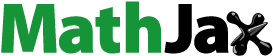
ABSTRACT
This research investigates the influence of socio-demographic attributes on students’ trip generation on the campus of Kwame Nkrumah University of Science and Technology (KNUST). A cross-sectional and mixed-method approach were employed, collecting primary data from 113 students through an online questionnaire. Multiple linear regression analysis and ordinal logistic regression explored the relationships between generated trips and socio-demographic attributes, including age, household size, vehicle ownership, educational level, income, and trip purpose. Age and household size were significant influencers of trip generation in the multiple linear regression. The ordinal logistic regression showed that, males are 2.40 times more likely to generate higher trip counts than females. Individuals aged 16–21 are 0.11 times less likely to produce more trips compared to older age groups. Single-occupant households have a 0.09 times lower likelihood of generating more trips than households with two or more occupants. However, individuals attending morning and afternoon lectures, only morning lectures, and morning and evening lectures among other activities have substantially higher odds of producing more trips, with odds ratios of 22.14, 15, and 567, respectively, compared to those exclusively attending evening lectures. Recommendations include improving shuttle services, pedestrian infrastructure, and promoting sustainable mobility solutions tailored to student demographics.
Background
Transportation prediction is a field that addresses issues arising in urban, regional, or national transportation planning, providing a systematic framework for decision-making, problem-solving, target setting, and goal development (Hedau & Sanghai, Citation2014). The process commences by determining the need for planning, defining problems, setting objectives, and formulating goals. Transportation prediction involves generating and analyzing solutions, evaluating alternative options, and subsequently implementing and executing them (Haial et al., Citation2021). It is vital to recognize that transportation planning is an integral element of overall urban and rural development (Baksh, Citation2020; Poku-Boansi & Cobbinah, Citation2018). Modifications to transportation systems yield diverse consequences. Therefore, transportation planning aims to comprehend these intricacies and propose strategies for secure and efficient movement of people and goods from one point to another (Manuka, Citation2022). This multifaceted approach encompasses activities ranging from scrutinizing existing transportation systems and designing new ones to estimating future travel demand, projecting travel patterns, and assessing environmental and societal impacts of transportation systems (Bibri et al., Citation2020). Effective transportation planning necessitates an extensive grasp of the interplay between transportation and land use, travel behavior, demographics, and economic conditions (Bibri et al., Citation2020; Poku-Boansi & Cobbinah, Citation2018; Hosseinzadeh & Baghbani, Citation2020). This underscores the importance of transportation prediction in transportation planning, which encompasses forecasting future travel demand and identifying requisite transportation infrastructure (Hedau & Sanghai, Citation2014). Key techniques in transportation prediction encompass trip generation, trip distribution, mode selection, and route allocation (Ahmed, Citation2012; Sillaparcharn, Citation2007).
A trip signifies a unidirectional movement of individuals employing a mode of transportation, featuring two endpoints – a point of origin and a destination (Hedau & Sanghai, Citation2014; Manuka, Citation2022). The origin denotes the initiation of the journey or trip generation, while the destination refers to its termination or attraction (Hedau & Sanghai, Citation2014). Trips are typically categorized as home-based or non-home-based (Hedau & Sanghai, Citation2014; Manuka, Citation2022; Sharpe et al., Citation1958). Home-based trips can be further classified into three subtypes: home-based work trips, home-based non-work trips, and home-based maintenance trips (Stiles & Smart, Citation2021). The former involves journeys for work-related purposes such as commuting or business trips, whiles the latter includes non-work journeys like shopping, visiting friends, or leisure activities (Hedau & Sanghai, Citation2014; Manuka, Citation2022; Stiles & Smart, Citation2021). The last category pertains to trips for household-related activities such as grocery shopping or errands. Non-home-based trips can also be subdivided based on their intent. Common non-home-based trip types encompass work-related trips, recreational trips, educational trips, and social trips (Stiles & Smart, Citation2021). Work trips relate to travel for business or commuting, recreational trips encompass leisure pursuits, educational trips serve learning objectives, and social trips involve interactions with friends, family, or social events (Hedau & Sanghai, Citation2014; Stiles & Smart, Citation2021).
Comprehending trip generation is pivotal for effective mobility of people and goods (Hedau & Sanghai, Citation2014). It forms a foundational step in transportation planning and prediction, encompassing the estimation of the number of trips individuals or households will undertake between specific origins and destinations (Ahmed, Citation2012; Calvo et al., Citation2019; Hedau & Sanghai, Citation2014; Manuka, Citation2022). The representation of trip generation typically adopts units of time, such as daily or hourly trips (Cheng et al., Citation2019). The initial phase entails surveys, data collection, and inventories regarding factors influencing travel behavior – like population characteristics, land use patterns, socioeconomic conditions, and transportation infrastructure (Ahmed, Citation2012; Cheng et al., Citation2019; Hedau & Sanghai, Citation2014). Subsequently, acquired data is evaluated to formulate models explaining individual travel behavior. These models commonly employ statistical techniques, such as regression analysis or logit models, to identify salient factors influencing travel patterns (Baksh, Citation2020; Calvo et al., Citation2019; Cheng et al., Citation2019; Hedau & Sanghai, Citation2014; Sharpe et al., Citation1958). They facilitate projecting trip generation under diverse scenarios, including changes in land use or transportation policies. The accuracy of trip generation models significantly impacts transportation planning and prediction, forming the basis for estimating future travel demand and crafting requisite transportation infrastructure (Calvo et al., Citation2019; Fleeze et al., Citation2013; Naess et al., Citation2013; Poku-Boansi & Cobbinah, Citation2018). Nevertheless, these models are subject to limitations, and their accuracy hinges on data quality and assumptions during model construction.
The purpose of a trip is a fundamental concept influencing transportation planning, research, travel behavior, and the development of transportation policies and infrastructure (Hedau & Sanghai, Citation2014). Activity-travel patterns encapsulate 24-hour representations of activity type, distance from home, distance from the last activity, mode of transportation, or other pertinent individual-level factors (Hedau & Sanghai, Citation2014). Recognizing activity-travel patterns is paramount for identifying travel behavior trends and informing transportation policies and infrastructure development that cater to individual and communal needs. One salient component of activity-travel patterns is the purpose of a trip (Hedau & Sanghai, Citation2014). Work-related activities, such as commuting and school trips, significantly drive travel demand (Calvo et al., Citation2019; Cheng et al., Citation2019; Hedau & Sanghai, Citation2014; Manuka, Citation2022; Stiles & Smart, Citation2021). Maintenance tasks, like shopping or dining out, also constitute crucial components. Recreational pursuits, such as visiting friends and attending social events, form significant facets. The purpose of a trip influences travel behavior, including mode selection and frequency. The study aimed to model the socio-demographic attributes influencing trip students’ generation on the campus of Kwame Nkrumah University of Science and Technology (KNUST).
Problem
depicts the flow chart summarizing the methodological steps of the study.
KNUST is located in Kumasi, the capital city of the Ashanti Region of Ghana. The University has a student population of 85,000 (KNUST Notice Board, Citation2022). The University is located on a large campus in the eastern suburbs of Kumasi, adjacent to the Kumasi Institute of Technology and Environment (KITE), and covers an area of approximately 4.8 square kilometers and is surrounded by residential areas and commercial centers (Ghartey & Gyabeng, Citation2017). The campus is relatively large and has several academic, administrative, and residential buildings, including lecture halls, laboratories, dormitories, staff quarters, and sports facilities (Ghartey & Gyabeng, Citation2017). KNUST is easily accessible by road and has several main entrances (Ghartey & Gyabeng, Citation2017). The campus has a road network that connects the different parts of the campus. There are also several bus stops and taxi ranks on the campus to allow students and staff to move around. Additionally, the campus has a shuttle service that transports students and staff between different parts of the campus.
However, there has been an increase in student population and human traffic on most roads, as well as congestion in the student bus services on campus recently. Congestion in the student bus services and the transport networks is due not only to the characteristics of roads, junctions, and route choice but some other factors including socio-demographic characteristics well as the number of trips generated and attracted at the various trip generation and attraction zones. Research have been conducted to forecast the impacts of socio-demographic characteristics and several other factors on trip generation (see e.g. Ahmed, Citation2012; Bwambale et al., Citation2019; Calvo et al., Citation2019; Chang et al., Citation2014; Cheng et al., Citation2019; Hedau & Sanghai, Citation2014; Talpur et al., Citation2023). Worriedly, there exist no empirical studies on socio-demographic attributes affecting students’ trip generation on the KNUST campus and many other Universities in Africa as a whole. This presents an opportunity to examine whether there is an influence of socio-demographic attributes such as gender, age, level of education, monthly income, household size, and vehicle ownership on trips generated on the campus of KNUST by students. Besides, the purpose of trips generated are investigated and the total number of trips quantified. The study provides policy directions for sustainable transportation planning to enhance mobility on KNUST campus. The findings of the study is relevant to be adapted in other university campuses across Africa to address similar transportation challenges.
Methods
depicts the profile of KNUST.
The study employed a cross-sectional design along with a mixed method approach to investigate its research questions. A cross-sectional study is a form of observational research that gathers data from a specific population or sample at a single point in time, aiming to illustrate the prevalence of particular outcomes or attributes within the population and explore potential relationships among variables (Kumar, Citation2011; Cameron, Citation2011). In this study, the cross-sectional design was utilized to examine the connection between the number of generated trips and various socio-demographic attributes. On the other hand, the mixed method approach, which combines quantitative and qualitative methodologies, was adopted to provide a comprehensive and nuanced understanding of the research phenomena (Cameron, Citation2011 Kumar, Citation2011), thus enabling a deeper exploration of the research objectives.
Both primary and secondary data were collected, involving field surveys and articles respectively.
In order to acquire a sample size that accurately represents the respondents, we employed the sample size determination formula established by Fisher et al. (Citation1998). The formula is expressed as:
Where:
The desired sample size (assuming a population exceeding 10,000).
The standard normal deviation typically set to 1.96 for a 95% confidence level.
The proportion in the target population with a specific characteristic (e.g. similar views on trip generation).
The complement of p (i.e. q = 1-p).
The degree of accuracy set to 0.05 for a 95% confidence level.
Given that the population of KNUST is 85,000 (KNUST Notice Board, Citation2022), z-statistic of 1.96, a degree of accuracy set at 0.05, a proportion (p) representing respondents with similar characteristics regarding their views on trip generation on the KNUST campus set at 92% (equivalent to 0.92), and q at 0.08 (i.e. 1–0.08), the resulting sample size is:
A sample size of 113 was generated from the total population of 85,000. An online questionnaire containing closed-ended questions was randomly distributed to these 113 respondents. Out of the 113 questionnaires distributed, 111 (98.2%) were accurately completed and served as the foundation for this study.
Quantitative and qualitative data were processed and analyzed using SPSS (version 26) and Microsoft Excel. Descriptive statistics such as frequencies and percentages were initially employed for data analysis. Subsequently, inferential statistics, particularly multiple linear regression analysis, was conducted to establish relationships between the dependent variable (number of trips) and independent variables (factors influencing trip generation).
Linear regression analysis is a statistical technique used to construct a model that captures the relationship between a dependent variable (referred to as the response variable) and one or more independent variables (referred to as predictor variables or factors) (Pandis, Citation2016; Seber & Lee, Citation2003; Uyanık & Güler, Citation2013). The aim is to determine the most appropriate linear relationship that accounts for the variability in the dependent variable based on variations in the independent variables (Pandis, Citation2016; Seber & Lee, Citation2003).
In simpler terms, the purpose of linear regression is to identify a straight line (expressed through a linear equation) that best fits the data points on a scatterplot (Pandis, Citation2016; Seber & Lee, Citation2003; Uyanık & Güler, Citation2013). This line is then employed for making predictions and understanding the interactions between the variables. The primary objective is to minimize the discrepancy between the actual observed values and the values predicted by the linear equation.
Mathematically, a basic linear regression model can be depicted as:
Where:
Dependent variable (response variable).
Intercept or constant, representing the y-value when
is zero.
Coefficient of the independent variable.
Independent variable (predictor variable).
In scenarios involving multiple linear regression, where there are more than one independent variables, the equation takes the form:
Where:
Dependent variable.
Intercept or constant.
Are the coefficients linked to each independent variable
Taking into account the relationship between the number of generated trips and various socio-demographic attributes (including gender, age, level of education, monthly income, household size, trip purposes, and car ownership), where the former is the dependent variable and the latter are the independent variables, the model development is guided by a confidence level of 95% (α 0.05). The hypothesis underlying the model construction is as follows:
H0:
Socio-demographic attributes influence trip generation on KNUST campus.
H1:
At least, one socio-demographic feature influences trip generation on KNUST campus.
The results of the study are presented in tables and graphs.
Alternatively, a probabilistic model, the Ordinal Logistic Regression was also employed to better understand the influence of the various categories of the independent variables on trip generation. It was also to compare and contrast the results of this model to that of the multiple linear regression. Ordinal logistic regression, as described by Keller et al. (Citation2021), is utilized to predict an ordinal response variable using one or more predictor variables. In a more specific context, this statistical test is used to determine which independent variable(s) exert a significant influence on the dependent variable and to assess the model’s efficacy in forecasting the dependent variable, as discussed by Kleinbaum and Klein (Citation2010). The ordinal logistic regression model can be represented as follows:
P (Y ≤ j) is the cumulative probability of the dependent variable Y being in category j or below.
P(Y>j) is the cumulative probability of the dependent variable Y being in a category above j.
αj is the intercept parameter for category j.
… ,
are coefficient parameters associated with the independent variables
The results of the logistic regression analyses are elucidated through odds ratio estimates derived from the models. When the odds ratio exceeds 1, it suggests that as the independent variable increases, the probability of the desired outcome also increases. Conversely, an odds ratio below 1 indicates that as the predictor variable rises, the probability of achieving the desired outcome decreases, as outlined by Field (Citation2013).
Results and discussion
Socio-demographic attributes
The study identified the socio-demographic characteristics of respondents that influence trip generation, as depicted in below.
Table 1. Socio-demographic features.
From the collected survey data, it was observed that 72 individuals (64.9%) identified as males, while 39 individuals (35.1%) identified as females. A significant portion of the respondents (50.5%) fell within the age range of 22 to 24 years. Furthermore, the study revealed that the majority of participants (84.7%) were pursuing undergraduate studies, whereas a smaller proportion (15.3%) were postgraduate students. In terms of accommodation, the study identified varying household sizes, with the highest being four occupants per room (40.5%), and the lowest being a single occupant per room (11.7%). The findings also indicated that the respondents exhibited a generally modest monthly income, with approximately 41.4% earning below the 200GHȻ income bracket. This can be attributed to the fact that the participants are primarily dependent on financial support from their parents and guardians for their subsistence. Intriguingly, an overwhelming majority of the respondents (94.6%) reported not owning a personal vehicle.
Purpose of trips
The study investigated the purpose for which trips are generated on KNUST campus, as shown in .
The findings indicated that trips on the KNUST campus were motivated by various factors, encompassing lecture-based trips for educational purposes, trips to markets or stores for shopping, personal outings, recreational excursions, and work-related travel, as indicated in below. When examining the breakdown of trip purposes, lecture-based trips emerged as the most frequent, totaling 246 trips. Following closely were personal trips, accounting for 97 instances. In contrast, work-related trips were the least common, amounting to 19 trips. Overall, the data revealed a cumulative count of 463 trips. It is worth highlighting that the respondents primarily consisted of students, underscoring that a substantial portion of their trips were centered around attending lectures.
Modelling socio-demographic attributes’ influence on trip generation
Multiple linear regression model
The research employed a multiple linear regression approach to analyze how socio-demographic attributes (including gender, age, level of education, monthly income, household size, trip purposes, and car ownership) collectively influenced the number of trips generated by students. This modeling was carried out with a confidence level of 95%. In this framework, the number of trips represented the dependent variable, while the socio-demographic features were the independent variables. Mathematically, the multiple linear regression is expressed in terms of EquationEquation (3)(3)
(3) (see methods in section 2 above).
Where:
Dependent variable (representing the number of trips).
Intercept or constant.
Are the coefficients linked to each independent variable
(socio-demographic factors).
A model fitness test was conducted using ANOVA to determine whether the multiple regression model is fits for the data, as shown in .
Table 2. Test for model fitness.
The ANOVA table provided insights into the regression component, revealing that the sum of squares for regression accounted for 16.573, with 6 degrees of freedom (df). The mean square (MS) for regression stood at 2.762. The F-statistic, designed to gauge the collective significance of the regression, yielded a value of 2.633. Notably, the corresponding p-value was calculated as 0.020. This p-value signifies that the multiple regression model significantly explains the variability observed in the dependent variable (number of trips).
Furthermore, furnishes the coefficients inherent to the multiple regression model.
Table 3. Coefficients of the regression model.
Based on the coefficients of the regression output, the model takes the form:
Number of trips 0.348
0.369 age
0.015 monthly income
0.236 household size
0.082 vehicle ownership
0.071 trip purposes
0.535 level of education
Starting with the model, the constant (Intercept) (B = 0.348) represents the fundamental number of trips when all other predictors hold values of zero. In practical terms, it signifies the number of trips for a student with zero age, income, household size, no vehicle ownership, no specific trip purposes, and zero level of education. Nonetheless, given the lack of practical relevance in these values, the intercept serves as a reference point for comparison rather than a directly meaningful interpretation.
The coefficient for the age category (B = 0.369, p = 0.040) indicates that, with each incremental unit in the age category variable, the number of trips generated increases by 0.369 units. The p-value of 0.040 suggests that the age category significantly influences the number of trips, as it falls below the conventional significance threshold of 0.05. Similarly, the coefficient for household size (B = 0.236, p = 0.032) illustrates that for each additional individual in the household, the number of trips increases by 0.236 units. The p-value of 0.032, below 0.05, suggests a statistically significant impact of household size on trip generation.
Conversely, the coefficients for monthly income (B = 0.015, p = 0.829) and level of education (B = 0.535, p = 0.117) indicate an increase of 0.015 and 0.117, respectively, for each unit increment in monthly income and education level. However, the relatively high p-values of 0.829 and 0.117 for monthly income and education level suggest that these variables lack statistical significance in influencing trip generation on the KNUST campus.
In contrast, the coefficients for vehicle ownership (B = −0.082, p = 0.857) and trip purposes (B = −0.071, p = 0.157) are negative, implying a reduction of 0.082 and 0.071 trips when students own a vehicle or undertake a trip for a particular purpose, respectively. Nevertheless, their higher p-values of 0.857 and 0.157 underscore their negligible influence on trip generation within the model.
Ordinal logistic regression analysis
The study sought to use the influence of socio-demographic characteristics on trip generation using ordinal logistic regression. A model-fitting test was performed to test the viability of the model as indicated in below.
Table 4. Omnibus test.
The ordinal logistic regression results indicate a statistically significant model fit with ().
The parameter estimates for the logistic regression is depicted below in .
Table 5. Parameter estimates.
From below, it is evident that vehicle ownership, level of education, and monthly income have no significant impact on the number of trips. In contrast, gender, age, household size, and trip purpose exhibit a significant influence on trip frequency. Specifically, males are 2.40 times more likely to have a higher number of trips compared to females. Furthermore, individuals aged 16–21 years are 0.11 times less likely to have more trips compared to those in different age categories. Regarding household size, individuals in single-person households (one in a room) are 0.09 times less likely to have more trips than those in households with multiple occupants (e.g. two in a room, three in a room, etc.). This implies that the number of people sharing a room or accommodation affects trip generation, with students in larger households making more trips. Moreover, individuals who attend both morning and afternoon lectures while engaging in other activities are 22.14 times more likely to have an increased number of trips compared to those with other trip purposes. Similarly, individuals who attend morning lectures and participate in additional activities are nearly 15 times more likely to have more trips than those with alternative trip purposes. Finally, those who attend both morning and evening lectures are a striking 567 times more likely to have more trips compared to those with different trip purposes. These findings suggest that the purpose of the trip, particularly academic and extracurricular activities, strongly influences trip generation.
Discussion
Numerous socio-demographic factors wield influence over the generation of trips, encompassing income, education level, trip purposes, monthly income, vehicle ownership, and household size, as indicated by prior research (Baksh, Citation2020; Bwambale et al., Citation2019; Calvo et al., Citation2019; Georggi, Citation1999; Dargay & Clark, Citation2012; Hedau & Sanghai, Citation2014; Sovacool et al., Citation2018). These variables were meticulously examined in this study to ascertain their impact on trip generation using multiple linear and ordinal logistic regression. The multiple regression model unveiled age and household size as the most pivotal factors affecting students’ trip generation on the KNUST campus as indicated in . This aligns with established findings highlighting the pronounced influence of age and household size on trip generation. Previous works by Bwambale et al. (Citation2019), Calvo et al. (Citation2019), Schuldiner (Citation1962), and Supernak et al. (Citation1983) have emphasized that varying age groups exhibit distinct travel needs and behaviors, ranging from school and leisure trips for the young to work-related and family outings for middle-aged individuals, and medical or leisure trips for seniors. Besides, the relevance of household size in trip generation is underscored, whereby larger households tend to generate more trips due to the diverse schedules and purposes of their members, while smaller households produce fewer trips (Bwambale et al., Citation2019; Calvo et al., Citation2019; Manuka, Citation2022; Supernak et al., Citation1983). The current study’s findings of age and household size as the primary influencers of students’ trip generation resonate with established literature on socio-demographic characteristics’ role in shaping travel behavior (see e.g. Bwambale et al., Citation2019; Calvo et al., Citation2019; Hedau & Sanghai, Citation2014; Schuldiner, Citation1962; Sharpe et al., Citation1958). The fact that all respondents fall within an active age range (see ) contributes to their propensity for a higher number of trips. Furthermore, the diverse household sizes among respondents, ranging from one occupant to four occupants per room, with the majority (40.5%) being four occupants per room (see ), significantly influences trip generation on campus across various households. This corroborates with findings from previous research (see e.g. Bwambale et al., Citation2019; Calvo et al., Citation2019; Manuka, Citation2022; Schuldiner, Citation1962; Supernak et al., Citation1983) highlighting the correlation between larger household sizes and increased trip frequency.
The research further explored the impact of the socio-demographic characteristics on trip generation through the utilization of ordinal logistic regression. The findings illuminated the significant role that sociodemographic factors, including gender, age, household size, and trip purpose, play in influencing the number of trips generated within the KNUST campus (see ). The disparities between the results obtained in the multiple linear regression and the ordinal logistic regression hint at the presence of additional contributing factors for the latter’s outcomes.
When considering age, it became apparent that individuals in the younger age category exhibit a lower likelihood of generating more trips compared to their counterparts in higher age categories. This could be attributed to the diverse academic programs available at KNUST, which cater to different age groups. For instance, younger students may predominantly engage in foundational coursework, while older students may partake in research projects, internships, or advanced courses necessitating additional travel. As noted earlier, this finding is consistent with that of Bwambale et al. (Citation2019), Calvo et al. (Citation2019), Schuldiner (Citation1962), and Supernak et al. (Citation1983) who emphasized that varying age groups exhibit distinct travel needs and behaviors.
Concerning gender, the study revealed that males are more inclined to embark on a greater number of trips than females. This variance may be linked to the array of campus activities, clubs, and organizations offered at KNUST, each with varying degrees of appeal to different genders. For instance, activities like sports, campus delivery services, and certain academic clubs draw more male participants, contributing to their increased trip frequency among males.
Furthermore, the research unveiled that individuals residing in single-occupant households (where only one person occupies a room) are less likely to generate more trips than those residing in households with two or more occupants sharing a room. This finding has been established in previous works (see e.g. Bwambale et al., Citation2019; Calvo et al., Citation2019; Manuka, Citation2022; Supernak et al., Citation1983), where larger households generated more trips while smaller households produced fewer trips. It is relevant to note that KNUST’s housing policies allocate accommodations of varying sizes based on household size. Consequently, students from larger households are placed in living spaces with multiple occupants, which, in turn, affects their travel patterns. To clarify, KNUST offers more accommodations with multiple occupants as opposed to those designed for single occupants, resulting in a reduced likelihood of generating more trips among individuals in single-occupant households.
Conversely, the study illustrated that individuals attending both morning and afternoon lectures while engaging in additional activities are more likely to increase their number of trips in comparison to those solely attending evening lectures. This phenomenon can be attributed to the scheduling of a greater number of lectures and other campus activities in the morning and afternoon compared to the evening. In essence, students with consecutive classes or those opting to attend multiple academic sessions are more prone to making additional trips.
Apparently, vehicle ownership, level of education (undergraduate or postgraduate), and income showed no significant influence on trip generation in both the multinomial linear regression and the ordinal logistic regression (see ). In relation to vehicle ownership, the study’s findings revealed a notable trend, with approximately 95% of students lacking personal vehicles (see ). Consequently, a significant portion of their travel is accomplished through walking or shuttle services, minimizing the influence of vehicle ownership on trip generation within this study. Interestingly, this discovery contradicts previous research that has emphasized the pivotal role of vehicle ownership as a determinant of trip generation (see e.g. (Baksh, Citation2020; Bwambale et al., Citation2019; Dargay & Clark, Citation2012; Sillaparcharn, Citation2007), demonstrating that owning a vehicle contributes positively to trip production. This implies that an increase in vehicle ownership leads to a commensurate rise in trip generation (Sillaparcharn, Citation2007). In other words, Baksh (Citation2020) and Bwambale et al. (Citation2019) argued that as vehicle ownership becomes more widespread, there is a corresponding increase in the frequency of generated trips. Similarly, Gulden et al., (Citation2013) highlighted the significance of automobile presence within a household as a key determinant in measuring the number of journeys undertaken by that particular household.
For level of education, the study unveiled that a substantial majority (84.7%) of respondents were undergraduate students residing on campus (see ), with a significant proportion engaging in similar types of trips. This scenario rendered the levels of education a less influential factor in shaping trip generation patterns within the model. While previous studies (see e.g. Anggraini et al., Citation2017; Cordera et al., Citation2017; Dargay & Clark, Citation2012) have offered valuable insights into the impact of education on trip production, this study demonstrates a divergence between level of education and trip generation outcomes.
Extensive research consistently underscores the substantial influence of income levels on travel behavior and travel patterns (Busari et al., Citation2017; Cheng et al., Citation2019; Talpur et al., Citation2023). As highlighted by Cheng et al. (Citation2019), individuals with higher incomes tend to exhibit distinct travel behaviors when compared to those with lower incomes. This phenomenon can be attributed to a multitude of factors. Firstly, individuals with greater financial resources often have access to a broader range of mobility options, including private vehicles, public transportation, and non-motorized modes of travel (Cheng et al., Citation2019). This expanded spectrum of transportation alternatives contributes to variations in the frequency and distance of trips taken. Additionally, individuals with higher incomes frequently engage in diverse activities that require more frequent travel, such as shopping at upscale establishments or participating in leisure and recreational pursuits that involve mobility (Busari et al., Citation2017; Cheng et al., Citation2019; Talpur et al., Citation2023). Moreover, income can influence housing choices and residential locations, thus impacting daily travel needs (Calvo et al., Citation2019; Cheng et al., Citation2019; Sillaparcharn, Citation2007). Furthermore, income plays a role in the selection of transportation modes for trips. Those with higher incomes are more likely to own and utilize private vehicles, resulting in a higher number of car trips (Cheng et al., Citation2019; Talpur et al., Citation2023). Conversely, individuals with lower incomes tend to rely more on public transit or non-motorized modes, influencing both the frequency and nature of their travel experiences. Interestingly, the findings of this study diverge from these established patterns, as income was not found to have a significant impact on trip generation (see ). The study observed that trips were predominantly localized, and respondents often utilized pedestrian mobility (walking) as the dominant mode of transportation on the KNUST campus. Even when considering vehicle travel preferences, the availability of free shuttles further diminished the influence of income on trip generation prediction. This contrasts with existing literature that emphasizes income’s role in shaping travel behaviors, highlighting the specific context of the KNUST campus and its proximity-based travel patterns.
However, a thorough examination of both the linear regression and ordinal logistic regression analyses uncovered the superior predictive capacity of the ordinal logistic regression in the study. It demonstrated a greater potential for capturing the influence of more socio-demographic attributes, including gender, age, household size, and trip purpose on trip generation in comparison to the linear regression model, which only identified age and household size as significant factors. This suggests that the ordinal logistic regression is a more robust and comprehensive approach for assessing the impact of socio-demographic variables on trip generation in this study.
Conclusion and policy implications
The research sheds light on the complex interplay between socio-demographic attributes and trip generation among students on the Kwame Nkrumah University of Science and Technology (KNUST) campus. The findings underscore the significance of gender, age, household size, and trip purpose as primary factors influencing students’ trip generation patterns. Interestingly, the study reveals that factors like vehicle ownership, level of education, and income do not hold as much sway over trip generation in this context, possibly due to the prevalence of walking and shuttle-based mobility options on campus. These findings challenge conventional assumptions and highlight the importance of considering context-specific factors in understanding travel behavior.
The findings of this research hold significant implications for shaping policies and strategies related to campus planning, transportation management, and fostering sustainable mobility. One of the key takeaways is the pressing need to ensure efficient and well-organized shuttle services on campus. With walking and shuttle-based travel emerging as dominant modes of mobility, creating a seamless and reliable shuttle system becomes paramount to facilitate convenient movement for students.
Moreover, the prevalence of walking as a primary mode of travel underscores the importance of investing in pedestrian infrastructure. Designing safe and accessible sidewalks, crosswalks, and pathways can encourage active commuting and reduce congestion. This shift towards pedestrian-oriented planning could promote a healthier and more sustainable campus environment.
In addition, the low influence of vehicle ownership suggests an opportunity to rethink campus transportation priorities. Instead of emphasizing parking infrastructure, campuses could invest in alternative mobility modes such as bicycle lanes and bike-sharing programs. By focusing on promoting eco-friendly options, institutions can contribute to reduced carbon emissions and a greener campus.
The research’s spotlight on age, gender, household size, and trip purpose as significant factors in trip generation emphasizes the need for tailored interventions. Crafting transportation solutions that cater to the distinct travel patterns of different student groups could lead to more effective mobility strategies. This could involve creating specialized services or providing resources that accommodate the unique needs of undergraduate and postgraduate students. Data-driven decision-making emerges as a critical approach. Campuses can harness real-time data to monitor transportation trends, allowing for swift adjustments and improvements. This proactive stance can lead to more efficient shuttle scheduling, enhanced pedestrian pathways, and better-informed policy decisions.
Limitations and future research
While the study provides valuable insights into the complex relationship between socio-demographic attributes and trip generation among students on the KNUST campus, it is essential to acknowledge certain limitations that may shape the scope and implications of the findings. The study’s context specificity calls for caution when generalizing the results beyond the KNUST campus, as different campuses may possess distinct spatial layouts, mobility options, and student demographics that could influence trip patterns differently. Additionally, the cross-sectional design captures a snapshot of trip generation at a particular point in time, potentially missing out on dynamic trends and seasonal variations that might impact travel behavior.
These limitations pave the way for promising avenues of future research. Longitudinal studies that track travel behavior over extended periods could capture the dynamics of trip generation more comprehensively, considering variations over time and seasons. Comparative studies across different campuses could reveal how contextual factors influence trip patterns and mobility choices. Exploring the behavioral aspects of trip generation through qualitative methods could uncover the underlying motivations and decision-making processes that drive students’ travel choices. Further investigations could delve into the influence of technology, such as ride-sharing services, on campus mobility. In-depth analyses of spatial distribution and GIS mapping could highlight congestion areas and opportunities for infrastructure optimization. Incorporating psychological factors and exploring the effectiveness of policy interventions, particularly those promoting sustainable transportation modes, could enrich our understanding of student travel behavior.
Ethical considerations
The research adhered to the ICMJE guidelines on Protection of Research Participants, the Belmont Report, and the Declaration of Helsinki. Specifically, the study conformed to the principles of informed consent, confidentiality, and anonymity. To ensure accurate understanding, researchers introduced themselves to participants, eliminating potential misconceptions that could hinder data collection. The study’s purpose was explained, enhancing participants’ comprehension. The questionnaire and interview guide structure was shared, aiding participants in providing informed responses. Questionnaires avoided personal identifiers like names and contact details, ensuring anonymity. Participants were assured their data would solely serve the study and would not be disclosed without valid reasons. Privacy rights were upheld, with sensitive questions minimized and participants given the choice to skip personal inquiries.
Contribution of authors
Jerry Julius Edem Dzivona* formulated the topic, worked on the literature review, discuss the results, and prepared the manuscript.
Reginald Nii Akotoa Quaob collected the data and performed the analysis of the results.
Disclosure statement
No potential conflict of interest was reported by the authors.
Data availability statement
The data would be made available upon reasonable request.
Additional information
Funding
References
- Ahmed, B. (2012). The traditional four steps transportation modeling using a simplified transport network: A case study of Dhaka city, Bangladesh. International Journal of Advanced Scientific Engineering and Technological Research, 1(1), 19–19. https://doi.org/10.3390/ijgi1010003
- Anggraini, R., Sugiarto, S., & Pramanda, H. (2017). Factors affecting trip generation of motorcyclist for the purpose of non-mandatory activities. AIP Conference Proceedings, 1903. https://doi.org/10.1063/1.5011565
- Baksh, M. A. (2020, October). Factors Relating to the Trip Generation in a Growth Centre. https://www.researchgate.net/publication/344862580
- Bibri, S. E., Krogstie, J., & Kärrholm, M. (2020). Compact city planning and development: Emerging practices and strategies for achieving the goals of sustainability. Developments in the Built Environment, 4:100021. https://doi.org/10.1016/j.dibe.2020.100021
- Busari, A., Oyedepo, J., Modupe, A., Bamigboye, G., Olowu, O., Adediran, J., & Ibikunle, F. (2017). Trip pattern of low density residential area in semi urban industrial cluster: Predictive modeling. International Journal Human Capital Urban Management, 2(3), 211–218.
- Bwambale, A., Choudhury, C. F., & Sanko, N. (2019). Car trip generation models in the developing world: Data issues and spatial transferability. Transportation in Developing Economies, 5(2), 1–15. https://doi.org/10.1007/s40890-019-0075-7
- Calvo, F., Eboli, L., Forciniti, C., & Mazzulla, G. (2019). Factors influencing trip generation on metro system in Madrid (Spain). Transportation Research Part D: Transport and Environment, 67(December 2018), 156–172. https://doi.org/10.1016/j.trd.2018.11.021
- Cameron, R. (2011). Mixed methods research: The five Ps framework. Electronic journal of business research methods, 9(2), pp96–108 . http://academic-publishing.org/index.php/ejbrm/article/view/1272
- Chang, J. S., Jung, D., Kim, J., & Kang, T. (2014). Comparative analysis of trip generation models: Results using home-based work trips in the Seoul metropolitan area. Transportation Letters, 6(2), 78–88. https://doi.org/10.1179/1942787514Y.0000000011
- Cheng, L., Chen, X., Yang, S., Wu, J., & Yang, M. (2019). Structural equation models to analyze activity participation, trip generation, and mode choice of low-income commuters. Transportation Letters, 11(6), 341–349. https://doi.org/10.1080/19427867.2017.1364460
- Cordera, R., Coppola, P., Dell’olio, L., & Ibeas, Á. (2017). Is accessibility relevant in trip generation? Modelling the interaction between trip generation and accessibility taking into account spatial effects. Transportation, 44(6), 1577–1603. https://doi.org/10.1007/s11116-016-9715-5
- Dargay, J. M., & Clark, S. (2012). Determinants of longdistance travel in Great Britain. Transport Research Part A, 46 (3), 576–587. https://doi.org/10.1016/j.tra.2011.11.016
- Field, A. (2013). Dicovering statistics using IBM SPSS statistics. 4th Edition 1 Oliver’s Yard, 55City Road, London EC1Y 1SP: Sage Publications Limited. 952. https://scholar.google.com/scholar?hl=en&as_sdt=0%2C5&q=Field%2C+A+%282013%29.+Discovering+statistics+using+IBM+SPSS+statistics&btnG=
- Fisher, A. J., Laing, J. S., & Townsend, J. (1998). Handbook for family planning operations research design. The Population Council.
- Fleeze, H., Anin, E., Han Annan, J., Harriet, T., Poku, K., & Kwabena Emmanuel, A. (2013, 3). An assessment of traffic congestion and its effect on productivity in urban Ghana. Related papers evaluating the role of mass transit and it s effect on fuel efficiency in t he Kumasi Metropolis: An assessment of traffic congestion and its effect on produ. International Journal of Business and Social Science, 4. www.ijbssnet.com
- Georggi, N. L. (1999, June). An analysis of Long distance travel behavior of the elderly and the low- income households in Florida. Center for Urban Transportation Research {CUTR), Department of Civil and Environmental Engineering, University of South Florida.
- Ghartey, E. C., & Gyabeng, B. A. (2017). KNUST Map Locator. 1–23. https://www.google.com/search?q=KNUST+MAP+LOCATOR+Ghartey%2C+Gyabeng+pdf&client=firefox-b-d&biw=1025&bih=539&sxsrf=ALiCzsYOI4WnnS4joo7FrvfiCc9j2cFw5Q%3A1659951157058&ei=NdjwYpqOA9bssAeSo7bgCQ&ved=0ahUKEwja_L3497b5AhVWNuwKHZKRDZwQ4dUDCA0&uact=5&oq=KNUST+MA
- Gulden, J., Goates, J. P., & Ewing, R. (2013). Mixed-use development trip generation model. Transportation research record, 2344(1), 98–106. https://doi.org/10.3141/2344-11
- Haial, A., Benabbou, L., & Berrado, A. (2021). Designing a transportation-strategy decision-making process for a supply chain: Case of a pharmaceutical supply chain. International Journal of Environmental Research and Public Health, 18(4), 1–29. https://doi.org/10.3390/ijerph18042096
- Hedau, A. L., & Sanghai, S. S. (2014). Development of trip generation model using activity based approach. International Journal of Civil, Structural, Environmental and Infrastructure Engineering, 4(3), 61–78. http://www.tjprc.org/view-archives.php?year=2014&jtype=2&id=26&details=archives
- Hosseinzadeh, A., & Baghbani, A. (2020). Walking trip generation and built environment: A comparative study on trip purposes. International Journal for Traffic and Transport Engineering, 2(10), pp402–414. https://mpra.ub.uni-muenchen.de/109025
- Keller, J., Mendonca, F. A. C., & Adjekum, D. (2021). Contributory factors of fatigue among collegiate aviation pilots: An ordinal regression analysis. Collegiate Aviation Review International, 39(2), 63–93. https://doi.org/10.22488/okstate.22.100233
- Kleinbaum, D. G., & Klein, M. (2010). Logistic regression. Springer.
- KNUST Notice Board. (2022). Welcome Message from KNUST Vice Chancellor to Students. https://knustnoticeboard.info/welcome-message-from-knust-vice-chancellor-to-students/
- Kumar, R. (2011). Research methodology: A step-by-step guide for beginners. 3rd Edition, 1–528, Sage Publications Limited, Research methodology: A step-by-step guide for beginners, London EC1Y 1SP, ISBN 978-84920-300-5. https://scholar.google.com/scholar?hl=en&q=ranjit+kumar”step+by+step”&oq=ranjit
- Manuka, D. A. (2022). Modeling trip generation pattern by adopting multiple linear regression in at existing traffic condition. Preprint from Research Square. 1–16. https://doi.org/10.21203/rs.3.rs-1959512/v1
- Naess, P., Hansson, L., Richardson, T., & Tennøy, A. (2013). Knowledge-based land use and transport planning? Consistency and gap between “state-of-the-art” knowledge and knowledge claims in planning documents in three Scandinavian city regions. Planning Theory & Practice, 14(4), 470–491. https://doi.org/10.1080/14649357.2013.845682
- Pandis, N. (2016). Multiple linear regression analysis. American Journal of Orthodontics and Dentofacial Orthopedics, 149(4), 581. https://doi.org/10.1016/j.ajodo.2016.01.012
- Poku-Boansi, M., & Cobbinah, P. B. (2018). Land use and urban travel in Kumasi, Ghana. GeoJournal, 83(3), 563–581. https://doi.org/10.1007/s10708-017-9786-7
- Schuldiner P. W. (1962). Trip generation and the home. Report No. 347.Washington DC, United States. Highway Research Board. http://onlinepubs.trb.org/onlinepubs/hrbulletin/347/347-005.pdf
- Seber, G. A., & Lee, A. J. (2003). Linear regression analysis (Vol. 330). John Wiley & Sons.
- Sharpe, G. B., Hansen, W. G., & Hamner, L. B. (1958). Factors affecting trip generation of residential land-use areas. Highway Research Board Bulletin Public Roads, 30(4), 88–99.
- Sillaparcharn, P. (2007). Vehicle ownership and trip generation Modelling. IATSS Research, 31(2), 17–26. https://doi.org/10.1016/s0386-1112(14)60218-1
- Sovacool, B. K., Kester, J., Noel, L., & de Rubens, G. Z. (2018). The demographics of decarbonizing transport: The influence of gender, education, occupation, age, and household size on electric mobility preferences in the Nordic region. Global Environmental Change, 52(June), 86–100. https://doi.org/10.1016/j.gloenvcha.2018.06.008
- Stiles, J., & Smart, M. J. (2021). Working at home and elsewhere: Daily work location, telework, and travel among United States knowledge workers. Transportation, 48(5), 2461–2491. https://doi.org/10.1007/s11116-020-10136-6
- Supernak J, Talvitie A. DeJohn A. (1983).Person-category trip-generation model. Report No. 944.500 Fifth Street, Washington DC, United States Transportation Research Board. http://onlinepubs.trb.org/Onlinepubs/trr/1983/944-011.pdf
- Talpur, M. A. H., Khahro, S. H., Ali, T. H., Waseem, H. B., & Napiah, M. (2023). Computing travel impendences using trip generation regression model: A phenomenon of travel decision-making process of rural households. Environment, Development and Sustainability, 25(7), 5973–5996. https://doi.org/10.1007/s10668-022-02288-5
- Uyanık, G. K., & Güler, N. (2013). A study on multiple linear regression analysis. Procedia - Social and Behavioral Sciences, 106, 234–240. https://doi.org/10.1016/j.sbspro.2013.12.027