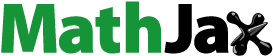
ABSTRACT
Management of variability in raw materials is critical in food manufacturing systems, where the variability of input attributes is a result of its biological nature. We present a methodology for the analysis of such production systems that provides a means for assessing tactical and strategic issues. To illustrate our method we partner with a large food manufacturer whose primary raw material is wheat flour to examine how variation in raw material attributes and the use of manufacturing controls impact the firm's finished goods quality and net revenue outcomes. At the strategic level, we investigate the supplier selection problem where the firm must choose which supplier criterion to emphasize: lower price or higher quality. We find that the firm's operations fall short of achieving optimal net revenue, which would represent an increase of 65%. We also show that flour price should be the driving force in supplier selection for our firm..
1. Introduction
Raw material variability is a critical factor to manage in the design of supply chains and production operations management systems. In some contexts, this may mean quality control programs for testing inputs to ensure they conform to production system requirements. In other situations, the burden of quality assurance may be shifted upstream to input suppliers, incorporating input quality management in the supply chain via contracting. In other contexts, even acceptable quality inputs may require adjustments to production controls in response to the input quality measures. Some of the inputs in food manufacturing are inherently variable because of the biological nature of their production process and a lack of perfect control of the production environment due to weather, climate, and potential pest infestations. This variability can span a number of attributes of the inputs, including moisture, nutritional properties, manufacturing properties, and so on. Managing this variability at the supplier level may be prohibitively expensive or impossible. For this reason, food manufacturing production systems are often designed with flexibility in mind, allowing for process controls to be adapted on the fly so as to compensate for inputs with attributes, or characteristics (terms we use interchangeably throughout our paper), that are not at ideal levels.
The food industry is large. In 2017 in the United States, it had revenues over $650 billion (Census Bureau, Citation2017), from which the snack food industry generated approximately $39 billion in revenues. Globally, the snack food industry is growing, and it weathered the economic downturn due to the global Covid-19 pandemic with increasing revenue as food consumption patterns changed toward food-at-home (Roesler, Citation2020). In the US, the snack food production market size between 2017 and 2022 increased by 0.5% each year (Snack Food Production in the US, Citation2022). The most common raw material for the snack food industry is wheat flour, where snack food based on wheat flour is an important component of daily consumption for many people. Flour is used in both healthy and indulgent snacks, and even as consumer trends are favoring more healthy eating, flour will remain a critical component of diets globally. Flour is also a common agricultural raw material, grown and processed globally, with variable attributes. Because food manufacturers typically rely on high volume production with low margins, improvements in efficiency can have large impacts on firms’ profits.
We partner with a large snack food manufacturing firm whose primary ingredient is wheat flour and whose primary finished good is a salty flour-based snack. The firm operates multiple plants and has sales over $2 billion per year, making it one of the largest firms in the industry. The largest raw material cost faced by our partner firm is wheat flour, which typically comprises more than 95% of the total ingredient cost of the finished goods. Wheat flour has four important characteristics for producing snack foods: moisture, protein, ash content, and mixing tolerance index (MTI). MTI is a measure of the flour’s quality, particularly related to mechanical handling; the lower the number, the higher the quality of the flour and the more robust it is to handling. The flour is stored at the manufacturing facility for less than 48 hours prior to use, and it is never blended on site. This means that each batch of flour is unique, and because the millers provide testing information by batch, its attributes are known at the time it is used in the manufacturing process. Each time the firm’s operations team manufactures a batch of finished goods, they must adjust the manufacturing controls to accommodate the flour characteristics and achieve high quality finished goods. This presents a challenge to the firm that is shared by other firms in the industry.
We study the firm’s manufacturing process for a specific packaged snack food at a dedicated production line in a large manufacturing facility. The firm’s operations team is responsible for manufacturing snacks 24 hours per day, over 360 days per year. Each day, the facility uses hundreds of thousands of pounds of flour delivered by three flour millers. The millers (suppliers) deliver flour in trucks containing between 40 and 50 thousand pounds each, multiple times per day. At delivery, the suppliers provide measurements of the flour attributes, from which we can observe that each miller consistently delivers flour with different average attribute levels and attribute variances.
The firm’s flour sourcing strategy routinely utilizes all three suppliers, where each miller stands out for a specific feature: miller 1 has the lowest cost flour; miller 2 provides the smallest flour attribute dispersion for the two most critical attributes, moisture and protein; and miller 3 provides the highest flour quality as measured by low average moisture and high average protein. See, for summary statistics by each supplier. The firm would like to know if prioritizing a specific miller would be beneficial. No supplier capability issues or demand uncertainties other than flour attribute variability have been indicated by the firm. Effectively, plant managers state that there are no problems of flour shortages and that ‘the plant can effectively sell everything it manufactures.’ The firm works with long-term fixed-price contracts with each millers, so flour prices are fixed.
Table 1. Flour attribute, manufacturing control, and quality summaries
Table 2. Flour quality descriptive statistics by supplier
The finished product is tested for moisture to ensure it falls within an appropriate range for quality. If it passes this quality test it is considered a premium product, and it is packaged and put in inventory for distribution. If it is too wet or dry, it is considered non-premium. Non-premium finished goods are either sent through secondary distribution channels, such as factory or dollar stores, or can be reworked – i.e. reused in the manufacturing process as filler in other products.
Given the context provided above and based on discussions with the firm’s managers, we pose the following questions:
Is an operational focus on maximizing product quality aligned with a higher level strategic focus based on maximizing net revenues?
Is the firm currently achieving its potential expected net revenues and if not what should be the changes to implement in the manufacturing process towards this goal?
What is the impact of supplier selection on flour supply quality, finished good quality and the firm’s profitability?
These three points are examples of analyses that can be done using our general method. Step 3 of our method can be used to address the firm’s first two tactical points above, and Step 4 can address the third strategic point.
1.1. Main contributions
Our unique data set and our analytical approach allow us to make the following two principle contributions. First, we propose a data-driven analytical method designed to identify the optimal management of manufacturing controls given raw material variability. This method, and its regression analysis and optimization modeling details, is general and flexible and can be applied to a broad set of firms that manufacture products using raw material with variable attributes and whose manufacturing controls can be easily adjusted. In the food industry, wheat-based products such as baked goods or pastas or the production of vegetable seeds (Chen et al., Citation2018) are processes that could be studied using our method. Industries beyond food manufacturing that face similar challenges include pharmaceuticals (Plante et al., Citation1999), the semiconductor silicon wafer industry, and materials manufacturing such as steel, paper, or glass (for which raw materials are mainly composed of a single input recycled material). Second, we empirically demonstrate the application of the method to a specific firm’s case. In doing so, we demonstrate the data development, construction of a production model relating input attributes and controls to system performance, tactical control optimization based on the production model, and evaluation of strategic design decisions.
2. Literature review
We briefly address three strands of literature: food operations management, raw materials variability, and input-output process estimation methods. We start by reviewing academic and practitioner literature in the Operations Management (OM) and Agribusiness areas, finding that there is substantial scope for continued work on operational decision-making in the food and agribusiness industries (Donk & Fransoo, Citation2006; Hernandez et al., Citation2020). Specifically, there is little work of which we are aware that addresses raw material variability in food manufacturing at an operational level.
2.1. Food operations management
The literature on food operations management is considerable on certain topics. A large body of literature covering the food and agricultural industries relates to perishability and uncertainty. There is a variety of high-quality literature reviews addressing these topics, such as deteriorating inventory (Bakker et al., Citation2012; Goyal & Giri, Citation2001), agribusiness supply chain risk management (Behzadi et al., Citation2018; Wang et al., Citation2012), agrifood supply chain planning models (Ahumada & Villalobos, Citation2009; Glen, Citation1987; Lowe & Preckel, Citation2004), and location analysis (Lucas & Chhajed, Citation2004).
Some gaps identified by the literature reviews that were subsequently filled include work on food and agricultural deterioration challenges within supply chains (Akkerman et al., Citation2010; Rong et al., Citation2011) and operational models integrating production and distribution systems (Azoury & Miyaoka, Citation2013; Devalkar et al., Citation2011; Kopanos et al., Citation2012). Recent work has also focused on challenges associated with fresh food supply chains (Blackburn & Scudder, Citation2009; Cai et al., Citation2010) and there is also a well-developed body of literature regarding food cold-chains (Shashi et al., Citation2018). Additionally, the study of sustainability in food supply chains (Genovese et al., Citation2017; Zhu et al., Citation2018).
Some literature in food manufacturing has focused on process efficiency. The most relevant works are the study of advanced process controls in the food industry (Haley & Mulvaney, Citation1995) and the recent book that oversees food processing for different types of food (Varzakas & Tzia, Citation2015). Moreover, a recent debate has started about digitalizing food manufacturing and the whole food supply chain (Bronson & Knezevic, Citation2016).
2.2. Raw materials variability
Work addressing the specific relationship between raw material and food processing covers issues such as raw material pricing, raw material processing, controls in manufacturing, contracts, planning, scheduling, and distribution. Before we delve into studies in the food processing sector, we highlight relevant studies in raw material variability in OM. The management of production processes in the presence of raw material supply uncertainty is an important problem in OM (Mundi et al., Citation2019; Yano & Lee, Citation1995). However, most of the focus has been on providing optimal ordering and production-level solutions from stylized analytical models that treat the production process as a black box (e.g. Bassok and Akella (Citation1991)). Our proposed method studies the capability of the manufacturing controls to absorb raw material variability based on a data-driven approach that aims to characterize the production process. Of particular relevance to our work is a paper by Plante et al. (Citation1999) where a pharmaceutical manufacturer exploits variability in raw materials to improve quality performance. A regression equation is used to drive a loss function, which is optimized in a matching process, an approach that is similar to ours.
Food processing and raw material management literature, with some similarity to the work presented here, covers pork packing firms. For example, Boland et al. (Citation1995) use a multivariate simulation to characterize raw material variability in the attributes of market hogs. They recommend that packers focus on procuring high quality (lean) raw material in order to increase the quality of the finished goods. Additional work covers the development of a distribution model alongside an MRP system to manage a firm’s variability in grape harvesting while minimizing costs (Schuster & Allen, Citation1998) and the study of raw material quality’s impact on refiner costs in the sugar beet industry (Boland & Marsh, Citation2006). There is also a growing body of work on food and agriculture planning and distribution problems, such as challenges faced by multi-site, multi-product, continuous process manufacturers (Kopanos et al., Citation2012), harvest risk control (Allen & Schuster, Citation2004), harvest planning (Bohle et al., Citation2010), harvest, packing, and distribution with perishability (Ahumada & Villalobos, Citation2011), and capacity investment decisions by processors using agricultural raw materials to produce a commodity output with a byproduct (Boyabatlı et al., Citation2017).
2.3. Input-output process estimation
Our analysis is structured in four stages, which integrate the creation of two models. The first is a data-driven model that should reflect the manufacturing process as accurately as possible and the second model should use this estimated system to optimize the process by determining the best manufacturing controls given input. Some scholars have called this type of analysis ‘system optimization’ or ‘input-output modeling optimization’. Different techniques have been used for each model, where the first model that consists of the input-output process estimation is the most challenging and interesting part. Thus, for this first model, regression analysis and regression metamodels are the most popular approaches, where regression metamodels consist of modeling a regression equation from data obtained by a simulation model (Tew & Wilson, Citation1994). Other techniques are in the area of machine learning, which relies heavily on data when there is very little knowledge of the manufacturing process. An example is the use of neural networks (Funes et al., Citation2015). When multiple input variables seem to affect output performance, ‘response surface methodology’ is a popular input-output modeling technique, especially in product design (Myers et al., Citation2016). Due to the fact that we have a simple input structure (i.e. a unique input variable displaying quality variability), limited data, and visibility and knowledge of the manufacturing process, our approach for the first model is to use a second-order regression model. For our second model, we use a simple constrained nonlinear optimization model.
3. General data-driven analytical methodology
Inspired by the points raised by our wheat-based snack manufacturing partner, we suggest a general data-driven analytical approach that can be utilized by many firms facing raw material variability and manageable manufacturing controls. The general steps of this process are illustrated in and described below, and the details of each step are presented in the next Section.
In step 1, it is essential to begin the analysis by collecting data on the performance of the production system that includes measures of both input and output qualities, the settings for production system controls, and to the extent possible, other relevant environmental factors that may impact output quality, such as ambient temperature, humidity, and so on. In step 2, a production model is developed that represents the relationship between input quality, controls and environmental factors (the independent variables), and output quality (the dependent variable or prediction goal). These models range from simple regression, to multinomial logit and probit models, other econometric models (see, e.g., Greene (Citation2003)) and supervised machine learning algorithms (Mullainathan & Spiess, Citation2017).
In step 3, an economic objective (e.g. expected revenues net of variable costs) can be optimized with respect to the controls for given levels of input quality and environmental factors to obtain optimal conditional controls. To the extent that there are uncertainties in the relationship between independent variables and output quality, these will be reflected in the output quality distribution, and an economic value function can be used to convert this distribution into expected revenue. Subtracting the costs associated with controls and input acquisition, we obtain the expected net revenue objective. This represents the ideal behavior of the operators of the production system.
In step 4, this ideal model of the production system is used to test the efficacy of various strategic changes to the input quality assurance program, environmental controls, and supply chain design. For example, if input quality specifications are changed, this may have an impact on the distribution of input quality, which in turn may have an effect on the distribution of output quality. Combining the changes in costs of the input quality assurance program and the impact on output quality allows assessment of these tradeoffs.
4. Application to a snack food manufacturing case
is a diagram of the manufacturing process for our partner firm, from raw material inputs to finished goods. We focus on five manufacturing controls: added water, mix time, extruder pressure, added salt, and kiln speed. In the case of our partner firm, flour cost comprises over 95% of the direct raw material cost of the finished good.
The manufacturing process starts at the mixing stage, where the operations manager determines how much water to combine with the flour and additional ingredients to make the dough. The quantities of flour and additional ingredients, except water and salt, are fixed by the recipe for any given product on a given production line. Water quantity is included in the recipe, but varies considerably batch-by-batch and is one of the key decisions an operator makes from their own experience and knowledge of the flour characteristics. After adding the water, the operator determines the mix time for the dough. After mixing, the dough is extruded and cut. The extruder shapes the snack product and the cutter separates one piece of product from another. The product then proofs on a conveyor belt before traveling under a salter. Once salted, it travels through an oven. In our model, we treat the oven temperatures as fixed between batches due to the impracticality of adjusting them batch by batch. The final oven zones are configured for drying (the kiln zones) and the speed at which the products are sent through (the kiln speed) is controllable. After drying, the finished product is sent to the packaging line. For some controls, the values in our data set are invariant – for example, the cutter and proofing speeds are fixed. For other controls, such as the kiln speed and extruder pressure, the data displays considerable variation.
Flour attributes interact with the controls in several ways. For example, an operator (a baker) might physically ‘feel’ the dough’s strength, to determine if it needs more or less water. If the dough is assessed to be too dry or too wet after mixing, the pressure of the extruder or the speed of the product through the drying kiln can be adjusted to compensate. If the mixing tolerance index (MTI) is high, the dough might be mixed for less time to compensate for lower mixing stability. Ash can affect water absorption, and the amount of water added during the mixing stage or in the amount of drying time needed at the kiln stage may be adjusted to compensate. We consider these interactions in developing a model to provide insights into the snack food manufacturing process.
As finished goods are completed, moisture is measured. The moisture level must be between upper (4%) and lower (3%) bounds, and ideally at 3.5%, in order to package the good. If the product is too wet, it could spoil, while if it is too dry, it will not be acceptable to consumers for aesthetic reasons. Moisture is the most challenging finished goods attribute to control and the one the firm and operators struggle with the most; out-of-bound moisture results in the majority of discarded materials and impacts other non-measured attributes, such as color. If a finished goods batch meets the criteria for packaging, it is packaged as ‘premium’ product and added to inventory for delivery to retailers. If the product does not meet the criteria, it has four potential destinations: filler material in an alternate product; sale as is via an alternate retail distribution channel, such as dollar or factory stores; sale as animal feed; or trash. In the data sets, we use, non-premium products virtually always have some residual value. For modeling purposes, we lump the outcomes of a batch into two categories – premium and non-premium and assign appropriate revenues to each. We set the value of ‘premium’ finished goods using confidential data provided by our partner firm and use a value of half that for ‘non-premium’ finished goods, reflecting the difference in marginal revenue between the two finished goods categories. In the Appendix A1.2, we conduct a robustness test on the price difference between premium and non-premium finished goods.
4.1. Step 1: data development
Data collection took place at a large, multi-product manufacturing facility in the United States whose primary raw material across all product lines is wheat flour. As mentioned earlier, the facility sources its flour from three local flour millers. We base our analysis on three data sets described below, where the specific elements contained in the data sets are summarized in .
Flour reports: Each observation is for a truckload of flour and includes measurements of the moisture, protein, ash, and mixing tolerance index (MTI), as well as the supplier identifier (miller), the date of delivery, and bill of lading number. Moisture, protein, and ash are measured as percentages by weight. MTI is measured in Brabender Units using a farinograph; a device used to measure various characteristics of flour. MTI is a measure of how well flour can tolerate mixing, and lower numbers typically indicate higher quality and flour that produces dough that can be mixed longer without breaking down. In general, lower moisture and ash content, and higher protein content indicate higher-quality flour. Our flour data spans a longer period than the manufacturing process data and contains 1,686 total observations (1,619 complete observations after deleting observations with missing data) of flour deliveries between 1 August 2016 and 31 May 2017. Deliveries are made by three suppliers multiple times per day. Summary statistics of this data are reported in .
Production data: Each observation is for a single batch of dough. The data is in the form of a handwritten data sheet that indicates the truckload of flour (and indirectly the flour attributes) and the control settings used to process the flour into finished product. These data span roughly 5 months (1 January 2017 to 31 May 2017). To limit the impact of omitted variables, we focused on a single snack product and a single production line.
Finished goods quality: Final moisture measurements were taken directly before packaging to determine whether the finished goods were premium or non-premium. Finished goods testing was not performed for every batch, but at a fixed time interval of every 30 minutes. At the average kiln speed, the manufacturer produces three batches per hour; so about two-thirds of the production batches could be associated with a final goods quality classification. The finished goods moisture test data included a time-stamp, production line number, and product.
We had a total of 1,219 useable observations for production batches available across all products and production lines. However, we decided to focus on one specific baked product out of the seven different types of products produced in that facility, and we focused on a single, dedicated production line for that product. Furthermore, we eliminated a few observations for batches that could not be identified and matched across the three data sets for flour, production, and finished goods quality. The final data set assembled for the analysis included 150 complete observations for the identified manufacturing line and product that consisted of complete matches of the same batch of each one of the three datasets: flour attribute data, production control settings, and finished goods moisture, so a cause-effect link can be studied. Summary statistics of these data are reported in . The anonymized data sets used in the paper can be shared upon request.
Table 3. Descriptive statistics of manufacturing controls
4.2. Step 2: production model creation
The goal of the production model is to relate the raw material characteristics and manufacturing controls to finished goods moisture (). Frequently, the recipe or controls change between batches to accommodate differences in the flour characteristics. Typically, a manufacturer uses a fixed quantity of flour and alters the amount of added water and salt to accommodate the protein, ash, moisture, and MTI characteristics of the batch of flour. The manufacturer might also alter the manufacturing controls, such as mixing time, extruding pressure, baking temperatures, or drying time (equivalent to kiln speed).
Due to the fact that we have visibility and knowledge of the food manufacturing process, we employ a second-order regression model to estimate this relationship. The goal of our analysis is not restricted solely to the prediction of output quality, where machine learning methods may have an advantage, but to the estimation of the impact of flour quality and controls on the distribution of output quality. Multiple estimation methods were considered and tested, and A1.1 of the Appendix provides more details on a few final candidate model specifications and an out-of-sample prediction test that we develop to help select our production model.
We build on the previous work by incorporating controls in our regression, not dissimilar to some work that examines methods that study the impact of flour attributes in baking (Goesaert et al., Citation2005). Our goal is to express the relationship between the final moisture of the finished goods and the given set of raw material characteristics and controls. This relationship along with the distribution of the error term can be used in Step 3 to express the probability that the finished goods batch will be premium or non-premium, i.e. whether final moisture will be between the required upper and lower bounds on final moisture. The regression model that we employ has the following general form:
where is the finished goods moisture of observation
,
is the level of the
flour attribute for observation
,
is the setting value of control
for observation
, and
is an error term that is assumed to be normally distributed. We consider a month fixed effect where
is the binary variable for the month
in which the flour from observation
was delivered. We have five months in our sample, January through May, and use May as the base month. We consider this to control for unexplained variables that are not possible to collect or observe, such as seasonal changes in the wheat quality or the monthly average humidity in the manufacturing facility, which we would also expect to change seasonally. We estimate the coefficients (
,
,
,
,
,
,
,
, and
) using ordinary least squares because it provides point predictions of final product moisture. The specific model presented in Section §5.1 reflects the observed manufacturing system by considering all independent variables (i.e. attributes and controls) selectively as first order, second order, or cross-product terms. The specific regression model is selected based on an exhaustive search that combines all possible terms and considers two goals: to maximize fit (by selecting the highest adjusted
) and to maximize predictive power as explained in the Appendix A1.1. These two goals are equally important in the context of the general data-driven analytical method that we are proposing because we not only are trying to find the distribution that best fits the production setting but we also want to find a model that is capable of predicting with high precision the quality outcome (premium or non-premium) of each batch of finished goods that are being produced.
Table 4. Estimated regression coefficients
4.3. Step 3: tactical control optimization model
From Equationequation (1)(1)
(1) we define the function
, which we use to express the probabilities of three regions of interest based on the upper and lower bounds of the finished goods moisture,
and
, respectively. The three regions are:
Each probability gives the likelihood that the realized moisture level, , lies within the relevant region. The realized moisture level is equal to the sum of the point prediction (
) and the normally distributed error term (
). The point prediction of the moisture level of the finished goods is in turn a function of flour attributes (
), manufacturing controls (
), and estimated coefficients from Equationequation (1)
(1)
(1) . Thus, these probabilities can be written as follows:
We use the estimated regression to define the bounds on the error term which is assumed to have a normal distribution with mean zero and constant variance, which is approximated by the sample variance of the estimated errors. These probabilities are functions of the flour attributes and manufacturing controls for each batch of flour. We next present a quality maximization model.
4.3.1. Quality model For each batch of flour, manufacturing controls are optimized given the observed flour attributes. The model objective is to maximize the probability that the finished goods are in the premium region. Objective (4) and constraints (5) compose the quality maximization problem for the -th batch (
):
where the limits of integration for the normal density are from to
, yielding the probability that the realized final moisture is in the premium range
.
is the total number of observations,
is the estimated finished goods moisture for batch
,
is the sample variance of the regression errors,
is the upper bound for control
, and
is the lower bound for control
. Constraints (5) impose upper and lower bounds on the controls
, with
and
denoting the fixed upper and lower bounds, respectively.
4.3.2. Net revenue model We also formulate an expected net revenue maximization model that incorporates prices and costs, and shifts the objective from a per batch basis to a per unit of time basis. The integrals from the objective for the quality model are used to express the probabilities that the batch is premium or non-premium, which are in turn multiplied by the per batch price of premium or non-premium product, respectively, to obtain expected revenue for the batch. We then subtract the costs for water, salt, and flour to obtain a net revenue per batch. Finally, we multiply by one over the cycle time for the batch to convert the objective to net revenue per unit of time, yielding an objective that is equivalent to maximizing annual returns (Burt, Citation1978). We do this by calculating the batches per hour that are produced while treating the kiln speed as the bottleneck in the process. Management indicates that the kiln is the bottleneck of the manufacturing process and that a faster kiln speed leads to increased throughput.
The net revenue maximization model accounts only for direct costs of all control variables. That is, we do not include labor or overhead costs. The manufacturing firm operates the plant and production lines continuously, spreading overhead across all batches. That is, labor and overhead are effectively fixed, not variable costs, and have no impact on the results of the maximization problem. We also note that the firm can market and sell all of the premium finished goods they can produce at full price. Thus, we do not have demand constraints in our problem. Objective (6) and constraints (7) compose the expected net revenue maximization problem for the -th batch (
):
s.t.
where and
are defined as in the previous problem,
is the premium finished goods price per batch,
is the non-premium finished goods price,
is the flour quantity per batch (constant)
is the flour price for batch
,
is the cost vector for the vector of control variables
,
is the specific control variable for the control kiln speed, and
is a factor that converts kiln speed to batches per hour.
In this problem, revenues are attributed to all three regions – premium, too dry, and too wet, with different prices for premium and non-premium batches. In (6), the integrals times prices collectively represent expected revenues from which the direct costs of flour and controls in dollars for the batch are subtracted. Thus, the term in braces represents the net revenue per batch. The kiln control has a direct cost of zero because it is virtually costless to adjust the kiln speed. However, the opportunity cost of the time spent in the kiln zone is a key determinant of throughput. Slowing the kiln down reduces throughput, and speeding it up increases throughput. We multiply net return per batch by the kiln speed () times a constant kiln factor, which converts the objective to net revenues per hour.
Problem () allows us to identify the expected net revenue per hour maximizing controls for any given batch given the flour attribute levels. In the next section, we assess the optimal controls and sourcing decisions using our firm’s data.
4.4. Step 4: strategic decisions
The firm is interested in assessing the best supplier selection strategy given that there are three suppliers, where miller 1 is the lowest cost, and of the two higher cost suppliers, miller 2 provides the smallest flour critical attribute (moisture and protein) dispersion, and miller 3 provides the highest average quality based on critical attributes. The flour data set contains 1,619 observations of attributes for deliveries to the firm, and is stratified by miller and treated as three empirical distributions of the flour attributes. Combining these miller-specific attribute distributions, optimal batch controls calculated from , and observed flour prices, we estimate long-term expected hourly net revenues for each miller.
5. Numerical results and managerial insights
This section details our numerical results. Section § 5.1 presents the results of the regression model (Step 2 of the framework) used to predict the distribution of final product moisture as a function of flour attributes and controls, which drives the probability of obtaining premium product for the () and (
) models. Section § 5.2 presents the results of these two problems (Step 3). Finally, Section § 5.3 uses the net revenue problem with our empirical flour data set stratified by miller to assess the miller selection problem from an expected net return perspective (Step 4).
The data analytics and data development for all steps were performed in R and SAS. The regression analysis was performed in R, and our mathematical programming solutions were obtained using the GAMS mathematical programming language with the CONOPT solver.
5.1. Production model estimation results
display the results of the regression model selected, including Shapiro–Wilk and Jarque–Bera tests for normality of the residuals. For both tests, the null hypothesis of normal residuals cannot be rejected. The specification achieves an adjusted R-squared of 0.314, which is the highest value obtained of all the regression scenarios studied, and a high predictive power of 92.7%. The selected regression model is the best model according to two goals: best fit and highest predictive power as explained in the Appendix A1.1. Additionally, we note that for our specific application we include a total of 150 observations, which limits our total number of regressors to a recommended maximum of 15. We note that the adjusted R-squared values obtained from our model, and all models tested, reveal that there is substantial variation that is not explained by the model’s regressors. This might be due to a number of factors including limited measurement precision and recording errors, rounding of the dependent and independent variables during recording, and unobservable variables (e.g. humidity and temperature of the facility).
Table 5. Estimated regression distribution tests
Next, we provide a more in-depth description of some of the terms included in our model. In our analysis, we have used monthly dummies (Month1 through Month4) to capture unobserved variables that may correlate to seasons or changes in wheat attributes correlated with time in storage that affect properties beyond our measured attributes. In this regard, we find that Month4 (April) is statistically significant relative to May, the base month, and it is included in the model as a first-order term. Ash squared is the only second-order term that is included in our model, and it is significant at the level. There are several interaction terms included between different flour attributes, flour attributes with controls, and between different controls. For example, flour moisture and water are included and highly significant, which is not surprising given the easy substitution between these inputs for determining dough moisture. Zone1 through Zone4 are the oven temperatures for the four oven zones. We are not including these variables as control variables for our optimization problems because it is not practical to adjust oven temperatures between batches; however, we include them in this analysis because they have significant effects and do vary within our dataset. The linear terms for zones 2, 3, and 4 are significant, and we also include the interaction term between zones 3 and 4, which is statistically significant at the
level. The kiln speed and zone 4 temperature interaction is also significant at the
level. We interact the kiln speed with the final oven zone because the kiln temperature is set by oven zone 4. In our optimization problem, we use fixed values for Zone1 to Zone4 temperatures, which are set to temperatures specified by the recipe.
5.2. Quality and net revenue maximization problems
Results indicate that any net revenue maximizing solution for our production data set also has quality at its maximum possible level, with expected final moisture precisely in the middle of the premium range. However, maximum quality does not necessarily imply maximum net revenues per hour. It appears there are many ways to make premium finished goods even for the same levels of flour attributes. This is consistent with our observations of the production data, where we find the firm’s operations team making premium finished product consistently with different control settings for similar or even identical flour batches. However, controls for the net revenue maximization solution appear to be more restricted. That is, for any batch of flour, if we constrain net revenue to its maximum value and attempt to change any of the controls while allowing all other controls to vary within the feasible region, no feasible changes are obtained other than those in which optimal mix time and extruder controls are interior point values (i.e. strictly between their lower and upper bounds). In such cases, there is some room to trade-off between these two virtually costless controls (for confidentiality reasons, we are not disclosing the specific values of the cost of the controls).
Remark 1. Operational managers (bakers) might have multiple ways to produce premium finished goods, but only a very limited latitude to produce maximum net revenues for a particular batch.
Our partner firm’s operations team confirmed that trade-offs in the manufacturing controls allow for multiple ways to produce premium finished goods for a given batch of flour. However, our numerical experiments indicate that there are few ways to maximize net revenue for a particular batch of flour.
In regard to the firm’s second point related to assessing whether the firm is achieving maximum net revenues, reports three columns of results. The first is the ‘Observed’ column that contains the means and standard deviations of controls, the measured final moisture, the predicted likelihood of premium product (Pr(Prem)), and the predicted expected net return for the 150 observations of the production data set used to estimate the regression model. The second column, (1), displays the means and standard deviations of optimized controls, the predicted final moisture, the predicted likelihood of premium product (Pr(Prem)), and the expected net return for the solutions to the net revenue maximization problem (
) for the case where the kiln speed is constrained to the observed level for each observation. This column allows for a comparison between the observed outcome and a net revenue maximizing outcome with a throughput rate constrained to equal the observed settings. The third column,
(2), displays statistics for the expected net revenue maximizing controls subject to the same bound constraints as
(1), except kiln speed is restricted to no more than 1,000 units.
Table 6. Observed vs. optimized results
The results in have two important features. First, the probability that a premium product is produced has virtually the same value when controls other than kiln speed are optimized – that is, quality performance is not impaired by failing to optimize kiln speed. Second, the observed behavior of the firm yields an expected net revenue per hour of $313.686, which is far below the value obtained with optimized controls, $516.266 per hour. The net revenue per hour when only the controls other than kiln speed are optimized is $371.664 per hour, an 18.5% increase. Thus, it appears that a big part of the foregone net revenue is due to running the kiln too slowly. When all controls are optimized (including kiln speed), the kiln speed is uniformly set to its maximum value of 1,000 units, regardless of the flour attributes. The optimal setting for the kiln speed is not surprising because there is essentially no cost to run the kiln conveyor faster (within observed limits), and the kiln stage is the bottleneck of the production process. Thus, increasing the kiln speed results in increased throughput, and hence expected revenue per hour, without increasing costs. The increase in expected net revenue relative to the observed is substantial at $202.58 (=516.266–313.686 from , row ‘NR/hr’) per hour, a 64.6% increase.
Remark 2. We find that our partner firm can increase its net revenues both by improvements in quality by applying slight changes in the controls and by increases in throughput (i.e. increasing kiln speed to the upper limit of 1000 units).
Our findings are both intuitive and confirmed by members of the firm’s R&D team. In particular, the recommendation to increase kiln speed to the upper limit (1,000 units) was confirmed to be feasible by the firm’s managers and our data include batches that were run at nearly this kiln speed (990 as indicated by ) that resulted in premium product.
5.3. Supplier selection problem
To assess which supplier will lead to the highest expected net revenue, the control optimization problem with the expected net revenue per hour objective was applied to the full 1,619 observation data set of flour attributes, stratified by supplier. These observations span months that extend beyond the 5 months used to develop the regression model. For this reason, the month dummy variable (Month4) is set to zero for the supplier selection analysis. The results are displayed in . The means and standard deviations of the net revenue optimizing controls appear in Panel A of this table. Not surprisingly, kiln speed is set at its upper bound of 1,000 units for every batch, regardless of supplier. Similarly, salt is equal to its lower bound of 35 pounds for virtually every observation. Average added water does not vary a lot between suppliers; it is slightly higher for the supplier that has flour batches of higher average moisture (supplier 1) and lower for the supplier of higher protein levels (supplier 3). Thus, it is counterintuitive to observe that mean added water is larger for the supplier that has larger flour moisture, which leads us to study underlying relationships between other flour attributes and controls that might dominate and result in this unexpected result for supplier 1. Indeed, looking at the correlations between each supplier’s flour attributes and the optimal added water control (Appendix A3) we observe that supplier 1ʹs protein levels are highly negatively correlated with added water, which seems to be the driving force. Flour batches with low protein levels do not retain sufficient water, thus needing extra added water. In turn, variability of added water is highest for supplier 1 connecting this with higher variability of flour protein for supplier 1. Optimal mix time and extruder are set to their upper and lower limits, respectively, for all supplier-type batches that is according to what is described in the only supplement S4, which studies how the control variables should be optimized. We also note that the upper and lower limits of the controls of this table () are set up according to the finished goods recipe limits, which vary slightly from the limits of the observed batches in .
Table 7. Supplier results
Panel B of shows the optimal value of the probability of achieving premium product, the flour price by supplier, and the expected net revenue per hour. Expected net revenues are highest when flour is sourced from supplier 1, the supplier with the lowest flour price. Indeed, if the flour price is replaced for all three suppliers by the simple average of the three flour prices, the probability of achieving premium product is unchanged, and the expected net revenue per hour is identical across suppliers to five significant digits. Any differences across suppliers and batches are minor and reflect the low-cost adjustments of ingredient controls. For this particular production system and within the range of flour quality attributes reflected in the historical data, the levels of flour attributes are unimportant. That is, regardless of attribute levels, premium product can be produced with equal probability, and combined with the minor cost of the controls; this means that expected gross revenues are virtually the same across all batches. From management’s perspective, this means that flour cost is the dominant factor in selecting a supplier in order to maximize expected net returns per hour.
Remark 3. Raw material variability is not a critical feature for the firm with effective, low-cost controls. For our partner firm, lower-quality flour can be turned into premium finished goods with equal probability as higher-quality flour. Thus, flour price should be the driving force in supplier selection when the sole goal is maximization of expected net revenues.
6. Conclusions and Discussion
Inspired by studying a wheat-based snack manufacturing process, we develop a general data-driven analytical method that could be utilized by firms facing raw material variability and manageable manufacturing controls. We start by modeling the production system yield including the impacts of attributes of inputs and manufacturing controls on finished goods quality. Using this model in an optimizing mode, we identify economically optimal controls and associated expected net revenues. Given this model of optimized production system behavior, we can identify a variety of strategic improvements such as selection of suppliers, design of quality assurance programs, and environmental control measures.
In applying our method to the context of our collaborating company, we find that the observed control settings of our partner firm appear to fall short of achieving expected net revenue maximization, as we were wondering in our second research question. Our findings indicate that our partner firm could increase an estimated 65% of expected net revenues by increasing kiln speed and thereby increasing throughput. Our model indicates that the firm can do this without loss of expected manufacturing yield by using the other manufacturing controls to compensate for a higher kiln speed. Thus, a focus on maximizing quality and net revenues is aligned as questioned in the first research question. Our work more broadly addresses the capability of manufacturing controls to absorb raw material variability and fills in gaps in the food manufacturing literature specific to optimizing strategic objectives under input variability.
Our supplier selection problem uses actual supplier flour batch data to determine whether some suppliers are better than others. We find that raw material variability in flour attributes is not a critical factor to the firm’s manufacturing outcome within the range of flour attributes observed in the data. As a result, the recommendation is to prioritize purchase price with some limited attention to flour quality to ensure delivered flour is within contract ranges. This strategic choice will not have uncontrollable impacts on flour supply quality or finished goods quality, and it will allow the firm to increase profits as we questioned in our third research question. The food manufacturing industry is large, and wheat flour is a common raw material; thus, many other firms may face very similar conditions and manufacturing processes that consist of mixing dough, shaping it, proofing and baking the product, and drying prior to packaging.
We believe that our paper can act as a seed for further research. While the proposed data-driven framework is applicable to other companies in food manufacturing and other industries, the contexts may differ, driving a need for further understanding of conditions under which our findings can be expanded. In particular, the costs of manufacturing controls may be more significant, and the controls may not be as universally effective. Another difference in context arises when the finished goods have different gradations or multiple measures of quality as opposed to only considering premium and non-premium products. Finally, we believe extensions of our model can incorporate additional factors that do not play a significant role for our partner firm’s setting but might be important for other settings. These include the impact of supply and demand risks, price risk, and technological advances in manufacturing on supplier selection.
Disclosure statement
No potential conflict of interest was reported by the author(s).
Additional information
Funding
References
- Ahumada, O., & Villalobos, J. R. (2009). Application of planning models in the agri-food supply chain: A review. European Journal of Operational Research, 196(195), 1–20. https://doi.org/10.1016/j.ejor.2008.02.014
- Ahumada, O., & Villalobos, J. R. (2011). Operational model for planning the harvest and distribution of perishable agricultural products. International Journal of Production Economics, 133(2), 677–687. https://doi.org/10.1016/j.ijpe.2011.05.015
- Akkerman, R., Farahani, P., & Grunow, M. (2010). Quality, safety and sustainability in food distribution: A review of quantitative operations management approaches and challenges. OR Spectrum, 32(4), 863–904. https://doi.org/10.1007/s00291-010-0223-2
- Allen, S. J., & Schuster, E. W. (2004). Controlling the risk for an agricultural harvest. Manufacturing & Service Operations Management, 6(3), 225–236. https://doi.org/10.1287/msom.1040.0035
- Azoury, K. S., & Miyaoka, J. (2013). Managing production and distribution for supply chains in the processed food industry. Production and Operations Management, 22(5), 1250–1268. https://doi.org/10.1111/poms.12019
- Bakker, M., Riezebos, J., & Teunter, R. H. (2012). Review of inventory systems with deterioration since 2001. European Journal of Operational Research, 221(2), 275–284. https://doi.org/10.1016/j.ejor.2012.03.004
- Baltas, G., Doyle, P., & Dyson, P. (1997). A model of consumer choice for national vs private label brands. Journal of the Operational Research Society, 48(10), 988–995. https://doi.org/10.1057/palgrave.jors.2600454
- Bassok, Y., & Akella, R. (1991). Ordering and production decisions with supply quality and demand uncertainty. Management Science, 37(12), 1556–1574. https://doi.org/10.1287/mnsc.37.12.1556
- Behzadi, G., Justin, O., Olsen, T. L., & Zhang, A. (2018). Agribusiness supply chain risk management: A review of quantitative decision models. Omega, 79(September), 21–42. https://doi.org/10.1016/j.omega.2017.07.005
- Blackburn, J., & Scudder, G. (2009). Supply chain strategies for perishable products: The case of fresh produce. Production and Operations Management, 18(2), 129–137. https://doi.org/10.1111/j.1937-5956.2009.01016.x
- Bohle, C., Maturana, S., & Vera, J. (2010). A robust optimization approach to wine grape harvesting scheduling. European Journal of Operational Research, 200(1), 245–252. https://doi.org/10.1016/j.ejor.2008.12.003
- Boland, M., Foster, K. A., & Akridge, J. (1995). Packer sorting strategies for fresh pork. Agribusiness, 11(5), 423–430. https://doi.org/10.1002/1520-6297(199509/10)11:5<423::AID-AGR2720110505>3.0.CO;2-X
- Boland, M., & Marsh, T. L. (2006). Input quality in the sugar beet industry. Journal of Agricultural and Resource Economics, 31(1), 114–128. https://doi.org/10.22004/ag.econ.7316
- Boyabatlı, O., Nguyen, J., & Wang, T. (2017). Capacity management in agricultural commodity processing and application in the palm industry. Manufacturing & Service Operations Management, 19(4), 551–567. https://doi.org/10.1287/msom.2017.0624
- Bronson, K., & Knezevic, I. (2016). Big data in food and agriculture. Big Data & Society, 3(1), 1. https://doi.org/10.1177/2053951716648174
- Burt, O. R. (1978). On the statistical estimation of isoquants and their role in livestock production decisions. American Journal of Agricultural Economics, 60(3), 518–523. https://doi.org/10.2307/1239949
- Cai, X., Chen, J., Xiao, Y., & Xu, X. (2010). Optimization and coordination of fresh product supply chains with freshness-keeping effort. Production and Operations Management, 19(3), 261–278. https://doi.org/10.1111/j.1937-5956.2009.01096.x
- Census Bureau. (2017). Quarterly financial report: U.s. manufacturing, mining, wholesale trade, and selected service industries, third quarter 2017.
- Chen, X., Kouvelis, P., & Biazaran, M. (2018). Value of operational flexibility in co-production systems with yield and demand uncertainty. International Journal of Production Research, 56(1–2), 491–507. https://doi.org/10.1080/00207543.2017.1402138
- Devalkar, S. K., Anupindi, R., & Sinha, A. (2011). Integrated optimization of procurement, processing, and trade of commodities. Operations Research, 59(6), 1369–1381. https://doi.org/10.1287/opre.1110.0959
- Donk, D. P. V., & Fransoo, J. C. (2006). Operations management research in process industries. Journal of Operations Management, 24(3), 211–214. https://doi.org/10.1016/j.jom.2005.01.001
- Funes, E., Allouche, Y., Beltrán, G., Jiménez, A. . (2015). A review: Artificial neural networks as tool for control food industry process. Journal of Sensor Technology, 5(1), 28. https://doi.org/10.4236/jst.2015.51004
- Genovese, A., Acquaye, A. A., Figueroa, A., & Koh, S. C. L. (2017). Sustainable supply chain management and the transition towards a circular economy: Evidence and some applications. Omega, 66(January), 344–357. https://doi.org/10.1016/j.omega.2015.05.015
- Glen, J. J. (1987). Feature article: Mathematical models in farm planning: A survey. Operations Research, 35(5), 641–666. https://doi.org/10.1287/opre.35.5.641
- Goesaert, H., Brijs, K., Veraverbeke, W. S., Courtin, C. M., Gebruers, K., & Delcour, J. A. (2005). Wheat flour constituents: How they impact bread quality, and how to impact their functionality. Trends in Food Science and Technology, 16(16), 12–30. https://doi.org/10.1016/j.tifs.2004.02.011
- Goyal, S., & Giri, B. (2001). Recent trends in modeling of deteriorating inventory. European Journal of Operational Research, 134(1), 1–16. https://doi.org/10.1016/S0377-2217(00)00248-4
- Greene, W. H. (2003). Econometric analysis. Pearson Education India.
- Haley, T. A., & Mulvaney, S. J. (1995). Advanced process control techniques for the food industry. Trends in Food Science & Technology, 6(6), 103–110. https://doi.org/10.1016/S0924-2244(00)88992-X
- Hernandez, J. E., Mortimer, M., & Panetto, H. (2020). Operations management and collaboration in agri-food supply chains. Production Planning & Control, 32(14), 1163–1164. https://doi.org/10.1080/09537287.2020.1796141
- IBISWorld. (2022). https://www.ibisworld.com/industry-statistics/market-size/snack-food-production-united-states. ( Retrieved: 2022-May-08)
- Kopanos, G. M., Puigjaner, L., & Georgiadis, M. C. (2012). Simultaneous production and logistics operations planning in semicontinuous food industries. Omega, 40(5), 634–650. https://doi.org/10.1016/j.omega.2011.12.002
- Lowe, T. J., & Preckel, P. V. (2004). Decision technologies for agribusiness problems: A brief review of selected literature and a call for research. Manufacturing & Service Operations Management, 6(3), 201–208. https://doi.org/10.1287/msom.1040.0051
- Lucas, M. T., & Chhajed, D. (2004). Applications of location analysis in agriculture: A survey. Journal of the Operational Research Society, 55(6), 561–578. https://doi.org/10.1057/palgrave.jors.2601731
- Mullainathan, S., & Spiess, J. (2017). Machine learning: An applied econometric approach. Journal of Economic Perspectives, 31(2), 87–106. https://doi.org/10.1257/jep.31.2.87
- Mundi, I., Alemany, M., Poler, R., & Fuertes-Miquel, V. S. (2019). Review of mathematical models for production planning under uncertainty due to lack of homogeneity: Proposal of a conceptual model. International Journal of Production Research, 57(15–16), 5239–5283. https://doi.org/10.1080/00207543.2019.1566665
- Myers, R. H., Montgomery, D. C., & Anderson-Cook, C. M. (2016). Response surface methodology: Process and product optimization using designed experiments. John Wiley & Sons.
- Plante, R., Moskowitz, H., Tang, J., & Duffy, J. (1999). Improving quality via matching: A case study integrating supplier and manufacturer quality performance. Manufacturing & Service Operations Management, 1(1), 36–49. https://doi.org/10.1287/msom.1.1.36
- Roesler, N. (2020, May). The snack trends predicted to persist post-pandemic. Food Business News. https://www.foodbusinessnews.net/articles/16078-the-snack-trends-predicted-to-persist-post-pandemic
- Rong, A., Akkerman, R., & Grunow, M. (2011). An optimization approach for managing fresh food quality throughout the supply chain. International Journal of Production Economics, 131(1), 421–429. https://doi.org/10.1016/j.ijpe.2009.11.026
- Schuster, E. W., & Allen, S. J. (1998). Raw material management at welch’s, inc. Interfaces, 28(5), 13–24. https://doi.org/10.1287/inte.28.5.13
- Shashi, C., R, Singh, R., Centobelli, P., Shabani, A., & Cerchione, R. (2018). Food cold chain management: From a structured literature review to a conceptual framework and research agenda. The International Journal of Logistics Management, 29(3), 792–821. https://doi.org/10.1108/IJLM-01–2017-0007
- Tew, J. D., & Wilson, J. R. (1994). Estimating simulation metamodels using combined correlation-based variance reduction techniques. IIE Transactions, 26(3), 2–16. https://doi.org/10.1080/07408179408966603
- Varzakas, T., & Tzia, C. (2015). Handbook of food processing: Food safety, quality, and manufacturing processes (Vol. 35). CRC Press.
- Wang, X., Li, D., & Shi, X. (2012). A fuzzy model for aggregative food safety risk assessment in food supply chains. Production Planning & Control, 23(5), 377–395. https://doi.org/10.1080/09537287.2011.561812
- Yano, C. A., & Lee, H. L. (1995). Lot sizing with random yields: A review. Operations Research, 43(2), 311–334. https://doi.org/10.1287/opre.43.2.311
- Zhu, Z., Chu, F., Dolgui, A., Chu, C., Zhou, W., & Piramuthu, S. (2018). Recent advances and opportunities in sustainable food supply chain: A model-oriented review. International Journal of Production Research, 56(17), 5700–5722. https://doi.org/10.1080/00207543.2018.1425014
Appendix
Raw material variability in food manufacturing: a snack food industry case
A1. Robustness checks: Alternative Models and Commodity Prices
We conduct two robustness checks on different parts of our analysis. First, we describe how we have tested for the selection of our specific econometric model. Second, we examine whether changes in premium and non-premium finished goods prices impact the results of our comparisons between the observed and optimal results from .
A1.1 Selecting an econometric model specification
The customized quadratic regression model that we use in the paper () has been selected based on an exhaustive search that considers linear, quadratic, and cross-product combinations (Equationequation 1(1)
(1) ) of all independent variables (i.e. all flour characteristics and controls). Initially, we also considered limited dependent variable methods, including logit and multinomial logit models, wherein we defined premium and non-premium outcomes as the categories. A limited dependent variable method may provide insight when full information about outcomes, such as point measurements of quality, is unavailable. Nonetheless, our suggested model was selected for having high accuracy on out-of-sample data and avoiding problems of overfitting. Indeed, in the case of our firm’s manufacturing process, we can observe the full process, understand the important interactions, and have full information about the finished good quality, so we believe that in this case our suggested model is the best option.
From the general quadratic regression model structure defined in Equationequation 1(1)
(1) , we studied various specifications that considered a subset of independent variables. In the selection of the best regression model specification, a balance between best fit (largest adjusted
) and best predictive power has been considered. Note that the focus is not only on the fit but also on finding a model with high capacity for predicting if the finished good product is premium or non-premium. For predictive power quality, we use the AIC criterion, the predicted R-squared value, and a customized out-of-sample procedure that is defined below.
To provide a representative sample of potential specifications, we describe our selection process using three of our candidate specifications. Our selected specification (in ) was identified using an exhaustive search algorithm that considered a subset of 15 variables or fewer of all possible combinations of independent variables while maximizing adjusted R-squared. The other models were identified using non-exhaustive and automated criteria based on fully saturated models. For example, Model B was identified using a bi-directional stepwise fit based on an AIC criteria, and model C was identified through a backward stepwise fit also based on an AIC criteria. Other models were considered with other variable selection criteria to generate potential specifications. For the sake of showing a representative sample, we show the regression results of models B and C, in respectively.
After identifying the potential specifications, we perform two accuracy tests (the results of which are provided in the columns ‘Predicted R-squared’ and ‘Accuracy’ in ). The first is to use the predicted residual error sum of squares to estimate the predicted r-squared. This is done by removing each observation one-at-a-time from the dataset, estimating the regressors’ coefficients, predicting the removed observation, and calculating the error sum of squares from the known value. The second accuracy test is to ensure that our specifications can predict premium and non-premium and uses the following steps to determine out of sample accuracy:
We randomly and without replacement partitioned our production dataset into two subsets: training, with 70% of observations, and test, with the remaining 30% of observations.
We estimated the regression on the training subset, and tested its predictive ability to distinguish between premium and non-premium outcomes on the test subset. We compared the point prediction to the observed finished goods moisture and whether it would have been classified as premium or non-premium in the estimated versus observed outcomes.
We repeated steps 2 and 3 one-thousand times for each of the specifications in step 1, randomly redrawing the training and test datasets with each iteration.
We calculate the fraction of the times the prediction accurately reflects whether the outcome was premium versus non-premium for each specification, and average the total accuracy across all 1,000 iterations to serve as a measure of the out-of-sample predictive power of the model.
Lastly, we determine the bias (column ‘Bias’ of ) by subtracting the estimated number of non-premium batches from the true number of non-premium batches. When positive, this reflects an overestimation of premium batches.
Table A1. Estimated regression coefficients of Model B
Table A2. Estimated regression coefficients of Model C
shows a comparison of different fit and accuracy results between the three final candidate specifications. We can observe that model B has the highest categorical prediction accuracy at 94.2% and the lowest BIC due to the simplicity of its model and Model C has the smallest percentage bias. However, the selected model has the best balance between the highest fit (highest adjusted R-squared) and also a highly acceptable categorical accuracy (at 92.7%) and the lowest AIC value and highest predicted R-squared. We also decided to select the model specification in because it considers 15 independent variables, which is an acceptable number given that we have a total of 150 observations (model C substantially exceeds the recommended number of independent variables and thus risks overfitting). Another feature of the selected model is that it includes all attributes and all manufacturing controls. We believe that all these variables play an important role in the manufacturing process.
Table A3. Comparison between the three candidate models
A1.2 Testing pricing assumptions
We also test the robustness of our finished goods pricing assumptions, primarily the ratio of premium to non-premium finished goods prices. In our net revenue model, we assume that the value of a premium product is twice that of a non-premium batch for our numerical analysis, an assumption validated by Baltas et al. (Citation1997) in their study of private label versus branded products. Using our production dataset with pooled suppliers and months, we tested different ratios of premium and non-premium product values and observed that when the value of premium is less than 12.9% higher than the value of non-premium, some flour batches are too costly to turn into premium product, and it is net revenue maximizing to turn them into non-premium product. This is a consequence of having a production process that is highly controllable with only minor costs. In any case, a 12.9% difference between premium and non-premium product is very low, and extremely unlikely to occur in the market. On the other hand, if the incentive for premium is increased, for example, if we more than double the value of a premium batch leaving the value of the non-premium batch unchanged, identical probabilities of producing a premium batch result. We conduct a final price test by setting the price of the non-premium product to zero and maintaining the full price for a premium product. In this case, the controls remain identical and this indicates that the price of the non-premium product is insensitive to our results and the revenue maximizing solutions also maximize quality. Thus, the results are robust to wide ranges of output prices and various distances between premium and non-premium finished goods.
A2. Observed Flour Attribute Graphs
The following graphs provide the monthly mean and variance for the observed flour attributes by supplier in our flour reports dataset. The attributes include protein (percent by weight), moisture (percent by weight), ash (percent by weight) and the mixing tolerance index, or MTI (Brabender Units, from a farinograph).
A3. Correlation Matrix of Added Water and Flour Attributes by Supplier
Table A4. Correlation matrix Supplier 1 Added water and Flour batch attributes
Table A5. Correlation matrix Supplier 2 Added water and Flour batch attributes
Table A6. Correlation matrix Supplier 3 Added water and Flour batch attributes