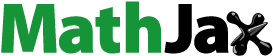
ABSTRACT
Walking and cycling are not just physical activities; they are essential transport modes for students in most rural parts of Africa. Therefore, calling for their promotion without critically examining accessibility in a context where the effect of distance-related land use variables and streets -a major active transport infrastructure - are assessed, leads to a futile agenda in the continent. This study seeks to address this by applying the three-step catchment floating method to assess education/school accessibility through walking and cycling in the Ayawaso East Municipal Assembly (AEMA) and Tamale Metropolitan Assembly (TaMA) in Ghana. Additionally, the differences in accessibility, along with the association of street connectivity and land use variables such as the number and density of schools and catchment areas with education/school accessibility are determined by correlation and Mann–Whitney’s U-tests. The findings revealed a poorly connected dendritic road network system at both locations and shortcomings in school and street network planning that have left some communities vulnerable to low education opportunities. Without policy and planning interventions to address school siting and improve street connectivity, walking and cycling will not only be hindered, but education for some communities, particularly in TaMA may be compromised.
1. Introduction
Accessibility to places and services is vital to peoples’ movement and influences travel choices, emergency access, and, more generally, quality of life (Handy et al., Citation2003). The concept is an important determinant of active transport patronage (Frank et al., Citation2003; Berrigan et al., Citation2010) and it is indispensable where trips are made on foot or by bicycles which depends solely on the users’ energies (Rahul and Verma, Citation2014; Tiwari et al., Citation2016).. Accessibility measures the proximity of people to a point (Stewart, Citation1948) or the ease of reaching destinations (Geurs & van Wee, Citation2004). Accessibility has been hugely associated with street connectivity, and the two have often been used to define the performance of the built environment (Berrigan et al., Citation2010). In transportation, accessibility embodies both land use patterns and street connectivity according to Tal and Handy (Citation2012), who further assert that the difference between accessibility and connectivity is hard to detect, making them mutually exclusive. Street connectivity on the other hand is the primary purpose of transport networks (Dill, Citation2004) and measures the density or quantity of connections between roads and paths (Litman, Citation2012; Tal & Handy, Citation2012). Street connectivity, like accessibility, has the potential to increase trips for all modes (Handy et al., Citation2003), particularly active transport modes. Connectivity does not only increase ease of movement through shorter routes but also improves trips between origin and destination through multiple route choices (Berrigan et al., Citation2010). Walking and cycling could be safer, more efficient, and easier where streets are highly connected (Mecredy et al., Citation2011). The two, accessibility and street connectivity, are therefore important elements of the built environment regarding active transport. Research has therefore sought to look at street connectivity and accessibility concerning active transport across continents (Berrigan et al., Citation2010; Jia et al., Citation2021; Tal & Handy, Citation2012). In some instances, accessibility was referred to as walkability or walk score for walking modes and bikeability for cycling (Carr et al., Citation2010; Forsyth, Citation2015; Molaei et al., Citation2021; Winters et al., Citation2013). The studies determined both accessibility and connectivity together or individually as built environment variables for active transport and acknowledged the bias towards either walking or cycling (Berrigan et al., Citation2010; Saghapour, Citation2017; Tal & Handy, Citation2012) with focus on dedicated infrastructure (Tal & Handy, Citation2012). In Africa, streets which are the principal part of the urban transport system (Stephen, Citation2004) emerge as a preferable infrastructure for studies other than dedicated infrastructure. They function as the main infrastructure for walking and cycling in Africa (I-ce, Citation2000; Timpabi et al., Citation2023) particularly since dedicated infrastructure is woefully inadequate or lacking (Nikitas et al., Citation2021).
Street connectivity is associated with increased active transport. From the review of existing literature, Dill (Citation2004) found that four street connectivity measures including street network density, intersection density, connected node ratio and link-node were positively correlated with active transport. Berrigan et al. (Citation2010) also based on GIS-derived measures and street connectivity in Los Angeles and San Diego Counties, concluded after comparing nine of the street connectivity measures that areas with short blocks and dense nodes which connotes high connectivity were more strongly associated with the propensity to report active transportation (AT) and positively associated with AT duration (Berrigan et al., Citation2010). Jia et al. (Citation2021) from their review of numerous studies on street connectivity and physical activity found mostly a positive association between street connectivity and physical activity, including walking and cycling, which emphasizes the importance of street connectivity to walking and cycling.
Research that sought to determine a relationship between active transport and the built environment in Africa was based primarily on perception (Oyeyemi et al., Citation2013), rather than objective measurement. Oyeyemi et al. (Citation2013) in their evaluation of the Neighbourhood Environment Walkability Scale (NEWS), a questionnaire that measures residents’ perception of their environmental attributes and their relationship with transportation (Cerin et al., Citation2006), found that residents in high-walkable neighbourhood in Nigeria perceived their environmental attribute on residential density, land use mix diversity and street connectivity to be better than residents of the low-walkable neighbourhood. Street connectivity was therefore related to highly walkable environments. Perception studies like that of Oyeyemi et al. (Citation2013) are important to understand the views of people subject to their understanding of connectivity or accessibility, however, for informed decision-making, a more objective assessment with measurements is important. Anciaes et al. (Citation2015), from a more objective approach, sought to map pedestrian accessibility and the quality of walking in Praia, Cape Verde, in Africa. Pedestrian accessibility was determined as the ratio of the number of destination types and the area of man-made land uses in a neighbourhood (Anciaes et al., Citation2015). The study concluded that walkability is highly variable with no disadvantage to any area of the city and social groups (Anciaes et al., Citation2015). The lack of disadvantage is attributable to the lack of consideration distance or cost decay to destination to incorporate a competition factor. The conclusion of the study with a lack of distance or cost consideration contrasts fundamental methods like the gravity method (Stewart, Citation1948) or other recent similar location-based methods like the floating catchment (Chen & Jia, Citation2019; Dumedah et al., Citation2021; Luo & Qi, Citation2009; Wardman et al., Citation2007) for accessibility.
This study therefore sought to measure accessibility by the three-step floating catchment method in determining school or education accessibility by considering walking and cycling other than cars and further identify the relationship of street connectivity and land-use and population variables on the derived accessibility. The three-step floating catchment area method (Wan et al., Citation2012) which is based on the gravity model and an improvement of the floating catchment methods regarding overestimating demand (Chen & Jia, Citation2019; Luo & Qi, Citation2009; Wardman et al., Citation2007) has been used in estimating spatial accessibility to identify healthcare shortage areas. Since the method has efficiently identified shortage areas in healthcare, its use in this study will define the extent of coverage for school accessibility to differentiate bikeable or walkable communities as well as shortage areas regarding school availability. The method measures the spatial access ratio of healthcare within four catchments of a population or destination and defines the catchment perimeters with acceptable or appropriate car travel times. This study will leverage the importance of the views of residents by defining catchment zones based not only on acceptable travel times but also on locally perceived modal and average walking and cycling travel times. The study will conclusively make an objective assessment of the built environment regarding walking and cycling by giving attention to important elements like accessibility, street connectivity, land use and population variables. It will be the first in-depth assessment of the built environment in Africa where active transport is deemed very important due to the huge walking share (Mokitimi & Vanderschuren, Citation2017) and bicycle use in rural areas in Africa (I-ce, Citation2000). It will also determine education instead of healthcare accessibility since schools are important to active transport growth in Ghana with walking and cycling being the main modes for school-related trips per the National Household Transport Survey in 2012 (MRH, MoT, & GSS, Citation2013). Students are potential users and promoters of active transport, especially cycling (Timpabi et al., Citation2021, Citation2023). Despite this good feat, there is a global decline in walking and cycling among young people and school-related trips (Oyeyemi & Larouche, Citation2018; Teixeira et al., Citation2019) which has led to the prevalence of obesity and overweight conditions (Adom et al., Citation2019; Ng et al., Citation2014; Oyeyemi & Larouche, Citation2018) that are associated with non-communicable diseases or Cardio-Vascular Diseases (CVDs) on the rise in Ghana (Kodaman et al., Citation2016). In Ghana, among other factors, the high ownership of vehicles of the medium- and high-income residents, long-distanced schools, and the high exposure of school children to risks associated with commuting by active transport to school along streets has led to the decline in active transport use (Ojo, Citation2018; Setorwofia et al., Citation2020). Parents self-transport their wards to school in cars whilst others depend on school-arranged buses (Agyeman & Cheng, Citation2020). Despite the shift to motorised transport by students, walking and cycling remain important modes for students and young people (Agyeman & Cheng, Citation2020; Mokitimi & Vanderschuren, Citation2017; Timpabi et al., Citation2021). It is therefore important that in addition to the perception studies (Timpabi et al., Citation2021), a determination of the performance of the built environment like streets and land use is made by actual measurement to identify their effect on walking and cycling to make informed policy and planning decision regarding active mode use promotion especially for students.
2. Measurement for connectivity and accessibility for active transport
The gravity model (Stewart, Citation1948) has formed the basis of measurement methods for accessibility, including recent ones like the floating catchment (Chen & Jia, Citation2019; Dumedah et al., Citation2021; Luo & Qi, Citation2009; Wardman et al., Citation2007). The floating catchment area method which has been used in estimating spatial access and identifying healthcare shortage areas does not only consider the distance to healthcare centers from home but also the capacity of the centers to serve a population (Chen & Jia, Citation2019; Luo & Qi, Citation2009; Luo & Whippo, Citation2012; Wan et al., Citation2012). The method works on a demand-and-supply approach, making it more logical and socially useful. The three-step floating catchment has three main equations. EquationEquation 1(1)
(1) in the section below is for Accessibility, denoted as Af, which is the sum product of EquationEquations 2
(2)
(2) and Equation3
(3)
(3) which are selection weight (Gij) and destination-to-demand ratio (Rj).
Like accessibility, street connectivity has numerous primal measurement methods that use various configurations of nodes and links of the streets. The gamma and alpha indices, Centered Node Ratio (CNR), Link-Node Ratio (LNR) and Intersection and Street density methods (Berrigan et al., Citation2010; Dill, Citation2004) use mainly nodes and/or links to determine street connectivity (Berrigan et al., Citation2010; Tal & Handy, Citation2012), block length (Berrigan et al., Citation2010; Cervero & Kockelman, Citation1997), block size (Stangl, Citation2015) and block density (Dill, Citation2004; Frank et al., Citation2000), make use of the block characteristics. Additionally, walking or cycling distances to activity locations (Aultman-Hall et al., Citation1997) and Pedestrian Route directness (PRD), among others, are known methods. The block-related measurements by their style are more applicable to streets with grid patterns, whilst all other methods apply to the tree-like or dendritic street patterns. Street connectivity measures without any inherent or referenced distance element such as CNR and LNR are deemed ineffective methods regarding active transport since the latter is highly distance-influenced (I-ce et al., Citation2008; Rahul and Verma, Citation2014) whilst others like the PRD are deemed to be more complex, making them unpreferable for planning and as a policy-making tool (Dill, Citation2004). Among those easy, useful, and good measurement tools for street connectivity regarding active transport are the street and intersection densities which have inherent distance element as well as the gamma and alpha indices (Berrigan et al., Citation2010; Dill, Citation2004). The gamma index, expressed as 3 * (# nodes −2), is the easiest of the two indices to interpret. It provides a good representation of the overall transport network and applies to bicycling and walking (Dill, Citation2004). The index ranges from 0 to 1, and it is expressed as a percent of the street connectivity where higher values indicate higher connectivity and 1 represents a grid network (Dill, Citation2004). The intersection density is the number of 3 or 4-way nodes or intersections per unit area, and street density is the length of links per unit area. Areas with clustered intersections and more links suggest high connectivity (Berrigan et al., Citation2010) and provide alternative shorter route options. Therefore, for this study, the gamma index was used to determine the street layout type, and intersection and street densities were used to measure street connectivity.
3. Materials and method
3.1. Scope of the study
Two locations were chosen for the measurement of street connectivity and accessibility of schools by walking and cycling, and they included the Tamale Metropolitan Assembly (TaMA) and the Ayawaso East Municipal Assembly (AEMA). The two are very different in terms of land size and have some similitude in ethnicity, settlement type (mostly medium class), religion, socio-economic characteristics (AEMA, Citation2020; Ghana Statistical Service, Citation2014) and reported cycling culture and share (Timpabi et al., Citation2021). The TaMA is about 170 times larger than the size of the AEMA. Whilst AEMA is 3.7 km2 (AEMA, Citation2020) by land size, TaMA is 646.90 km2 (Tamale Metro Assembly, Citation2021). The choice of locations with disparity in land size is intended to expose the planning regime of both micro and macro neighbourhoods. In determining accessibility, the origins of trips were the community centroids whilst the destinations were schools. Students in the context of this study applied to all persons who make school-related trips. Thirty-four (34) schools with an average student population of 392 were identified in AEMA, and they comprised kindergartens, primary schools, Junior High Schools (JHS) and a tertiary institution of both private and public origins (AEMA, Citation2020). In the case of the Tamale Metropolitan Assembly, 228 schools were mapped which comprised kindergartens, primary schools, JHS, Senior High Schools (SHS), Technical/Vocational Schools and a University (Tamale Metropolitan Health Directorate, Citation2011). The average student population per school in TaMA was 398. School enrolment capacity and student population at homes were the supply and demand components used in the accessibility method, respectively.
3.2. Data for accessibility and street connectivity determinations
3.2.1. Land use and spatial data
The study made use of both secondary and primary data. Spatial data (e.g. building and school location, street network) were sourced from Openstreet Map (OSM) since they were not locally available; a challenge noted in Ghana by Dumedah and Eshun (Citation2020). The GPS locations of the list of schools from the local office were mapped. Building data from OSM repositories was cleaned of all destinations, and the rest were assumed to be for homes. It is possible that due to the rapid growth and urbanization in TaMA coupled with sprawling houses over the last few years (Fuseini and Kemp, Citation2016), location data may not reflect the current situation. The AEMA is an already built-up area, and variation in school numbers and locations is therefore not expected. In all, 28,354 and 6207 homes were extracted for TaMA and AEMA, respectively. The distribution of homes and schools at TaMA and AEMA are shown in and . At both locations, there is a relatively low number of schools as compared to homes. The ratio of schools to homes is 1:180 for AEMA and 1:120 for TaMA.
Figure 1. Home and school locations within communities in the TaMA study area (source: developed by authors).

With the assistance of the local people, the study locations were demarcated into communities based on anecdotal geographic boundaries. In all, 30 and 14 communities were carved out for TaMA and AEMA, respectively. The 115 communities in TaMA (Ghana Statistical Service, Citation2014) were agglomerated into 30 communities per guidance from the inhabitants in the absence of existing boundary data. The centroid of the communities denoted as i in the accessibility Equations and shown in and was the reference point for the population or demand.
3.2.2. Trip characteristic data for catchment sub-zoning
Primary data collection was done through questionnaires and observational surveys. The observational survey focused on measuring the speed of pedestrians and cyclists commuting to school or work. In each study location, a 100-m distance was established on the street at two specific locations. Observers documented the time when a pedestrian or cyclist initiated and completed the 100-m distance. The cyclists and pedestrians were post-informed of the exercise to avoid the influence of their awareness of the exercise on their speeds. The data of those who did not consent were excluded from the analysis. In all, 10 pedestrians and 10 cyclists at each of the locations in AEMA were surveyed, whilst in the case of TaMA, 17 pedestrians and 10 cyclists of various ages and gender at each of the 2 locations were obtained arbitrarily and were not related to the questionnaire respondents. The speeds were investigated mainly to determine the equivalent distances in km from the questionnaire survey to better understand the extent of walking or cycling trip distances of respondents. The questionnaire survey enquired about pedestrians’ and cyclists’ trip distances in minutes. All participants were pre-informed of the survey’s purpose and further consented to participate in the survey. In all 638 and 688 valid participants were obtained from AEMA and TaMA respectively out of a sample size of 700 each. The questionnaires were administered through the Kobo Collect app by a simple random sampling approach (Timpabi et al., Citation2023). It sought to determine trip characteristics like purpose, travel mode and travel time per trip and in minutes. The average and modal travel time per walk or cycle trip were used to define mostly the second and third subzones of the catchment for accessibility determination. A 60-minute walking and cycling travel time which represents the acceptable amount of Moderate-to-Vigorous Physical Activity (MVPA) per day recommended for children and adolescents (Bull et al., Citation2020) was used for the extended zone or 60th-minute catchment. The four subzones were therefore defined to be 6 min, 15 min, 30 min, and 60 min distance from the community centroids denoted as i. The 6th-minute zone defined the time equivalence of the acceptable minimum walking distance of 400 m (Dill, Citation2004; Saghapour et al., Citation2016) and the 15th and 30th-minute subzones encompassed the average and modal daily walking or cycling trip distances of respondents who made school-related trips.
3.3. Data analysis
3.3.1. Location/land-use based accessibility
The accessibility of students was generally determined as a Spatial Access Ratio (SPAR) by the three-step catchment floating method (Wan et al., Citation2012). Student population potential at homes and enrolment capacity of schools were the demand and supply respectively, whilst the street network facilitated the creation of the catchments with the ORS tool in QGIS 3.30.3. Enrolment data were sourced from the local government offices. In the absence of household student population data, an estimate was made from the national population data which resulted in 7 and 15 students for each household comprising 12 and 32 members for TaMA and AEMA, respectively (AEMA, Citation2020; Ghana Statistical Service, Citation2014).
Accessibility, in this case for education availability, according to Wan et al. (Citation2012), is expressed in Equation 1 as:
where, Gij is the selection weight between location i and service site j, Dist (i, k) is the travel cost (minutes) from i to any service site k within the catchment, and d0 is the catchment size which is the maximum distance of 60 min. Gij is expressed in EquationEquation (2)(2)
(2) as:
Where Tij and Tik are the assigned Gaussian weights for j and k
Rj is the school enrolment-to-student population ratio of j within the catchment, and it is expressed in EquationEquation (3)(3)
(3) as:
Pk is the student population at catchment k within the catchment of destination, j. Sj is the student enrolment/student capacity within location j. Gkj is the selection weight between j and population site k, and Wr is the distance weight for the rth travel time zone calculated from the Gaussian function. Wr was interpolated and extrapolated from (Wan et al., Citation2012) to reflect the dimension in minutes of catchment zones including 6, 15, 30, and 60 min at their mean distances of 3, 10.5 22.5 and 45 min shown in . Further analysis was carried out with Excel and in QGIS 3.30.3 to develop choropleth maps which showed the levels of education accessibility with walking or cycling.
Table 1. Gaussian weights for subzone based on distance impedance coefficient for walking in TaMA and AEMA.
3.3.2. Street connectivity
To carry out the connectivity analysis, nodes and links were extracted from the street network data and analysed in QGIS 3.30.3 to determine intersection and street densities, and gamma index. All categories and conditions of streets were involved, including unpaved and paved. Intersection and street densities were determined in 1 km-by-1 km grids, and their magnitudes were visualized as a choropleth map. Statistical analysis was undertaken further by correlation and Mann Whitney’s U tests with Excel and Statistical Package for Social Sciences (SPSS).
4. Results and discussion
4.1. General overview of the street network in AEMA and TaMA
From , the gamma index values suggest that the street network pattern at both locations is not grid which has a gamma index of 1 (Dill, Citation2004). The street patterns are dendritic for both AEMA and TaMA.
Table 2. Street connectivity variables for TaMA and AEMA.
4.2. Street connectivity
4.2.1. TaMA
From , almost half of the land space in Tamale (white areas) have no streets. About half of the Tamale metropolis are not connected with streets, and these unconnected areas are mostly rural. There are relatively more grids for street density in than there are for intersection density in which further implies that there are longer streets than there are intersections or junctions to give people more alternative routes. The metropolis has an average street intersection and street density of 31 nodes/km2 and 4 km/km2, respectively. The higher street densities of more than 5 km/km2 are concentrated in the Central Business District (CBD) in Tamale and its adjourning communities.
Beyond the CBD and in most communities located at its outskirts or within the rural part of the metropolis, connectivity is sparse. Movement to and from the CBD from its outskirts may occur through informal, undocumented routes, which are recognized as the most commonly utilized paths in the metropolis (Timpabi et al., Citation2023) or by the closest arterial streets associated with more direct (Winters et al., Citation2010) but unsafe conditions (Damsere-Derry & Bawa, Citation2017; Damsere-Derry et al., Citation2010). Utilitarian pedestrians or cyclists from a community like Tugu, may have to rely on the longer arterial road through the CBD to Moyer. The exposure of pedestrians and cyclists who have low speeds to arterial or major roads that have very high speeds is precedence for unsafe outcomes (Marshall & Garrick, Citation2010), high pedestrian fatalities (Damsere-Derry et al., Citation2019) and longer travel distances that make walking and cycling less attractive. There will therefore be a high inclination towards faster modes as witnessed in TaMA (Damsere-Derry & Bawa, Citation2017; Timpabi et al., Citation2021). The rural and peripheral communities with low or no connectivity have high numbers of utilitarian users of walking and cycling (Acheampong & Siiba, Citation2018; Jennings, Citation2015) due to a lack of affordability for other modes. Low street connectivity could therefore be a contributor to the high latent desire to shift from bicycles in the metropolis (Timpabi et al., Citation2021).
The street network in Tamale Metropolis is about 60% connected at a gamma index value of 0.6. This level of connectivity is attributed to the CBD area in Tamale, and its adjourning town and not metropolitan-wide.
A comparison of both street connectivity measures (street and intersection density) shown in emphasizes the extent of disconnectedness of some existing links. The street layout of TaMA is poorly planned leading to poor connectivity and a source of longer travel time for all modes, especially walking and bicycles. Streets are therefore not direct and cohesive to support active transport, hence the increase in motorization especially motorcycles which are more affordable and faster mode, over the last few years (Acheampong & Siiba, Citation2018; Damsere-Derry & Bawa, Citation2017).
4.2.2. AEMA
The size of AEMA is about 170 times that of TaMA. Its size therefore exposes street planning at a micro-level which is expected to be efficient with better street connectivity indices. The intersection and street densities do not exceed 152 nodes/sq. km and 15 km/sq. km respectively. The maximum street and intersection densities are 11.2 km/sq. km and 110 nodes/sq. km. The Kanda area which is relatively well planned has the highest connectivity by both intersection and street density compared to Nima as shown in . Even though the level of street connectivity in AEMA is higher than that of TaMA, connectivity at the two locations is relatively low. Amsterdam, with a high active transport patronage, has street and intersection densities of 30.7 km/km2 and 314.4, respectively, and Ouagadougou in Burkina Faso, with a high bicycle ownership (Oke et al., Citation2015), has average street and intersection densities of 14.8 km/km2 and 87, respectively (UN-Habitat, Citation2020). The narrative on bicycle usage and ownership in these countries and their street connectivity compared to Ghana’s suggests an influence of street connectivity on active mode usage. In essence, street network connectivity for smaller land areas in Ghana remains as poor as the large ones. The streets in TaMA and AEMA which serve as infrastructure for active transport (I-ce, Citation2000; Timpabi et al., Citation2023) do not support walking and cycling due to their poor planning and connectivity lapses.
Planners and engineers may consider connectivity as a design criterion to enhance street network connectivity which will indirectly address mobility for all modes. Any of the two measurement methods (street density and intersection density) can be used for the street connectivity assessment since both were highly correlated with a Pearson value, r, of 0.892 for TaMA and 0.912 for AEMA. The variables were normally distributed with the least p-value of 0.133 for intersection density for TaMA per the Shapiro–Wilk test (Ahad et al., Citation2011); hence, the correlation test was applied which aimed to establish the association between the two continuous variables at a p-value below 0.05. Consequently, areas exhibiting higher intersection density are likely to demonstrate increased street density, as illustrated in which aligns with the research findings by Berrigan et al. (Citation2010).
4.3. Determining accessibility using SPAR
4.3.1. Setting catchment dimensions
From responses from the questionnaire survey and as shown in , most respondents walked and cycled on average for 10 and 15 min from their homes at TaMA and AEMA, respectively, to schools daily which is consistent with the findings from the 2012 National Transport Survey (MRH, MoT, & GSS, Citation2013). Travel times for those who walk to school are marginally lesser than those who cycle at both locations. Respondents’ average daily walking time from home to school is 19 and 27 min at TaMA and AEMA respectively and are more at an observed walking speed of 4 km/hr compared to 400 m (6 min) for the minimum acceptable walking distance (Dill, Citation2004; Yang & Diez-Roux, Citation2012) and 1 km for most walking trips (Buehler & Pucher, Citation2012). At a recorded speed of 13 km/hr, cyclist respondents’ average travel times of 20 and 27 min which were equivalently 4.3 km and 5.9 km for AEMA and TaMA, respectively, were consistent with known cycling trip distances of 3 km and 5 km (Buehler & Pucher, Citation2012; CROW, Citation2016). The findings reiterate walking and cycling rates in Africa as being high (Green Africa Foundation, & UN-Habitat, Citation2014; Oyeyemi & Larouche, Citation2018)
Table 3. Pedestrian and cyclist’s speeds and trip distances.
Accessibility of schools was determined as a Spatial Access Ratio (SPAR) with the four described zones in each of the communities. The SPAR values at the various impedance levels from 440 to 1050 did not vary as observed by Wan et al. (Citation2012), and subsequent grouping of SPAR was based on the range of their values.
4.3.2. SPAR determination for TaMA and AEMA
From and , it is observed that bicycle catchments are wider which exposes cycling as a more prudent form of active transport in terms of distance coverage. Communities with 0 SPAR, depicted in white, indicate school shortage areas. At these areas, educational facilities or street infrastructure to support the formation of catchment areas are absent. SPAR for the 30 communities in TaMA ranged from 0.00119 to 1.57199 and 0.02309 to 3.674875 with averages of 0.20345 and 0.84198 for cycling and walking, respectively. Even though catchment areas for bicycles are wider, their SPAR values were lower than walking. This is attributed to larger areas having a comparatively higher concentration of students and exacerbating the challenge posed by an already limited number of schools.
Figure 7. Accessibility of students from homes per catchments by walking in TaMA (source: developed by authors).

For an area like Tamale, where schools and street densities are comparatively higher (Ghana Statistical Service, Citation2014), accessibility to school by those who will choose walking is low due to the relatively high student demand. Students may choose to seek schools farther away from the Tamale community centroid. Conversely, students residing at the peripheries of the metropolis, where schools are less available, are likely to walk towards the CBD in pursuit of educational opportunities. The two situations can lead to increased education accessibility in intermediary communities like Zujung, Bamvim Bipela, Kotingli, Aduyeli, and Viteng, as shown in . Students within Tamale and the peripheral areas of the metropolis will potentially be subjected to longer walking distances over 60 min , resulting in round trips for only school purposes exceeding the recommended 60-min daily physical activity levels for children and adolescents (Bull et al., Citation2020). Walking, of the two, will be more tiring and longer due to the associated low speeds hence initiatives like the free Bamboo Bicycle (Whiting, Citation2020) where students cannot afford bicycles can support their trips. Other policies like the reduction of bicycle cost, bike-sharing, and the creation of more schools at the metropolis peripheries and within 30 min or less than 800 m reach of the population as recommended for school siting (Brittin et al., Citation2015; Moussa et al., Citation2017) will reduce school trip distances, promote school attendance, and encourage walking and cycling among students. For cycling, as seen in , apart from Kiong which has a higher accessibility due to its low student demand, the rest of the communities had accessibility below 0.50. Kiong is secluded from the other communities by distance and low road network connectivity and has no student spill-over to and from other communities, leading to a low demand and high education accessibility. With bicycles, education is possible fora larger number of students due to the large catchment area, but the extent of school availability is low because of high demand levels associated with large student population and constantly low school numbers. While there is a reduction in the level of education accessibility for people who cycle, education access coverage is increased for those who choose to cycle.
emphasizes the difference between SPAR for walking as being higher than that for cycling. For most communities at the peripheries of the metropolis, it is mostly not possible for the student population to access schools by bicycle or walking due to long distances, lack of streets to connect catchments with schools or lack of schools generally. School accessibility by walking and cycling is low or zero at these locations identified mostly as rural or peri-urban. This lapse in accessibility is a source of demotivation for school attendance in the absence of alternative mode. The potential for low education rates in these rural areas is unavoidable.
Figure 9. Education accessibility per community in TaMA with peripheral or rural communities denoted with * (source: developed by authors).

Aside the descriptive assessment, the differences between school accessibility by the two mode types and location were determined statistically. Results from the Shapiro–Wilk test (p-value of each variable < 0.001) indicated that the SPAR data for TaMA did not meet the normality test (Ahad et al., Citation2011). The Mann–Whitney U-test, like the independent sample t-test, is used to determine whether a statistically significant difference exists between two groups (MacFarland & Yates, Citation2016). Whilst the Mann–Whitney U-Test is used with nonparametric data, the independent t-test is for data that meet the assumptions associated with parametric distributions including normal distribution (MacFarland & Yates, Citation2016). The Mann–Whitney U-test was therefore employed at a level of significance of 0.05. The evaluation by Mann–Whitney U-Test identified no statistically significant difference between walking (median = 0.4188, n = 60) and cycling (median = 0.1243, n = 60) in TaMA in terms of education accessibility (U = 346.0, p = 0.121, z = 1.552, r (effect size) = 0.20). The test outcome suggests that regardless of the active transport mode type, policy, and planning interventions to improve education accessibility like proportional school siting for those who choose to walk to school may possibly also address accessibility for those who choose cycling and vice versa.
In the case of AEMA, the catchment for the 15th-min to the 60-min zones for both cycling and walking extended beyond the boundary of the municipality across all 14 communities. Students may most likely make school trips outside the municipality if they choose to walk or cycle for more than 30 min. There are no school shortage areas in AEMA. Even though SPAR was generally lower for both cycling and walking in AEMA than TaMA as shown in , like TaMA, those who walked had more school availability than those who cycled. The highest, average, and least SPAR values for cycling in AEMA are 0.08247, 0.0676, and 0.05510, respectively, and that for walking are 0.12263, 0.05498, and 0.02612, respectively. The highest for cycling and walking occurred within the Jubilee House and Kaukudi communities, respectively, which are locations within the Kanda part of the municipality where the general population or school demand which is a denominator for accessibility is low compared to Nima.
Figure 10. Accessibility of students for all 14 communities in AEMA by either walking or cycling (source: developed by authors).

Across the communities in AEMA as observed in and unlike the observation in TaMA, SPAR for walking was generally lower than cycling except in the case of Kaukudi.
Figure 11. Education accessibility levels per community in AEMA with outskirt communities denoted with * (source: developed by authors).

The Mann–Whitney U-test was similarly used to verify the difference between school accessibility for students who will choose to walk against those who will cycle in AEMA. The data deviates from normal distribution since the maximum p-value is 0.007 per the Shapiro–Wilk test (Ahad et al., Citation2011), making them non-parametric. The Mann–Whitney U-test revealed a statistically significant difference in school accessibility for students who will choose cycling (median = 0.0669, n = 14) and those who will walk (median = 0.05498, n = 14), U = 54, z = 2.022, p = 0.043, r = 0.38. The effect size, r, which is less than 0.20, between 0.02 and 0.50, and more than 0.50 were considered benchmarks for small, medium, and large effect sizes, respectively (Cohen, Citation1969). Therefore, whilst accessibility with cycling remains high, the effect size of the difference is medium. This makes a case for the promotion of bicycle use to school by students in AEMA to improve overall education/school accessibility and subsequently potentially improve school attendance.
4.4. Influence of location on accessibility
The study also sought to determine whether there is a difference in accessibility at the two locations (AEMA and TaMA). The Mann–Whitney U-Test was used for the determination since the data is non-parametric. Despite their median differences, there was no statistically significant difference between accessibility for students who choose walking in AEMA (median = 0.05341, n = 14) and TaMA (median = 0.4188, n = 30), U = 168, z = 1.065, p = 0.287, r = 0.16. It can therefore be implied per this study context that, in addition to the identifiable ethnic, religious, and other similarities between the two locations (AEMA, Citation2020), education accessibility for those who choose walking may be counted as one such similarity. Policies and planning to retrofit the built environment and land use for walking in AEMA can be replicated for TaMA and vice versa. For cycling, a statistically significant difference was found between accessibility (SPAR) at TaMA (median = 0.1243, n = 30) and AEMA (median = 0.20345, n = 14), U = 125, p < 0.003, z = 2.123. The median education accessibility for students who will choose to cycle is higher in AEMA than it is in TaMA and the effect of the difference is medium at r = 0.32. Even though TaMA has a huge cycling potential, access to education/school by cycling is low compared to AEMA. The poor spatial distribution of destinations (schools) contributes to the low accessibility in TaMA, coupled with the high student demand and longer commuting distances observed in view of the large area. The latter is already a major source of the shift to faster and unsustainable modes like motorcycles observed over the years (Damsere-Derry & Bawa, Citation2017; Timpabi et al., Citation2021).
At a micro-level where land sizes are smaller as seen in the case of AEMA and more effective planning can be undertaken, the outlook on accessibility is poor which emphasizes the notable lack of or poor planning in Ghana (Amoako & Boamah, Citation2015). The urban areas are left with low accessibility due to high demand; the peri-urban areas are either enjoying or suffering from the spill-over effect of inefficiencies in planning and land use location, whilst the peripheral and rural areas have scarcity or shortages in street network and schools which pose a huge challenge to education.
4.5. Relationship between accessibility, street connectivity and land-use variables
The association between land use variables, population variables, street connectivity (intersection density (ID)) and accessibility of students to school was further determined by correlation (r) at a confidence interval of 95% as shown in . The land use variables included the number of schools (SNo), number of homes (HNo), Area of catchment (AC) and school density (SD). The population variables included student density (StD) and school-to-student ratio per community (SStC). For AEMA, the catchments extended beyond the municipal boundary resulting in similar values among each of the variables except accessibility. Correlation coefficients were therefore not defined. Education accessibility with walking and cycling as transport modes in TaMA was jointly tested given their earlier established statistical equivalence. Education or school accessibility in TaMA was correlated with catchment area, number of schools, number of homes and student density. The association was, however, weak and negative. This suggests that areas with wider catchment areas may have less accessibility regarding schools and explains the low accessibility associated with bicycle modes. The number of homes, students and all other factors related to student population have reducing effects on accessibility hence their negative relationship. The high student population inundates the potential positive correlation between more schools in a location like Tamale community, and accessibility. Proportionally distributing schools aside other drivers like school quality could diminish the effect of population demand on accessibility and reduce the tendency of students making longer trips to schools due to high demand within densely populated areas.
Table 4. Correlation test on accessibility, street connectivity and other land-use elements.
Street connectivity, on the other hand, was highly associated with all the variables except accessibility. Schools and homes (students) may have the tendency to cluster where street intersections are denser or more cohesive as seen in the case of the urbanised communities with more intersection densities like Tamale in TaMA. In addition to the variables associated with viability of destination locations including proximity and density, among others (Yenisetty & Bahadure, Citation2020), the availability of street networks can be one of such. Street connectivity measure is an important criterion to be included in spatial designs by planners and engineers. High street connectivity will not only provide alternative routes which may reduce the relatively longer distances people travel to school by walking and cycling but also encourage the use of the two modes among students (Brittin et al., Citation2016) and improve school-going for those mostly in the peripheral areas of TaMA where accessibility and faster mode affordability is low.
5. Conclusions, recommendations, future research
The study was undertaken in AEMA and TaMA to determine school accessibility by walking and cycling modes and its association with street connectivity and land use elements. Street networks were found to be more dendritic than gridiron at both locations with average street and intersection densities being low at 7.7 km/km2 and 54 nodes/km2 for AEMA and 4 km/km2 and 31 nodes/km2 for TaMA. Especially in the peripheral areas of the metropolis which cover almost half of the land space, streets are either absent or sparsely connected. Education accessibility for students who may choose to walk, or cycle was below 3.68 and 0.123 for TaMA and AEMA respectively and equally low. Whilst no difference was found for accessibility in the two locations with regard to walking, AEMA was found to have high accessibility when it comes to using bicycles to go to school which presents a good opportunity to encourage and promote the use of bicycles through policies such as reduced cost or free bicycles to increase education availability to a greater number of students. On the other hand, the lack of development in roads and poor land use planning for the rural and peripheral areas of the Tamale metropolis is a potential cause of the low education accessibility or the lack of school availability for active transport users especially those who walk. The rural areas are the locations with high captive active transport users. The longer travel time to school, coupled with the poorly connected roads, will hugely disincentivize school attendance which has the potential to impact education at these locations. Aside the known social, demographic and economic factors that hinder walking and cycling and school attendance, the built environment including street connectivity, school numbers, siting and distribution and distance surely has a role to play in education accessibility and inclusiveness as well as active transport usage.
Street connectivity should be an important criterion for engineers and planners to consider incorporating into street design to enhance accessibility for all, including active transport users. Policy interventions on school siting and distribution are recommended. To enhance the coverage of school for students, policies such as the reduction of the cost of bicycles or free bicycles could be introduced by government and other stakeholders for students. These interventions have the potential to improve school availability, travel time, accessibility, and incentivize students to go to school thereby reducing the potential high illiteracy prevalence in the rural or lowly accessible areas and increasing walking and cycling as a sustainable mode.
The study was based on location-based accessibility and did not consider in its determination temporal and environmental factors such as the effect and condition of dedicated infrastructure and other external frictions like topography and alternative available modes and their costs among others known to influence accessibility. The study assumed a simplified approach to make the adoption of the measurement methods easier for planners and other policymakers. Future studies that desire to make a more economical or in-depth measurement may look at other external frictions which are important to the estimation of accessibility and connectivity and further determine the influence of these on accessibility.
Disclosure statement
No potential conflict of interest was reported by the author(s).
Correction Statement
This article has been corrected with minor changes. These changes do not impact the academic content of the article.
Additional information
Funding
References
- Acheampong, R. A., & Siiba, A. (2018). Examining the determinants of utility bicycling using a socio-ecological framework: An exploratory study of the tamale metropolis in Northern Ghana. Journal of Transport Geography, 69(March), 1–24. https://doi.org/10.1016/j.jtrangeo.2018.04.004
- Adom, T., De Villiers, A., Puoane, T., & Kengne, A. P. (2019). Prevalence and correlates of overweight and obesity among school children in an urban district in Ghana. BMC Obesity, 6(1), 1–11. https://doi.org/10.1186/s40608-019-0234-8
- AEMA. (2020). PROFILE.
- Agyeman, S., & Cheng, L. (2020). Analysis of barriers to perceived service quality in Ghana: Students’ perspectives on bus mobility attributes. Transport Policy, 99, 63–85. https://doi.org/10.1016/J.TRANPOL.2020.08.015
- Ahad, N. A., Yin, T. S., Othman, A. R., & Yaacob, C. R. (2011). Sensitivity of normality tests to non-normal data. Sains Malaysiana, 40(6), 637–641.
- Amoako, C., & Frimpong Boamah, E. (2015). The three-dimensional causes of flooding in Accra, Ghana. International Journal of Urban Sustainable Development, 7(1), 109–129.
- Anciaes, P., Nascimento, J., & Silva, S. (2015). Mapping pedestrian accessibility and the quality of walking in an African city: Praia, Cape Verde.
- Aultman-Hall, L., Roorda, M., & Baetz, B. W. (1997). Using GIS for evaluation of neighborhood pedestrian accessibility. Journal of Urban Planning and Development, 123(1), 10–17. https://doi.org/10.1061/(ASCE)0733-9488(1997)123:1(10)
- Berrigan, D., Pickle, L. W., & Dill, J. (2010). Associations between street connectivity and active transportation. International Journal of Health Geographics, 9(1), 1–18. https://doi.org/10.1186/1476-072X-9-20
- Brittin, J., Sorensen, D., Trowbridge, M., Lee, K. K., Breithecker, D., Frerichs, L., & Huang, T. (2015). Physical activity design guidelines for school architecture. PloS ONE, 10(7), e0132597. https://doi.org/10.1371/journal.pone.0132597
- Buehler, R., & Pucher, J. (2012). Walking and cycling in Western Europe and the United States. Transportation Research News, 280(May–June), 34–42.
- Bull, F. C., Al-Ansari, S. S., Biddle, S., Borodulin, K., Buman, M. P., Cardon, G. , & Willumsen, J. F. (2020). World Health Organization 2020 guidelines on physical activity and sedentary behaviour. British Journal of Sports Medicine, 54(24), 1451–1462. https://doi.org/10.1136/BJSPORTS-2020-102955
- Carr, L. J., Dunsiger, S. I., & Marcus, B. H. (2010). Walk Score™ as a global estimate of neighborhood walkability. American Journal of Preventive Medicine, 39(5), 460–463. https://doi.org/10.1016/J.AMEPRE.2010.07.007
- Cerin, E., Saelens, B. E., Sallis, J. F., & Frank, L. D. (2006). Neighborhood environment walkability scale: Validity and development of a short form. Medicine & Science in Sports & Exercise, 38(9), 1682–1691. https://doi.org/10.1249/01.mss.0000227639.83607.4d
- Cervero, R., & Kockelman, K. (1997). Travel demand and the 3Ds: Density, diversity, and design. Transportation Research, Part D: Transport & Environment, 2(3), 199–219. https://doi.org/10.1016/S1361-9209(97)00009-6
- Chen, X., & Jia, P. (2019). A comparative analysis of accessibility measures by the two-step floating catchment area (2SFCA) method. International Journal of Geographical Information Science, 33(9), 1739–1758. https://doi.org/10.1080/13658816.2019.1591415
- Cohen, J. (1969). Statistical power analysis for the behavioural sciences. Academic Press.
- CROW. (2016). Design Manual for Bicycle Traffic. https://crowplatform.com/product/design-manual-for-bicycle-traffic/
- Damsere-Derry, J., & Bawa, S. (2017). Bicyclists’ accident pattern in Northern Ghana. IATSS Research. https://doi.org/10.1016/j.iatssr.2017.10.002
- Damsere-Derry, J., Ebel, B. E., Mock, C. N., Afukaar, F., & Donkor, P. (2010). Pedestrians’ injury patterns in Ghana. Accident Analysis and Prevention, 42(4), 1080–1088. https://doi.org/10.1016/j.aap.2009.12.016
- Damsere-Derry, J., Ebel, B. E., Mock, C. N., Afukaar, F., Donkor, P., & Kalowole, T. O. (2019). Evaluation of the effectiveness of traffic calming measures on vehicle speeds and pedestrian injury severity in Ghana. Traffic Injury Prevention, 20(3), 336–342. https://doi.org/10.1080/15389588.2019.1581925
- Dill, J. (2004). Measuring network connectivity for bicycling and walking. 83rd annual meeting of the Transportation Research Board, Washington, DC, 2003 July 9, Leuven, Belgium, (pp. 11–15) .
- Dumedah, G., Arthur, J., Kokroko, J. S., Ampofo, S. T., Okyere, P. A., & Tweneboah Kodua, E. (2021). Characterizing the geography of potential healthcare accessibility in informal settlements in the Greater Kumasi area of Ghana. African Geographical Review, 42(1), 46–71. https://doi.org/10.1080/19376812.2021.1991811
- Dumedah, G., & Eshun, G. (2020). The case of paratransit-‘Trotro’service data as a credible location addressing of road networks in Ghana. Journal of Transport Geography, 84, 102688.
- Forsyth, A. (2015). What is a walkable place? The walkability debate in urban design. Urban Design International, 20(4), 274–292. https://doi.org/10.1057/udi.2015.22
- Frank, L., Engelke, P., & Schmid, T. (2003). Health and community design: The impact of the built environment on physical activity. Island Press.
- Frank, L. D., Stone, B., & Bachman, W. (2000). Linking land use with household vehicle emissions in the central puget sound: Methodological framework and findings. Transportation Research, Part D: Transport & Environment, 5(3), 173–196. https://doi.org/10.1016/S1361-9209(99)00032-2
- Fuseini, I., & Kemp, J. (2016). Characterising urban growth in tamale, Ghana: An analysis of urban governance response in infrastructure and service provision. Habitat International, 56, 109–123. https://doi.org/10.1016/j.habitatint.2016.05.002
- Geurs, K. T., & van Wee, B. (2004). Accessibility evaluation of land-use and transport strategies: Review and research directions. Journal of Transport Geography, 12(2), 127–140. https://doi.org/10.1016/j.jtrangeo.2003.10.005
- Ghana Statistical Service. (2014). Tamale Metropolis. www.statsghana.gov.gh.
- Green Africa Foundation, & UN-Habitat. (2014). Bicycle use study and analysis report for Nairobi business district. http://www.greenafricafoundation.org/publications/Bicycle%20use%20survey%20within%20Nairobi%20BD%20Report.pdf
- Handy, S., Paterson, R. G., & Butler, K. (2003). Planning for street connectivity: Getting from here to there.
- I-ce. (2000). The significance of non motorised transport for developing countries: Strategies for policy development. 136 p. http://siteresources.worldbank.org/INTURBANTRANSPORT/Resources/non_motor_i-ce.pdf
- I-Ce, T. G., Arora, A., & Jain, H. (2008). Bicycling in Asia. October.
- Jennings, G. (2015). A bicycling renaissance in South Africa? Policies, programmes & trends in Cape. Satc 2015, 27, 486–498.
- Jia, P., Zou, Y., Wu, Z., Zhang, D., Wu, T., Smith, M., & Xiao, Q. (2021). Street connectivity, physical activity, and childhood obesity: A systematic review and meta‐analysis. Obesity Reviews, 22(S1), e12943. https://doi.org/10.1111/obr.12943
- Kodaman, N., Aldrich, M. C., Sobota, R., Asselbergs, F. W., Poku, K. A., Brown, N. J., Moore, J. H., Williams, S. M., & Tayo, B. O. (2016). Cardiovascular disease risk factors in Ghana during the rural-to-urban transition: A cross-sectional study. PLOS ONE, 11(10), e0162753. https://doi.org/10.1371/JOURNAL.PONE.0162753
- Litman, T. (2012). Evaluating accessibility for transportation planning: Measuring People’s ability to reach desired goods and activities. Transportation Research, 62.
- Luo, W., & Qi, Y. (2009). An enhanced two-step floating catchment area (E2SFCA) method for measuring spatial accessibility to primary care physicians. Health and Place, 15(4), 1100–1107. https://doi.org/10.1016/j.healthplace.2009.06.002
- Luo, W., & Whippo, T. (2012). Variable catchment sizes for the two-step floating catchment area (2SFCA) method. Health and Place, 18(4), 789–795. https://doi.org/10.1016/j.healthplace.2012.04.002
- MacFarland, T. W., Yates, J. M., MacFarland, T. W., & Yates, J. M. (2016). Mann–whitney u test. Introduction to nonparametric statistics for the biological sciences using R. 103–132.
- Marshall, W. E., & Garrick, N. W. (2010). Street network types and road safety: A study of 24 California cities. Urban Design International, 15(3), 133–147. https://doi.org/10.1057/udi.2009.31
- Mecredy, G., Pickett, W., & Janssen, I. (2011). Street connectivity is negatively associated with physical activity in Canadian youth. International Journal of Environmental Research and Public Health, 8(8), 3333–3350. https://doi.org/10.3390/ijerph8083333
- Mokitimi, M. M., & Vanderschuren, M. (2017). The significance of non-motorised transport interventions in South Africa – a rural and local municipality focus. Transportation Research Procedia, 25, 4798–4821. https://doi.org/10.1016/J.TRPRO.2017.05.491
- Molaei, P., Tang, L., & Hardie, M. (2021). Measuring walkability with street connectivity and physical activity: A case study in Iran. World 2021, 2(1), 49–61. https://doi.org/10.3390/WORLD2010004
- Moussa, M., Abou Elwafa, A., & Elwafa, A. A. (2017). School site selection process. Procedia Environmental Sciences, 37, 282–293. https://doi.org/10.1016/j.proenv.2017.03.059
- MRH, MoT, & GSS. (2013). REpublic of Ghana second national household transport survey repoRT 2012. chrome-extension: //efaidnbmnnnibpcajpcglclefindmkaj/https://www2.statsghana.gov.gh/docfiles/publications/Second%20National%20Household%20Transport%20Survey%20Report%202012.pdf
- Ng, M., Fleming, T., Robinson, M., Thomson, B., Graetz, N., Margono, C., Mullany, E. C., Biryukov, S., Abbafati, C., Abera, S. F., Abraham, J. P., Abu-Rmeileh, N. M. E., Achoki, T., Albuhairan, F. S., Alemu, Z. A., Alfonso, R., Ali, M. K., Ali, R., Guzman, N. A. … Gakidou, E. (2014). Global, regional, and national prevalence of overweight and obesity in children and adults during 1980–2013: A systematic analysis for the global burden of disease study 2013. The Lancet, 384(9945), 766–781. https://doi.org/10.1016/S0140-6736(14)60460-8
- Nikitas, A., Tsigdinos, S., Karolemeas, C., Kourmpa, E., & Bakogiannis, E. (2021). Cycling in the era of covid-19: Lessons learnt and best practice policy recommendations for a more bike-centric future. Sustainability, 13(9), 4620. https://doi.org/10.3390/su13094620
- Ojo, T. K. (2018). Seat belt and child restraint use in a developing country metropolitan city. Accident Analysis & Prevention, 113, 325–329. https://doi.org/10.1016/J.AAP.2018.02.008
- Oke, O., Bhalla, K., Love, D. C., & Siddiqui, S. (2015). Tracking global bicycle ownership patterns. Journal of Transport & Health, 2(4), 490–501. https://doi.org/10.1016/j.jth.2015.08.006
- Oyeyemi, A. L., & Larouche, R. (2018). Prevalence and correlates of active transportation in developing countries. In Children’s active transportation (pp. 173–191). Elsevier. https://doi.org/10.1016/B978-0-12-811931-0.00012-0
- Oyeyemi, A. L., Sallis, J. F., Deforche, B., Oyeyemi, A. Y., De Bourdeaudhuij, I., & Van Dyck, D. (2013). Evaluation of the neighborhood environment walkability scale in Nigeria. International Journal of Health Geographics, 12(1), 16. https://doi.org/10.1186/1476-072X-12-16
- Rahul, T. M., & Verma, A., (2014). A study of acceptable trip distances using walking and cycling in Bangalore. Journal of Transport Geography, 38, 106–113. https://doi.org/10.1016/j.jtrangeo.2014.05.011
- Saghapour, T. (2017). Accessible neighbourhoods: Towards active transportation [Doctoral dissertation]. (RMIT University .
- Saghapour, T., Moridpour, S., & Thompson, R. G. (2016). Public transport accessibility in metropolitan areas: A new approach incorporating population density. Journal of Transport Geography, 54, 273–285. https://doi.org/10.1016/j.jtrangeo.2016.06.019
- Setorwofia, A. E., Emmanuel, J., Otoo, N., Arko, E. A., Adjakloe, Y. A., & Ojo, T. K. (2020). Self-reported pedestrian knowledge of safety by school children in cape coast metropolis, Ghana. Urban, Planning and Transport Research, 8(1), 158–170. https://doi.org/10.1080/21650020.2020.1758203
- Stangl, P. (2015). Block size-based measures of street connectivity: A critical assessment and new approach. Urban Design International, 20(1), 44–55. https://doi.org/10.1057/udi.2013.36
- Stephen, M. (2004). Streets and Patterns - Stephen Marshall - Google Books. https://books.google.com.gh/books?hl=en&lr=&id=6rBFKX3MmcgC&oi=fnd&pg=PR3&dq=marshall+2005+street+patterns&ots=1FZ7pkJ26D&sig=4f7R_GEc4yBJR5LZtIdUSSK2E6Y&redir_esc=y#v=onepage&q=marshall2005 street patterns&f=false
- Stewart, J. Q. (1948). Demographic gravitation: Evidence and applications. Sociometry, 11(1/2), 31–58. https://doi.org/10.2307/2785468
- Tal, G., & Handy, S. (2012). Measuring nonmotorized accessibility and connectivity in a Robust Pedestrian Network. Transportation Research Record: Journal of the Transportation Research Board, 2299(1), 48–56. https://doi.org/10.3141/2299-06
- Tamale Metro Assembly. (2021). Tamale Metro Assembly – Tamale Metro Assembly. https://tamalemetro.gov.gh/#
- Tamale Metropolitan Health Directorate. (2011). Metropolitan development Tamale metropolitan Report 2011 (Issue January).
- Teixeira, J. F., Silva, C., & Neves, J. V. (2019). School mobility management case study: German School of Oporto (Deutsche Schule zu Porto). Case Studies on Transport Policy, 7(1), 13–21. https://doi.org/10.1016/J.CSTP.2018.11.002
- Timpabi, A. P., Anum, C., Kwame, A., Osei, K., Adams, C. A., & Kwakwa Osei, K. (2023). The role of infrastructure and route type choices for walking and cycling in some cities in Ghana. Urban, Planning and Transport Research, 11(1). https://doi.org/10.1080/21650020.2023.2222838
- Timpabi, A. P., Osei, K. K., & Adams, C. A. (2021). Bicycle ownership and utilization in Tamale metropolis; influencing factors and impacts to sustainable transport. Heliyon, 7(6), e07133. https://doi.org/10.1016/J.HELIYON.2021.E07133
- Tiwari, G., Jain, D., & Ramachandra Rao, K. (2016). Impact of public transport and non-motorized transport infrastructure on travel mode shares, energy, emissions and safety: Case of Indian cities. Transportation Research, Part D: Transport & Environment, 44, 277–291. https://doi.org/10.1016/j.trd.2015.11.004
- UN-Habitat. (2020). Proportion of land allocated to street street density and intersection density 2013 | proportion of land allocated to street street density and intersection density 2013 | Urban Indicators database. https://data.unhabitat.org/datasets/5755782d19584514a5a8c65df908a0ca/explore
- Wan, N., Zou, B., & Sternberg, T. (2012). A three-step floating catchment area method for analyzing spatial access to health services. International Journal of Geographical Information Science, 26(6), 1073–1089. https://doi.org/10.1080/13658816.2011.624987
- Wardman, M., Lythgoe, W., & Whelan, G. (2007). Rail passenger demand forecasting: Cross-sectional models revisited. Research in Transportation Economics, 20, 119–152. https://doi.org/10.1016/S0739-8859(07)20005-8
- Whiting, K. (2020). Ghana’s bamboo bikes are making cycling more sustainable | world economic forum. https://www.weforum.org/agenda/2020/07/ghana-bamboo-bike-cycling-sustainability
- Winters, M., Brauer, M., Setton, E. M., & Teschke, K. (2013). Mapping bikeability: a spatial tool to support sustainable travel. Environment and Planning B: Planning and Design, 40(5), 865–883. https://doi.org/10.1068/b38185
- Winters, M., Teschke, K., Grant, M., Setton, E. M., & Brauer, M. (2010). How far out of the way will we travel? Built environment influences on route selection for bicycle and car travel. Transportation Research Record, 2190(1), 1–10. https://doi.org/10.3141/2190-01
- Yang, Y., & Diez-Roux, A. V. (2012). Walking distance by trip purpose and population subgroups. American Journal of Preventive Medicine, 43(1), 11–19. https://doi.org/10.1016/J.AMEPRE.2012.03.015
- Yenisetty, P., & Bahadure, P. (2020). Measuring accessibility to various ASFs from Public Transit using spatial distance measures in Indian cities. ISPRS International Journal of Geo-Information, 9(7), 446. https://doi.org/10.3390/ijgi9070446