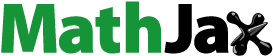
ABSTRACT
The monsoon pattern has shifted across Kerala. This, combined with significant deforestation and hill denuding, has resulted in catastrophic floods and landslides, particularly during the Southwest monsoon. Urgent relief services were to be delivered in a timely and accurate manner in order to sustain the lives of the impacted people. Even though resources were sent to several relief camps, they were either in excess or shortage on multiple instances. The conditions that prevailed during the monsoon time, as well as concerns and challenges during disaster relief efforts, must first be investigated for the effective operation of these supply chain activities. This research aims to develop a disaster logistics hub location selection decision support system, based on the Fuzzy Analytic Hierarchy Process (FAHP) and Best Worst Method (BWM), to meet the needs of disaster victims and rescue teams in the event of flooding, and to implement the proposed systems in Kuttanad, Kerala. Initially, the criteria are determined and structured in the hierarchy, and the weightage for the criteria is done via a questionnaire technique. Expert opinion on nine points scale was gathered from a set of experts working under different levels of positions during disaster management. The weights obtained by the FAHP and BWM methods were statistically analysed and compared for the reliability of the two techniques. The suggested model and application results may throw light on future work, particularly in the realm of disaster logistics management.
1. Introduction
1.1. Background
Floods present a significant global challenge, particularly affecting urban populations, especially in developing nations Kundzewicz et al. (Citation2014). Rapid urbanisation and population growth have exposed several immigrants to flood-prone regions, placing them at danger. The severity and frequency of urban floods are influenced by topographical and socioeconomic parameters associated to flood ‘exposure, ‘sensitivity’, and ‘adaptive capacity’. Human actions and the consequences of climate change aggravate this susceptibility Bigi et al. (Citation2021). China, India, the United States, and Indonesia stand out as some of the nation’s most susceptible to flooding worldwide. Urban development and growth have a considerable impact on the occurrence of floods, with the most severe consequences being substantial financial losses and danger to human life Gao et al. (Citation2022). In India, large cities such as Hyderabad (2000), Kolkata (2007), Delhi (2010), Chennai (2015), Mumbai (2017), and Bangalore (2020) have been devastated by urban flooding Prathipati et al. (Citation2019). Further the list of recently occurred major floods in India are shown in . These floods are triggered mostly by significant precipitation, strong thunderstorms, changes in river flows, and encroachment on floodplains Vazhuthi and Kumar (Citation2020). Numerous flood-related disaster relief initiatives are conducted in order to aid people during these difficult times. However, these operations face numerous obstacles, including logistical difficulties in transporting aid due to infrastructure damage, coordination and communication issues among response entities, resource limitations, difficulties in accessing isolated flood-affected areas, safety concerns for responders, management of displaced populations, and addressing health risks in the aftermath. These obstacles highlight the crucial significance of comprehensive disaster preparedness, effective coordination, and international cooperation to guarantee timely and successful relief efforts in the event of flood-related disasters. The current study selected Alappuzha, Kerala, India, one of the most flood-prone districts in Kerala, as a case study to address operational issues in disaster relief operations through the development of a decision support system that integrates MCDM approaches.
Table 1. List of recent major flood events in India (source: NDMA).
8.7% of the overall geographical area of Kerala, which is blessed with 44 rivers, backwaters, and inland waterways, is deemed flood-prone by the National Disaster Management Authority (NDMA Citation2008). The monsoon pattern in Kerala has been shifting. In 2018, the India Meteorological Department (IMD) issued a warning for extremely heavy rainfall (above 210 mm) in advance of the monsoon season. Whenever a disaster strikes, the response mechanism is initially questioned. Recent research has revealed the growing importance of supply logistics in disaster relief management. To support the lives of the impacted population, prompt and accurate emergency assistance services were required. Despite the fact that the resources were delivered to various relief camps, they were frequently either in excess or deficient. For the proper operation of these supply chain operations, the conditions that prevailed during the monsoon season, as well as the issues and difficulties encountered during disaster relief operations, must be researched beforehand. In emergency situations, the distribution of essentials to those in need, the lack of necessities, the buildup of products, and cost-related obstacles such as high transportation costs, storage costs, maintenance costs, etc. pose significant obstacles. In the aftermath of a flood, the essentials were not distributed appropriately. This requires an efficient supply network. Many locations had a plenty of resources, while others lacked them. Inadequate time management and ineffective transportation channels also contributed to the risk increase.
To effectively address the operational challenges in disaster relief operations, this study aimed to develop a decision support system that tackles the major issues and enables suitable location selection for relief logistics hubs. To achieve this, the study employed multi-criteria decision-making (MCDM) techniques, specifically the Fuzzy Analytic Hierarchy Process (FAHP) and Best Worst Method (BWM). These methods were used to select and rank the necessary criteria for developing a decision support system for disaster logistics hub location selection.
The study’s primary objective was to identify and rank the most significant obstacles encountered during disaster relief activities. These obstacles included commodity-related obstacles, cost-related obstacles, transportation-related obstacles, infrastructure-related obstacles, and geographical obstacles. The study aims to create decision-making aids and disaster distribution models that efficiently manage traffic during disruptive conditions by rating these difficulties according to their importance.
By employing MCDM methodologies and considering a variety of variables, the decision support system developed for this study aims to aid in the selection of locations for relief logistics hubs. This method takes the identified obstacles and their relative significance into consideration, allowing decision-makers to make strategic decisions that optimise the efficiency and efficacy of disaster relief activities.
1.2. Aim and objectives
Aim. This study aims to develop a disaster logistics hub location selection decision support system, based on Fuzzy analytic hierarchy process and best worst methods, to meet the needs of disaster victims and rescue teams after a potential flood, and to implement the proposed systems in Alappuzha district.
Objectives.
To rank and weight the planning and consolidation disaster management strategies from the literature in the city of Alappuzha.
To determine which are the most important challenges suffered during the disaster relief operations.
To examine the similarities of the obtained rankings/weights of two multi-criteria decision-making techniques
To develop decision-making guides and disaster distribution models for managing traffic under disruptive conditions
In summary, this research focused on tackling the operational issues in disaster relief operations by designing a decision support system that combines MCDM methodologies. The method aids in identifying and prioritising obstacles, ultimately leading to the creation of decision-making guidelines and disaster distribution models that improve traffic management during disruptive conditions.
The remainder of this work is structured as follows. The second section provides a comprehensive assessment of the literature relative to the various Multiple Criteria Decision Making (MCDM) methodologies used to choose optimal locations for catastrophe logistics hubs. In Section 3, a succinct description of the case study is presented, emphasising the research’s specific setting and aims. Section 4 describes the study’s methodology, including the stages and techniques used to analyse the data and draw significant conclusions. In Section 5, the data analysis and results are presented, explaining the study’s principal findings and outcomes. Section 6 concludes with a review of the findings, along with concluding remarks, implications, and recommendations for future research to further improve the understanding and efficacy of disaster logistics management.
2. Literature review
The selection of optimal locations for disaster logistics hubs is critical for efficient disaster response and management. Selecting the best location for disaster logistics hubs is a complex process that necessitates a thorough study of various crucial aspects. As Amorim et al. (Citation2019) and Ekmekçioğlu et al., (Citation2021) point out, proximity to disaster-prone areas is critical for prompt action. Furthermore, as proposed by Pagell and Shevchenko (Citation2014) and Noor (Citation2018), accessible and robust transportation infrastructure enables efficient operations during emergencies. Geography, including vulnerability assessments, as stated by Mottahedi et al. (Citation2021), and strong coordination with local authorities and communities, as discussed by Altay and Green (Citation2006), are critical. Consideration of population density and demographics, quantitative risk and impact analyses Lawrence (Citation2006), environmental sustainability, and compliance with political and regulatory requirements Allaoui et al. (Citation2019) are all important components in making well-informed decisions about disaster logistics hub locations. As a result, thorough location selection should consider these factors in order to improve disaster response and recovery efficacy. This literature review aims to provide an in-depth analysis of the existing research on decision support systems (DSS) for disaster logistics hub location selection using multi-criteria decision-making (MCDM) techniques. The review focuses on the methodologies, applications, and contributions of MCDM-based DSS in this context, providing relevant citations and references for further exploration.
The review of existing research reveals that MCDM techniques, such as the Analytic Hierarchy Process (AHP) developed by Saaty (Citation1980), the Technique for Order Preference by Similarity to Ideal Solution (TOPSIS) proposed by Wang and Elhag (Citation2006), and fuzzy set theory, have been widely employed in this context. These techniques enable decision-makers to handle complex decision-making processes by considering multiple criteria and addressing uncertainties.
Several decision support systems (DSS) have been developed to aid in disaster logistics hub location selection. Liu and Chen (Citation2006) introduced a GIS-based DSS that integrated spatial analysis and MCDM techniques to assist decision-makers in identifying optimal hub locations, considering factors such as transportation accessibility, population density, and hazard vulnerability. Another approach proposed by Tzeng et al. (Citation2007) integrated AHP, TOPSIS, and fuzzy set theory in an integrated MCDM-DSS framework, effectively considering diverse criteria and decision-maker preferences. In addition, Hassan Ahmadi et al. (Citation2021) applied GIS, PROMETHEE, MOORA, and other tools to evaluate crisis management locations in Iran, indicating four ideal possibilities in Tehran to demonstrate the efficacy of the strategy. Assessed SeyedAmir et al. (Citation2022) emergency center and vehicle performance during epidemics, using the Delphi method for criteria division, and finds Center A outperforms Center B due to superior prevention indicators and compliant vehicles, particularly Ambulance A-1 and A-2.
In terms of applications and contributions, Zhang and Chen (Citation2011) applied a fuzzy-AHP approach to select locations for regional distribution centers in disaster response scenarios. Their DSS incorporated criteria such as transportation infrastructure, demand coverage, and disaster vulnerability, enabling decision-makers to make informed choices. Additionally, Tang et al. (Citation2017) conducted a case study in China and combined TOPSIS with mathematical programming to optimize emergency logistics distribution. Their research facilitated the optimal allocation of relief resources and the selection of hub locations.
In addition, Manopiniwes et al. (Citation2018) proposed a model for lowering the whole cost of relief operations, which includes warehousing expenses, shipping costs, and holding costs for relief items. The model was created based on an analysis of Thai floods as a case study. Vayvay (Citation2016) conducted research on an AHP-based decision support system for the location selection of disaster centres in Istanbul. The study included two decision support systems, fuzzy and crisp, for selecting emergency logistics centre locations, as well as an Istanbul case study. For flood susceptibility mapping in Cedar Rapids, Iowa, Bui et al. (Citation2016) applied a fuzzy analytical hierarchy process (AHP). The study analysed geophysical-based flood risk, socioeconomic risk, and combined flood risk, indicating high- and very-high-risk flood susceptibility zones concentrated in lower-elevation urban cores. Noor et al. (Citation2018) suggested a model for sustainable supply chain management based on the fuzzy analytic hierarchy process (FAHP) computed with MATLAB. The criteria included cost, quality, delivery time, and supplier location, and both approaches yielded identical, 100 percent accurate, and error-free findings. In addition to MCDM techniques, programming, simulation algorithm techniques, and spatial data analysis with Arc GIS were utilised for sustainable supply chain management, as shown in . These techniques included optimal planning for relief logistics, dynamic relief-demand management in emergency logistics, and maximum covering resource allocation.
Table 2. Literature review on various techniques used for sustainable supply chain management.
Overall, the literature review highlights the significance of MCDM techniques and DSS in the decision-making process for disaster logistics hub location selection. The use of methodologies such as AHP, TOPSIS, and fuzzy set theory, along with the integration of spatial analysis and mathematical programming, has contributed to more effective decision support in this domain. Further research can focus on refining these techniques and developing comprehensive and integrated DSS to address evolving challenges in disaster logistics management.
3. Case study profile – Alappuzha, Kerala
Kerala is geographically bordered by the Arabian Sea to the west and the Western Ghats to the east. Kerala is prone to multiple hazards. The higher the Human Development Index (HDI), the larger the capacity for coping, but also the cumulative loss potential and hence the degree of risk. Thus, Kerala has a greater risk of natural disasters than the rest of the country. Nearly 14.5% of the state’s land area is susceptible to flooding, and the proportion can reach 50% in certain places. Alappuzha is the most flood-prone district in Kerala, with a flood-prone area of 763 square kilometres as shown . The district’s low-lying regions have been inundated. Kuttanad, a submerged region spanning the districts of Alappuzha and Kottayam, is practically engulfed by rains. 55% of the total land is vulnerable to flood. 70% of the area is highly vulnerable to flooding (>100000 population), remaining under moderately vulnerable (10000–100000) Flooding is a perennial problem plaguing the Kuttanad region and numerous measures have been implemented over the years to solve it. The total area of Kuttanad tehsil is 289.39 square kilometres with population density of 667 per sq.km, population of 193,007 (2011 census). The land use distribution is shown in .
A dedicated warehouse is functioning at Cargo Terminal near Punnamada Finishing point, opened during the July 2018 floods that hit the Kuttanad area Chengannur (29%) and Kuttanad (27%) are the tehsils which having high density of camps were situated. 318 relief camps were opened in the district during the mega floods which accommodated 90,589 people (Alappuzha flood report, Citation2018). Further, shows the road network and relief camps density maps of Alappuzha district. The total percentage for transportation is 5%, while the criteria for Urban and Regional Development Plans Formulation and Implementation (URDPFI) are 15%. The AC Route, a 24-kilometer road between Alappuzha and Changanacherry, was planned to facilitate transportation and communication inside Kuttanad.During flooding time this road will disrupted initially, then all other travel depends on water transport only. Movement of relief goods to the waterlogged Kuttanad area through boats.
3.1. Disaster logistic hub location selection
The possible locations for the disaster logistics center determined by the specialists working in Disaster management authority in Kerala were nearer to Kochi port, Alappuzha city (district center) and Chengannur. Proposing logistic center nearer to Alappuzha city can serve 65% of the relief camps (both north and south) part of the district. Comparatively plain terrain and no chances of affecting flooding during the heavy rain condition. 2 bypassing (NH 11 and NH66) roads connect with the district center. All the water transport nodes are starting from district center (SWTD starting jetty).
4. Methodology
A systematic methodology is used to evaluate a decision support system for disaster management employing multi-criteria decision techniques such as Fuzzy Analytic Hierarchy Process (FAHP) and Best Worst Method (BWM), as illustrated in . It begins with the characterization of the problem and the selection of criteria, followed by data collection to gather information and expert opinions. Then, taking uncertainty and imprecision into account, fuzzy AHP is utilised to assess the relative relevance of criteria. BWM analysis compares and scores criteria and sub-criteria to establish their weights. The decision support system is evaluated using the weights that have been calculated, and sensitivity analysis may be performed to examine the robustness of the results. The evaluation findings are analysed, and recommendations for improving the system’s design and functionality are made. By analysing and optimising the system’s performance and applicability, this methodology allows effective decision-making in crisis management scenarios.
The study has been developed with the aim of developing a decision support system model. As a decision support tool, fuzzy AHP and BWM methods are used individually. In this section the fuzzy set theory (background of fuzzy AHP) and BWM methods are discussed in detail.
4.1. Fuzzy set theory
In contrast to the vast majority of cases involving human judgement, crisp sets partition the given universe of discourse into two fundamental categories.
Individuals who unquestionably belong to the set.
Non-members, who most assuredly do not.
This delimitation, deriving from their mutually exclusive structure, necessitates that the decision maker draw a clear line between the choice variables and alternatives. As human preferences involve a degree of ambiguity, it is incredibly difficult to turn human judgement and reasoning into numerical measuring indications in the real world. Moreover, decision makers may be unwilling or unable to supply important numerical values for comparing judgements.
Fuzzy Analytic Hierarchy Process (FAHP) is a fuzzy logic-based Analytic Hierarchy Process (AHP) approach Tesfamariam et al. (Citation2006). The Fuzzy AHP approach works in the same way as the AHP method. It’s only that the Fuzzy AHP technique translates the (Celik et al., Citation2009; Chang, Citation1996). This approach has a long history, dating back to the 1980s and the seminal work of Van Laarhoven and Pedrycz (Citation1983). Buckley’s (Citation1985) introduction of fuzzy ratios in pair-wise comparisons, as well as Mikhailov’s (Citation2003) fuzzy preference programming technique, were both significant contributions to the field. Shin et al. (Citation2007) defined Chang’s extent analysis as a notable current addition. This method is chosen by Tabari et al. (Citation2008) because to its computational simplicity, ease of comprehension, and ability to handle both qualitative and quantitative data. As demonstrated by Aguila Lasserre et al., its practical applications include the prioritisation of customer requirements in quality function deployment, the ranking of human capital evaluation indicators, and the development of ranking systems for semiconductor production (Aguila Lasserre et al., Citation2009).
The first stage is known as weight scaling. This approach is carried out with the help of by doing a comparison in pair by scale a complete number to criteria which is superior and reciprocal judgement for least importance.
Table 3. Scaling of FAHP.
The FAHP is then used in the second phase. The core principle of triangular fuzzy AHP is illustrated here. The triangular FAHP in consists of three variables as shown in EquationEquation (1)(1)
(1) . If the compared alternative is weaker, EquationEquation (2)
(2)
(2) will be utilized.
To meet each objective, an analysis of each object was conducted, followed by a pairwise comparison. Consequently ‘m’ the extent analysis values for each object were discovered as shown in EquationEquation (3)(3)
(3) :
Whereby presented as triangular fuzzy numbers. Chang’s extent analysis is further broken down into four further steps that build on the first. In the third phase, as given in EquationEquation (4)
(4)
(4) , compute the fuzzy synthetic extent value with regard to each object.
To calculate , fuzzy summation operation value of m extent analysis will be carried out as shown in EquationEquations (5)
(5)
(5) & (Equation6
(6)
(6) ):
The l, m and u are the different criteria’s done the same way for further calculate using EquationEquation (9)(9)
(9)
The fifth step is to ensure that the maximum degree of convex fuzzy is greater than k convex fuzzy. Convex fuzzy must have a larger value than k convex fuzzy, as stated by EquationEquation (10)(10)
(10) &; (11).
For k = (1,2,3 … … … n); k ≠ i Then, weight vector is presented in EquationEquation (12)(12)
(12)
Whereby Ai (i = 1,2,3, … … n) are elements obtained after computation. The final step, sixth step is to make sure summation equal to 1 through adding elements and divide using each object as shown EquationEquation (13)(13)
(13) .
4.2. FAHP modelling in MATLAB programming
Scaling many objective criteria will be used in this experiment. Both criteria are crucial. As a result, the scaling will be done by giving the product cost the most weightage. Two approaches will be used at the same time. The first option is to calculate FAHP manually. The second approach is to develop MATLAB code that reads an excel file. The MATLAB programme reads data from an excel spreadsheet precisely. also depicts the FAHP approach.
4.3. BWM method
The Best-Worst Method (BWM) is a vector-based decision-making method with many criteria Rezaei (Citation2015). BWM also compares alternatives, such as AHP, pairwise. Before the selection maker may establish the best and worst criteria without comparing them, a set of selection criteria must be chosen for comparison, which is how a BWM analysis is accomplished, which is a big difference from the AHP technique. The best criteria are then compared to other criteria, and the worst criteria are compared to other criteria as well. In the last stage, the optimal criterion weights are determined. Alternatives are compared on a scale of 1 to 9, with 1 representing equal choice and 9 denoting that something is nine times more essential as shown . Higher consistency ratio comparisons are less reliable. Because it uses fewer comparison data (alternatives) and more consistent comparisons, BWM gives more accurate conclusions. BWM and other MCDM techniques are interchangeable. BWM can replace more comfortable multicriteria decision making strategies.
Table 4. Scaling of BWM.
5. Data collection
The criteria for this research study were chosen mostly based on the recommendations of the post-disaster need assessment analysis for the Kerala floods and landslides in the World Bank report 2018. The framework for analysing planning and consolidation strategies, as well as their changes among possible locational characteristics, is depicted in . The diagram depicts the framework for analysing planning and consolidation tactics, as well as their variations among possible locational factors.
Challenges are broadly classified into five categories such as commodity, cost, transportation, infrastructure, and geographic features. Again, sub-criteria were identified under these areas. The criteria and sub-criteria for the proposed DSS were determined using a questionnaire filled out by specialists from the Kerala disaster management authority (KDMA), the State water transport department (SWTD), extensive discussions with experts from several NGOs, and the use of several studies on disaster and supply chain management in Alappuzha (eg. IITB flood study report of Kuttanadu 2019) as shown in . The city’s placement possibilities are established based on ideas from KDMA specialists. After determining the criteria and placement possibilities, KDMA professionals were asked to state the relevance of each criterion, which was then included in the suggested model. depicts the suggested hierarchical model’s skeleton, which includes criteria, sub-criteria, and alternatives.
Table 5. Sampling.
6. Data analysis and results
This study used two separate approaches to construct a decision support system specialized
for disaster management: the Fuzzy Analytic Hierarchy Process (FAHP) and the Best Worst Method (BWM). The study’s methodology section precisely detailed the systematic, step-by-step procedures for both strategies. Following that, the findings resulting from the use of both approaches have been extensively described in this section, allowing for a clear grasp of the analytical outcomes.
6.1. FAHP results
In the following tables , , , , and , the sub-attributes and alternatives are compared with each other, and all weight vectors gathered from these matrices are used to select the best option with respect to the main goal.
Table 6. Pairwise comparison matrix of sub criteria with respect to commodity.
Table 7. Pairwise comparison matrix of sub criteria with respect to cost.
Table 8. Pairwise comparison matrix of sub criteria with respect to cost.
Table 9. Pairwise comparison matrix of sub criteria with respect to Infrastructure.
Table 10. Pairwise comparison matrix of sub criteria with respect to location.
Similarly, each sub criteria comparison matrices to be generated. A total of 22 matrices are generated and they are manually inserted into the MATLAB programming using an Excel call function.
6.2. BWM results
In the following tables , , , and , weightages of each criterion from Best worst method are shown.
Table 11. Pairwise comparison matrix of Commodity.
Table 12. Pairwise comparison matrix of Cost.
Table 13. Pairwise comparison matrix of transportation.
Table 14. Pairwise comparison matrix of Infrastructure.
Table 15. Pairwise comparison matrix of location.
According to the survey conducted among experts, it was found that 45% of them identified the quality of the commodity as the major problem faced during disaster relief operations. Additionally, 53% of the experts stated that network resilience was the primary transportation-related issue encountered in such operations. Furthermore, 58% of the experts highlighted transportation cost as the major cost-related problem during disaster relief efforts. Lastly, 55% of the experts rated gas and water supply as the major infrastructure-related issue faced in disaster relief operations. These findings indicate the significant challenges that need to be addressed to improve the effectiveness of disaster relief efforts.
The results obtained from the Analytic Hierarchy Process (AHP) and Best Worst Method (BWM) techniques provided weightings for each supply chain management challenge. The outcomes of both techniques revealed variations in the weightages assigned to the challenges as shown in . To assess the similarity of the rankings or weightings obtained, Kendall’s tau-b and Spearman’s rho statistical tests were employed as shown in . These tests analyzed the ranks generated by the AHP and BWM techniques, providing insights into the degree of agreement or correlation between the two methods.
The p-values for both Kendall’s tau-b and Spearman’s rho statistical tests were found to be less than 0.05, indicating a statistically significant correlation between the obtained ranks of the AHP and BWM techniques. This suggests that the rankings produced by both methods are similar and exhibit a strong association. Thus, the AHP and BWM techniques provide consistent predictions and offer comparable results for evaluating the supply chain management challenges.
7. Discussions and conclusion
The findings of the study demonstrate that the weights assigned to supply chain management challenges during disaster situations are consistent when using both the Fuzzy Analytic Hierarchy Process (FAHP) and Best Worst Method (BWM) techniques. However, the FAHP method is specifically suitable for predicting the location of disaster logistic centers. On the other hand, the BWM technique offers the advantage of presenting weighing scales in a more easily understandable manner for stakeholders. It is worth noting that the consistency index of the FAHP method is closer to 1 compared to the BWM method, indicating a higher level of consistency in the FAHP results. Although the FAHP method may be time-consuming, utilizing MATLAB software can significantly reduce the time required for calculations. Decision-makers can utilize prebuilt code that can be updated annually to account for changes in the weightage of disaster challenges. The implementation of such decision support system models is particularly beneficial in regions that are naturally prone to disasters like flooding, earthquakes, and drought. States such as Assam, Uttarakhand, and Rajasthan, which face geographical vulnerabilities, can leverage DSS models to mitigate the severity of the effects caused by disasters.
The Best Worst Method (BWM) results have thrown light on crucial discoveries on the obstacles and issues that must be addressed to improve the success of disaster relief operations across multiple categories. Notably, a significant weightage (45 percent) was allocated to the quality of commodities distributed during disaster relief activities within commodity-related criteria. Similarly, experts underlined that managing transportation expenses (58%) is a key concern in the context of cost-related difficulties. When it comes to transportation issues, network resilience (53%) was seen as a major worry when contrasted to criteria such as mode of transportation, delivery times, and frequency. Similarly, when it comes to infrastructure factors, the majority of experts (55%) listed gas and water supply as a big concern. Furthermore, when it came to the geographical location of emergency logistics centres, experts (65%) agreed that they should be at district centres rather than at key supply or demand points. The Fuzzy Analytic Hierarchy Process (FAHP), on the other hand, provided more specific insights by offering a complete analysis of disaster logistics centre locations based on criterion and sub-criteria weightages. Furthermore, FAHP’s distinct advantages over BWM, such as its ability to handle uncertainty, accommodate complex decision models, incorporate subjective opinions, provide ranked alternatives, enable sensitivity analysis, and perform consistency checks, establish it as a robust and adaptable decision-making tool in the volatile domain of disaster management.
Based on the results of the survey among experts, several strategies can be proposed to address the identified challenges in disaster relief operations.
Enhancing Commodity Quality: Given that 45% of the experts identified the quality of the commodity as a major problem, efforts should be made to improve the quality control and monitoring processes throughout the supply chain. Implementing rigorous inspection procedures, ensuring proper storage and handling of relief items, and establishing partnerships with reliable suppliers can help ensure the delivery of high-quality commodities during disaster relief operations.
Strengthening Network Resilience: Since 53% of the experts highlighted network resilience as a significant transportation-related issue, measures should be taken to improve the resilience and robustness of transportation networks. This can include investing in infrastructure improvements, diversifying transportation routes, establishing alternative modes of transportation, and developing contingency plans to mitigate disruptions and ensure timely and efficient delivery of relief supplies.
Managing Transportation Costs: Considering that 58% of the experts identified transportation cost as a major cost-related problem, strategies should be implemented to optimize transportation expenses without compromising the effectiveness of relief operations. This can involve exploring cost-saving measures such as route optimization, consolidation of shipments, utilization of shared transportation resources, and negotiating favorable contracts with transportation service providers.
Ensuring Reliable Gas and Water Supply: With 55% of the experts rating gas and water supply as a significant infrastructure-related issue, it is crucial to establish robust systems for the provision of these essential resources during disaster relief operations. This can be achieved through effective coordination with utility companies, establishing backup power and water supply sources, implementing contingency plans for emergency situations, and conducting regular maintenance and inspections of infrastructure facilities.
These techniques are intended to solve the stated obstacles and enhance the efficacy and efficiency of disaster relief operations. By emphasising commodity quality, network resilience, transportation cost control, and dependable infrastructure, organisations and stakeholders participating in disaster response can better serve impacted areas and lessen the effects of disasters.
In addition, the study identifies critical areas of future research that must be conducted to improve disaster management techniques, strengthen decision-making processes, and lessen the effects of catastrophes on vulnerable populations.
First, a thorough evaluation of altering monsoon patterns in Kerala is required to comprehend their impact on flooding and landslides. This research should involve reviewing historical meteorological data, examining climate change trends, and doing hydrological modelling in order to assess the shifting monsoon patterns and their consequences for disaster management. Second, an integrated method to resource allocation for disaster relief should be established to solve the problem of resource surplus or deficiency in relief camps. Future study could concentrate on integrating real-time data, improved forecasting approaches, and optimization models to enable the prompt and accurate delivery of resources based on the actual needs of affected populations. Thirdly, an evaluation of post-flood rescue and rehabilitation operations is essential for determining the efficacy of current tactics and identifying areas for improvement. This research could examine the effects of floods on infrastructure and services, evaluate the reaction time and coordination among various stakeholders, and provide solutions to improve the efficiency and efficacy of post-flood operations.
In addition, a comparative analysis of decision support approaches should be performed to determine their applicability and dependability in the context of catastrophe logistics management. While this study already compares the FAHP and BWM methodologies, future research can investigate additional strategies, such as machine learning algorithms, multi-objective optimization approaches, and other multi-criteria decision-making tools. Finally, it is vital to scale up the proposed decision support system model for catastrophe logistics hub location selection. The model, which is unique to the Kuttanad region of Kerala, should be extended and tested in other places prone to flooding or other sorts of natural disasters. This would need taking into account differences in infrastructure, resources, and local conditions, as well as evaluating the model’s relevance and efficacy in various crisis scenarios.
By addressing these areas of research, useful insights can be gained, leading to the enhancement of disaster management techniques, more informed decision-making processes, and improved catastrophe impact mitigation for vulnerable populations.
Disclosure statement
No potential conflict of interest was reported by the authors.
References
- Aguila Lasserre, A. A., Stainer, R., & Montoya-Torres, J. R. (2009). A decision support system for the optimal planning and control of semiconductor manufacturing. International Journal of Production Research, 47(22), 6321–22. https://doi.org/10.1080/00207540802262135
- Allaoui, H., Guo, Y., & Sarkis, J. (2019). Decision support for collaboration planning in sustainable supply chains. Journal of Cleaner Production 229, 761–774. ISSN 0959-6526. https://doi.org/10.1016/j.jclepro.2019.04.367
- Altay, N., & Green, W. G. (2006). OR/MS research in disaster operations management. European Journal of Operational Research, 175(1), 475–493. https://doi.org/10.1016/j.ejor.2005.05.016
- Amorim, M., Antunes, F., Ferreira, S., & Couto, A. (2019). An integrated approach for strategic and tactical decisions for the emergency medical service: Exploring optimization and metamodel-based simulation for vehicle location. Computers & Industrial Engineering, 137. https://doi.org/10.1016/j.cie.2019.106057
- Bigi, V., Comino, E., Fontana, M., Pezzoli, A., & Rosso, M. (2021). Flood vulnerability analysis in an urban context: A socioeconomic sub-indicators overview. Climate, 9(1), 12. https://doi.org/10.3390/cli9010012
- Buckley, J. J. (1985). Fuzzy hierarchical analysis. Fuzzy Sets and Systems, 17(3), 233–247. https://doi.org/10.1016/0165-0114(85)90090-9
- Bui, D. T. (2016). Flood susceptibility mapping using fuzzy analytical Hierarchy Process for Cedar Rapids, Iowa. Natural Hazards, 82(2), 1377–1396.
- Celik, E., Gumus, A. T., & Celik, N. (2009). Fuzzy AHP approach for supplier selection in a washing machine company. Expert Systems with Applications, 36(8), 11302–11312.
- Chang, D. Y. (1996). Applications of the extent analysis method on fuzzy AHP. European Journal of Operational Research, 95(95), 649–655. https://doi.org/10.1016/0377-2217(95)00300-2
- Ekmekçioğlu, Ö., Koc, K., & Özger, M. (2021). District-based flood risk assessment in Istanbul using fuzzy analytical hierarchy process. Stochastic Environmental Research and Risk Assessment, 35(3), 617–637. https://doi.org/10.1007/s00477-020-01924-8
- Flood report 2018 of Alappuzha. https://reliefweb.int/report/india/study-report-kerala-floods-august-2018
- Gao, M., Wang, Z., & Yang, H. (2022). Review of urban flood resilience: Insights from Scientometric and systematic analysis. International Journal of Environmental Research and Public Health, 19(16), 8837. https://doi.org/10.3390/ijerph19148837
- Hassan Ahmadi, C., Jahangoshai Rezaee, M., Ghasemi, P., & Saberi, M. (2021). Efficient crisis management by selection and analysis of relief centers in disaster integrating GIS and multicriteria decision methods: A case study of Tehran. Mathematical Problems in Engineering 2021, 1–22. Article ID 5944828. https://doi.org/10.1155/2021/5944828
- Kundzewicz, Z. W., Kanae, S., Seneviratne, S. I., Handmer, J., Nicholls, N., Peduzzi, P., Mechler, R., Bouwer, L. M., Arnell, N., Mach, K., Muir-Wood, R., Brakenridge, G. R., Kron, W., Benito, G., Honda, Y., Takahashi, K., & Sherstyukov, B. (2014). Flood risk and climate change: Global and regional perspectives. Hydrological Sciences Journal, 59(1), 1–28. https://doi.org/10.1080/02626667.2013.857411
- Lawrence, V. S. (2006). Facility location under uncertainty: A review. IIE Transactions, 38(7), 547–564. https://doi.org/10.1080/07408170500216480
- Liu, S. T., & Chen, C. W. (2006). A GIS-based decision support system for urban emergency logistics planning. Computers & Operations Research, 33(8), 2294–2313.
- Manopiniwes, W. (2018). A multi-objective Model for relief operations in flood-affected areas: A case study of Thai floods. International Journal of Disaster Resilience in the Built Environment, 9(2), 141–158.
- Mikhailov, L. (2003). Fuzzy analytic hierarchy process: A general view. Fuzzy Sets and Systems, 134(3), 293–297. https://doi.org/10.1016/S0165-0114(02)00383-4
- Mottahedi, A., Sereshki, F., Ataei, M., Nouri Qarahasanlou, A., & Barabadi, A. (2021). The resilience of critical infrastructure Systems: A systematic literature review. Energies, 14(6), 1571. https://doi.org/10.3390/en14061571
- NDMA. (2008). Annual Report 2008 (english) (pp. 1–126). https://www.jru.orthop.gu.se/archive/AnnualReport-2008-eng.pdf%5Cnpapers2://publication/uuid/023A3055-01BC-49D3-A2A5-AD650F0176E8
- Noor, A. Z. M. (2018). Sustainable supply chain Model for material flow cost accounting: A case study in Malaysia. Journal of Cleaner Production, 172, 3322–3338.
- Pagell, M., & Shevchenko, A. (2014). Why research in sustainable supply chain management should have no future. The Journal of Supply Chain Management, 50(1), 44–55. https://doi.org/10.1111/jscm.12037
- Prathipati, V. K., CV, N., & Konatham, P. (2019). Inconsistency in the frequency of rainfall events in the Indian summer monsoon season. International Journal of Climatology, 39(13), 4907–4923. https://doi.org/10.1002/joc.6113
- Rezaei, J. (2015). Best-Worst multi-criteria decision-making method. Omega, 53, 49–57 Zhu, K J, Jing, Y, and Chang, D Y (1999), “A discussion on extent analysis method and applications of fuzzy AHP”, European Journal of Operational Research, Vol. 116, pp. 450. https://doi.org/10.1016/S0377-2217(98)00331-2
- Saaty, T. L. (1980). The Analytic Hierarchy Process. McGraw-Hill.
- SeyedAmir, H., Ahmadi Choukolaei, H., Ghasemi, P., Dardaei-Beiragh, H., Sherafatianfini, S., & Pourghader Chobar, A. (2022). Evaluating the performance of emergency centers during coronavirus epidemic using multi-criteria decision-making methods (case study: Sari city). Discrete Dynamics in Nature & Society, 2022, 13. Article ID 6074579. https://doi.org/10.1155/2022/6074579
- Shin, H., Lee, K., & Park, Y. (2007). An integrated approach for the selection of a reverse logistics provider. Supply Chain Management: An International Journal, 12(5), 397–405.
- Tabari, M., Haghani, A., & Danesh, A. (2008). Application of fuzzy AHP to select the best pavement maintenance technique. Expert Systems with Applications, 34(1), 518–527.
- Tang, L., Zhou, X., & Zhang, Q. (2017). Optimization of emergency logistics distribution for a sudden disaster: A case study in China. Transportation Research Part E Logistics & Transportation Review, 101, 15–31.
- Tesfamariam, S., & Sadiq, R. (2006). Risk-based environmental decision-making using fuzzy analytic hierarchy process (F-AHP). Stochastic Environmental Research and Risk Assessment, 21(1), 35–50. https://doi.org/10.1007/s00477-006-0042-9
- Tzeng, G. H., Huang, J. J., & Ong, C. S. (2007). Multiple attribute decision making: Methods and applications. CRC Press.
- Van Laarhoven, P. J. M., & Pedrycz, W. (1983). A fuzzy extension of Saaty’s priority theory. Fuzzy Sets and Systems, 11(1–3), 229–241. https://doi.org/10.1016/S0165-0114(83)80082-7
- Vayvay, Ö. (2016). AHP-Based decision support system for disaster centre location selection: A case study for Istanbul. Natural Hazards and Earth System Sciences, 16(2), 419–431.
- Vazhuthi, H. I., & Kumar, A. (2020). Causes and impacts of urban floods in Indian cities: A review. International Journal of Emerging Technologies, 11, 140–147.
- Wang, Y. M., & Elhag, T. M. S. (2006). Fuzzy TOPSIS method based on alpha level sets with an application to bridge risk assessment. Expert Systems with Applications, 31(2), 309–319.
- Zhang, H., & Chen, S. (2011). Location selection for regional distribution centers: A fuzzy-AHP approach. Expert Systems with Applications, 38(3), 1378–1388.