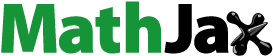
ABSTRACT
Contemporary cargo vessel transport constitutes a vital part of the global trade and economy. Thus, it is of primary engineering and design importance to further develop more efficient novel risk analysis and risk assessment methods for large cargo ships. Classical risk assessment and risk analysis methodsmay not always have advantages of efficiently dealing with dynamic system’s high dimensionality along with cross-correlations between various critical dimensions. Current study benchmarks state-of-art Gaidai spatiotemporal structural risk assessment method, suitable for multivariate structural dynamic systems versus a well-established bivariate risk assessment method. As an example, for this risk assessment study, an operating container vessel has been selected. The vessel hull had been subjected to large deck panel stresses and accelerations during cross-Atlantic sailing. Risks of losing cargo containers are caused by extreme dynamics being one of the primary concerns for vessel cargo transport. Gaidai multivariate risk assessment methodology, advocated here, opens up new possibilities of predicting efficiently, yet simply potential structural damage/failure risks for nonstationary, nonlinear, multivariate cargo ship dynamic systems, as a whole. Note that advocated novel multivariate risk assessment method may be applied to a wide range of complex marine and offshore engineering systems.
1. Introduction
Extreme value predictions problems in contemporary engineering being challenging and frequent, especially in case when measured data are scarce (Abioye et al., Citation2021; Chen et al., Citation2021; Drummen et al., Citation2008; Dulebenets, Citation2022; Elmi et al., Citation2022; European Maritime Safety Agency EMSA, Citation2021; ISSC, Citation2009; Jovanović et al., Citation2022; Parunov et al., ; Y. Wang & Wang, Citation2021). Thus, from practical design standpoint, it is essential to develop novel, effective, accurate risk assessment approaches. As container vessels play undeniably significant role within commercial marine transport industry, this study primarily has been concerned with cargo vessel’s safety and risk assessment. MSC Napoli, post-Panamax container vessel, had experienced engine room bulkhead’s damage in Jan 2007. Evaluations of MSC Napoli’s hull girder risk and risk assessment at the time of the accident have been presented by (Parunov et al.,). Hull girder ultimate strength was representative for vessel’s structural capacity; loads included still water vessel hull bending moments, vertical wave-induced bending moments, whipping-induced bending moments. Load combinations of wave bending moments and whipping have been taken into account, first-order risk assessment approach had been utilized to assess failure risks (Parunov et al.,). Another post-Panamax container vessel, MOL Comfort, had been damaged in Jun 2013 (Bureau Veritas, Citation2012; Ellermann, Citation2008; Falzarano et al., Citation2012; Gaidai, Yakimov, et al., Citation2023; Rahim, Citation2019; Su, Citation2012). Both cargo vessels have been damaged due to overloading of the main deck panel’s girder, resulting in a decreased collapse strength, compared to other comparable vessels. These significant failure events, involving cargo ships, have seriously rattled maritime industry, calling for thorough investigations. Next, one may mention human-related error risk assessment aspects, typical within maritime cargo transportation industry (Bicen et al., Citation2021). A wide range of intricate factors, such as insufficient experience, training, system monitoring, poor communication, or a failure to draw lessons from previous mistakes, can lead to human error.
This study’s major research object has been 4400 TEU (Twenty-foot Equivalent Unit) Panamax container vessel, 245-meter long (ISSC, Citation2009). Motion sensors have been installed on this 4400 TEU Panamax container vessel, during trans-Atlantic voyages during 2010–2020 years (Abioye et al., Citation2021; Drummen et al., Citation2008; Dulebenets, Citation2022; Elmi et al., Citation2022). Captain had made additional measures, in order to avoid worst incoming storms. 4400 TEU dynamic vessel panel stress sensor’s placements had been carried out following latest DNV (Det Norske Veritas) container vessel’s safety regulations and standards (Chen et al., Citation2021; Ellermann, Citation2008; European Maritime Safety Agency EMSA, Citation2021; Falzarano et al., Citation2012; Jovanović et al., Citation2022; Parunov et al., ; Rahim, Citation2019; Su, Citation2012). On-board data’s sampling time-step has been s, monitored continuously through complete voyage’s duration. For more details on vessel’s parameters, see e.g. (Bicen et al., Citation2021; Bureau Veritas, Citation2012; DNV-RP-H103, Citation2011; Gaidai, Yakimov, et al., Citation2023). On-board measured dataset had not been post-processed/filtered, hence raw dataset had been analyzed; all available voyage-datasets have been coalesced into a single 1D (1-Dimensional), jointly representative timeseries. Evaluating extreme vessel accelerations and deck panel’s stresses along with associated low risks/probabilities being challenging task, only limited progress had been made within the past decade (Andersen & Jensen, Citation2014; Gaidai et al., Citation2010a; Gaidai, Yakimov, et al., Citation2023; Storhaug & Moe, Citation2007; Sun, Gaidai, Xing, et al., Citation2023; Tomic et al., Citation2018). Numerical MC (Monte Carlo) simulations, being based on underlying model’s assumptions, often being unable to capture all necessary wave-induced accelerations and vessel deck panel’s stresses.
When assessing design loads given current vessel regulations, vessel hulls being mostly modelled as rigid. Cargo vessels being in fact flexible, with waves causing their structural hull vibrations. Environmental loads, along with accumulated fatigue damage, are being exacerbated due to vessel hull vibrations. Full-scale measurements for Capesize bulk type carriers have provided evidences of key risk contributions, but impacts on container overall’s overall risk assessment had not been yet comprehensively studied. 294-m-long cargo vessel sailing in North Atlantic had been used in this study in order to assess areal deck panel stresses, along with vessel’s accelerations. Wave radar along with wind data had been utilized to complement stress’s records amidships, those measurements have been evaluated aboard, in real time, thanks to hull onboard monitoring system, giving captain updated live information. Axial forces, warping, horizontal as well as vertical bending moments all are contributing to increased stress levels, along with fatigue damage. Differences between overall fatigue damage, compared to wave frequency-related damage being typically utilized to compute fatigue damage, caused by wave-induced hull vibrations. Wave-induced hull vibrational effects are depending on vessel’s size, its eigen-periods, built materials, initial cracks. To decrease maintenance’s downtime throughout whole course of ship’s service lifetime, wave-induced vibrational effects have to be taken into account at the design verification stage for any large cargo ship. On-board measured dataset being vital to correctly assess structural vessel’s risk assessment, as well as calibrate software numerical tools, contribute to vessel design rules/regulations.
Constructed in the year 2003, 4400 TEU vessel has followed a contemporary design. DNV has assessed on-board stresses and acceleration records from a 4400 TEU container vessel having comparable size, age as a part of AOG (Active Operator Guidance) DNV research project. Full-scale type measurements, recorded in North Pacific exhibited contribution up to 50% depending on season, in contrast to about 35% according to head sea model tests (Drummen et al., Citation2008), .
Figure 2. Sensor, being glued to the deck panel’s stiffener web (Storhaug & Moe, Citation2007).

4400 TEU vessel full scale on-board measurements, along with wave-induced vibrational analysis tools have been provided by the DNV.
Table 1. 4400 TEU vessel main particulars.
presents 4400 TEU cargo vessel, exploited in a trade between Germany, UK, France and Canada. presents brief summary of vessel’s essentials. Vessel received notations: +1A1, EO, Container Carrier, NAUTICUS (New-building), W1-OC, ICE-C, DG-P, constructed according to DNV class. Vessel had typical cross-sectional shape, however running with somewhat lower draft, compared to other ships of one’s size. HT32 steel was utilized, with HT36 above top deck, hatch coaming plating was 65 mm in thickness, shear strake along with top deck were built of 60 mm thick steel plates. Sensors SW, IS, indicated in have been intended to be placed within stiffener web’s neutral axis, at stiffener’s momentary zero point, to monitor bending caused by lateral pressures.
In the next Section, state-of-art Gaidai multivariate risk assessment methodology to be introduced in some detail, following by practical application of Gaidai multivariate risk assessment method to 4400 TEU cargo ship’s on-board measured data.
2. Gaidai multivariate risk assessment methodology
Assessing structural vessel system’s safety, utilizing conventional design/engineering risk assessment methods being often quite challenging (Abioye et al., Citation2021; Chen et al., Citation2021; Drummen et al., Citation2008; Dulebenets, Citation2022; Elmi et al., Citation2022; European Maritime Safety Agency EMSA, Citation2021; Falzarano et al., Citation2012; Jovanović et al., Citation2022; Parunov et al., ; Rahim, Citation2019; Y. Wang & Wang, Citation2021). Risk assessment of complex multivariate structural vessel systems may be directly assessed either by conducting extensive direct numerical MC simulations or by possessing sufficiently representative simulated/measured dataset. Most of the dynamic nonstationary engineering marine systems being often too complex for be tackled by both direct MC computational, as well as experimental lab/onboard methods. Authors have recently developed state-of a-t multivariate risk assessment approach, suitable for structural vessel hull systems in an effort to further reduce computational/measurement costs.
Ocean waves have been typically thought as following quasi-stationary, homogenous, piecewise-ergodic stochastic process, having 3-h storms stationary ‘windows’. Think of an offshore engineering or naval structure having large number of degrees of freedom, subjected to random external environmental in situ loadings, that are temporally quasi-stationary, e.g. environmental in situ waves and wind. Another option being to regard dynamic process as being reliant on a number of covariates, whose dynamic change over time may be regarded a quasi-ergodic process. Dynamic vessel system’s vector being now composed/assembled of either measured or numerically MC simulated vessel hull system’s critical/key components
synchronously recorded though sufficient (representative) timelapse
. Dynamic vessel’s system 1D key/critical component’s global maxima, being measured through whole (representative) timelapse
and denoted here as
,
,
, respectively. With large-enough
value one means large value, compared to dynamic vessel hull system’s relaxation, as well as auto-correlation temporal scales (Andersen & Jensen, Citation2014; Balakrishna et al., Citation2022; Gaidai et al., Citation2010a, Citation2010b, Citation2016, Citation2018, Citation2022b; Gaidai, Xu, et al., Citation2022; Gaidai, Yakimov, et al., Citation2023, Citation2023; Gaidai, Yan, et al., Citation2022; Storhaug & Moe, Citation2007; Sun, Gaidai, Xing, et al., Citation2023). Let
be temporally subsequent dynamic vessel hull system critical/key component’s process’s
local maxima, measured/recorded at discrete time-instants
within the observational time period
. System’s critical/key components
being either vessel system’s key/critical loads, or vessel hull’s dynamic responses, on their own. Local maxima for the rest of MDOF dynamic vessel system’s components, e.g.
, being designated as
etc. Ship system’s key/critical component’s local maxima had been assumed being positive. Assessing target damage/hazard/failure risk/probability for a dynamic vessel hull system being now primary objective.
with
being target vessel system’s survival (non-exceedance) chances/probability for critical/key values of dynamic vessel hull system’s critical components ,
,
,… with
denoting logical unity operator «or»;
representing the joint PDF (Probability Density Function) of global vessel system’s key/critical component’s maxima, within timelapse
. Because of high dimensionality possessed by most dynamic engineering systems, along with underlying dataset limitations, in practice being is not possible to straightforwardly assess later vessel hull joint system’s PDF,
. Dynamic vessel system being regarded to have been instantaneously damaged/failed (or have entered state of hazard) at the temporal instants, when either
exceeding
, or
exceeding
, or
exceeding
, etc., fixed damage/hazard/failure levels
,
,
,… being potentially different for each single dynamic vessel system’s 1D component.
,
,
, and so forth. Temporal instants corresponding to local maxima
being now coalesced into one single temporal synthetic system’s vector
preserving temporally increasing order, with
,
Occurrence times of vessel system’s key/critical component’s local maxima have been denoted here by
. MDOF risk/damage/limit/hazard vector
, have been introduced, consisting of hazard/damage/failure limits of dynamic vessel hull system’s key/critical components
. 1D dynamic system’s key load/response critical/key 1D component’s local maxima being now combined into single synthetic vector
, following merged system’s temporal vector
. In order to reduce limit/damage/hazard values for all load/response critical component, scaling parameter
to be introduced. MDOF limit/damage/hazard vector
to be now introduced, having
,
,
, … The latter introduces the unified vessel hull system’s limit/hazard/damage/risk vector
, having each critical component
being equal to either
, or
or
, … , depending on which specific vessel system’s critical/key component has supplied given local maxima, recorded at given temporal moment
; in case of simultaneous occurrence of few vessel hull system critical/key component’s local maxima at specific temporal instant
, values of
have been chosen here to be equal maximum value of those recorded failure/damage/hazard limits, e.g.
. In the above, target dynamic vessel hull system’s survival chances/probability
being defined as a regular function of the scaling parameter
; with
following Eq. (1). Now it is well possible to assess target vessel hull system’s non-exceedance (survival) probability
directly
Due to inter-dependencies between the neighboring are not always be negligible, level of conditioning
to be imposed after 1-step statistical/risk assessment memory’s approximation
for (level of conditioning
). Eq. (4)‘s approximation to be further assessed
where (level of conditioning
) etc. Statistical inter-correlations due to clustered/grouped local maxima may be better captured by the latter approximations’ series
with
being dependent only on level of conditioning
and being independent of
. Now, modified (4-parameter) Weibull’s approach to be utilized for survival (non-exceedance) probability tail extrapolation (Balakrishna et al., Citation2022; Gaidai et al., Citation2010a, Citation2010b, Citation2016, Citation2018, Citation2022b; Gaidai, Yakimov, et al., Citation2023; Gaidai, Yan, et al., Citation2022)
MUR (Mean Up-crossing Rate) function may be utilized when expressing exceedance probability/risk (Gaidai, Fu, et al., Citation2022; Gaidai, Wang, et al., Citation2022; Gaidai & Xing, Citation2022c; Gaidai, Xu, et al., Citation2022; Gaidai et al., Citation2022a; Stanisic et al., Citation2018; X. Xu et al., Citation2019; J. Zhang et al., Citation2018, Citation2019), following Eq. (5). If conditioning parameter being large
Eq. (6) for representing MUR function
Non-dimensional synthetic vector that was previously introduced , being represented as scaled MDOF dynamic vessel system’s coalesced vector
Next,
being MUR function of non-dimensional vessel system’s failure/damage/hazard level
. Although vessel hull system’s quasi-stationarity had been pre-assumed, Gaidai multivariate risk assessment methodology being equally suitable for non-stationary systems. Provided in-situ scatter diagram, having
environmental in-situ ocean/sea states, with each of which having probability/chance of occurrence of
with
, matching the long-term equality
with matching particular short-term ambient ocean/sea condition, having index m. For high/extreme values of
(approaching non-dimensional target level
) probability-related
functions, introduced above, being often quite regular within PDF’s tail (
). PDF frequently exhibits tail behavior, often resembling
, with four parameters
being constants/parameters, fitted to roper PDF tail’s cut-on
value. SQP (Sequential Quadratic Programming) approach, created by NAG (Numerical Algorithms Group) library (Gaidai et al., Citation2016), may be well utilized in order to optimally fit parameters/variables
, optimal parameter values, see (Gaidai & Xing, Citation2022a, Citation2022b; Gaidai, Wang, et al., Citation2022; Gaidai, Wu, et al., Citation2022; Gaidai, Xing, et al., Citation2023; Gaidai, Xu, et al., Citation2022, Citation2022; Gaidai, Yan, et al., Citation2023).
3. Results
This section presents Gaidai multivariate risk assessment method’s validation, utilizing synthetic, along with analogue measured on-board datasets.
3.1. Synthetic MC example
Current section presents synthetic MC example, when exact underlying PDF analytical solution being known. That allows statistical methods direct validation versus the exact analytical prediction. Let us consider 3.65-dayly maxima windspeeds process that has been MC simulated, across the whole representative timelapse
. Underlying non-dimensionalized normalized stochastic/random processes
has been modelled here as Gaussian stationary process, having zero mean, unit standard deviation. Thus, it had been assumed that
MUR function satisfies equality
, where
year. The latter artificial assumption being not uncommon within wind and offshore engineering (Gaidai, Fu, et al., Citation2022). In this MC numerical example, the dataset contained
datapoints, equivalent to a 100 years record, as windspeed’s maxima process
has 365/3.65=
datapoints per year. Underlying windspeed’s process
resulting in 3.65 days maxima CDF (Cumulative Distribution distribution)
for windspeed’s 3-day maxima process
, with
. 3 Archimedean dependence copulas, being commonly in use: Clayton, Gumbel-Haugaard, Frank. 1st, Gumbel-Haugaard’s copula
between 2 peak windspeed’s marginal variables
and identically distributed cross-correlated stochastic process
to be generated
having parameter expressed through correlation coefficient
between 2 processes
and
in this section
has been set to 0.5. Underlying windspeed’s processes
,
being identically distributed and stationary Gaussian processes, Gumbel-Haugaard dependence copula had been quite easy to fit to 4-parameter 2D (bivariate) Weibull’s method. It was expected that there will be no any significant differences between the Gaidai multivariate risk assessment method and 4-parameter bivariate Weibull’s method forecasts, in terms of the predicted decimal log probability level, for a given critical/risk level of interest. In this section, following critical bivariate level had been chosen:
,
. 2D extreme value CDF corresponding to local peak events dataset being
illustrates MC-simulated stochastic timeseries, along with 4-parameter 2D Weibull method contour, and Gaidai multivariate risk assessment method’s forecast, based on vector, the star in indicates the target risk/probability level. As has been expected, agreement between two methods has been quite good. 2nd, Clayton dependence copula
, being an asymmetric Archimedean copula, had been applied in a similar way, instead of the Gumbel-Haugaard’s dependence copula
Figure 4. Left: 4-parameter 2D Weibull’s contour. Right: matching Gaidai multivariate risk assessment method’s forecast, based on system’s synthetic vector.

Clayton’s copula being less convenient for fitting by 4-parameter 2D Weibull’s method, as it was not within ‘copula library’. Thus, in the latter case, 4-parameter 2D Weibull method being expected to perform less accurate, than Gaidai multivariate risk assessment method, since Gaidai multivariate risk assessment does not rely on any dependence copula’s assumptions. All numerical parameters had been unchanged, wrt previous Gumbel-Haugaard’s copula case. Series of synthetic independent MC tests have shown that Gaidai multivariate risk assessment method predicted exceedance probability/risk decimal log-level even more accurately, than 4-parameter 2D Weibull’s method. For the numerical setup, described above, it has been found that Gaidai multivariate risk assessment method performed nearly 20% more accurately, than 4-parameter 2D Weibull’s method. Synthetic dataset has been based on underlying Gaussian process, as well as Archimedean copulas. Gaidai multivariate risk assessment methodology key advantages may be way more pronounced in case of real field measured non-Gaussian, inter-correlated by non-Archimedean dependence copula dataset. Computational effort, attributed to 4-parameter 2D Weibull’s method being way heavier, compared to Gaidai multivariate risk assessment methodology, for any given 2D failure/damage limit, as 4-parameter 2D Weibull’s method performing 2D surface’s interpolation. The latter being significant, if analysing large datasets, as in will be shown in the next section.
3.2. Cargo vessel on-board analysis
This section benchmarks Gaidai multivariate risk assessment method’s efficiency, proven by means of application to specific container ship on-board measured acceleration and deck panel stresses dataset. It has been known that container vessel panel’s dynamic stresses and accelerations, coupled to cargo vessel hull’s vibrations constituting highly nonlinear, multivariate, cross-correlated dynamic system, that is quite challenging to study. Cargo vessel dynamic system’s risk assessment study being important for large container ships, crossing Atlantic’s, experiencing potentially extreme ocean/weather conditions. MSC Napoli had been broken in vessel’s engine room’s bulkhead, while MOL Comfort had been broken amidships. Although these two ships may not have been designed/approved according to the best practise, recommended for collapse strength, compared to other similar container vessels, both vessels had been broken due to ship hull girder overloading. 4400 TEU Panamax container vessel had been instrumented with numerous motion on-board sensors during all trans-Atlantic voyages, DNV-instrumented (DNV-RP-H103, Citation2011). Further explanation of the measuring systems setup is given in (Storhaug & Moe, Citation2007). Container ship has been instrumented, using standard hull monitoring systems, in accordance with DNV hull monitoring standards/rules (DNV-RP-C205, Citation2010). Vessel has operated in trading activities between Germany, Belgium and Canada. Ship has not been built following DNV class but was transferred to DNV class, in the year 2004 receiving notation + 1A1, ICE-1A, EO, NAV-O, Container Carrier, TMON. The ship had been built in the year 1998, having pronounced bow flare, having somewhat old-fashioned cross-sectional vessel design. Two different ship panel stresses, located mid and aft of the ship have been chosen as critical components hence constituting practical example of 2D dynamic cargo ship dynamic system.
presents 4400 TEU strain sensors on-board placements, along with observed initial crack positions. Sensor’s placement has been done following DNV container ship’s regulations/rules. Only the upper vessel deck (see upper circle) sensor measurements have been utilised for this study. Analogously, sensors had been placed aft of the ship, resulting in on-board measured stresses following longitudinal direction on the flat bar, below upper deck. In this section, the following abbreviations to be utilized:
P – Port; S – Starboard
DL – Deck Longitudinal
DLP – Deck Longitudinal Port sensor (port side)
AFV – vessel’s forward acceleration
For unification of two measured timeseries , scaling had been performed, following Eq. (11), making two key components non-dimensional with identical failure/damage/hazard limits, equal to 1. All local maxima, extracted from two measured timeseries have been merged/coalesced into one single timeseries, by keeping them in a temporally increasing order:
. In order to unify two measured timeseries
, following scaling had been performed
with ,
being dimensional maxima, recorded over the whole observational timelapse, for dynamic vessel system’s key/critical components
, respectively.
refers only to a chosen stress-acceleration couple (2D failure/damage/hazard point).
Figure 6. Synthetic vector , derived from non-dimensionalized DLP (
) and AFV (
) vessel dynamic components.

left presenting 4-parameter 2D Weibull’s method contours for DLP, AFV (Gaidai & Xing, Citation2023; Gaidai, Cao, Xing, et al., Citation2023; Gaidai, Cao, Xu, et al., Citation2023; Gaidai, Wang, et al., Citation2023; Gaidai, Xing, et al., Citation2023; Gaidai, Xu, et al., Citation2023, Citation2023; Xing & Gaidai, Citation2023). As seen from left, 4-parameter 2D Weibull’s method fits various Gumbel copula to the underlying measured dataset; hence, there is an inherent error, due to particular copula choice. For more details on 4-parameter 2D Weibull’s method, see (Gaidai, Xing, et al., Citation2023; Gaidai, Yan, et al., Citation2023; Gaidai et al., Citation2023a; Liu et al., Citation2023; Yakimov, Gaidai, Wang, & Wang, Citation2023; J. Zhang et al., Citation2023). For further details on Gaidai multivariate risk assessment method, [77]- (Gaidai, Yakimov, et al., Citation2023). 2D failure/hazard non-dimensional design point ,
had been chosen, as seen in left; synthetic vector
has been derived from non-dimensionalized DLP and AFV, namely, AFV acceleration record has been scaled by means of multiplying with factor
, see . Probability/risk threshold/level
corresponding to this particular contour line had been then compared with Gaidai multivariate risk assessment method’s estimate, see right. It has been found that modified (4-parameter) 2D Weibull method risk/probability level forecast lied well within 95% CI, predicted by Gaidai multivariate risk assessment method, [87]
Figure 7. a) 4-parameter 2D Weibull’s method contours for non-dimensionalized DLP and AFV; b) corresponding Gaidai multivariate risk assessment forecast, dashed lines indicate 95% CI (confidence intervals).

The SODP (second-order difference plot) originated from the Poincare-type plot. SODP-type pllot reflects statistical patterns, residing within consecutive differences within the underlying timeseries dataset.
presents a second-order SODP-type plot, these kinds of plots may be used for dataset pattern recognition, QC (Quality Control), and comparison with other relevant similar datasets, for example using entropy AI (Artificial Intelligence) recognition approach (Gaidai et al., Citation2024; Gaidai et al., Citation2010a; Gaidai et al., Citation2010b; Gaidai et al., Citation2022; Gaidai et al., Citation2023b; Gaidai et al., Citation2023; Sun et al., Citation2023; Yakimov et al., Citation2023; Gaidai, Sheng, Cao, Zhu, et al., Citation2024).
Gaidai multivariate risk assessment method treats ship’s dynamic system as black box, having large number of covariates/random parameters NDOF (Number of Degrees Of Freedom), i.e. the number of vessel system’s key dimensions being virtually unlimited, and all vessel system’s critical components being coalesced/merged into 1D systems synthetic vector . Cargo vessel’s panel stress PSD (Power Spectral Density) within most stationary 3 h ocean/sea states containing two peaks (i.e. bimodal spectrum). WF (Wave Frequency) part was related to the wave-induced loads, and HF (High Frequency) part was caused by the transient load/resonant vibrations, known as springing/whipping. Subsequent research endeavours pertaining to the whipping phenomena need to scrutinize the whole dynamics of stress and accelerations in this study and discretely tackle the responses filtered at the low and high passes. The novel Gaidai multivariate risk assessment approach is applicable to higher dimensional cooperatively quasi-stationary, nonlinear dynamic systems. Key failure/damage mechanisms such as sudden shock damage, fatigue failures, corrosion-type cracks, or other ultimate collapse processes connected to the dynamic system of a cross-coupled cargo vessel may be introduced as additional dimensions or failure/damage modes to the dynamic system of interest if such measurements are available. The study’s long-term goal is to create a reliable code for ship route design that takes excessive loads and fatigue build-up into account. This is a crucial task, and future study may build on the suggested risk assessment strategy. It may be advised that further research to be done on other stochastic structural models for wind-wave environments in realistic oceanic regions. Potential research outcomes will greatly advance design practices of contemporary cargo vessels.
4. Discussion
The cargo ship on-board dataset was used to validate state-of-art multivariate risk assessment approach that this study offered. Most realistic choices for complex dynamic systems are out of reach, such as the direct MC simulation technique, lengthy laboratory tests, or, in the best-case scenario, on-board measurements. While the proposed Gaidai multivariate risk assessment methodology has no restrictions on the number of system dimensions, existing risk assessment methodologies utilized in naval design being mostly restricted to 2D systems. Traditional risk assessment techniques, pertaining to timeseries, lack the benefit of effectively managing systems with high dimensionality and cross-correlation across distinct critical components inside vessel hull’s dynamic system. The capability of the presented approach to examine the risk assessment of high-dimensional dynamic systems is a key benefit. Previous validation of the approach described in this study was done using a variety of simulation models, but only for one-dimensional system responses. Overall, highly accurate predictions have been made. This work aims to design a multivariate risk assessment approach that is resilient, easy to use, and general-purpose.
Regarding on-board measurements, confidentiality concerns mean that these datasets being rarely made accessible for public research presentations. Current study was able to make use of a genuine, sizable, raw, measured on-board dataset. The goal of this research is to support the creation of new DNV safety guidelines and standards for the modern marine transportation sector, which may have an impact on future cargo transport insurance and cargo vessel operating policies.
5. Conclusions
This study examined ship dynamic response timeseries and a synthetic windspeed data set that were collected on board during more than 70 transatlantic trips by 4400 TEU Panamax container ships in the 2010s. A detailed explanation of the theoretical basis for the suggested approach is provided. It should be noted that it is appealing to analyse the dynamic system risk assessment using direct measurement or large-scale MC simulations. The enormous dimensionality and complexity of dynamic systems, however, need the development of novel, reliable, yet accurate algorithms that can work with a small amount of accessible data while making the most use of it. Using both synthetic and real on-board measured ship panel stress datasets, the innovative technique was verified against the 4-parameter bivariate Weibull method bivariate method. Finally, a variety of engineering domains can employ the proposed technique. The example of naval architecture given does not restrict the domains in which Gaidai multivariate risk assessment approach methodology may be utilized.
For future studies, larger container vessels along with increased number of sensor readings at additional crucial points on deck panels can be studied. Larger TEU vessel hull whipping responses – which are concurrently captured at many vessel hull locations – to receive special attention. When discussing constraints and limitations of Gaidai multivariate risk assessment methodology, non-stationary dynamic vessel systems with underlying trends should be brought up. In the latter scenario, the Gaidai multivariate risk assessment approach can still be used once the underlying trend has been first determined and then removed from the raw information.
List of abbreviations
TEU | = | twenty feet equivalent unit |
MC | = | Monte Carlo |
AOG | = | Active Operator Guidance |
CI | = | Confidence Interval |
P | = | port, S – starboard side |
DL | = | deck longitudinal |
DLP | = | deck sensor on port side |
AFV | = | vessel’s forward acceleration |
Author contributions
All authors have contributed equally.
Disclosure statement
No potential conflict of interest was reported by the author(s).
Data availability statement
Data will be available on request from the corresponding author.
Additional information
Funding
References
- Abioye, O., Dulebenets, M., Kavoosi, M., Pasha, J., & Theophilus, O. (2021). Vessel schedule recovery in Liner shipping: Modeling alternative recovery options. IEEE Transactions on Intelligent Transportation Systems, 22(10), 6420–21. https://doi.org/10.1109/TITS.2020.2992120
- Andersen, I. M. V., & Jensen, J. J. (2014). Measurements in a container ship of wave induced deck panel girder stresses in excess of design values. Marine Structures, 37, 54–85. https://doi.org/10.1016/j.marstruc.2014.02.006
- Balakrishna, R., Gaidai, O., Wang, F., Xing, Y., & Wang, S. (2022). A novel design approach for estimation of extreme load responses of a 10-MW floating semi-submersible type wind turbine. Ocean Engineering, 261, 112007. https://doi.org/10.1016/j.oceaneng.2022.112007
- Bicen, S., Kandemir, C., & Celik, M. (2021). A human risk assessment analysis to crankshaft overhauling in dry-docking of a General cargo ship. Proceedings of the Institution of Mechanical Engineers Part M: Journal of Engineering for the Maritime Environment, 235(1), 93–109. https://doi.org/10.1177/1475090220948338
- Bureau Veritas, B. V. (2012). NR 493, classification of mooring systems for permanent offshore units.
- Chen, X., Yan, R., Shining, W., Zhiyuan, L., Haoyu, M., & Wang, S. (2021). A fleet deployment model to minimize the covering time of maritime rescue missions. Maritime Policy & Management, 50(6), 724–749. https://doi.org/10.1080/03088839.2021.2017042
- DNV-RP-C205. (2010). Environmental conditions and environmental loads.
- DNV-RP-H103. (2011). Modelling and analysis of marine operations.
- Drummen, I., Storhaug, G. , & Moan, T. (2008). Experimental and numerical investigation of fatigue damage due to wave-induced vibrations in a containership in head seas. Journal of Marine Science and Technology, 13, 428–445. https://doi.org/10.1007/s00773-008-0006-5
- Dulebenets, M. (2022). Multi objective collaborative agreements amongst shipping lines and marine terminal operators for sustainable and environmental-friendly ship schedule design. Journal of Cleaner Production, 342(12), 130897. https://doi.org/10.1016/j.jclepro.2022.130897
- Ellermann, K. (2008). Nonlinear dynamics of offshore systems in random seas. In IUTAM symposium on fluid-structure interaction in ocean engineering (pp. 45–56). Springer.
- Elmi, Z., Prashant, S., Vamshi, K., Meriga, V., Goniewicz, K., Borowska-Stefanska, M., Wisniewski, S., & Dulebenets, M. (2022). Uncertainties in Liner shipping and ship schedule recovery: A state-of-the-art review. Journal of Marine Science and Engineering, 10(5), 563. https://doi.org/10.3390/jmse10050563
- European Maritime Safety Agency (EMSA). (2021). Annual overview of Marine casualties and incidents. European Maritime Safety Agency.
- Falzarano, J., Su, Z., Jamnongpipatkul, A. (2012), Application of stochastic dynamical system to nonlinear ship rolling problems, Proceedings of the 11th International Conference on the Stability of Ships and Ocean Vehicles, Athens, Greece
- Gaidai, O., Cao, Y., & Loginov, S. (2023). Global cardiovascular diseases death rate prediction. Current Problems in Cardiology, 48(5), 101622. https://doi.org/10.1016/j.cpcardiol.2023.101622
- Gaidai, O., Cao, Y., Xing, Y., & Balakrishna, R. (2023). Extreme springing response statistics of a tethered platform by deconvolution. International Journal of Naval Architecture and Ocean Engineering, 15, 100515. https://doi.org/10.1016/j.ijnaoe.2023.100515
- Gaidai, O., Cao, Y., Xing, Y., & Wang, J. (2023). Piezoelectric Energy Harvester Response Statistics. Micromachines, 14(2), 271. https://doi.org/10.3390/mi14020271
- Gaidai, O., Cao, Y., Xu, X., & Xing, Y. (2023). Offloading operation bivariate extreme response statistics for FPSO vessel. Scientific Reports, 13(1). https://doi.org/10.1038/s41598-023-31533-8
- Gaidai, O., Fu, S., & Xing, Y. (2022). Novel risk assessment method for multidimensional nonlinear dynamic systems. Marine Structures, 86, 103278. https://doi.org/10.1016/j.marstruc.2022.103278
- Gaidai, O., Liu, Z., Wang, K., & Bai, X. (2023). Current COVID-19 Epidemic Risks in Brazil. Epidemiology International Journal, 7(2), 1–10. https://doi.org/10.23880/eij-16000259
- Gaidai, O., Sheng, J., Cao, Y., Zhang, F., Zhu, Y., & Loginov, S. (2024). Public health system sustainability assessment by Gaidai hypersurface approach. Current Problems in Cardiology, 49(3), 102391. https://doi.org/10.1016/j.cpcardiol.2024.102391
- Gaidai, O., Sheng, J., Cao, Y., Zhu, Y., & Loginov, S. (2024). Generic COVID-19 epidemic forecast for Estonia by Gaidai multivariate risk assessment method. Franklin Open. https://doi.org/10.1016/j.fraope.2024.100075
- Gaidai, O., Sheng, J., Cao, Y., Zhu, Y., Wang, K., & Liu, Z. (2024). Limit hypersurface state of art gaidai reliability approach for oil tankers Arctic operational safety. Journal of Ocean Engineering and Marine Energy. https://doi.org/10.1007/s40722-024-00316-2
- Gaidai, O., Storhaug, G., Naess, A. (2010a). Extreme value statistics of ship rolling. PRADS Proceedings. Practical Design of Ships and Other Floating Structures. Vol 2, 457–466.
- Gaidai, O., Storhaug, G., Naess, A. (2010b). Extreme value statistics of whipping response for large ships. PRADS Proceedings. Practical Design of Ships and Other Floating Structures. Vol 2, 1210–1221.
- Gaidai, O., Storhaug, G., & Naess, A. (2016). Extreme large cargo ship panel stresses by bivariate 4-parameter bivariate Weibull method method. Ocean Engineering, 123, 432–439. https://doi.org/10.1016/j.oceaneng.2016.06.048
- Gaidai, O., Storhaug, G., & Naess, A. (2018). Statistics of extreme hydro elastic response for large ships. Marine Structure, 61, 142–154. https://doi.org/10.1016/j.marstruc.2018.05.004
- Gaidai, O., Wang, F., Cao, Y., & Liu, Z. (2024). 4400 TEU cargo ship dynamic analysis by Gaidai risk assessment method. Journal of Shipping and Trade, 9(1), 2024. https://doi.org/10.1186/s41072-023-00159-4
- Gaidai, O., Wang, F., & Sun, J. (2024). Energy harvester risk assessment study by Gaidai risk assessment method. Climate Resilience and Sustainability, 3(1). https://doi.org/10.1002/cli2.64
- Gaidai, O., Wang, K., Wang, F., Xing, Y., & Yan, P. (2022). Cargo ship aft panel stresses prediction by deconvolution. Marine Structures, 88, 103359. https://doi.org/10.1016/j.marstruc.2022.103359
- Gaidai, O., Wang, F., Wu, Y., Xing, Y., Medina, A., & Wang, J. (2022). Offshore renewable energy site correlated wind-wave statistics. Probabilistic Engineering Mechanics, 68. https://doi.org/10.1016/j.probengmech.2022.103207
- Gaidai, O., Wang, F., Wu, Y., Xing, Y., Rivera Medina, A., & Wang, J. (2022). Offshore renewable energy site correlated wind-wave statistics. Probabilistic Engineering Mechanics, 68, 103207. https://doi.org/10.1016/j.probengmech.2022.103207
- Gaidai, O., Wang, F., Yakimov, V. (2023). COVID-19 multi-state epidemic forecast in India. Proceedings of the Indian National Science Academy. https://doi.org/10.1007/s43538-022-00147-5
- Gaidai, O., Wang, F., Yakimov, V., Sun, J., & Balakrishna, R. (2023). Lifetime assessment for riser systems. Green Technology, Resilience, and Sustainability, 3(1). https://doi.org/10.1007/s44173-023-00013-7
- Gaidai, O., Wu, Y., Yegorov, I., Alevras, P., Wang, J., & Yurchenko, D. (2022). Improving performance of a nonlinear absorber applied to a variable length pendulum using surrogate optimization. Journal of Vibration and Control, 30(1–2), 156–168. https://doi.org/10.1177/10775463221142663
- Gaidai, O., & Xing, Y. (2022a). A novel bio-system risk assessment approach for multi-state COVID-19 epidemic forecast. Engineered Science. https://doi.org/10.30919/es8d797
- Gaidai, O., & Xing, Y. (2022b). A novel multi regional risk assessment method for COVID-19 death forecast. Engineered Science. https://doi.org/10.30919/es8d799
- Gaidai, O., & Xing, Y. (2022c). Novel risk assessment method validation for offshore structural dynamic response. Ocean Engineering, 266(5), 113016. https://doi.org/10.1016/j.oceaneng.2022.113016
- Gaidai, O., & Xing, Y. (2023). Prediction of death rates for cardiovascular diseases and cancers. Cancer Innovation, 2(2), 140–147. https://doi.org/10.1002/cai2.47
- Gaidai, O., Xing, Y., & Balakrishna, R. (2022). Improving extreme response prediction of a subsea shuttle tanker hovering in ocean current using an alternative highly correlated response signal. Results in Engineering, 15, 100593. https://doi.org/10.1016/j.rineng.2022.100593
- Gaidai, O., Xing, Y., Balakrishna, R., & Xu, J. (2023). Improving extreme offshore windspeed prediction by using deconvolution. Heliyon, 9(2), e13533. https://doi.org/10.1016/j.heliyon.2023.e13533
- Gaidai, O., Xing, Y., & Xu, X. (2023). Novel methods for coupled prediction of extreme windspeeds and wave heights. Scientific Reports, 13(1). https://doi.org/10.1038/s41598-023-28136-8
- Gaidai, O., Xing, Y., Xu, J., & Balakrishna, R. (2023). Gaidai-xing risk assessment method validation for 10-MW floating wind turbines. Scientific Reports, 13(1). https://doi.org/10.1038/s41598-023-33699-7
- Gaidai, O., Xu, J., Hu, Q., Xing, Y., & Zhang, F. (2022). Offshore tethered platform springing response statistics. Scientific Reports, 12(1), 41598–022–25806–x. https://doi.org/10.1038/s41598-022-25806-x
- Gaidai, O., Xu, X., & Xing, Y. (2023). Novel deconvolution method for extreme FPSO vessel hawser tensions during offloading operations. Results in Engineering, 17, 100828. https://doi.org/10.1016/j.rineng.2022.100828
- Gaidai, O., Xu, J., Xing, Y., Hu, Q., Storhaug, G., Xu, X., & Sun, J. (2022). Cargo vessel coupled deck panel stresses risk assessment study. Ocean Engineering, 268, 113318. https://doi.org/10.1016/j.oceaneng.2022.113318
- Gaidai, O., Xu, J., Yakimov, V., & Wang, F. (2023a). Analytical and computational modeling for multi-degree of freedom systems: Estimating the likelihood of an FOWT Structural Failure. Journal of Marine Science and Engineering, 11(6), 1237. https://doi.org/10.3390/jmse11061237
- Gaidai, O., Xu, J., Yakimov, V., & Wang, F. (2023b). Liquid carbon storage tanker disaster resilience. Environment Systems and Decisions. https://doi.org/10.1007/s10669-023-09922-1
- Gaidai, O., Xu, J., Yan, P., Xing, Y., Wang, K., & Liu, Z. (2023). Novel methods for risk assessment study of multi-dimensional non-linear dynamic systems. Scientific Reports, 13(1), 3817. https://doi.org/10.1038/s41598-023-30704-x
- Gaidai, O., Xu, J., Yan, P., Xing, Y., Wu, F., & Zhang, Y. (2022). Novel methods for windspeeds prediction across multiple locations. Scientific Reports, 12(1), 19614. https://doi.org/10.1038/s41598-022-24061-4
- Gaidai, O., Yakimov, V., & Balakrishna, R. (2023). Dementia death rates prediction. BMC Psychiatry, 23(691). https://doi.org/10.1186/s12888-023-05172-2
- Gaidai, O., Yakimov, V., Hu, Q., & Loginov, S. (2024). Multivariate risks assessment for complex bio-systems by Gaidai risk assessment method. Systems and Soft Computing, 6, 200074. https://doi.org/10.1016/j.sasc.2024.200074
- Gaidai, O., Yakimov, V., Niu, Y., & Liu, Z. (2023). Gaidai-Yakimov risk assessment method for high-dimensional spatio-temporal biosystems. Biosystems, 235, 105073. https://doi.org/10.1016/j.biosystems.2023.105073
- Gaidai, O., Yakimov, V., Sun, J., & van Loon, E.-J. (2023). Singapore COVID-19 data cross-validation by the Gaidai risk assessment method. Npj Viruses, 1(1). https://doi.org/10.1038/s44298-023-00006-0
- Gaidai, O., Yakimov, V., & van Loon, E. (2023). Influenza-type epidemic risks by spatio-temporal Gaidai-Yakimov method. Dialogues in Health, 3(2), 100157. https://doi.org/10.1016/j.dialog.2023.100157
- Gaidai, O., Yakimov, V., Wang, F. (2024). Gaidai Multivariate Reliability Method for Energy Harvester Operational Safety, given manufacturing imperfections. International Journal of Precision Engineering and Manufacturing. https://doi.org/10.1007/s12541-024-00977-x
- Gaidai, O., Yakimov, V., Wang, F., Hu, Q., Storhaug, G., & Wang, K. (2023). Lifetime assessment for container vessels. Applied Ocean Research, 139, 103708. https://doi.org/10.1016/j.apor.2023.103708
- Gaidai, O., Yakimov, V., Wang, F., Sun, J., & Wang, K. (2024). Bivariate risk assessment analysis for floating wind turbines. International Journal of Low-Carbon Technologies”, 19, 55–64. https://doi.org/10.1093/ijlct/ctad108
- Gaidai, O., Yakimov, V., Wang, F., Xing, Y., & Zhang, F. (2023). Safety design study for energy harvesters. Sustainable Energy Research, 10(1). https://doi.org/10.1186/s40807-023-00085-w
- Gaidai, O., Yakimov, V., Wang, F., Zhang, F., & Balakrishna, R. (2023). Floating wind turbines structural details fatigue life assessment. Scientific Reports, 13(1). https://doi.org/10.1038/s41598-023-43554-4
- Gaidai, O., Yakimov, V., & Zhang, F. (2023). COVID-19 spatio-temporal forecast in England. Biosystems, 233, 105035. https://doi.org/10.1016/j.biosystems.2023.105035
- Gaidai, O., Yan, P., & Xing, Y. (2022a). A novel method for prediction of extreme windspeeds across parts of Southern Norway. Frontiers in Environmental Science, 10. https://doi.org/10.3389/fenvs.2022.997216
- Gaidai, O., Yan, P., & Xing, Y. (2022b). Prediction of extreme cargo ship panel stresses by using deconvolution. Frontiers in Mechanical Engineering, 8. https://doi.org/10.3389/fmech.2022.992177
- Gaidai, O., Yan, P., & Xing, Y. (2023). Future world cancer death rate prediction. Scientific Reports, 13(1). https://doi.org/10.1038/s41598-023-27547-x
- Gaidai, O., Yan, P., Xing, Y., Xu, J., Wu, Y. (2022). A novel statistical method for long-term coronavirus modelling.F1000 research. https://orcid.org/0000-0003-0883-48542
- Gaidai, O., Yan, P., Xing, Y., Xu, J., & Wu, Y. (2023). Gaidai risk assessment method for long-term coronavirus modelling. F1000 Research, 11, 1282. https://doi.org/10.12688/f1000research.125924.3
- Gaidai, O., Yan, P., Xing, Y., Xu, J., Zhang, F., & Wu, Y. (2023). Cargo vessel under ice loadings. Scientific Reports, 13(1). https://doi.org/10.1038/s41598-023-34606-w
- ISSC. (2009). Proceedings of the 17th international ship and offshore structures congress: III.1 ultimate strength. In C. D. Jang, S. Y. Hong, Eds. International ship and offshore structures congress, Korea.
- Jovanović, I., Perčić, M., Koričan, M., Vladimir, N., & Fan, A. (2022). Investigation of the viability of unmanned autonomous container ships under different carbon pricing scenarios. Journal of Marine Science and Engineering, 10(12), 1991. https://doi.org/10.3390/jmse10121991
- Liu, Z., Gaidai, O., Xing, Y., & Sun, J. (2023). Deconvolution approach for floating wind turbines. Energy Science & Engineering, 11(8), 2742–2750. https://doi.org/10.1002/ese3.1485
- OCIMF, Oil Company International Marine Form. (2007). Mooring Equipment Guide.
- Rahim, G. P. (2019). Container ship accident analysis due to container stacked on deck as an attempt to improve maritime logistic system. E3S Web of Conferences, 130, 01010. https://doi.org/10.1051/e3sconf/201913001010
- Stanisic, D., Efthymiou, M., Kimiaei, M., & Zhao, W. (2018). Design loads and long term distribution of mooring line response of a large weathervaning vessel in a tropical cyclone environment. Marine Structures, 61, 361–380. https://doi.org/10.1016/j.marstruc.2018.06.004
- Storhaug, G., Moe, E. (2007). Measurements of wave induced vibrations on-board a large container vessel operating in harsh environment. Proceedings of the 10th international symposium on practical design of ships and other floating structures, Houston, US (pp. 64–72).
- Su, Z. (2012). Nonlinear response and stability analysis of vessel rolling motion in random waves using stochastic dynamical systems. Texas University.
- Sun, J., Gaidai, O., & Wang, F. (2023). Gaidai risk assessment method for fixed offshore structures. Journal of the Brazilian Society of Mechanical Sciences and Engineering, 46(27). https://doi.org/10.1007/s40430-023-04607-x
- Sun, J., Gaidai, O., Xing, Y., Wang, F., & Liu, Z. (2023). On safe offshore energy exploration in the Gulf of Eilat. Quality and Risk Assessment Engineering International, 39(7), 2957–2966. https://doi.org/10.1002/qre.3402
- Tomic, B., Turk, A., & Čalić, B. (2018). Recent advances in damage stability assessment with application on a container vessel. Journal of Maritime & Transportation Science, 2(Special edition 2), 167–184. https://doi.org/10.18048/2018-00.167
- Wang, Y., & Wang, S. (2021). Deploying, scheduling, and sequencing heterogeneous vessels in a liner container shipping route. Transportation Research Part E: Logistics & Transportation Review, 151(2). https://doi.org/10.1016/j.tre.2021.102365
- Xing, Y., & Gaidai, O. (2023). Multi-regional COVID-19 epidemic forecast in Sweden. Digital Health, 9(9), 205520762311629. https://doi.org/10.1177/20552076231162984
- Xu, X., Sahoo, P., Evans, J., & Tao, Y. (2019). Hydrodynamic performances of FPSO and shuttle tanker during side-by-sideoffloading operation, ships and offshore structures. Ships and Offshore Structures, 14(sup1), 292–299. https://doi.org/10.1080/17445302.2019.1580845
- Yakimov, V., Gaidai, O., Wang, F., & Wang, K. (2023). Arctic naval launch and recovery operations, under ice impact interactions. Applications in Engineering Science, 15, 100146. https://doi.org/10.1016/j.apples.2023.100146
- Yakimov, V., Gaidai, O., Wang, F., Xu, X., Niu, Y., & Wang, K. (2023). Fatigue assessment for FPSO hawsers. International Journal of Naval Architecture and Ocean Engineering, 15, 100540. https://doi.org/10.1016/j.ijnaoe.2023.100540
- Zhang, J., Gaidai, O., & Gao, J. (2018). Bivariate extreme value statistics of offshore jacket support stresses in Bohai bay. The Journal of Offshore Mechanics and Arctic Engineering, 140(4), 041305. https://doi.org/10.1115/1.4039564
- Zhang, J., Gaidai, O., Ji, H., & Xing, Y. (2023). Operational risk assessment study of ice loads acting on cargo vessel bow. Heliyon, 9(4), e15189. https://doi.org/10.1016/j.heliyon.2023.e15189
- Zhang, J., Gaidai, O., Wang, K., Xua, L., Ye, R., & Xu, X. (2019). A stochastic method for the prediction of icebreaker bow extreme stresses. Applied Ocean Research, 87, 95–101. https://doi.org/10.1016/j.apor.2019.03.019