shown in , this article adapts various research methods to primarily address the following two research questions:
Research Question 1: How successful is the Opportunity Area policy in London as an urban regeneration scheme?
This paper will begin by assessing and evaluating the effectiveness of the Opportunity Area policy in London to investigate whether relevant economic performance improved after its implementation.
Research Question 2: Is there endogeneity in the previous analysis? And which local/contextual factors may affect the effectiveness of urban regeneration policies in general?
After evaluating the Opportunity Area policy, this paper aims to delve deeper into the complex urban contexts and explain spatial variations by identifying new contextual factors and variables relevant to urban regeneration policies.
Ultimately, drawing from experiences in London, this paper also expects to offer valuable insights into how cultural, social, and economic factors within the context can lead to diverse outcomes in urban regeneration policies. The article will discuss how its analysis contributes to general research in urban regeneration policies and outlines future research directions for urban regeneration policy-making.
The single-variable analysis involves the GIS spatial analysis method, which overlays the geographical boundaries of opportunity areas with graduated classifications of relevant economic indicators. The spatial analysis method is expected to contribute to answering the first research question by investigating whether the areas covered by opportunity area boundaries exhibit better economic performance. Careful consideration will be given to the selection and justification of appropriate economic indicators, which will serve as dependent variables to measure the impact of Opportunity Areas on economic development. Subsequently, the geographical boundaries of the Opportunity Areas will be mapped and superimposed with the chosen economic indicators at the level of London Wards to ascertain whether the designated Opportunity Areas have indeed led to tangible improvements in local economic performance. It is essential to acknowledge that due to complex factors and challenges influencing the development of Opportunity Areas, regional variations are anticipated in the extent to which local economies around these designated areas exhibit improvements.
Given the intricate interplay of various factors affecting the success of Opportunity Areas, it is worth noting that the Opportunity Area itself can be considered an endogenous independent variable. Furthermore, since Opportunity Area Planning Frameworks (OAPFs) incorporate strategies from diverse aspects, different types of strategies may hold varying degrees of significance in determining the effectiveness of Opportunity Areas in fostering local economic development. Consequently, the second section of the research will focus on selecting additional independent variables based on existing research and empirical evidence. The geographically weighted regression (GWR) analysis will explore how distinct independent variables exert differential impacts on local economies across various areas of London. The GWR is a combination of spatial and statistical methods and it is especially helpful in answering the second research question. Because the GWR can spatially visualize the correlations between other relevant factors like cultural infrastructure and economic indicators to explore if certain areas have remarkably higher correlational coefficients. In addition, GWR can also provide statistical indexes like R-squared to measure to what extent other relevant variables can explain the local economic performance incorporating spatial dimensions.
Finally, the third section of the study will undertake localized analyses to explore and compare how detailed local contexts and factors can influence the success of Opportunity Areas in different locations. The spatial and localised analysis methods adapted in the final section are also useful in addressing the second research question and provide broader informative insights in how local factors may affect urban regeneration policies in general. Multiple locations will be selected to compare and contrast, informing significance of various local social and economic factors in the success of urban regeneration policies. Based on the experience of these case studies, the final discussion will also extract broader insights of how different types of local contextual factors can affect urban regeneration policies and suggest future research directions on strategies to address these complicated factors at the strategic urban and local scales.
Section one: opportunity areas locations and local economic performance improvement
Dependant variable choices & research hypothesis
The overall spatial analysis process is displayed in the . For the first stage, the selection of indicators to assess urban regeneration projects was initially addressed by a commission tasked with evaluating the East Brighton New Deal regeneration program in 1999, as documented by Ness (Citation2001). In the context of this research project, which seeks to investigate the success of urban regeneration efforts, the chosen indicators, acting as dependent variables, must accurately reflect and measure the degree to which urban regeneration objectives have been accomplished. In line with the criteria outlined by the East Brighton Community, appropriate indicators for assessing urban regeneration should capture and quantify changes in both economic and social conditions, aligning with the specific improvements targeted by the regeneration initiatives.
According to the London Plan and various Opportunity Area Planning Frameworks for Croydon, Royal Park, and London Riverside Area, the primary objectives of Opportunity Areas include enhancing employment opportunities, increasing housing supply, and revitalizing local economic activities (Greater London Authority, Citation2011a, Citation2011b, Citation2013). As such, the selected economic indicators should accurately reflect the changes in relevant measurements that the Opportunity Areas aim to improve. Firstly, the unemployment rate can be a pertinent indicator to assess employment opportunities. The spatial research hypothesis is that there will be a decrease in the unemployment rate near the designated Opportunity Areas if more job opportunities are created, as supported by previous research (Lane, Citation2019; Mayor of London, Citation2011a). Secondly, the housing price is also a crucial economic indicator for measuring the effectiveness of urban regeneration projects like Opportunity Areas. Empirical studies have demonstrated that urban regeneration projects may lead to a continuous increase in local housing prices both before and after the completion of relevant reconstruction efforts, which leads to the second research hypothesis (Ki & Jayantha, Citation2010; Liang et al., Citation2019; Property Investor Today, Citation2019). Specific studies conducted in the UK, such as those by CBRE, reveal that most regeneration projects result in ‘house prices within a 750-meter radius of a regeneration zone growing faster than the wider market, by up to 3.6% per annum on average’ (Lane, Citation2019). This is attributed to the significant improvements in living quality, urban environment, and employment opportunities in the local community, which subsequently drive up housing prices.
However, scholars have criticized traditional economic performance indicators for their potential omission of local social and cultural issues. Ambrose (Citation2005) emphasizes the importance of considering diverse factors relevant to public participation and partnership working, in addition to purely economic indicators, when assessing the success of urban regeneration projects. While top-down investment in urban regeneration can lead to local economic growth, Ambrose (Citation2005) points out the risk of such success being isolated and not benefiting the broader communities. This could be attributed to the need for more community participation and a failure to recognize the existing regional unique cultural values and local demographic structures (Ho, Citation1999; Cansu and Osman, Citation2020). Consequently, it is essential to recognize that relying solely on selected quantitative economic indicators may not comprehensively reflect the success of Opportunity Areas from a social and local perspective.
In light of this, it is crucial to acknowledge that unique local contextual factors may significantly influence the effectiveness of Opportunity Areas and their impact on economic performance. Section three of the study will delve into these factors to gain a deeper understanding of their implications on the overall success of Opportunity Areas. By considering social aspects and local perspectives, the research aims to provide a more comprehensive evaluation of Opportunity Areas’ effectiveness in promoting economic development and community well-being. After the variable selection, section one then proceeds to spatial analysis stage as shown in .
Spatial analysis
Rate of increase in housing prices
above displays the spatial distribution of Opportunity Areas in London and the corresponding rate of increase in average housing prices within local regions organized by Wards. Adjustments have been made to housing prices in 2018 by considering the Consumer Price Index (CPI) and the inflation rate to reflect the real changes in housing prices accurately, using the annual CPI in the UK (Rateinflation.com, Citation2022). However, it is essential to note that direct housing prices may only partially capture the effects resulting from the implementation of Opportunity Areas due to the diverse regional and local contexts. To address this concern and better assess the impact of Opportunity Areas, a temporal analysis has been conducted. This analysis involves calculating the percentage increase in housing prices from 2011 to 2018, allowing for a more comprehensive understanding of the changes brought about by the Opportunity Areas policy over the specified period. The calculation process for each region is shown as below in Equationequation (1)(1)
(1) :
= Real housing price increase rate of the region from 2011 to 2018
= Average real housing price of the region in 2018
= Average housing price of the region in 2011
The real housing price of each region in 2018 can be obtained by adjusting to the CPI using 2011 price level as the base, the real housing price is obtained through the following formula (2):
= Real average housing price for each region adjusted to inflation rate in 2018
= Nominal average housing price for each region in 2018
= The consumer price index in the UK for 2018
= The consumer price index in the UK for 2011
reveals that several Wards regions, covered by the allocated Opportunity Areas, exhibit a considerable rate of increase in housing prices, surpassing 0.439. This finding suggests that Opportunity Areas play a role in stimulating local economic activities, leading to a prosperous local economy that, in turn, boosts housing prices. Therefore, the results presented suggest that the first research hypothesis is largely true. Notably, the geographical boundaries of the Opportunity Areas show significant overlap with Wards that experience high housing price growth in areas such as Hounslow, Haringey, Enfield, Hackney, Waltham Forest, and Islington. However, certain outliers are evident, with Canary Wharf being one notable example. Despite being renowned for its high economic activities and housing prices, the map illustrates a more subtle increase in housing prices in the Canary Wharf area. This peculiarity can be attributed to the predominant land use in the area, which heavily leans towards commercial activities rather than residential housing. Additionally, Hammond (Citation2019) has highlighted that although there have been substantial increases in housing supply in the Canary Wharf region, the growth in the local workforce has been slower due to the high average housing prices in the area.
Decrease in the unemployment rate
As illustrated in , opportunity areas also overlap with London Wards areas with higher decreasing rate in the local unemployment rate. It can be identified that wards covered by the opportunity area, such as Greenwich, Haringey, Waltham Forest, Islington and Newham, all have a relatively higher decreasing rate in the unemployment rate, which is generally higher than 0.527. This may imply that the opportunity area helped to revive the local economies and several infrastructure construction projects and other economic policies managed to create more job opportunities for residents. However, some opportunity areas in the west, far east and south of London have lower decrease rates due to transport accessibility and local demographic structures, which will be further explored in section 2 and 3. Unemployment rate data at the Ward level is only available for 2011, as collected during the national census. To conduct the temporal analysis, the unemployment rate at the Ward level can be roughly calculated using the number of job seekers’ allowances divided by the total population aged between 16 and 64 years within each Ward boundary.
The calculation processes are shown as below in Equationequations (3)(3)
(3) and (Equation4
(4)
(4) ):
= Calculated rough unemployment rate in 2019 of the local ward region
= total number of job seekers in each ward region in 2019
= working age population in each ward region in 2019
It should be noted that as the newest UK national census statistics will be gradually fully released in late 2022 and early 2023, there may be more accurate data on unemployment for recent years, which will benefit any further studies on local economic performances. Then the rate of decrease in unemployment rate is calculated as follows in Equationequation (4)(4)
(4) ;
= Decrease rate of the unemployment rate from 2011 to 2019
= Unemployment rate of each ward region in 2011
= Calculated unemployment rate of each ward region in 2019
Section two: multivariate factor analysis
Spatial analysis processes
Section One analyzed geographic correlations between the allocation of Opportunity Areas and identified relevant economic indicators. However, Opportunity Areas encompass diverse strategies targeting various sectors. As shown in the , section two will initially identify essential independent variables pertinent to Opportunity Areas and local economies. Subsequently, it will conduct multivariate regression analysis to determine whether introducing additional independent variables enhances the explanation of economic indicator changes. Geographically Weighted Regression (GWR) will be employed to identify the independent variables with a more pronounced impact on economic indicators and the success of Opportunity Areas across different locations. It should be noted here that the GWR analysis was conducted using the ArcGIS Pro software and the version of the ArcGIS Pro is 2020 version.
The method of GWR is more advantaged than the traditional linear regression method in this case because GWR considers how the spatial variation can partially affect the dependent variables. In the quantitative analysis sector, the spatial dimension can be understood from Tobler’s First Law of Geography: ‘Everything is related to everything else, but near things are more related to each other’. Brunsdon et al. (Citation1998) initiated the idea of Geographically Weighted Regression, which considers the spatial dimension while conducting linear regression analysis. Therefore, apart from the correlation between the dependent and independent variables, the significance of independent variables may also vary due to spatial geographic spaces. GWR also allows users to analyse multiple independent variables together to investigate individual independent variables’ significance and R-square values.
Before conducting the GWR analysis, it should be checked if both dependent variables samples follow a normal distribution to ensure the GWR analysis’ validity. This test is conducted and can be found in Appendix 1.
Independent variable choices
Lawless and Gore (Citation1999) have studied the urban regeneration program in Sheffield from 1992–1996 and initiated five main influential factors which affect the success of regeneration policies. These five factors include image, property development, land use, business locations and types and labour market. The following independent variables are selected based on this initiative.
Public transport accessibility
Public transport accessibility serves as an independent variable in this study due to its potential impact on Opportunity Area development and local economic performance, especially in high-density cities like London and Hong Kong. In Hong Kong, previous research has demonstrated its pivotal role in influencing local economic and social dynamics. Moreover, it closely intertwines with urban regeneration and renewal processes (Wang et al., Citation2022; Leeand Chan,Citation2008). Scholarly discourse has argued that transport accessibility represents more than just convenience; it embodies the ‘right to the city’. Improved transport accessibility provides access to a broader range of job opportunities and significantly affects commuting times (Wang et al., Citation2022). Consequently, the equitable distribution of public transport accessibility within local communities is a matter of concern. Wang et al. (Citation2022) highlight that unequal accessibility in specific urban regeneration projects could exacerbate inequalities and gentrification issues as areas with higher transport accessibility gain advantages.
Commercial land use
The allocation of land use and site planning can significantly impact the performance of urban regeneration programs and local economies. Oléron-Evans and Salhab (Citation2021)onducted a case study based on the Heathrow Opportunity Area, exploring the optimal allocation of local land uses to maximize housing delivery, job opportunities, and gross value added. Using Glover Martinson’s method for multi-objective optimization, they found that suitable land use allocations for maximizing employment opportunities and GVA creation prioritize financial services, office-based businesses, and shops.
In the London context, site allocation data can help analyze the relationship between land use allocation, Opportunity Areas, economic performance, and explain regional variations in Opportunity Areas’ performance and economic development. Points of interest (POI) data can provide a comprehensive way to analyze local land use and site allocation. Therefore, we retrieved London’s POI data using Python code and the OSMnx package from the OpenStreetMap data store and its API (Boeing, Citation2017). We specifically focused on commercial building POIs, as urban regeneration projects around intensified commercial areas are expected to create more local employment and improve economic performance (Dixon, Citation2005). Then centroid points are created in relation to these commercial buildings and counted them within each ward region.
Cultural infrastructure
Indeed, criticisms of Opportunity Areas have arisen due to concerns that they may prioritize financial values over other essential factors, potentially neglecting public participation and social considerations (Ho, Citation1999; Parkinson, Citation1988). Hwang (Citation2014) argues that urban regeneration plans should be carefully crafted, considering the local community’s specific characteristics and needs. This is because the economic vitality of a place is deeply intertwined with its unique cultural environment (Montgomery, Citation2003). Therefore, a successful and comprehensive urban regeneration plan should actively consider the cultural activities and historical context of the sites under redevelopment. Enhancing community participation is vital to enable planning authorities to better understand and appreciate the significant cultural characteristics residents value. In light of these considerations, this study selects cultural infrastructure as another independent variable. Cultural infrastructure represents the local social infrastructure capacity and has been shown to be beneficial in guiding local planning decisions (Maginn, Citation2007).
Research hypothesis
Therefore, as section 2.1 provides the reasons behind choosing the GWR research method and section 2.2 provides the theoretical framework of the independent variables selections of public transport accessibility, commercial land use and cultural infrastructure, the spatial and statistical research hypothesis may be listed as follows:
In general statistical aspects, the R-squared and adjusted R-squared should be at decent ratio, indicating that introduction of new independent variables will contribute to the variation in economic performances
From a spatial perspective, the classifications of coefficients may display some particular patterns, which may either limit or promote the opportunity area policy.
Geographically weighted regression analysis: housing price change as the dependent variable
The figures above illustrate the output of the Geographically Weighted Regression analysis. And the maps are mapped using the value of coefficients of three selected independent variables (commercial land use, transport accessibility and cultural infrastructure) respectively, while the dependent variable is the housing price increase rate.
General statistical results presentation & discussion
Firstly, the R-squared value for each of the three independent variables is relatively close (0.3956 VS 0.4041 VS 0.3975). This indicates that about 40% of the housing price change spatial variations can be explained using the selected independent variables and the explanatory power of each of the three independent variables are very similar for housing price increase. Even though this is a decent explanatory percentage weighting, this also indicates that there may potentially be other localized variables contributing to explaining the spatial variations in London housing price increase. Moreover, if all three independent variables are considered together, the produced R-square output (0.4304) is larger than each of the independent variables’ R-square values. With the adjusted R-square being positive at 0.2517, adding more independent variables will help explain the housing price change spatial variations better.
In general, the results presented above suggest that the first statistical research hypothesis may hold true when using housing prices as the dependent variable. This finding is significant for two main reasons. Firstly, the decent R-squared and adjusted R-squared values validate the selection of independent variables, indicating their high explanatory power. Secondly, these results contribute to addressing the second research question of this paper. The high explanatory power suggests that public transport accessibility, cultural infrastructure, and commercial land uses are all pertinent factors in urban regeneration schemes. Furthermore, they may exert a significant influence on the effectiveness of the Opportunity Area policy in London
Spatial correlation results presentation
The three maps above depict varying significance levels of the selected independent variables concerning housing price increases in different areas of London. indicates that an increase in commercial land use can lead to faster housing price growth, particularly in London’s Northwest and Southeast regions, with coefficients exceeding 0.0096. This phenomenon may be attributed to the potential growth capacity for commercial development in areas like Barnet, Brent, and Bromley (CEBR, Citation2019).
Transport accessibility demonstrates a similar geographic pattern, albeit over a more expansive area. illustrates that potential improvements in transport accessibility can significantly boost housing prices in the north, northwest, west, southeast, and south regions of London, with coefficients generally exceeding 0.0094. Notably, these areas with higher accessibility coefficients tend to be situated on the city’s outskirts. Given their relatively lower accessibility to the city center, which offers more employment opportunities and entertainment, any enhancement in local transport accessibility has the potential to substantially increase housing prices by reducing commuting time costs. This, in turn, allows local communities to better connect with employment opportunities (Wang et al., Citation2022).
Conversely, in the , cultural infrastructure’s significance is primarily concentrated in the southeast and west areas, particularly in the boroughs of Hammersmith & Fulham, Enfield, and Waltham Forest, where the coefficients generally exceed 0.004. This suggests that while these areas have well-developed commercial land uses, they may lack cultural infrastructure, such as entertainment facilities.
Spatial correlation results discussion & insights
In summary, the second spatial research hypothesis may be accepted as well as each of the selected independent variable all display some distinctive geographic patterns. This also contributes to the understanding of the second overall research question at the local and contextual scale because these spatial results illustrate that factors may have various significance at multiple locations. For example, the commercial land use appears to have the highest correlation coefficients in Brent, which means that the Wembley Opportunity Area’s effectiveness in economic performance may be greatly affected by other commercial land developments. In addition, as suggests that transport development in peripheral areas may be much more significant than other policies like opportunity areas there.
However, these results can also provide insights in the urban regeneration policies in two ways. Firstly, these results would be beneficial for policy-makers to recognise the importance of local contexts and avoid the ‘standardized’ policy for all areas. And local governments should take the responsibility of making individualized development schemes and urban regeneration policies and also increase the public participation (reference). Secondly, different coefficients also provide directions for policy-makers to improve the regeneration schemes as they can include extra policies addressing the sector which may be predicted to be the most beneficial for improving local economic performance (i.e. has the highest correlational coefficients with the economic indicator).
Geographically weighted analysis: unemployment decrease rate as the dependent variable
General statistical results presentation & discussion
The R-squared value of the commercial land use (0.7111) is significantly higher than the other two independent variables, implying that commercial land use may have the most substantial explanatory power in spatial variations of unemployment decrease. The first sectional research hypothesis is only partially accepted as while the R-squared value of the transport accessibility is decent at 0.4329, the value of cultural infrastructure is especially lower than the other two independent variables at 0.2057, indicating that only 20% of spatial variations in unemployment decrease may be explained by cultural infrastructure. Moreover, the overall R-square value for three independent variables is 0.7672. It is higher than any individual independent variables’ R-square value, which indicates that adding more selected independent variables may help to explain spatial variations better and decrease estimation bias. This reinforces the conclusion in the early section 2.4.1 that the selection of three variables (cultural infrastructure, commercial land-use and transport accessibility) may be appropriate independent variables as they are relevant to economic performance indicators like housing prices and decrease in unemployment rate. However, the cultural infrastructure has lower explanatory power, meaning that there are potentials of discovering more significant variables which are more relevant to decreasing the unemployment rate under urban regeneration contexts. This may be expected in some metropolitan cases as Rius-Ulldemolins (Citation2014) suggested that the cultural infrastructure plays more like an instrumental and subordinate role in facilitating urban regeneration processes rather than the main influential factors.
Spatial correlational results presentation
Once again, the three maps above highlight the varying significance of different independent variables in different local regions of London. demonstrates that improvements in commercial land use can lead to a more significant drop in the unemployment rate in the west, northwest (Barnet, Harrow, Hillingdon), middle (Hackney, Kensington, and Chelsea), and south areas (Sutton), with coefficients exceeding 0.0106. Notably, the spatial pattern of these commercial coefficients mirrors the distribution of housing price increases, with the highest coefficients concentrated in the west and northwest areas.
In contrast, cultural infrastructure appears to be significant primarily in northern and northwestern boroughs such as Enfield and Harrow. Transport accessibility is more critical for reducing local unemployment in areas farther from the city center, following a pattern similar to the distribution of accessibility coefficients in relation to housing price increases. As illustrated in , areas in the north, east, and south boroughs exhibit more substantial coefficients, exceeding 0.0056.
Spatial correlational results discussion
Firstly, based on the previous results and , it is intriguing to observe that the three-variable Geographically Weighted Regression (GWR) analysis for reducing the unemployment rate exhibits a similar spatial concentration pattern to the analysis for increasing housing prices, despite differences in the spatial concentration of the cultural infrastructure coefficients. This alignment in the spatial analysis correlation for unemployment rate reduction further affirms the appropriateness of the selection of independent variables, reinforcing the acceptance of the second research hypothesis. Both GWR analyses reveal distinct and comparable geographic patterns for two economic indicators, enhancing the reliability of the results.
Overall, Section 2 contributes to addressing the second research question in this article by identifying various economic, social, and urban variables relevant to local economic performance. These variables will impact the comprehensive assessment of the Opportunity Area policy. Furthermore, this section highlights the significance of local contextual factors, which exhibit varying correlation coefficients across different locations in London, often following distinct geographic patterns. Sometimes local contextual factors will also significantly affect the urban regeneration schemes (Wainwright, Citation2021). Additionally, this section suggests that spatial analysis using GWR can provide valuable insights for the development of strategies in local urban regeneration policies by identifying the most significant variables for local development.
Section three: localized analysis
Based on the results of the Section One and Section Two analysis, it is expected that the designated opportunity areas and more explanatory variables introduced in section 2 can explain some improvement in local economies. However, there may also be other local factors contributing to spatial variations. Therefore, this section aims to zoom the analysis into a smaller scale by selecting typical sites and explore their unique local contextual factors. The typical areas may be defined with particularly significant or insignificant improvement in the local economic indicators. The areas with contrasting economic situation are preferred to be selected as the local factors affecting the effectiveness of opportunity areas would be more contrasting and obvious to identify.
Summary of selected areas
The selection of sites and localized analysis is based on three primary aspects. Firstly, the chosen area must fall within the designated geographical boundaries of London Opportunity Areas to investigate the effects of the Opportunity Area policy effectively. Secondly, economic indicators were considered because they serve as measures of local economic success and the effectiveness of Opportunity Areas. Lastly, the coefficients of independent variables from Section two were taken into account, as they can provide valuable insights into the most essential and effective strategies for enhancing local economies. The Geographically Weighted Regression (GWR) analysis allows users to obtain specific coefficients and R-squared values for each ward area, facilitating localized analysis.
As a result of these criteria, several ward regions overlapping with Opportunity Areas’ boundaries in Enfield, Kensington, Chelsea, and Croydon were selected for localized analysis, as shown in . These areas exhibited both significant increases in housing prices and decreases in unemployment rates. Therefore, the goal is to uncover the reasons behind the successful economic improvements in these Opportunity Areas. In contrast, Croydon was also chosen for comparison analysis because neither of its economic indicators improved significantly. Consequently, a local investigation will be conducted to recommend future strategies for more effective local economic enhancement.
Relevant coefficients, t values, and economic improvement have been stated in . The statistical significance of coefficients is determined by calculating the T values and comparing if the absolute T value is larger than the T-test statistic at a 10% significance level (1.282). The statistically significant coefficients are highlighted using stars and will be discussed later. The following formula (5) was used to obtain the T value for each selected regions based on the GWR output:
Table 1. Summary of statistics for selected Areas*a.
= T Value
= The coefficient value of the independent variable from GWR
= The standard error of the coefficient value
Lee Valley opportunity area localized analysis
As shown in the , the selected opportunity area at the boundary between Enfield and Haringey displays a diversified and active overall pattern. Firstly, this area is enclosed between two main transport networks with crucial transport stations like Meridian Water, White Hart Lane, and Silver Street at an accessible distance. The local transport accessibility score is 10.6, which is generally higher than surrounding areas (around 4.3–7.2). Secondly, the land use pattern is diversified with a decent amount of lands devoted to water-bodies (Banbury Reservoir, Lockwood Reservoir), green-spaces (Tottenham Marshes), cultural infrastructure (Tottenham Hotspur Stadium), and commercial uses (West Mews). There are 42 cultural infrastructures concentrated in this opportunity area, and about 28% of lands are designated for commercial/business use (Greater London Authority, Citation2011c). Since the balance between different land use types is maintained well with high transport accessibility, this opportunity area achieved decent progress in relevant economic indicators (0.586 and 0.41). Furthermore, there are still capacities for potential growth reflected in the high coefficients of accessibility (0.0113 & 0.0072) and cultural infrastructure (0.0042 & 0.0057).
The table suggests that transport accessibility and local cultural infrastructure may serve as more effective strategies for improving economies and achieving the objectives of the Opportunity Area. They have higher coefficients (0.0113 & 0.0042 vs. 0.0006 for housing price increase and 0.0072 & 0.0057 vs. −0.0142 for unemployment decrease) and are statistically significant. This aligns with certain criticisms of Enfield’s local plan. Enfield’s local plan aims to implement a new regeneration scheme, involving the construction of more than 6,000 homes and mixed-use commercial spaces on current Greenbelt lands (Enfield Council, Citation2019). However, this scheme has sparked controversy, with residents expressing opposition. They are concerned that the redevelopment of brownfield land areas designated by the local plan may encroach upon a vast amount of Greenbelt lands, potentially harming local conservation efforts and cultural heritage that residents hold dear (Cracknell, Citation2021).
Furthermore, the local campaign group, Better Homes Enfield, has argued that further development of commercial land uses may be ‘overwhelming’ and ineffective for promoting local economic development (Cracknell, Citation2021). In summary, both local plans and studies indicate that further commercial land use development may be ‘overwhelming’ and may not effectively benefit local development. Additionally, there should be increased attention to cultural conservation in future urban regeneration strategies.
Park Royal opportunity area localized analysis
According to local land use profiles, 38% of the land in the Park Royal Opportunity Area is dedicated to storage and distribution, 14% to industrial purposes, 8% to motor trades, and 5% to business (Greater London Authority, Citation2011b). The Park Royal Opportunity Area primarily consists of several industrial parks, such as Origin Business Park and Matrix Industrial Park in the west, and the Central Business Park on Action Lane. In the east, you’ll find key transportation nodes, including the Old Oak Common station, Depot, and Willesden Substation in the north. However, the local transport accessibility score is just 7.3 due to a relative shortage of local bus stops (Enfield Council, Citation2019). Notably, there is also a Powerday Recycling Centre located northeast of the Opportunity Area, which will be discussed in the following paragraph as a crucial variable influencing local resources, land use allocation, and economies. Overall, the Park Royal Opportunity Area is predominantly comprised of industrial parks and land dedicated to logistics and storage, with several transportation nodes as well. It has achieved a decent improvement in economic performance, indicated by increases in housing prices and a decrease in the unemployment rate (0.497 & 0.58). However, the number of local cultural infrastructures may be lower compared to the other two selected sites (19 vs. 42 and 48 for the other sites).
The GWR statistical outputs in illustrate that coefficients of transport accessibility (0.0079) are higher than coefficients of cultural infrastructure (0.0063) and commercial land uses (−0.0013) for the housing price increase. Empirical studies proved that there are other local factors like circular economies with waste management systems, which may help improve local economies directly or indirectly by supporting better transport infrastructure provisions. Scholars from University College London argued that there is an embedding circular economy principle into urban regeneration and waste management scheme in some opportunity areas in London, including Ealing and Hammersmith, and Fulham, which helped to maximize local optimal resources use and allocation, improving the local urban environment and housing prices (Domenech & Borrion, Citation2022). This argument is underpinned by the existing Powerday Recycling Centre highlighted in the red circle in which specializes in efficient waste management circulation (Powerday, Citation2021). The Park Royal OAPF also stated that more lands would be required to accommodate facilities for waste management (Greater London Authority, Citation2011b). The local waste management system contribute to forming a circular economy of regeneration and waste management, encouraging effective transport and other soft infrastructural provisions (Domenech & Borrion, Citation2022; McDowall et al., Citation2017). The housing price may also rise because the waste management system enhances urban environments.
In contrast, the GWR analysis showed that all three independent variables seem to negatively affect the local unemployment rate decrease (−0.0142, −0.0027, and −0.0012), but only the commercial land use coefficient is statistically significant. The local plan and the Opportunity Area Planning Framework stated potential problems with existing commercial lands. Firstly, Hammersmith & Fulham borough expressed concerns regards the incremental expansion of car retail activity. This is because the overwhelming expansion may harm the potential small business initiatives and economic diversification (Greater London Authority, Citation2011b). Secondly, most local commercial developments were constructed over the past 25 years and may be incapable of modern business operational requirements. A higher design standard is required to meet the modern technological and operational needs (Greater London Authority, Citation2011b).
Croydon opportunity area localized analysis
According to the , there are 48 cultural infrastructures concentrated in the local area, such as theatres, libraries, and museums around Barclay Road and Wellesley Road. Moreover, on the west side of the opportunity area, there are also vast green-space parks and shopping locations for commercial land uses. The also shows that 50% of local lands were developed as commercial lands. The also illustrates a detailed land use allocation of the opportunity area, with commercial use being the most popular classification. Therefore, they are already plenty of cultural infrastructures and commercial land planned locally, which may limit the development capacity. From the transport accessibility perspective, the local transportation networks are very effective compared to other nearby regions in Croydon, as its transport accessibility scores are significantly higher than surrounding areas (25.3 VS 5.4, 8.3, and 10.2). This may explain the most significant coefficient in transport accessibility in this opportunity because in areas far from the city centre, the accessibility would be appreciated as it connects local areas with better economic and employment opportunities, reducing commuting time costs effectively.
Figure 18. Local land uses map (Greater London Authority, Citation2011b).

Figure 20. Local land use percentages (Greater London Authority, Citation2013).

Nevertheless, in Croydon, both economic indicators are not improved significantly (0.426 and 0.21), which may be mainly due to local commercial and industrial structures. According to the Croydon OAPF, its land use redevelopment approach focuses on delivering 7300 new residential buildings and supporting the development of 95,000 square meters of new commercial space (Greater London Authority, Citation2013). However, Clifford et al. (Citation2019) assessed the resident-to-office land use redevelopment in Croydon and criticized some issues raised by residential and commercial housing deliveries. Due to the large number of units planned, the residential quality and overall local services industry may not be sufficient to accommodate such fast expected economic growth. As the residential spaces constitute only 6% of local land uses, it is also questioned whether residential can facilitate planned growth.
What is more, the local commercial, and industrial structure is unbalanced. There is a lack of commercial base industries like supermarkets or restaurants which can support the upper-level business in offices (Clifford et al., Citation2019). Consequently, people may find these local places more boring and depressing without sufficient commercial services to facilitate their daily needs. Furthermore, the housing prices and unemployment rate decrease may not be improved well as people may be held back from moving into the Croydon area.
Summary of localized analysis
In summary, the localized analysis reveals a broader range of factors affecting the local policy effectiveness. These factors also expose limitations of selected variables as they fail to consider social and unique local elements. From previous case studies and localized analysis, the contextual economic and social factors affecting urban redevelopment are categorized as below:
The over-speed commercial redevelopment may suffer from quality problems and even harm local development because other locally valued assets like greenspaces and cultural infrastructures are threatened.
There can be unbalancing in local commercial structure. For example, the local over-expanded business may restrict the emergence of more innovative smaller-scale businesses, harming local economic diversification and employment opportunities.
Sometimes the upper-commercial industries are not supported by sufficient number of base service industries.
Conclusion
The first section of this paper addressed the first research question using a spatial analysis method. Through the overlay of economic indicator improvements and the geographical boundaries of designated Opportunity Areas, it became evident that substantial geographical overlaps exist between areas with the highest decrease in unemployment rates or increases in housing prices and the allocated Opportunity Areas. This observation suggests a spatial correlation between Opportunity Areas and local economic improvement, despite the presence of some spatial variations. Consequently, the Opportunity Area policy in London can be considered overall successful, as most of the areas covered by Opportunity Areas showed significant improvements in economic indicators. However, some Opportunity Area-covered areas did not exhibit obvious economic improvement, leading to the exploration of the second research question, which delves into the complex multi-factorial influences on urban regeneration and development.
Section two firstly identified three critical factors, including commercial land use, transport accessibility levels, and cultural infrastructure, which may affect the effectiveness of Opportunity Areas and local economic performance. Then, the Geographically Weighted Regression analysis is conducted using selected independent variables concerning two economic indicators individually. The results helped with answering the second research question effectively in two areas. Firstly, the general statistical analysis confirms the previous hypothesis that there would be diverse social and economic factors affecting urban redevelopment and local economic development. Moreover, the GWR also illustrates that local geographical content may influence urban regeneration and development as independent variables’ coefficients follow specific concentrating patterns.
Additionally, this spatial and factor analysis section provides three informative insights. Firstly, it suggests that GWR analysis can assist in local urban regeneration policy-making by indicating which social or infrastructural factors can predict the most economic growth in specific local locations. Secondly, Section 2 leads to localized discussions in Section 3 to explore whether locally significant factors indeed impact urban regeneration and economic growth. Moreover, it argues that local governments should take responsibility for managing growth, improving the planning process, and implementing local urban regeneration schemes.
Section three aims to zoom into local contexts by selecting typical and contrasting sites and exploring more localized reasons that cause spatial coefficient variations. Localized factors may form the critical aspect of the research project as they supply explanations from more realistic and social aspects, which are not considered in the previously selected economic variables. The Lee Valley and Park Royal Opportunity Area have been selected as successful examples, while Croydon Opportunity Area is selected as a comparison. Documentary analysis and discussions reveals that, generally, increased commercial land use may not improve the local economies effectively with lower/negative coefficients in all selected local areas (shown in the second column of ) due to the local commercial and industrial structures. Secondly, it should also be recognized that some local unique contextual factors may affect local economic performances significantly. For example, the waste management facilities are crucial for sustainable economic growth in the Park Royal Opportunity Area; Some areas further from the city Centre, like Croydon, has transport accessibility as the vital factor in improving local economies. Additionally, the Greenbelt lands are greatly valued as local cultural assets in the Lee Valley Opportunity Area.
These local factors supplement the limitations of previously selected economic variables. Therefore, when implementing strategic planning and regeneration projects, the local authority and planners should always conduct detailed local analysis to understand local areas’ unique features and demands from sustainability and cultural aspects so that more effective economic growth may be achieved and people may be more willing to stay to live and work. This discussion also reinforces the point made in section 2, which argues that local governments should take responsibilities and increase its capacity in addressing local problems while making urban regeneration plans.
Future research directions
These local factors supplement the limitations of previously selected economic variables. Therefore, when implementing strategic planning and regeneration projects, the local authority and planners should always conduct detailed local analysis to understand local areas’ unique features and demands from sustainability and cultural aspects so that more effective economic growth may be achieved and people may be more willing to stay to live and work. This discussion also reinforces the point made in section 2, which argues that local governments should take responsibilities and increase its capacity in addressing local problems while making urban regeneration plans.
Overall, this paper thoroughly examines the Opportunity Areas in London from both statistical and geographical perspectives, employing econometric and spatial analysis methods. The paper’s conclusions and insights raise two key research questions:
What are the other factors affecting urban regeneration schemes?
When formulating urban regeneration plans, how can governments and planners effectively address local contexts?
Future research can focus on identifying additional factors relevant to urban regeneration, utilizing geospatial and econometric methods to assess these factors’ relevance and localized effects. Furthermore, research efforts should explore ways to incorporate specific local contexts into local regeneration plans. For instance, local participation has been widely discussed as a means to facilitate urban affairs such as public housing and infrastructure planning (Barton et al., Citation2005; Coutinho-Rodrigues et al., Citation2011). By integrating geographic, econometric methods, and institutional research, it is expected that these research directions can form a comprehensive mechanism to enhance urban regeneration schemes, taking local contexts into account.
Author Contributors
The author’s associated institution has changed in September 2023, when this paper was written, the associated institution was UCL. Currently, the author’s institution is the University of Cambridge, Department of Architecture. Email: [email protected].
However, since the author was associated with UCL when the paper was written, UCL was put here as the associated institution. If there are any further problems, please contact the author.
Acknowledgments
I would like to thank Mrs. Weigang Yan, a researcher at the Land Economy Department at the University of Cambridge. She continuously provided feedback and suggestions throughout this research project.
Disclosure statement
No potential conflict of interest was reported by the author.
Data availability statement
All the datasets used in this research paper can be obtained through some open access portals:
Data Store, L., 2022. Opportunity Areas – London Datastore. [online] Data.london.gov.uk. Available at: <https://data.london.gov.uk/dataset/opportunity_areas> [Accessed 17 July 2022].
London Data Store., 2019. Average House Prices by Borough, Ward, MSOA & LSOA – London Datastore. [online] Data.london.gov.uk. Available at: <https://data.london.gov.uk/dataset/average-house-prices> [Accessed 17 July 2022].
London Data Store, 2019. Cultural Infrastructure Map – London Datastore. [online] Data.london.gov.uk. Available at: <https://data.london.gov.uk/dataset/cultural-infrastructure-map> [Accessed 17 July 2022].
London Data Store., 2011. Ward Profiles and Atlas – London Datastore. [online] Data.london.gov.uk. Available at: <https://data.london.gov.uk/dataset/ward-profiles-and-atlas> [Accessed 17 July 2022].
London Data Store., 2015. Public Transport Accessibility Levels – London Datastore. [online] Data.london.gov.uk. Available at: <https://data.london.gov.uk/dataset/public-transport-accessibility-levels> [Accessed 16 July 2022].
London Data Store., 2019. Indices of Deprivation – London Datastore. [online] Data.london.gov.uk. Available at: < https://data.london.gov.uk/dataset/indices-of-deprivation> [Accessed 1 September 2023].
Nomis – Official Census and Labour Market Statistics. 2019. [online] Available at: <https://www.nomisweb.co.uk/> [Accessed 17 July 2022].
Dataset of POIs’ DOI is referenced as below:
References
- Ambrose, P. (2005). Urban regeneration: Who defines the indicators? In D. Taylor & S. Balloch (Eds.), The politics of evaluation: Participation and policy implementation (pp. 41–33). https://doi.org/10.46692/9781847421210.004
- Barton, J., Plume, J., & Parolin, B. (2005). Public participation in a spatial decision support system for public housing. Computers, Environment and Urban Systems, 29(6), 630–652. https://doi.org/10.1016/j.compenvurbsys.2005.03.002
- Boeing, G. (2017). Osmnx: New methods for acquiring, constructing, analyzing, and visualizing complex Street networks. Computers, Environment and Urban Systems, 65, 126–139. https://doi.org/10.1016/j.compenvurbsys.2017.05.004
- Brunsdon, C., Fotheringham, S., & Charlton, M. (1998). Geographically weighted regression. Journal of the Royal Statistical Society: Series D (The Statistician), 47(3), 431–443. https://doi.org/10.1111/1467-9884.00145
- Cansu, K., & Osman, B. (2020). Sustainability of urban regeneration in Turkey: Assessing the performance of the north Ankara urban regeneration project. Habitat International, 95(102081), ISSN 0197–3975. https://doi.org/10.1016/j.habitatint.2019.102081
- CEBR. (2019). London’s business base: A report for London councils. rep. London Councils.
- Clifford, B., Ferm, J., Livingstone, N., & Canelas, P. (2019). The Croydon story: Slums of the future? In Understanding the impacts of deregulation in planning (pp. 83–110). Palgrave Pivot. https://doi.org/10.1007/978-3-030-12672-8_7
- Coutinho-Rodrigues, J., Simão, A., & Antunes, C. H. (2011). A GIS-based multicriteria spatial decision support system for planning urban infrastructures. Decision Support Systems, 51(3), 720–726. https://doi.org/10.1016/j.dss.2011.02.010
- Cracknell, J. (2021). Sadiq Khan set to object to green belt housing plans. Enfield Dispatch. Retrieved July 30, 2022, from <https://enfielddispatch.co.uk/sadiq-khan-set-to-object-to-green-belt-housing-plans/>.
- Dixon, T. (2005). The role of retailing in urban regeneration. Local Economy: The Journal of the Local Economy Policy Unit, 20(2), 168–182. https://doi.org/10.1080/13575270500053266
- Domenech, T., & Borrion, A. (2022). Embedding circular economy Principles into urban regeneration and waste management: Framework and metrics. Sustainability, 14(3), 1293. https://doi.org/10.3390/su14031293
- Donnelly, M. (2020). Deputy mayor overrules borough to allow 5,000-home scheme on east London industrial site [online]. Retrieved July 18, 2022, from <https://www.planningresource.co.uk/article/1691465/deputy-mayor-overrules-borough-allow-5000-home-scheme-east-london-industrial-site>.
- Drozdz, M. (2020). Is global urbanism in crisis? The case of London. L’ Institut Paris Region, Retrieved July 31, 2022, from <https://en.institutparisregion.fr/know-how/urban-planning/cities-change-the-world/is-global-urbanism-in-crisis-the-case-of-london/>.
- Elvery, M. (2019). The wealthiest and most deprived areas of London have been revealed. MyLondon. Retrieved July 31, 2022, from <https://www.mylondon.news/news/zone-1-news/wealthiest-most-deprived-areas-london-17171816>.
- Enfield Council. (2019). The Enfield local plan. London: Enfield Council
- Greater London Authority. (2011a). London riverside Opportunity Area planning framework. London: Greater London Authority.
- Greater London Authority. (2011b). Park Royal Opportunity Area planning framework. London: Greater London Authority.
- Greater London Authority. (2011c). Upper Lee Valley Opportunity Area planning framework. London: Greater London Authority.
- Greater London Authority. (2013). Croydon Opportunity Area planning framework. London: Greater London Authority.
- Hammond, G. (2019). Canary Wharf has a flood of new homes but only a trickle of returning workers. Financial Times
- Ho, S. Y. (1999). Evaluating urban regeneration programmes in Britain: Exploring the potential of the realist approach. Evaluation, 5(4), 422–438. https://doi.org/10.1177/135638999400830084
- Hwang, K. (2014). Finding urban identity through Culture-led urban regeneration. Journal of Urban Management, 3(1–2), 67–85. https://doi.org/10.1016/S2226-5856(18)30084-0
- Ki, C., & Jayantha, W. (2010). The effects of urban redevelopment on neighbourhood housing prices. International Journal of Urban Sciences, 14(3), 276–294. https://doi.org/10.1080/12265934.2010.9693685
- Lane, M. (2019). The regeneration effect – new research suggests it results in strong price growth. Property Investor Today. Retrieved July 31, 2022, from <https://www.propertyinvestortoday.co.uk/breaking-news/2019/11/the-regeneration-effect–new-research-suggests-regeneration-results-in-strong-price-growth>.
- Lawless, P., & Gore, T. (1999). Urban regeneration and transport investment: A case study of Sheffield 1992-96. Urban Studies, 36(3), 527–545. https://doi.org/10.1080/0042098993510
- Lee, G. K., & Chan, E. H. (2008). Factors affecting urban renewal in high-density city: Case study of Hong Kong. Journal of Urban Planning and Development, 134(3), 140–148.
- Liang, C., Lee, C., & Yong, L. (2019). Impacts of urban renewal on neighborhood housing prices: Predicting response to psychological effects. Journal of Housing and the Built Environment, 35(1), 191–213. https://doi.org/10.1007/s10901-019-09673-z
- Maginn, P. J. (2007). Towards more effective community participation in urban regeneration: The potential of collaborative planning and applied ethnography. Qualitative Research, 7(1), 25–43. https://doi.org/10.1177/1468794106068020
- Mayor of London. (2011a). The London plan. The Greater London: Mayor of London.
- Mayor of London. (2011b). [Online] what are Opportunity areas? Retrieved July 31, 2022, from <https://www.london.gov.uk/what-we-do/planning/implementing-london-plan/opportunity-areas/what-are-opportunity-areas?fbclid=IwAR18nI1ekkIWoF_y78ATx04ev0JrpuI8D-Lm_-eM9FAtS22tUdhSIXueOpU>.
- McDowall, W., Geng, Y., Huang, B., Barteková, E., Bleischwitz, R., Türkeli, S., Kemp, R., & Doménech, T. (2017). Circular economy policies in China and Europe: Circular economy policies in China and Europe. Journal of Industrial Ecology, 21(3), 651–661. CrossRef. https://doi.org/10.1111/jiec.12597
- Montgomery, J. (2003). Cultural quarters as mechanisms for urban regeneration. Part 1: Conceptualising cultural quarters. Planning Practice and Research, 18(4), 293–306. https://doi.org/10.1080/1561426042000215614
- Ness, J. (2001). Urban regeneration in Brighton: The new deal for communities. University of Calgary.
- Oléron-Evans, T., & Salhab, M. (2021). Optimal land use allocation for the Heathrow opportunity area using multi-objective linear programming. Land Use Policy, 105, 105353. https://doi.org/10.1016/j.landusepol.2021.105353
- Parkinson, M. (1988). The cities under mrs Thatcher: The centralisation and privatisation of power. Working Paper, Centre for Urban Studies, University of Liverpool.
- Powerday. (2021). Recycling & transfer station in London | Powerday. [Online]. Retrieved July 31, 2022, from <https://powerday.co.uk/waste-management/recycling-transfer-station/>.
- Property Investor Today. (2019). The regeneration effect – new research suggests it results in strong price growth. Retrieved July 18, 2022, from <https://www.propertyinvestortoday.co.uk/breaking-news/2019/11/the-regeneration-effect–new-research-suggests-regeneration-results-in-strong-price-growth1>.
- Rateinflation.com. (2022). UK historical consumer price index (CPI) - 1988 to 2022. Retrieved July 31, 2022, from <https://www.rateinflation.com/consumer-price-index/uk-historical-cpi/>.
- Rius-Ulldemolins, J. (2014). Culture and authenticity in urban regeneration processes: Place branding in central Barcelona. Urban Studies, 51, 3026–3045. https://doi.org/10.1177/0042098013515762
- Wainwright, O., (2021). Penthouses and poor doors: How Europe’s ‘biggest regeneration project’ fell flat. Guardian. Retrieved July 18, 2022, from <https://www.theguardian.com/artanddesign/2021/feb/02/penthouses-poor-doors-nine-elms-battersea-london-luxury-housing-development>.
- Wang, S., Esther, H. K. Y., Yu, Y., & Tsou, J. Y. (2022). Right to the city and community facility planning for elderly: The case of urban renewal district in Hong Kong. Land Use Policy, 114, 105978. https://doi.org/10.1016/j.landusepol.2022.105978
Appendix
To ensure that the GWR analysis is valid, the samples of dependent variables must follow a Gaussian distribution. Above figures show the histograms of the sample distribution of housing price increase and unemployment rate decrease rates. These diagrams illustrate that both dependent variables roughly follow normal distributions, confirming the validity of GWR analysis.