Abstract
Cancer, the biggest cause of death globally, remains a tough illness despite enormous advances in therapy. In the present study, 1,3-benzodioxole-tagged dacarbazine derivates were investigated as microtubule inhibitors in order to control cancer as microtubules are involved in cell proliferation. The tubulin protein was analyzed and its structure was validated by various protein validation tools. The binding potential of 1,3-benzodioxole-based dacarbazine-tagged derivatives with tubulin was checked using molecular docking software HEX 8.0 CUDA and AutoDock Vina. Swiss ADME online Web server and pkCSM are used for studying pharmacokinetic and pharmacological studies of compounds. The docking analysis ADME studies displayed that Compounds 1 and 2 bind effectively with the tubulin protein and showed potential properties to use as a potent anticancer drug.
Introduction
Cancer is a major public health problem worldwide and the second leading cause of death in the United States. Adjuvant chemotherapies and combination therapies for many cancers contributed to a drop in cancer deaths [Citation1]. Chemotherapy is a better choice for treatment because the tumour cells are transmigrated to other sites in the body and their eviction by surgery is arduous [Citation2]. The problems are the noxious effect on normal cells [Citation3] and the solubility of chemotherapeutic agents, restricting the use of chemotherapy and demanding the search for new molecules in this area.
Tubulin Microtubules, the dynamic pipe-like protein composed of α,β-heterodimers [Citation4], are integral components of the mitotic spindle which is intimately involved in cell division [Citation5]. Along with α- and β-tubulin as the main components of MT, γ-tubulins, are involved in nucleating M, Ts, and δ-tubulin are implicated in the formation of basal bodies and centrioles. Microtubules contribute to the cell proliferation process in the segregation of chromosomes during mitosis which is the attractive stage for obtrusion of normal function. Since microtubule dynamics play important roles in various cellular functions including mitosis, it is a potential drug target for several diseases including cancer, neuronal, fungal, and parasitic diseases [Citation6,Citation7]. There is no end of clinical trials on microtubule-targeted agents [Citation8]. Microtubule inhibitor-based chemotherapy is a major sanative approach for cancer medicament.
1,3-benzodioxole molecule is one of antimitotic medications, such as podophyllotoxin, steganacin, and combretastatin A-2. In the middle of the 1980s, several 1,3-benzodioxoles were identified as potent tubulin binders, antimitotic agents, and effective in vivo treatments for P388 lymphocytic leukaemia and other cancers. Additionally, it has been noted that a class of 1,3-benzodioxole derivatives are cytotoxic to a number of human tumour cell lines, including human colon carcinoma cells and multi-drug resistant nasopharyngeal carcinoma cells [Citation9–15].
Our research group is working on ionic liquids as potential anticancer agents for better prospects [Citation3,Citation10,Citation15–17]. This paper is in the continuation of research interest in the development of 1,3-benzodioxole based ionic liquids as anticancer drug and further to enhance its impact 1,3-benzodioxole tagged with dacarbazine. Dacarbazine is an antineoplastic which used as a chemotherapy drug for the treatment of malignant melanoma, Hodgkin’s lymphoma [Citation18–20].
The binding of drugs with proteins in blood and serum is an important process in determining the activity, distribution, rate of excretion and toxicity of drugs in the body. To check the binding potential of the target receptor and to get most potent drug in-silico molecular docking analysis of 1,3-benzodioxole derivatives is carried out. By making use of advanced pharmacological computational routes, pharmacokinetic and toxicity properties are perceived [Citation21–23]. The analysis of these parameters helps in validating the further use of designed compounds for preclinical analysis [Citation10].
Methodology
Preparation of target protein
The protein target (PDB ID: 1TUB) structure for docking studies was retrieved from RSCB Protein Data Bank (PDB) repository [Citation24,Citation25]. Preparation of protein was carried out by using Protein preparation Wizard, Whatif server (https://swift.cmbi.umcn.nl/servers/html/prepdock.html).
Structural assessment of protein
The 3D structure of tubulin protein was validated using online structural analysis and verification servers viz., MOLprobity [Citation26] (http://molprobity.biochem.duke.edu/index.php), PDBsum [Citation27] (http://www.ebi.ac.uk/thornton-srv/databases/pdbsum/), VERIFY3D [Citation28] (https://www.doe-mbi.ucla.edu/verify3d/) and ProSA [Citation29] (https://prosa.services.came.sbg.ac.at/prosa.php). The MOLprobity server generated the Ramachandran plot for protein showing Ramachandran favoured rotamers and Ramachandran outliers. The PDBsum was used to know protein-protein interactions, protein-ligand interactions, and types of ligands. A higher Verify3D score indicates the good quality of protein structure. Overall protein structural quality is defined by the Z-score obtained from ProSA web server.
Ligand preparation and optimization for molecular docking
The known anticancer compound 1,3-Benzodioxole-based dacarbazine tagged derivatives () were drawn by using Chemdraw Ultra software (CambridgeSoft Corp., Cambridge, MA) [Citation30]. The derivatives obtained by replacing the anionic part of the parent ionic liquid were further imported to Chimaera for geometry optimization.
Table 1. The HEX server and AutoDock Vina docking score of Compound 1 to 10 with tubulin protein.
Molecular docking analysis of designed compounds
Rigid docking was accomplished with the help of HEX 8.0 CUDA program followed by semi-flexible molecular docking using AutoDock Vina in order to strengthen the optimized results with two different molecular docking algorithms. In HEX, prediction of total energy was based on Shape-only correlation type and 3-D FFT mode. To bind the ligands on the target, a grid box dimension was set to 40 × 40 × 40 using AutoDock4 software
Non-bond interactions and binding residues involved in the binding of the docked complex were evaluated using PLIP server and then with Acceryls Discovery Studio for better graphical presentation.
Pharmacological studies
Lipinski Rule of Five (scfbio-iitd.res.in) helps in the characterization of drug and non-drug-like behaviour [Citation31]. The physicochemical and pharmacokinetic properties of 1,3-benzodioxole derivatives were predicted by Swiss ADME online Web server http://swissadme.ch/ and pkCSM (http://bleoberis.bioc.cam.ac.uk/pkcsm/) [Citation23,Citation32–34]. The bioavailability radar elucidates the drug-likeliness of the compound by considering the physicochemical properties like LIPO (lipophilicity), SIZE, POLAR (Polarity), INSOLU (Insolubility), INSATU (Insaturation), and FLEX (Flexibility) [Citation35, Citation36].
The intestinal absorption and brain penetration properties were predicted from a simple and intuitive graph (BOILED-Egg model) as a function of lipophilicity (described by WLOGP) and permeability (described as TPSA) [Citation37].
The hERG safety is required for testing a new bioactive molecule [Citation38]. Many chemical compounds from different therapeutic areas induced cardiac arrhythmia by affecting the cardiac ion channel currents [Citation39]. The hERG liability was checked by openly available Pred-hERG web-server (http://predherg.labmol.com.br/predict).
Results
Structure determination and evaluation of tubulin protein
The tubulin protein comprises chains: Chain A and Chain B, which contain 440 amino acid and 427 amino acid residues respectively and three ligands Guanosine-5′-triphosphate (GTP) 500 A, Guanosine-5′-diphosphate (GDP) 500B, and Taxotree (TXL) 501B. The two chains were interacting by one salt bridge, six hydrogen bonds, and 298 non-bonded contacts (disulfide bonds) (). Statistics Data in MOLprobity has shown 89.5% favoured rotamers, 15.4% Ramachandran outliers, 71.8% Ramachandran favoured, 4.5% poor rotamers, and −5.72 ± 0.22 Rama distribution Z-score (). VERIFY3D plot revealed that 90.31% amino acid residues had an average 3D-1D score ≥0.2 (). Similarly, ProSA analysis unveiled the Z score value −7.21 which confirmed that the structure was put up in the X-Ray region ().
Figure 1. (a) Ramachandran Plot obtained from MOLprobity for tubulin protein showing favourable and poor rotamers. (b) ProSA plot (number of residues along x-axis and Z-score along y-axis) showing the protein structure (black dot) accommodated in X-ray zone. (c) 3D structure of protein derived from Chimaera showing blue-coloured Chain A and orange-coloured Chain B. The ligands are presented in ball model. (d) Verify3D plot represented the average score for amino acid residues for Chain A lying in between 0 to 0.1 Å. (e) Verify3D plot represented the average score for amino acid residues for Chain B lying in between 0 to 0.1 Å.

Molecular docking analysis
Attempting to characterize biologically potent anticancer agent, in silico studies were conducted on Compounds 1 to 12 (). Compound 1 (–339.98 kcal/mol), compound 2 (–338.16 kcal/mol), compound 11(–378.26 kcal/mol) and compound 12 (-354.53 kcal/mol) are found to be most potent among all derivatives on the basis of binding energy possessed by the docked complex (). The amino acid residues of receptor protein which interacted as H-bond acceptor or donor in case of compound 1 and compound 2 are shown by respective colours in and .
Figure 2. (a) Docked complex of Compound 2 with tubulin protein. The CPK balls represent the ligand and surface view for protein. (b) The zoomed-in view of docked ligand observed in the Biovia Discovery Studio Client. (c) The amino acid residues of protein interacting with Compound 1 shown: Dashed lines of Orange color for electrostatic interactions, green colour for hydrogen bond, purple colour for hydrophobic interaction and cyan colour for halogen bond. (d) H-bond donor and acceptor residues are shown in surface view: purple colour indicates donor and green colour indicates acceptor.
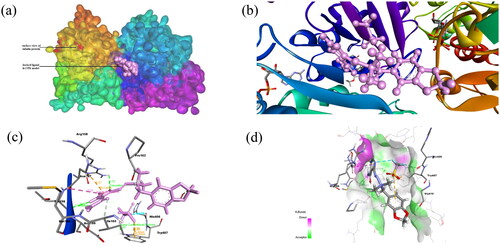
Figure 3. (a) Docked complex of Compound 2 with tubulin protein. The CPK balls represent the ligand and surface view for protein. (b) The zoomed-in view of ligand in docked complex observed in Biovia Discovery Studio Client. (c) The amino acid residues of protein interacting with Compound 2 shown: Dashed lines of Orange color for electrostatic interactions, green colour for hydrogen bond, purple colour for hydrophobic interaction and cyan colour for halogen bond. (d) H-bond donor and acceptor residues are shown in surface view: purple colour indicates donor and green colour indicates acceptor.

Binding interaction studies using molecular docking
PLIP server has shown that Compound 1 interacted with tubulin protein through four hydrogen bonds with Pro162B, Met166B, and Thr198B; pi-cation interactions with Arg158B and halogen bond with His406A (). Similarly, Compound 2 interacted with tubulin protein through three hydrogen bonds with Pro162B, Met166B and Thr198B and hydrophobic interactions with Trp407A (). The amino acid residues involved in non-bond interactions with protein are mentioned in .
Table 2. Binding interactions of ligand with tubulin protein observed in PLIP server.
Pharmacological studies
Among compound 1, compound 2, compound 11, and compound 12 which show good binding energy, compound 11 violates two Lipinski parameters ().
Table 3. Lipinski Parameters observed for all compounds.
For drug design and development, computational approaches enable us to predict the pharmacokinetic properties of the designed compound and to find out the most potent one comparatively [Citation22].
The bioavailability radar obtained from SwissADME () showed that LIPO as XLOGP3 lying within the range of >−0.7 and < +5.0 for all compounds, SIZE as molecular weight in gm/mol lying within the range of >150 and <500 for all compounds except Compound 11; POLAR as TPSA in Å2 lying in the range of >20 and <130 Å2 for compound 5,7,8, 9 and 10; INSOLU in Log (ESOL) lying within the range of >−6 and <0 for all compounds; INSATU as Fraction Csp3 lying within the range of >0.25 and <1, FLEX as number of rotatable bonds lying within the range of >0 and <9 for all compounds.
Figure 4. The Bioavailability Radar displayed for a rapid appraisal of drug-likeness of the compounds considering the parameters lipophilicity, size, polarity, insolubility, insaturation and flexibility.

In the BOILED-Egg model (), compounds which were lying in the grey region indicated that these compounds would not be absorbed by GI and BBB non-permeant. The blue colour of the dot predicted these compounds as P-glycoprotein substrates and could be effluated from the central nervous system. Compound 11 and compound 12 are showing high TPSA which indicated that these compounds did not exhibit good intestinal absorption.
Figure 5. BOILED-Egg Model for designed compound 1 to compound 12 observed in SwissADME web server. The yellow region indicates blood brain barrier permeability, white region indicates human intestinal absorption, the blue colour of dot represents p-glycoprotein substrate.
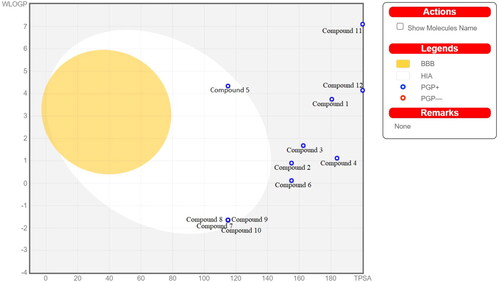
After calculating the ADMET properties (), it was verified that Compound 1 and Compound 2 had a low percentage of human intestinal absorption (43.1% and 48.1%, respectively) (), demonstrating that these compounds might have good oral bioavailability. Caco-2 cell permeability value expressed the gastrointestinal passability [Citation22,Citation40,Citation41] (). The drug delivery and chemical toxicity parameters were affected by the skin which was a barrier between the internal and external body surroundings [Citation42]. The skin permeability parameter value was −2.74 (expressed in log Kp). All these compounds were P-glycoprotein substrate which indicates the active efflux of drugs out of the cell by combining with these membrane-bound transporter [Citation43]. The steady-state volume of distribution (VDss) () defined as a ratio of the total quantity of drug in the body to the total concentration of drug in plasma at steady state conditions [Citation34]. The drug considered to effectively cross the cell membrane when its greater proportion remains unbound to a protein termed as fraction unbound. Compound 1 and Compound 2 were unable to penetrate the CNS (Log PS <−3.0) (). The graph predicted the lower side effects of these compounds in the CNS. It was also observed that Compound 1, Compound 2, Compound 3, and Compound 6 would not cause the inhibition of cytochrome P450, CYP3A4 isoform, the main enzyme responsible for drug metabolism; omitting that there should not be a serious adverse effect due to these compounds.
Figure 6. The prediction results of ADMET properties of ligands (Compound 1 to Compound 12) using pkCSM. (a) The blue colour represents the water solubility in log mol/litre and red colour for Caco2 permeability measured in log papp. (b) The percentage of the compound absorbed through human intestine. (c) The blue colour indicated the VDss measured in log L/kg; red bars for Fraction unbound; green bars represented the blood brain barrier permeability in logBB terms and blue bars for CNS permeability measured in terms of logPS. (d) The parameters for toxicity are shown by coloured bars; blue for maximum tolerated dose, Orange for Oral Rat acute toxicity, grey for Oral Rat chronic toxicity and yellow for minnow toxicity.
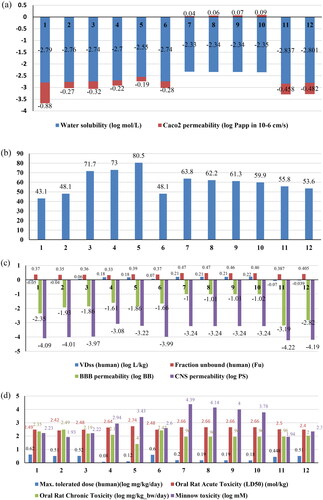
The total clearance parameter of excretion properties was related to bioavailability and important in determining dosage rates to achieve steady-state concentrations. The data from pkCSM demonstrated that Compound 1 and Compound 2 exhibited lower Total Clearance as compared to Compound 5,7,8,9 and 10 values. Thus, these compounds could be excreted slowly by the kidneys and will require longer administration intervals to maintain desired therapeutic concentrations.
The toxicity of the compounds was analyzed through various parameters shown in . The lead compounds would not inhibit the potassium channel encoded by hERG (human ether-a-go-go gene). The blockage of hERG potassium channels lead to a cardiac disorder and sudden death [Citation44].The cardiac toxicity via hERG inhibition assessed by pred-hERG is shown in . The data in the graph verified that these ligands showed hepatotoxic effects. Therefore, 1,3-benzodioxol ligands of better interaction with tubulin might have a physiological or pathological event that could be associated with hepatic injury, suggesting that these compounds cannot be well tolerated by the liver when they are tested in a biological environment. The negative AMES test indicated that these compounds were not mutagenic. The log LC50 value was greater than −0.3 regarding no toxicity. These potent compounds would not cause skin sensitization as analyzed by pkCSM web server.
Conclusion
The derivatives of 1,3-benzodioxole were designed and tested for anticancer activities against tubulin protein (PDB ID: 1TUB) using molecular docking. The ligands are bound with protein near Pro162B, Met166B, Thr198B, Trp407A, and His406A residues through hydrogen bond and electrostatic interactions. Among all, Compound 1, Compound 2, compound 11 and compound 12 showed good binding energy with protein but ADMET properties of compound 1 and compound 2 agreed with the required drug-like properties. The in silico and ADMET studies suggested that Compound 1 and Compound 2 could be potential drug candidates. To ascertain the efficacy of these compounds in treatment would need further confirmatory analysis and validation.
Author’s contributions
Miss Sonaxi and Miss Sangeeta wrote the main manuscript text, prepared all table & figures, performed the molecular docking. Dr. Ravi Tomar made the concept and wrote the main manuscript designed & directed the project and reviewed the manuscript. Dr. Anshul Singh and Prof. Anjana Sarkar made the concept and reviewed the manuscript.
Acknowledgment
Authors would like to thank SGT University, Netaji Subhas University of Technology and Baba Mastnath University for providing instrumentation facility and financial assistance this work. Sangeeta and Sonaxi are thankful to UGC for the award of Junior Research Fellowship.
Disclosure statements
No potential conflict of interest was reported by the author(s).
Data availability statement
The data that support the findings of this research are available upon reasonable request from the corresponding author R. Tomar.
Additional information
Funding
References
- Siegel RL, Miller KD, Fuchs HE, et al. Cancer statistics, 2022. CA Cancer J Clin. 2022;72(1):7–33. doi: 10.3322/caac.21708.
- Jordan A, Hadfield JA, Lawrence NJ, et al. Tubulin as a target for anticancer drugs: agents which interact with the mitotic spindle. Med Res Rev. 1998;18(4):259–296. doi: 10.1002/(SICI)1098-1128(199807)18:4<259::AID-MED3>3.0.CO;2-U.
- Tomar V, Kumar N, Tomar R, et al. Biological evaluation of noscapine analogues as potent and microtubule-targeted anticancer agents. Sci Rep. 2019;9(1):19542. doi: 10.1038/s41598-019-55839-8.
- Amos LA. Microtubule structure and its stabilisation. Org Biomol Chem. 2004;2(15):2153–2160. doi: 10.1039/b403634d.
- Löwe J, Li H, Downing KH, et al. Refined structure of αβ-tubulin at 3.5 Å resolution. J Mol Biol. 2001;313(5):1045–1057. doi: 10.1006/jmbi.2001.5077.
- Singh P, Rathinasamy K, Mohan R, et al. Microtubule assembly dynamics: an attractive target for anticancer drugs. IUBMB Life. 2008;60(6):368–375. doi: 10.1002/iub.42.
- Islam M, Iskander M. Microtubulin binding sites as target for developing anticancer agents. Mini Rev Med Chem. 2004;4(10):1077–1104. doi: 10.2174/1389557043402946.
- Mikstacka R, Stefański T, Różański J. Tubulin-interactive stilbene derivatives as anticancer agents. Cell Mol Biol Lett. 2013;18(3):368–397. doi: 10.2478/s11658-013-0094-z.
- Yong C, Devine SM, Abel A-C, et al. 1,3-benzodioxole-modified noscapine analogues: synthesis, antiproliferative activity, and tubulin-bound structure. ChemMedChem. 2021;16(18):2882–2894. doi: 10.1002/cmdc.202100363.
- Sehrawat H, Kumar N, Tomar R, et al. Synthesis and characterization of novel 1,3-benzodioxole tagged noscapine based ionic liquids with in silico and in vitro cytotoxicity analysis on HeLa cells. JMol Liq. 2020;302:112525. doi: 10.1016/j.molliq.2020.112525.
- Micale N, Zappala M, Grasso S. Synthesis and antitumor activity of 1,3-benzodioxole derivatives. ChemInform. 2003;34(36):2–9. doi: 10.1002/chin.200336096.
- Sangeeta Sonaxi Tomar R, Agrawal S, Sarkar A, et al. 1, 3-benzodioxole tagged lidocaine based ionic liquids as anticancer drug : synthesis, characterization and in silico study. Chemistry Select. 2023;8:1–9.
- Micale N, Zappalà M, Grasso S. Synthesis and cytotoxic activity of 1,3-benzodioxole derivatives. Note II. Farmaco. 2003;58(5):351–355. doi: 10.1016/S0014-827X(03)00053-3.
- Jurd L, Narayanan VL, Paull KD. In vivo antitumor activity of 6-benzyl-l,3-benzodioxole derivatives against the P388, L1210, B16, and M5076 murine models. J Med Chem. 1987;30(10):1752–1756. doi: 10.1021/jm00393a012.
- Kumar N, Tomar R, Pandey A, et al. Preclinical evaluation and molecular docking of 1,3-benzodioxole propargyl ether derivatives as novel inhibitor for combating the histone deacetylase enzyme in cancer. Artif Cells Nanomed Biotechnol. 2018;46(6):1288–1299. doi: 10.1080/21691401.2017.1369423.
- Sehrawat H, Kumar N, Sood D, et al. Mechanistic interaction of triflate based noscapine ionic liquid with BSA: spectroscopic and chemoinformatics approaches. J Mol Liq. 2020;315:113695. doi: 10.1016/j.molliq.2020.113695.
- Sehrawat H, Kumar N, Sood D, et al. Unraveling the interaction of an opium poppy alkaloid noscapine ionic liquid with human hemoglobin: biophysical and computational studies. JMolLiq. 2021;338:116710. doi: 10.1016/j.molliq.2021.116710.
- Alshitari W. Investigating the binding measurements of human α-acid glycoprotein with chlorambucil and dacarbazine in the presence of imidazolium based -ionic liquid by affinity capillary electrophoresis. Arab J Chem. 2020;13(10):7445–7452. doi: 10.1016/j.arabjc.2020.08.020.
- Tagne JB, Kakumanu S, Nicolosi RJ. Nanoemulsion preparations of the anticancer drug dacarbazine significantly increase its efficacy in a xenograft mouse melanoma model. Mol Pharm. 2008;5(6):1055–1063. doi: 10.1021/mp8000556.
- Al-Badr AA, Alodhaib MM. Dacarbazine. Profiles Drug Subst Excip Relat Methodol. 2016;41:323–377. doi: 10.1016/bs.podrm.2015.12.002.
- Li AP. Screening for human ADME/tox drug properties in drug discovery. Drug Discov Today. 2001;6(7):357–366. doi: 10.1016/s1359-6446(01)01712-3.
- Mvondo JGM, Matondo A, Mawete DT, et al. In silico ADME/T properties of quinine derivatives using SwissADME and pkCSM webservers. IJTDH. 2021;42:1–12. doi: 10.9734/ijtdh/2021/v42i1130492.
- Kar S, Leszczynski J. Open access in silico tools to predict the ADMET profiling of drug candidates. Expert Opin Drug Discov. 2020;15(12):1473–1487. doi: 10.1080/17460441.2020.1798926.
- Rose PW, Bi C, Bluhm WF, et al. The RCSB protein data bank: new resources for research and education. Nucleic Acids Res. 2013;41(Database issue):D475–D482. doi: 10.1093/nar/gks1200.
- Elokely KM, Doerksen RJ. Docking challenge: protein sampling and molecular docking performance. J Chem Inf Model. 2013;53(8):1934–1945. doi: 10.1021/ci400040d.
- Williams CJ, Headd JJ, Moriarty NW, et al. MolProbity: more and better reference data for improved all-atom structure validation. Protein Sci. 2018;27(1):293–315. doi: 10.1002/pro.3330.
- Laskowski RA, Jabłońska J, Pravda L, et al. PDBsum: structural summaries of PDB entries. Protein Sci. 2018;27(1):129–134. doi: 10.1002/pro.3289.
- Luthy R, Bowei J, Einsenberg D. Verify3D: assessment of protein models with three-dimensional profiles. Meth Enzymol. 1997;277:396–404.
- Wiederstein M, Sippl MJ. ProSA-web: interactive web service for the recognition of errors in three-dimensional structures of proteins. Nucleic Acids Res. 2007;35(Web Server issue):W407–410. doi: 10.1093/nar/gkm290.
- Cousins KR. Computer review of ChemDraw ultra 12.0. J Am Chem Soc. 2011;133(21):8388–8388. doi: 10.1021/ja204075s.
- Mendie LE, Hemalatha S. Molecular docking of phytochemicals targeting GFRs as therapeutic sites for cancer: an in silico study. Appl Biochem Biotechnol. 2022;194(1):215–231. doi: 10.1007/s12010-021-03791-7.
- Daina A, Michielin O, Zoete V. SwissADME: a free web tool to evaluate pharmacokinetics, drug-likeness and medicinal chemistry friendliness of small molecules. Sci Rep. 2017;7(1):42717. doi: 10.1038/srep42717.
- Pires DEV, Blundell TL, Ascher DB. pkCSM: predicting small-molecule pharmacokinetic and toxicity properties using graph-based signatures. J Med Chem. 2015;58(9):4066–4072. doi: 10.1021/acs.jmedchem.5b00104.
- Yeni Y, Rachmania RA. The prediction of pharmacokinetic properties of compounds in hemigraphis alternata (burm.F.) T. Ander leaves using pkCSM. Indones J Chem. 2022;22(4):1081–1089. doi: 10.22146/ijc.73117.
- Tripathi P, Ghosh S, Nath Talapatra S. Bioavailability prediction of phytochemicals present in calotropis procera (aiton) R. Br. by using Swiss-ADME tool. World Sci News. 2019;131:147–163.
- Jyothi R, et al. Swiss ADME prediction of phytochemicals present in Butea monosperma (lam.) taub. J Pharmacogn Phytochem. 2020;9:1799–1809.
- Daina A, Zoete V. A BOILED-Egg to predict gastrointestinal absorption and brain penetration of small molecules. ChemMedChem. 2016;11(11):1117–1121. doi: 10.1002/cmdc.201600182.
- Braga RC, Alves VM, Silva MFB, et al. Pred-hERG: a novel web-accessible computational tool for predicting cardiac toxicity. Mol Inform. 2015;34(10):698–701. doi: 10.1002/minf.201500040.
- Danker T, Möller C. Early identification of hERG liability in drug discovery programs by automated patch clamp. Front Pharmacol. 2014;:203. doi: 10.3389/fphar.2014.00203.
- Firdausy AF, Muti’ah R, Rahmawati EK. Predicting pharmacokinetic profiles of sunflower’s (helianthus annuus L.) active compounds using in silico approach. JIM. 2020;4(1):1–7. doi: 10.18860/jim.v4i1.8840.
- Azzam KA,. SwissADME and pkCSM webservers predictors: an integrated online platform for accurate and comprehensive predictions for in silico ADME/T properties of artemisinin and its derivatives. KIMS/CUMR/MShKP. 2023;11; 325(2):14–21. doi: 10.31643/2023/6445.13.
- Pecoraro B, Tutone M, Hoffman E, et al. Predicting skin permeability by means of computational approaches: reliability and caveats in pharmaceutical studies. J Chem Inf Model. 2019;59(5):1759–1771. doi: 10.1021/acs.jcim.8b00934.
- Psimadas D, Georgoulias P, Valotassiou V, et al. Molecular nanomedicine towards cancer. J Pharm Sci. 2012;101(7):2271–2280. doi: 10.1002/jps.23146.
- Mitcheson JS. hERG potassium channels and the structural basis of drug-induced arrhythmias. Chem Res Toxicol. 2008;21(5):1005–1010. doi: 10.1021/tx800035b.