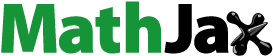
ABSTRACT
Ethiopia’s economy has made significant strides forward since the beginning of the 21st century. However, along with this, there is a high rate of urbanization, and internal migration, which lead to an increase in the number of vehicle owners in Addis Ababa city, causing traffic congestion problems. This is adversely impacting the socioeconomic and well-being of the residents. Therefore, this study attempted to identify the perception of drivers and passengers on the socio-economic impact (SEI) of traffic congestion in Addis Ababa city by taking the study corridors in the Kolfe keraniyo sub-city. Nine segments were sampled. Data collection included questionnaires distributed among 3240 respondents. A generalized ordered logit model was used. Accordingly, age, income, household size, gender, education, and respondent type (driver or passenger) were significant variables. The descriptive statistical results also indicated that the first, second, and third significant SEI perceived by the respondents were late arrival to the office, extra time added to the car or bus journey, and the increased risk of being in an accident. The outcomes of this study, including the responses to the research inquiries and the acquisition of extensive scientific knowledge, will contribute to the formulation of comprehensive mitigation strategies and management plans.
1 Introduction
Traffic congestion has been found to have a multitude of detrimental effects, encompassing economic losses as well as harmful repercussions on the environment and society (Andarge & Teklu, Citation2017; Sitotaw & Tekilu, Citation2019). The socio-economic impact of traffic congestion includes, among other things increase in travel time, unable to estimate travel time (unreliability), an increase in vehicle operating cost, and not making a driving trip that one would otherwise make (mobility cost) (HDR for the Office of Economic and Strategic Analysis, Citation2009; The World Bank Group, Citation2014). It also includes an increase in the risk of being in an accident (Anciaes et al., Citation2017; González-Sánchez et al., Citation2021; Vencataya et al., Citation2018) an increase in health costs (Levy et al., Citation2010; Vencataya et al., Citation2018), an increase in stress (Kumarage, Citation2004), increase in traffic noise (Anciaes et al., Citation2017b), decreases the time available for parents to spend with their families (severance) (Elisonguo, Citation2013; Pocock et al., Citation2009) and late arrival to office hours (Elisonguo, Citation2013; Oluwaseyi, Citation2017).
Ethiopia has been undergoing swift urbanization, particularly in its principal city, Addis Ababa, thus encountering notable challenges related to traffic congestion and road safety issues, as reported by the United Nations Human Settlements Programme (United Nations Human Settlements Program UN-HABITAT, Citation2017). The significant population surge in the urban area, owing with the high individual influx that migrating from various regions of the nation to the metropolis, has resulted in significant traffic congestion. Extended lines of individuals awaiting transportation services and congested traffic patterns at various intersections throughout the metropolis during peak commuting hours frequently observed. This results in the squandering commuters’ leisure or professional time and an escalation in vehicle operation and maintenance expenditures (HDR for the Office of Economic and Strategic Analysis, Citation2009; The World Bank Group, Citation2014). In relation to this, studies were conducted to explore the effects of traffic congestion. These studies primarily focused on evaluating direct costs of traffic congestion related to time loss, excessive fuel expenses, unreliable costs, inflated mobility, environmental and health costs (Elisonguo, Citation2013; HDR for the Office of Economic and Strategic Analysis, Citation2009; Ndatho, Citation2018; The World Bank Group, Citation2014).
According to Anciaes et al. (Citation2017) estimating these costs is based on mathematical models that estimate costs in the current state (actual) and the uncongested state (free flow). Nonetheless, the established framework cannot readily quantify certain socio-economic consequences in, where these impacts depend on the perceptions of the affected individual. The extent, characteristics, and magnitude of these effects are contingent upon the perceptions of the impacted individuals.
The research conducted by Fattah et al. (Citation2022) highlights a limitation in the direct cost calculation method, specifically its failure to account for the preferences of local communities regarding the varying importance of different consequences. Furthermore, research examining the correlation between commuters’ perceptions of the socio-economic consequences of traffic congestion and segments with various speed reduction indices also lacking. Additionally, the previous study should have considered the distinct analysis of drivers and passengers as separate entities to comprehend how these particular groups of the population interact with various influences. Kumarage (Citation2004) and Vencataya et al. (Citation2018) have posited that extended periods of commuting are associated with heightened levels of stress and aggressive driving tendencies. This behavior, in turn, may contribute to the development of road rage, which is often triggered by feelings of frustration stemming from sluggish traffic conditions.
Therefore, the objective of this study was to analyze the perceptions about the potential traffic-related impacts on the well-being of drivers and passengers on roads in the Kolfe-keraniyo sub-city of Addis Ababa. More specifically, the research tried to answer the following questions: a) what is the relative importance individuals attach to the different socio-economic impacts of road traffic congestion? b) what is the role of demographic and traffic-related factors in forming perceptions about the impacts? c) is there a significant difference between the perception of drivers and passengers in rating the impacts?
2 Literature review
Various kinds of research have been conducted to identify the socio-economic impacts of traffic congestion. So far, three methods have been utilized to identify the impacts. The first method estimates direct costs such as travel time, vehicle operating, unreliability, mobility, accident, and health costs (HDR for the Office of Economic and Strategic Analysis, Citation2009; The World Bank Group, Citation2014). The other alternative approach involves employing the concept of willingness to pay in order to indirectly mitigate a particular impact (Gabr et al., Citation2018; Samal et al., Citation2020; The World Bank Group, Citation2014). The third approach involves the identification of individuals’ perceptions regarding the impacts (Harriet et al., Citation2013; Vencataya et al., Citation2018; Wang, Citation2010).
Studies have been undertaken to examine the phenomenon of traffic congestion, with particular emphasis placed on the issue of employees experiencing delay for arrival to workplace (Elisonguo, Citation2013; Ndatho, Citation2018). Elisonguo (Citation2013) study revealed that road congestion has been identified as a significant factor contributing to delayed arrival at workplaces, which in turn resulting in negative socioeconomic consequences such as fewer delivery, lower productivity,and slower economic growth. In a similar vein, Ndatho (Citation2018) demonstrated that traffic congestion has the effect of impeding individuals’ commute to workplaces, thus resulting in a significant loss of productive hours and thereby induces a considerable challenge to employers. In relation to this, study conducted by Oluwaseyi’s (Citation2017) showed that nearly half (48.9%) of the survey participants expressed dissatisfaction with their consistent delay in commuting to and from work. This issue was found to have significant implications for their overall productivity.
Many researchers have also explored the extra time added to journeys in a direct congestion estimation method and identified that most of the traffic congestion cost is associated with travel time (Gabr et al., Citation2018; HDR for the Office of Economic and Strategic Analysis, Citation2009; The World Bank Group, Citation2014). According to a study conducted by Samal et al. (Citation2020), travelers experience a 50% loss in traffic efficiency per hour during their journey. The surplus time has the potential to be converted into valuable productive hours that could make a positive impact on the broader economy. According to Harriet et al. (Citation2013), a significant time which is equivalent to more than two working days per month lost due to additional commuting time. The extended duration of travel has also had an impact on family dynamics and is widely recognized as a contributing factor to conflicts between work and family responsibilities, as it reduces the amount of time parents are able to spend with their families (Pocock et al., Citation2009).
In addition, Elisonguo (Citation2013) identified that congestion has negative outcomes such as increased school dropout rates, drug addiction, and early engagement in sexual activities among children. This is attributed to the fact that parents spend a significant amount of time on the roads, resulting in reduced time spent at home with their children. Furthermore, it causes commuters to get extremely stressed and frustrated, especially drivers who need to pay closer attention and concentrate when driving in difficult circumstances (Vencataya et al., Citation2018). According to Kumarage (Citation2004), extended periods of commuting contribute to heightened stress levels and the manifestation of aggressive driving tendencies, ultimately fostering road rage as a result of frustration stemming from sluggish traffic. Consequently, individuals become more susceptible to stress-related ailments and experience a decline in work productivity. In the study conducted by Berisha (Citation2016), participants were asked regarding their affective disposition during instances of being immobilized in traffic congestion. The findings indicated that a proportion of 20.6% of individuals exhibited calmness, while a majority of 45.8% experienced frustration. Additionally, a significant portion of 33.6% reported feelings of boredom, and a substantial proportion of 44.6% reported experiencing stress. Additionally, the data revealed that a majority of the participants who reported experiencing negative emotions were from older age cohorts.
Moreover, it has been found in previous studies that traffic congestion has a direct correlation with the likelihood of accidents occurring (Anciaes et al., Citation2017; González-Sánchez et al., Citation2021; Vencataya et al., Citation2018). According to Vencataya et al. (Citation2018), the descriptive statistics revealed that accidents pose a threat to the safety of individuals who commute. Anciaes et al. (Citation2017) employed the conditional logit model to ascertain the significance of the risk of accidents as a social impact of traffic congestion. Their findings revealed that the risk of accidents ranked fourth in terms of significance, with an odds ratio of 0.42. Households that include children and respondents with higher income levels placed greater significance on accidents compared to individuals with different socioeconomic indicators (SEIs). In a recent study conducted by Sánchez González et al. (Citation2021), the authors employed big data analysis and a Poisson model to ascertain the impact of a 10% decrease in traffic delay on accident rates. Their findings revealed that such a reduction in traffic delay would result in a corresponding decrease of 3.4% in accidents. This reduction in accidents is estimated to amount to over 72 thousand traffic accidents. Furthermore, it has been observed that the presence of heavy traffic congestion frequently leads to an increased occurrence of accidents, as drivers often engage in risky behaviors in an attempt to navigate through congested roadways more swiftly than their counterparts (Elisonguo, Citation2013).
Another source of congestion that has been identified in the academic literature is the issue of mobility. Mobility refers to the capacity of individuals and commodities to navigate with convenience, security, expediency, and dependability (Falcocchio & Levinson, Citation2015). According to the HDR for the Office of Economic and Strategic Analysis in 2009, the presence of congestion serves as a deterrent for individuals and businesses to utilize the road network for their travel needs. According to a report by the Office of Economic and Strategic Analysis in 2009, the US Department of Transportation projected the monetary impact of congestion on mobility to be approximately $3.1 billion, representing approximately 4% of the overall cost of congestion. In the study conducted by Anciaes et al. (Citation2017), mobility was recognized as the eighth social impact among a total of 20 other social and environmental indicators (SEIs). The odds ratio associated with mobility was found to be 0.27.
Vehicle operating costs are recognized as a constituent element of the effects of traffic congestion. According to Elisonguo (Citation2013), the phenomenon of traffic congestion has been found to have a direct impact on the fuel consumption and depreciation of vehicles. Consequently, commuters are compelled to allocate a greater portion of their budget towards fuel expenses. According to the Office of Economic and Strategic Analysis (2009), the HDR report provided an estimation of the vehicle operating cost associated with congestion, which amounted to $11.2 billion. This cost was found to constitute approximately 13% of the overall congestion cost. Errampalli et al. (Citation2015) conducted a study in which they observed that the impact of congestion on fuel costs is more pronounced in the context of heavy commercial trucks. According to the study conducted by Oluwaseyi (Citation2017), it was determined that a proportion of 10.8% of the participants reported an increased consumption of fuel, resulting in subsequent delays in the transportation of their merchandise.
Similarly, the occurrence of traffic congestion is associated with adverse health outcomes. Based on a survey conducted by Vencataya et al. (Citation2018), individuals who commute and have asthma or other respiratory conditions may have an increased susceptibility to more severe illnesses as a result of exposure to air pollution generated by vehicle emissions. Additionally, it leads to untimely fatalities caused by individuals inhaling primary PM2.5 and particles formed as a secondary effect of traffic congestion (Levy et al., Citation2010). According to the findings of Fattah et al. (Citation2022), a significant proportion of respondents, specifically 67%, expressed the perception that there is a correlation between the occurrence of severe traffic congestion and the prevalence of diseases. Furthermore, it is worth noting that a significant proportion of individuals, specifically 35%, experience headaches. Additionally, a considerable percentage of individuals, amounting to 24%, encounter an increase in body temperature upon returning home. Moreover, a notable 28% of participants report feelings of fatigue, while a smaller subset, comprising 13%, indicate experiencing eye-related issues. The multitude of health concerns that emerge due to traffic congestion may potentially affect the productivity of operators. In addition, individuals residing in proximity to heavily trafficked roadways are consistently exposed to noise levels that surpass the permissible thresholds. The persistent exposure to noise pollution raises concerns regarding its detrimental effects on human health (Halim & Abdullah, Citation2014). The noise was determined to be the second most significant social impact when compared to 20 other social and environmental indicators (Anciaes et al., Citation2017), with an odds ratio of 2.17.
The demographic characteristics of respondents have been found to influence various impacts, including perceptions of traffic (Alinizzi et al., Citation2022; Anciaes et al., Citation2017). In their study, Anciaes et al. (Citation2017) examined several demographic factors, including age, gender, number of children in the family, employment type, income, and distance from the main roadway, in order to assess their influence on respondents’ decision-making process when selecting a particular impact. The researchers employed the conditional logit model to ascertain the relationship between factors and their corresponding impacts. Consequently, the proximity to the primary roadway was determined to be a significant determinant in the selection of vibration as a crucial factor. Likewise, the presence of children and a high income were identified as factors that significantly influence the selection of accident risk. The selection of air pollution as an impact was influenced by factors such as low income and the number of children. The identification of gender as a determinant in the decision to refrain from making driving trips that would have otherwise been undertaken has been recognized as a significant influence.
In their study, Alinizzi et al. (Citation2022) categorized the factors influencing the perception of socio-economic consequences of traffic congestion during the COVID-19 pandemic into two distinct categories: internal and external. The internal factors encompass various variables such as family size, job characteristics, educational attainment, age, vehicle ownership, and driver availability. The external factors encompass variables such as proximity to the shopping center, city size, departure delay, and duration of delay. The statistical analysis using the chi-square test indicated that there is a significant association between commuters with larger family sizes and the delay in their departure from home to the shopping center.
In a similar vein, Fattah et al. (Citation2022) employed analysis of variance to ascertain the influence of various demographic factors, namely age, gender, employment, education, and income groups, on the psychological strain induced by traffic congestion and the level of satisfaction reported by respondents. The respondents experienced a substantial and varying level of impact from traffic congestion, which was found to be significantly influenced by factors such as age, gender, employment, education, and income groups. The highest score (3.39 ± 0.60) was observed among individuals employed as garment workers.
In addition, the research done by Samuel et al. (Citation2023) pertaining to the perception of vehicle traffic emissions and their associated health risks shown that a significant proportion (78.9%) of the participants exhibited awareness of the presence of hazy air pollution caused by automobiles, as well as its detrimental impact on human health. The findings demonstrated that age, education gender, marital status, job status, and road proximity, exhibited statistical significance with individuals’ perception of traffic emissions.
Despite the challenges posed by congestion in terms of its social, economical and environmental consequences, governments throughout the globe have adopted remedial actions, although with various degrees of public support. This phenomenon encompasses a change in individuals’ behavior, namely a preference for using public transportation instead of private vehicles, as well as making personal sacrifices (Johan & Permilla, Citation2020). However, the success of this behavioral adjustment is contingent upon the desire of individuals to embrace and adopt these practices (Francisco et al., Citation2023).
Maria-Alexandra (Citation2023) conducted a comprehensive review on the influence of transportation decisions on the development of sustainable mobility and the environment. The study conducted an analysis of several instances of both success and failure, attributing them to the level of willingness shown by those within the respective sector. According to the reviewer, policy measures aimed at addressing sustainable transportation include implementing car-sharing programs, establishing electric vehicle charging stations, and enhancing public transportation options. These measures can be further reinforced by promoting car-free mobility, developing an extensive network of cycling infrastructure, promoting energy-efficient housing, and creating green spaces.
Hussein et al. (Citation2016) performed a research which revealed that the inclination to choose for more sustainable modes of transportation is contingent upon individuals’ degree of knowledge of the environmental consequences of air pollution resulting from automotive traffic. Furthermore, the increasing benefits of reducing pollution encourage citizens to choose for other modes of transportation such as bicycles and buses.
The Minister of Transport and Logistics of the Federal Democratic Republic of Ethiopia (Citation2020) has identified many measures to address congestion and mitigate its effects. These measures include the promotion of cycling, improvement of public transit, and regulation of private car use. According to the research conducted by Eleni et al. (Citation2023) about transport mode choice in Addis Ababa, it was observed that there has been a notable rise in the usage of public buses. The observed phenomenon may be ascribed to several variables, including the accessibility of reasonably priced transportation prices and the enhanced time effectiveness, especially in the context of long-distance journeys. The researchers observed that a considerable proportion of the city’s inhabitants go from far areas for their daily commute, and the presence of public buses plays a crucial role in addressing socioeconomic and environmental issues.
3 Material and methods
3.1 Description of the study area
Addis Ababa is the capital city of Ethiopia which was established in 1886. In geographical terms Addis Ababa is located between 8°50’ to 9°06’N latitude and 38°39’ to 38°55’E longitude covered 540 square kilometers. The City Administration is subdivided into 11 sub-cities and 118 woredas (districts) for administrative purposes (). The city’s spatial population distribution is mainly towards the central city’s eastern, southern, and South Western parts.
Kolfe Keraniyo is one of the 11 sub-cities. According to Addis Ababa city administration data, the sub-city has a population of 546,219 people, with 220,859 men and 235,360 women, and covers an area of 61.25 square kilometers. According to the Central Statistics Service (CSS), the people in the sub-city work in manufacturing and industry, trade and commerce, home making, agriculture, hotel and catering services, and health and social service. Aside from residents of Addis Ababa’s rural areas, city dwellers also participate in animal husbandry and garden cultivation.
The sub city is located in the western part of the city and functions as a central point for residents traveling from several regional towns, such as Woletie, Alemgena, Sebeta, and Burayu, to participate in their daily activities inside the city of Addis Ababa. The sub city is well-known for its uncontrolled expansion of urban areas in a horizontal manner and the existence of informal settlements. These settlements are formed when residents move out from the city center to obtain land at a comparatively cheaper price and build houses without according to legal building requirements. Moreover, due to the significant surge in rental prices inside the core section of the city, residents have been exploring more economically viable housing alternatives at the periphery of Addis Ababa. As a result the sub city’s population size is seen to be progressively expanding over time. The sub city has also two out of the total five gateways that connect Addis Ababa with the regional towns. The arrival and departure of a large number of persons in the sub city throughout the morning and evening sessions has resulted in a notable problem of traffic congestion, varying from moderate to high levels. Therefore, the choice of the sub city as a study subject is associated with the fact that its roadways function as a means of transportation for both residents of the sub city and those coming from other places. Consequently, a comprehensive evaluation of all routes inside the sub city has still to be done.
4 Method
4.1 Population, sampling technique and sample size determination
After selecting Kolfe keraniyo sub city as a study area by considering it as a cluster, exhaustive analysis was made on all roads in the sub city. Four groups of populations linked together to estimate the perception of drivers and passengers on the socio-economic impact of traffic congestion were identified in the study area. These are routes (segments), vehicles, drivers, and passengers. Fourteen routes (7 for taking and 7 for returning) or 36 segments were identified in the study area. The routes convey passengers from the endpoints of the sub-city to the business districts, i.e. Addis sub-city, Lideta sub-city, and Nifasilik lafto. In addition, they can serve as an entry from the suburbs (specifically from Woletie, Ashewa Media, and Burayu) to Addis Ababa city. In addition, some of these routes implemented the same policy measures to reduce traffic congestion.
A census was done to identify the segments that have acceptable congestion (Speed reduction index (SRI)>5) (Afrin & Yodo, Citation2020; He et al., Citation2016; Mohan Rao & Ramachandra Rao, Citation2012) between 7 AM to 8 PM. As can be seen in and , the prior census on the identified segments indicated that nine segments qualify for the congestion criteria. Furthermore, seven of these segments qualify only in the morning between 7 AM and 11 AM, while two qualify for congestion criteria only in the evening between 4 and 8 PM. However, no acceptable congestion was obtained in the study area between 11 AM and 4 PM. Therefore, the segments identified to represent the sub-city traffic congestion are given in the following graph and table.
Table 1. Population and sample size of the study population.
Following segment identification, data were collected on the number and type of vehicles passing through endpoints at 30-minute intervals during pick hours (7 AM to 11 AM and 4 PM to 8 PM).In addition vehicle occupancy rate of each vehicle groups were identified. As a result, shows the population size passing through each segment and the study’s sample size determined based on Yamane (Citation1967).Accordingly, a total of 343,020 drivers and passengers were identified as a study population.
According to Yamane (Citation1967), the sample size of the population is calculated using the following formula
n = N/(1+N(e)2.
Where N= population sizen= desired sample size e = the margin error in the calculation
Therefore, the sample size of the study is given in the following table
Since each of the nine segments has a different population, the sample was drawn per each segment based on simple random sampling. The population using the specified segment or passing at each traffic point is grouped into two strata, i.e. the drivers and the passengers. After determining the sample size for each stratum, the sampling distribution was carried out so that drivers and passengers from all vehicle groups were included. After determining the sample size in each segment, equal weight was assigned to both strata (the driver and the transport users), as shown in . This was done to include more drivers in the sample, which is critical to answering the research question.
As a result, structured survey questionnaires were distributed to transport users and drivers to determine their perception of the socio-economic impact of vehicle traffic congestion. The questionnaire has three major parts: The first part contains information about the demographic characteristics of the respondents, the second part about travel behaviors, and the third part about the socio-economic impacts to be rated by the respondents (see annex 1). The samples were drawn randomly from various types of vehicles arriving at the traffic points (those in the queue). Data was collected from April 4 to May 4/2022.
Previous research and reports about traffic management and congestion were also reviewed to substantiate the research output found by other means.
4.2 Research design
This study utilized a descriptive research design to describe the socio-economic impacts and socio- demographic factors and a correlational design to identify the factors that influence drivers’ and passengers’ perceptions of the socio-economic impact. Quantitative data were collected using a survey method. The preliminary (pilot) survey and consultation of experts identified ten socioeconomic impacts, including traffic noise, increased risk of being involved in an accident, extra time added to car or bus journeys, not driving trips that would otherwise be made, increased stress, affected health, severance (decreases time available for parents to spend with their families), inability to estimate travel time, increase in vehicle operating cost, and late arrival at the office. Finally, the identified SEI were served to respondents (drivers and passengers) to know their perception on a Likert scale (strongly disagree, disagree, neutral, agree, and strongly).
Apart from knowing the level and the type of the impacts, the study was geared to know how the perceived impacts were associated with the demographic characteristics and other related variables using an ordinal logistic regression model. The rationale for choosing this model is that the dependent variable is categorical (have five outcomes) and is ordered in preference.
According to Agresti (Citation2002), ordinal logistic regression model is parameterized as:
It is also possible to compute the predicted probabilities as,
Following the theoretical models described above, the specification of the models considered in this study are given below.
Where π k are probabilities for k = 1, 2, …, K.; K is the number of distinct categories of the response; k equals 1 … ., K − 1; θk is constant; β is a vector of coefficients from the logit equation; θk is constantly associated with the kth distinct response category; x is a vector of predictor variables;
In our model, we have five ordered response variables (strongly disagree, disagree, neutral, agree, and strongly agree). i.e. k = 5 and ten predictor variables, x: Age, Gender, Marital status, Income, Family size, Work experience, Occupation, education, Segment speed reduction index, and the Respondent type and; y is the outcome of the dependent variable (perceived socio-economic impact). The estimated coefficients are calculated using maximum likelihood estimation.
The explanatory variables, in general, are related to demographic characteristics (age, gender, marital status, income, work experience, respondent type, and education) as well as traffic-related variables (segment speed reduction index). Anciaes et al. (Citation2017) identified the demographic variables specified as explanatory variables. In addition, they include traffic-related variables in their study. Every perception survey is indeed affected by the respondents’ demographic characteristics. An ordinal logistic regression model was run on SPSS to identify the factors influencing the respondents’ decision on the Likert scale per the identified socio-economic impact.
The variables considered in the study and descriptive characters are briefly summarized in respectively.
Table 2. Description of variables.
Table 3. Descriptive characteristics of the respondents.
5 Result
5.1 Demographic characteristics of the respondents
The study planned to incorporate 3560 samples using the survey method. However, because of the nature of the data collection, which is distributing the questionnaire on the roads to the respondents and collecting it on the other days (maximum of one month time), the response rate was 91%.i.e., 3240 drivers and passengers were participating in this study. As shown in , 77.5 % of the respondents were males, while 22.5% were females. In addition, the majorities (48%) of the respondents were in the age range between 26 and 35, whereas 24.4% were in the age range between 36 and 45, and 16.1% were below 25. The remaining 8.9% and 2.5% were in the age range between 46 & 55 and over 56, respectively. Regarding respondents’ education level, 39.4% hold their diplomas, while 36.25 completed their high school studies.18.5% graduated with their degree or master’s degree. The remaining 5.8% were below 8th grade. In addition, 60.2% of the respondents were married, 37.5% were single, and the remaining 2.5% were divorced.
Furthermore, 57.1% of the respondents had an income level between 5,001 &7,500, while 24.5% were between 2,001 & 5,000. The remaining 8.1%, 5.6%, 2.3%, and 2.4% had income levels less than 2,000, between 7,501 & 10000, 10001 &12,500, and greater than 12,501 respectively. This indicates that most of the respondents were in the income range between 2,001 &10,000 (79.6%). According to salary explore (www.salaryexplorer.com), the national average salary of a person in Ethiopia is 8900 birrs, with the salary ranging between 2250 (lowest average) and 39,700 (highest average). This generally indicates that the samples were in the national range.
Regarding the occupation of the respondents, 44.7% were working as an employee in a private office, while 39.5% were working at their private job. The remaining 15.8% were working as an employee in the government office. Furthermore, 51.3 % of the respondents have experienced more than 10 years, while the remaining 48.7% are below 10.
5.2 Perceived impacts
As depicted in , the mean of different impacts was found to be 3.9, indicating that most respondents agreed with the identified possible impacts. The result in also indicated that late arrival to an office was the first significant perceived socio-economic impact of traffic congestion, and extra time added to the car or bus journey was the subsequent significant perceived socio-economic impact of traffic congestion with a mean value of 4.277 and 4.155, respectively. Not making driving trips one would normally go stood third as a significant perceived socio-economic impact of traffic congestion with a mean value (of 4.1278). A similar result was obtained using different questions administered to the respondents. The result in shows that late arrival to an office ranked first by 16.1% of the total respondents, while extra time added to car or bus journeys stood second by 12.4%. Not making driving trips one would have otherwise made stood third with a share of 9.3%. The non-parametric test using chi-square, which indicates the significance of the variation between the expected and actual answers by the respondents, depicted that all the socio-economic impacts included in the study had a significant difference from the expected.
Table 4. Perceived socio-economic impact (1) = strongly disagree, (2) = disagree, (3) = neutral, (4) = agree, (5) = strongly agree.
Table 5. Major/An essential socio-economic impact.
The regression result in indicated that the type of the respondent (driver or passenger), age, gender, education, household size, income, and occupation significantly affect the respondents’ perception. In contrast, segment type (Speed reduction index), marital status, and work experience do not affect the perception of the response. The likelihood ratio chi-square test indicates that there is a significant improvement in the fit of the final model over the null model (Chi-Square value = 2115.996; p value = .000).
Table 6. Parameter estimates of ordinal logistic regression.
The finding from the regression result in shows that the respondents’ age, income, and household size are significant and are positive predictors of the respondent’s perception in rating the socio-economic impacts. For example, for every one-unit increase in age, income, and household size, there is a predicted increase of 0.044, 0.354, and 0.106 in the log odds of a respondent being in a higher(as opposed to lower) category of the socio-economic impacts. Similarly, for gender (0= female and 1=male) and respondent type (0=passenger and 1=driver), the result from regression output indicates that for every one-unit increase in the number of males and drivers, there is a predicted increase of 0.412 & 0.209 in the odds of falling at a higher level of the response variable respectively.
Gender and Respondent type were also significant positive predictors of the perception of the socio-economic impacts. Since both are binary variables, the slope represents the difference in log-odds between female & male and passenger & driver, respectively. The log odds of being at a higher level on the responses in the Likert scale were 0.412 points higher on average for those who were male compared to females. Similarly, the log odds of being at a higher level on the responses in the Likert scale were 0.209 points higher on average for drivers compared to passengers.
Predictors with three and more categories are interpreted differently by considering one reference category within the group. Among the predictors with three categories, only education is significant. Only two categories (below grade 8 and 9–12 grade) significantly varied with the perception of the responses when compared to respondents with master level. For every one-unit increase in the lowest education category compared to the masters’ group, there is a predicted decrease of 0.735 in the log odds of being in a higher level of the responses in the Likert scale (agree or strongly agree). Similarly, for every one-unit increase in the grade 9–12 education category compared to the masters’ group, there is a predicted decrease of 0.486 in the log odds of being in a higher level of the responses in the Likert scale (agree or strongly agree) at 10% level of significant.
The goodness-of-fit test indicates that the deviance statistics (p-value = 1.000) exhibits a significant magnitude. This observation suggests that the model is a good fit for the data. In addition, it is observed that Nagelkerke’s R-Square value is 0.583s, indicating that the explanatory variables included in the model account for approximately 58.7% of the variability observed in the response variables. The remaining 35.3% of the variability is attributed to error terms and unobserved factors.
6 Discussion
The objective of this study was to evaluate the perceived socio-economic consequences of traffic congestion on road users in Addis Ababa, Ethiopia. The calculated composite mean of 3.8 suggests that a significant proportion of the participants are in concurrence with the identified effects. The findings align with the current body of literature in terms of the average values observed for individual factors and items. According to Fattah et al. (Citation2022), the respondents’ overall perception leaned towards perceiving a greater number of positive socio-economic impacts rather than negative ones. In addition, a study conducted by Samuel et al. (Citation2023) identified that a significant proportion (78.9%) of the participants acknowledged the adverse effects of vehicle traffic emission on human health. In their study, Sulaiman and Wahyuni (Citation2023) also observed that around 90.61% and 91.84% of the participants expressed agreement or strong agreement on the influence of congestion on the social well-being and economy of drivers respectively. Furthermore, according to the aforementioned study, a significant majority of respondents (91.84%) expressed agreement or strong agreement regarding the environmental implications of congestion.
The results of the ordinal logistic regression analysis revealed that age, income, and household size were found to be statistically significant predictors of respondents’ perception in rating the socio-economic impacts. Furthermore, these predictors exhibited a positive relationship with the perceived impacts. This suggests that the effects are more pronounced among participants who are older, have higher incomes, and have larger households, compared to those who are younger, have lower incomes, and have smaller households, respectively. As individuals progress in age, they assume greater obligations in the management of their own livelihoods as well as the livelihoods of others. Therefore, the degree of perception of the impacts varies accordingly. This is supported by different researches. Francisco et al. (Citation2023) identified age as a positive predictor of the degree of perceived social concern to act on vehicle-caused air pollution. Furthermore, Samuel et al. (Citation2023) identified age as positive predictors of the perception of participants on effects of vehicle traffic emission on human health.
Likewise, individuals with higher levels of income perceive traffic impacts as being more favorable in comparison to those with lower levels of income. The revenue of the exposed groups is implied by any spare time sacrificed as a result of congestion. There is a mixed result on the relationship between income and perception of traffic related impacts. According to the HDR for the Office of Economic and Strategic Analysis (Citation2009) and The World Bank Group (Citation2014), there exists a direct relationship between the lost revenue due to traffic congestion and the revenue generated per hour. In contrast, Francisco et al. (Citation2023) identified that income levels were found to negatively affect the individuals’ concern about pollution arose from vehicle traffic emission. According to Anciaes et al. (Citation2017), individual with higher income tend to place more significance on accident risk, while those with lower income tend to prioritize reduced automobile trips and place less emphasis on air pollution, in comparison to individuals with middle income.
Moreover, individuals residing in larger households exhibited a greater degree of concern regarding the consequences, in contrast to those residing in smaller households. The extended duration of travel has a significant influence on the dynamics of family life and is widely recognized as a contributing factor to work-family conflicts due to its detrimental effect on the amount of time parents are able to allocate to their families (Pocock et al., Citation2009). According to Alinizzi et al. (Citation2022), the departure of commuters from home to shopping centers is delayed by individuals with larger family sizes. Furthermore, Anciaes et al. (Citation2017) identified that households with more household size perceived accident, one of the socioeconomic impacts of congestion more than other SEIs.
In a similar vein, it was observed that both gender and respondent type exhibited a noteworthy positive correlation with the perception of socio-economic impacts. According to a study conducted by Lailulo et al. (Citation2015), there is a notable gender disparity in various areas of responsibility within Addis Ababa city. Specifically, it was observed that a larger proportion of males are engaged in sectors such as business, office work, and driving, compared to females. Anciaes et al. (Citation2017) identified Gender as a significant variable for the ‘avoid car trips’ impact indicating that females were less concerned with this problem than males.
The results of the study also indicated that drivers had a greater perception of the impacts compared to passengers. This phenomenon primarily arises due to the fact that drivers constitute the primary demographic that is exposed to congestion. The individuals in question are both responsible for the occurrence of congestion and also bear the consequences of it. The perception of the impacts varies, as drivers are the ones who bear the vehicle operating costs, in addition to the common issue of congestion experienced by passengers. The extended duration of commuting has been found to be a contributing factor to heightened levels of stress and the manifestation of aggressive behavior among motorists, which in turn leads to incidents of road rage. This can be attributed to the frustration experienced by individuals when faced with the slow progress of traffic (Kumarage, Citation2004; Vencataya et al., Citation2018).
Of the predictors examined, only education demonstrated statistical significance within the three categories. There is a notable disparity in the perception of responses between respondents with a master’s level of education and those in two specific categories: below grade 8 and grades 9–12. The relationship between the perception of impact and academic level is negative, indicating that commuters with lower grades perceived the impact to be less significant compared to those with a master’s level of education. Typically, individuals with a higher level of education are presumed to possess a greater understanding of the ramifications associated with traffic congestion compared to those with a lower level of education. Furthermore, it is worth noting that individuals with higher levels of education tend to receive higher salaries compared to those with lower levels of education. This disparity in income has a significant impact on their overall earnings. According to the findings of Samuel et al. (Citation2023), those who have had formal education, including both basic and university education, have a higher level of awareness of automobile emissions compared to those who are illiterate. In a study conducted by Francisco et al. (Citation2023), it was shown that educational attainment had a beneficial influence on people's level of worry over pollution. Additionally, higher levels of education were associated with a greater recognition of the need for action in addressing this issue. Specifically, persons with advanced educational degrees demonstrated a heightened awareness of environmental concerns and placed greater significance on taking proactive measures to combat pollution.
Another unexpected finding is that there is no discernible variation in the perception of commuters who travel in different segments with varying speed reduction indices. The congestion cost function exhibits variation across segments due to differing speed reduction indices. According to The World Bank Group (Citation2014), there is a positive correlation between the increase in the index and the expected increase in congestion cost, as well as a negative correlation in the opposite direction. The primary explanation for the minimal disparity observed across these segments is primarily attributed to the fact that a commuter may travel on multiple segments within a single day. Hence, it is not possible to ascertain the impact based on the segments, as is typically done in congestion estimation.
According to the results presented in , the primary socio-economic consequence identified by the participants was a delayed arrival to the workplace. Several studies have also indicated that traffic congestion has a significant effect on employees’ punctuality, resulting in late arrival to the office (Elisonguo, Citation2013; Ndatho, Citation2018; Oluwaseyi, Citation2017). The consequences of employees arriving late to their workplaces include decreased output, missed deliveries, diminished productivity, and hindered economic growth (Elisonguo, Citation2013; Oluwaseyi, Citation2017). Likewise, the commuters identified an additional time allocation to their car or bus journeys as another notable socio-economic impact. This phenomenon is further supported by the literature, as indicated by the studies conducted by Gabr et al. (Citation2018), the HDR for the Office of Economic and Strategic Analysis (Citation2009), and The World Bank Group (Citation2014). According to a study conducted by Samal et al. (Citation2020), it was found that travelers experience a significant loss of 50% of their traffic time per hour during their journeys. This loss of time represents a missed opportunity for productive work that could potentially contribute to the overall economy. The extended duration of travel has a notable influence on familial dynamics and is widely recognized as a contributing factor to work-family conflicts due to its reduction of parental involvement in family activities (Pocock et al., Citation2009). One of the notable socio-economic impacts identified by the commuters was the heightened probability of being involved in a vehicular collision. Several other studies have also recognized this influence (Anciaes et al., Citation2017; González-Sánchez et al., Citation2021; Vencataya et al., Citation2018). According to the study conducted by Anciaes et al. (Citation2017), the fourth major social impact was found to be the risk of accidents. In their study, Anciaes et al. (Citation2017) determined that air pollution, noise, and vibration were the initial three socio-economic impacts in their original model. The coefficients of the intercept for these impacts were found to be 2.173, 2.135, and 1.782, respectively.
7 Limitation of the study
This research has endeavored to ascertain the socioeconomic impacts of traffic congestion by careful identification of the study population. In light of the dispersed nature of the populations under consideration, our study aimed to ascertain the extent to which these populations engage with the segments in question. Our findings indicate that more than 90% of the populations surveyed are regular users of these segments. It is also known that a significant proportion of drivers on the streets of Addis are male, indicating the presence of a gender bias. The researcher has made efforts to address this prejudice by attempting to include more female participants; however limitations in achieving gender balance still persist. Furthermore, there exists a certain degree of bias in the age distribution, since a larger proportion of young individuals are seen to be operating vehicles on the streets of Addis Ababa. Finally, in light of the absence of a sampling framework for selecting a sample based on simple random sampling prior to data collection, we opted to distribute the questionnaire randomly when cars arrived at the designated segments. This approach was necessitated by our limited access to the list of population traveling these segments.
8 Conclusion
The present study aimed to investigate the perceived socio-economic impacts of traffic congestion in Addis Ababa, with a specific focus on the kolfe keraniyo sub-city as the designated study area. Therefore, we endeavored to ascertain the significance of these perceived impacts. We also examined the potential influence of demographic and segment characteristics on the perception and rating of the response variable, as well as identified the most substantial impacts. The results of the study revealed that the composite index of the response variable was 3.8, suggesting that a significant proportion of the participants held the perception that the impacts were either agreeable or strongly agreeable. Furthermore, it was observed that the primary SEIs reported by individuals were as follows: late arrival to the office accounted for 19.8% of the responses, extra time added to the car or bus journey constituted 16.5% of the responses, and an increased risk of being involved in an accident was identified as the third most prevalent SEI, accounting for 13.2% of the responses. The results of the ordinal logistic regression analysis indicate that age, income, household size, gender, and respondent type were found to be statistically significant predictors of the respondent’s perception in rating the socio-economic impacts. Furthermore, these predictors were positively associated with the respondent’s perception. Surprisingly, there is no discernible correlation between the segment index and the perception of impacts. However, the type of respondents (whether they are drivers or passengers) does have a noteworthy influence. The study adhered to a rigorous methodology, encompassing the design of the survey, the selection of the population and samples, in order to obtain data that accurately represents the target population. This approach instills confidence in the reliability of the study’s findings. The outcomes of this study, including the responses to the research inquiries and the acquisition of extensive scientific knowledge, will contribute to the formulation of comprehensive mitigation strategies and management plans. These plans will incorporate stakeholder engagement in the context of sustainable transportation planning.
Availability of data and material
Data will be availed on request.
Disclosure statement
No potential conflict of interest was reported by the author(s).
Additional information
Funding
References
- Afrin, T., & Yodo, N. (2020). A survey of road traffic congestion measures towards a sustainable and resilient transportation system. Sustainability (Switzerland), 12(11), 4660. https://doi.org/10.3390/su12114660
- Agresti, A. (2002). Categorical data Analysis (2nd Revised ed.). John Wiley & Sons, Inc.
- Alinizzi, M., Haider, H., & Alresheedi, M. (2022). Assessing traffic congestion hazard period due to commuters’ home-to-shopping center departures after COVID-19 curfew timings. Computation, 10(8), 132. https://doi.org/10.3390/computation10080132
- Anciaes, P. R., Metcalfe, P. J., & Heywood, C. (2017). Social impacts of road traffic: Perceptions and priorities of local residents. Impact Assessment and Project Appraisal, 35(2), 172–24. https://doi.org/10.1080/14615517.2016.1269464
- Andarge, K., & Teklu, B. (2017). Analysis of traffic congestion and its economic cost in Addis Ababa: A case study of Meskel Square to kality interchange. http://etd.aau.edu.et/bitstream/handle/123456789/15925/Kefyalew%20Tadele.pdf?sequence=1&isAllowed=y
- Berisha, B. (2016). Alleviating Traffic Congestion in Prishtina [ Thesis]. Rochester Institute of Technology. https://scholarworks.rit.edu/theses/9054
- Eleni, G., Berhanu, W., & Mintesnot, W. (2023). Travel mode preference in Addis Ababa, Ethiopia: empirical observations. Urban, Planning and Transport Research, 11(1), 1, 2228380. https://doi.org/10.1080/21650020.2023.2228380
- Elisonguo, A. D. (2013). The social-economic impact of road traffic congestion in Dar Salaam region [ Dissertation].
- Errampalli, M., Senathipathi, V., & Thamban, D. (2015). Effect of congestion on fuel cost and travel time cost on multi-lane highways in India. International Journal for Traffic and Transport Engineering, 5(4), 458–472. https://doi.org/10.7708/ijtte.2015.5(4).10
- Falcocchio, J. C., & Levinson, H. S. (2015). The impact of traffic congestion on mobility. In Book series Springer tracts on transportation and traffic (Vol. 7, pp. 119–131). Springer International Publishing. https://doi.org/10.1007/978-3-319-15165-6_10
- Fattah, M. A., Morshed, S. R., & Kafy, A. A. (2022). Insights into the socio-economic impacts of traffic congestion in the port and industrial areas of Chittagong city, Bangladesh. Transportation Engineering, 9, 9. 100122. https://doi.org/10.1016/j.treng.2022.100122
- Francisco, A., Mireia, F., Cristina, E., & Sergio, A. (2023). Who wants to change their transport habits to help reduce air pollution? A nationwide study in the Caribbean. Journal of Transport & Health, 33(2023), 101703. https://doi.org/10.1016/j.jth.2023.101703
- Gabr, A., Shoaeb, A., & El-Badawy, S. (2018). Economic impact of urban traffic congestion on the main routes in Mansoura city, Egypt. International Journal for Traffic and Transport Engineering, 8(2), 148–165. https://doi.org/10.7708/ijtte.2018.8(2).01
- González-Sánchez, G., Olmo-Sánchez, M. I., Maeso-González, E., Gutiérrez-Bedmar, M., & García-Rodríguez, A. (2021). Needs for international benchmarking of road safety management based on mobility exposure measures and risk patterns. International Journal of Environmental Research and Public Health, 18(23), 12851. https://doi.org/10.3390/ijerph182312851
- Halim, H., & Abdullah, R. (2014). Equivalent noise level response to number of vehicles: A comparison between a high traffic flow and low traffic flow highway in Klang Valley, Malaysia. Frontiers in Environmental Science, 2(13), 1–4. https://doi.org/10.3389/fenvs.2014.00013
- Harriet, T., Poku, K., & Kwabena Emmanuel, A. (2013). An assessment of traffic congestion and its effect on productivity in urban Ghana. International Journal of Business & Social Science, 4(3). www.ijbssnet.com
- HDR for the Office of Economic and Strategic Analysis. (2009). Assessing the full costs of congestion on surface transportation systems and reducing them through pricing. https://www.transportation.gov/sites/dot.dev/files/docs/Costs%20of%20Surface%20Transportation%20Congestion.pdf
- He, F., Yan, X., Liu, Y., & Ma, L. (2016). A traffic congestion assessment method for urban road networks based on speed performance index. Procedia Engineering, 137, 425–433. https://doi.org/10.1016/j.proeng.2016.01.277
- Hussein, M., Koorosh, B., Mohammad, T., & Pegah, N. (2016). The impact of outcome framing and psychological distance of air pollution consequences on transportation mode choice. Transportation Research, Part D: Transport & Environment, 46(2016), 328–338. https://doi.org/10.1016/j.trd.2016.04.012
- Johan, H., & Permilla, I. (2020). Mode choice in home-to-work travel in mid-size towns: The competitiveness of public transport when bicycling and walking are viable options. Transportation Research Procedia, 48(2020), 1635–1643. https://doi.org/10.1016/j.trpro.2020.08.204
- Kumarage, A. S. (2004). Urban traffic congestion: The problem & solutions. https://www.researchgate.net/publication/311375042
- Lailulo, Y. A., Susuman, A. S., & Blignaut, R. (2015). Correlates of gender characteristics, health and empowerment of women in Ethiopia. BMC Women’s Health, 15(1). https://doi.org/10.1186/s12905-015-0273-3
- Levy, J. I., Buonocore, J. J., & von Stackelberg, K. (2010). Evaluation of the public health impacts of traffic congestion: A health risk assessment. Environmental Health: A Global Access Science Source, 9(65). https://doi.org/10.1186/1476-069X-9-65
- Maria-Alexandra, I. (2023). Sustainable Mobility and the Environment: How our transportation choices shape our future. E3S Web of Conferences 436, 11003. (2023) ICED2023. https://doi.org/10.1051/e3sconf/202343611003
- Minister of Transport and Logistics of the Federal Democratic Republic of Ethiopia. (2020). Ethiopia non-motorized transport strategy. 20-29.://unhabitat.org/sites/default/files/2020/07/ethiopia_nmt_strategy_en_200629.pdf
- Mohan Rao, A., & Ramachandra Rao, K. (2012). Measuring urban traffic congestion-A review. International Journal for Traffic and Transport Engineering, 2(4), 286–305. https://doi.org/10.7708/ijtte.2012.2(4).01
- Ndatho, M. N. (2018). Socio-economic effects of traffic congestion on urban mobility along Jogoo road, Nairobi city c-Kenya. http://ir-library.ku.ac.ke/handle/123456789/19938
- Oluwaseyi, A. (2017). Socio-economic impact of road traffic congestion on urban mobility: A case study of Ikeja local government area of Lagos state, Nigeria Pacific Journal of Science and Technology, 18. https://www.researchgate.net/publication/322103771
- Pocock, B., Skinner, N., & Ichii, R. (2009). Work, life workplace flexibility a n d. https://www.unisa.edu.au/siteassets/episerver-6-files/documents/eass/cwl/publications/awali-09_full-for-website.pdf
- Samal, S. R., Gireesh Kumar, P., Cyril Santhosh, J., & Santhakumar, M. (2020). Analysis of traffic congestion impacts of urban road network under Indian condition. IOP Conference Series: Materials Science and Engineering, 1006(1), 012002. https://doi.org/10.1088/1757-899X/1006/1/012002
- Samuel, A., Charles, A., Gift, D., Atinuke, A., Augustus, A., Williams, A., & Adekunle, K. (2023). Public perceptions of vehicular traffic emissions on health risk in Lagos metropolis Nigeria: A critical survey. Heliyon, 9(2023), e15712. https://doi.org/10.1016/j.heliyon.2023.e15712
- Sánchez González, S., Bedoya-Maya, F., & Calatayud, A. (2021). Understanding the effect of traffic congestion on accidents using big data. Sustainability (Switzerland), 13(13), 7500. https://doi.org/10.3390/su13137500
- Sitotaw, S. S., & Tekilu, B. (2019). Assessing the socio-economic impact of road traffic congestion in Addis Ababa city in the case of Megenagna to CMC Michael road segment [Institute of Technology]. http://etd.aau.edu.et/handle/123456789/18412
- Sulaiman, H., & Wahyuni, W. (2023). Traffic congestion effect on socio-economic of road users in Palembang city. ICOMSI 2022, ASSEHR 751, 80–90. https://doi.org/10.2991/978-2-38476-072-5_9
- United Nations Human Settlements Program (UN-HABITAT). (2017). The state of Addis Ababa : The Addis Ababa We Want. UN-Habitat. https://unhabitat.org/sites/default/files/download-manager-files/State%20of%20Addis%20Ababa%202017%20Report-web.pdf
- Vencataya, L., Pudaruth, S., Dirpal, G., & Narain, V. (2018). Assessing the causes & impacts of traffic congestion on the Society, economy, and individual: A case of Mauritius as an emerging economy. Studies in Business and Economics, 13(3), 230–242. https://doi.org/10.2478/sbe-2018-0045
- Wang, C. (2010). The relationship between traffic congestion and road accidents: an econometric approach using GIS [ PhD thesis]. Kingston University of London. https://doi.org/10.13140/RG.2.1.3180.0087
- The World Bank Group. (2014). Cairo congestion study Executive note. https://www.worldbank.org/content/dam/Worldbank/TWB-Executive-Note-Eng.pdf
- Yamane, T. (1967). Statistics, an Introductory Analysis (2nd ed.). Harper and Row.