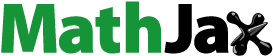
ABSTRACT
This paper presents a method for determining the performance of shuttle-based storage and retrieval systems (SBS/RS) with tier-captive, single-aisle shuttles serving tiers of multiple-deep storage and using a class-based storage policy. This approach is used in the design process of SBS/RS and in the upgrading process of existing SBS/RS. With this approach, it is possible to evaluate the improvement in the performance of multiple-deep storage system by applying a class-based storage policy. The basis of this calculation method is a continuous-time, open-queueing system with limited capacity. The cycle times of lifts and shuttles, as determined by a spatial value approach, combined with a probability-based approach to mention the storage policy. To take the multiple-deep storage into account, another probability-based approach is applied. A European material handling provider had given the data used in this publication. Through an example, the influences of the storage policy and the storage depth are depicted.
1. Introduction
In the global supply chain, warehouses play a very important part. Because of the rapid development, further innovations of the storage technology are necessary. To meet the high requested performance of storage and retrieval systems, shuttle-based storage and retrieval systems (SBS/RS) are developed. These systems replaced the traditional picker-to-part warehousing systems to save labour and space (Azadeh et al., Citation2019; Boysen et al., Citation2019; Liu et al., Citation2021).
Because space and the physical parameters are limited, new inventions are made. One of these innovations is the application of advanced storage policies, such as a class-based storage policy. The reason for the evolution through different storage policies is that these policies highly influence the expected throughput (Azadeh et al., Citation2019; Silva et al., Citation2020). This change of the policy can help to meet further performance requirements without changing the physical parameters of the system (Eder, Citation2020b).
The second way of innovation that is discussed in this paper is the passing from single-depth storage to multiple-deep storage. This leads directly to a smaller footprint of the storage system, with the same capacity of storage (Eder, Citation2020a).
For designing warehouse systems, the expected throughput, e.g. the expected travel time, is a fundamental step (Azadeh et al., Citation2019). Thus, the aim of this paper is to provide an analytical decision tool that accurately evaluates the throughput of SBS/RS with multiple-deep storage and a class-based storage policy in combination of these two evolutions. The invented approach is not limited to a certain storage depth and also not to a fixed number of classes in the storage system. Implementing a class-based storage strategy is possible with no physical change of the SBS/RS with different storage depths. This leads to an implementation cost only on the organization side of the storage system.
In contrast to other publications that try to develop and describe new systems, such as Jerman et al. (Citation2021), this paper tries to show possibilities to increase the performance of known systems via the storage strategy and the storage system design such as the storage depth. This is a huge benefit for material handling providers as it allows them to improve the performance of their existing systems. Implementing a class-based storage strategy is possible with no physical change of the SBS/RS with different storage depths. This leads to an implementation cost only on the organization side of the storage system.
This paper is organized as follows: lists the abbreviations used in this paper. Section 2 deals with an explanation of the existing literature related to travel time measurement of tier-captive SBS/RS. The description of the treated SBS/RS and the underlying assumptions are depicted in Section 3. In Section 4, the analytical approach to treat such systems is shown. Section 5 deals with a numerical study to discuss the influence of the storage depth and the class-based storage policy on the throughput. The parameters used in this study are given by a European material handling provider. Finally, in Section 6, a summary of this paper and an outlook on future research are presented.
Table 1. Abbreviations
2. Literature review
SBS/RS is a special version of an autonomous vehicle storage and retrieval system (AVS/RS) due to the similarity of their physical configurations (Carlo and Vis, Citation2012; Ekren et al., Citation2017). A great number of studies have considered travel time measures of tier-captive SBS/RS through simulation (e.g. Kriehn et al. (Citation2018); Lin et al. (Citation2021)) and analytical models (e.g. Eder (Citation2019); Lerher (Citation2016)).
Especially in the field of class-based storage policies in SBS/RS, there are only few publications. The first paper that has to be mentioned is by Kuo et al. (Citation2008). In this paper, a queueing network is applied to describe the SBS/RS with class-based storage. The limitation of such an approach is that this approach can only calculate the waiting times within one handling cycle, but the largest throughput cannot be extracted out of this approach (Eder Citation2019). The next paper that treats class-based storage policies in SBS/RS is by Ekren et al. (Citation2015). It is a simulation study of such SBS/RS. Also Kriehn et al. (Citation2018) published a simulation study of class-based storage policies in SBS/RS. This paper tries to optimize the class-based storage policy and to quantify the reachable benefit of the storage policy.
Schloz et al. (Citation2017) tries in their paper another way to discuss storage policies in SBS/RS. They developed an optimization approach to find the best places for the different classes in the rack. As can be seen above, there are few papers that discuss class-based storage policies in SBS/RS.
The second kind of publications relevant to this paper are the publications that treat SBS/RS with multiple-deep storage. The approaches of Lerher (Citation2016), Eder and Kartnig (Citation2016) and Zhan et al. (Citation2021) are limited to a storage depth of 2. Lerher (Citation2016) assumes that the rear storage locations are filled first and that the front storage locations are filled with a filling degree of . Eder and Kartnig (Citation2016) do not make such assumptions. Instead, the selection of storage slots is random, resulting in different probabilities and times within the service-time approach. Manzini et al. (Citation2016) develop a cycle-time model that is continuous in space. But this approach does not capture the distance-dependent differences in speed profiles. Tappia et al. (Citation2017) assume that a shuttle transports a satellite, which transports the totes from a storage slot into a shuttle. The movement of these two vehicles is decoupled in the investigated system. Because this differs from the disgust system in this paper, that publication is not treated further in detail. Another publication that must be mentioned is Lehmann and Hußmann (Citation2021). This paper tries to describe the influence of the multiple-deep storage by applying a Markov chain. The result of the approach is like the results of the approach according to Eder (Citation2020a) at the interesting filling degree from
to
. The publication of Marolt et al. (Citation2022) discusses a multiple-depth storage system with different storage strategies, such as a depth-first assignment strategy or a random storage strategy.
As seen above, there is no publication that treats the influence and the correlation of multiple-deep storage in combination with a class-based storage policy. There is literature on multiple-deep storage (e.g. Lerher (Citation2016)) and on class-based storage strategy (e.g. Kriehn et al. (Citation2018)), but the combination of both cannot be found in the literature.
In this paper, the main idea of Eder (Citation2020a) and Eder (Citation2020b) should be used and advanced. The reason for the selection of these two publications is that these two papers depict a simple and accurate way to describe an SBS/RS. Assumptions made in these publications, such as the continuous time model and the spatial discretization, represent the storage system like its proper behaviour. The whole assumptions can be found in the next chapter.
3. System description
The system that is investigated in this paper is a tier-captive single-aisle SBS/RS as shown in . A way of zones due to the class-based storage is shown by different colours in the figure. In front of each rack, there is a lift that transports the totes from the input–output point (I/O point) to the tier and back. The I/O points are on the first tier in front of the lifts. In each tier, there are buffers between lifts and shuttles. Each shuttle is assigned to one tier in one aisle, so there are as many vehicles as tiers in a rack. Furthermore, these vehicles can handle one tote at a time along the aisle. The racks are multiple-deep and double-sided, and each storage location can hold one tote (Eder, Citation2019, Citation2020a).
Assumptions (and some notations) proposed in this paper were like those for an SBS/RS produced by a European material handling provider. The assumptions have also been published under Eder (Citation2020b) and Eder (Citation2020a):
Both lifts realize transactions in a single-command cycle under a first-come-first-served (FCFS) rule; thus, one lift is for the input and the other is for the output.
The shuttles realize the transactions in single and double cycles under an FCFS rule.
The dwelling point for the input lift is the I/O point.
The dwelling point for the output lift is in front of the tier where the next tote has to be restored.
The dwelling point of the shuttle is in front of the buffer slots.
The lifts and shuttles speed up/decelerate with constant acceleration/deceleration. In case the acceleration/deceleration is not constant, the actual acceleration/deceleration must be transferred to an acceleration/deceleration, which gives the same behaviour as the actual acceleration/deceleration of the SBS/RS.
There is no difference in time between transferring totes to or from the lifts. If there is a difference in the proper system, this has no effect on the calculation since only the sum of times is used for loading and unloading.
There are different transfer times from and to the shuttle depending on the depth of the rack.
There are always totes on the I/O point waiting to be stored. This assumption is necessary to achieve the largest throughput. Otherwise, the input lift must wait for incoming totes, which would affect the performance of the SBS/RS under consideration.
The order of the totes to be restored next is evenly distributed among all stored totes of the same class.
The tote to be restored is restored to the next free storage location.
The order of the totes from different classes is as given by the storage policy.
The assignment of the class to a tote is static and cannot be changed while the tote is stored.
The filling degree is limited to a known value to enable a relocation cycle.
4. Analytical approach
For the determination of the performance of an SBS/RS system, a single aisle is modelled. Due to the uniform distribution of the storage and retrieval transactions among all aisles, the performance of the system can be evaluated by modelling a single aisle (Epp et al., Citation2017; Heragu et al., Citation2011; Marchet et al., Citation2012). The analytical approach for SBS/RS with class-based storage strategy and multiple-deep storage presented in this paper is based on the approach as found in Eder (Citation2019; Citation2020a, Citation2020b).
The reason for the selection of this approach is that it is very stable and needs a few computing time. In further use, this is very useful for the design process of SBS/RS with class-based storage policy and a multiple-deep rack. Another advantage of this approach is that the calculation is continuous in time. This means that there is no error due to the discretization on time. Especially at the quantification of the waiting time between lifts and shuttles, this is a significant advantage. The second fundamental assumption, the spatial discretization, is an advantage due to the possibility of mentioning the different speed profiles of a transporter through the distance between two locations. Even in real storage systems, the storage locations are discretely distributed.
This approach uses an open queueing model with limited capacity (M G
1
K). This model has three main parts to determine the throughput, the inter-arrival time to one tier, the service time of the shuttles, and the last part is the open queueing model M
G
1
K. To adopt this approach, all these three parts have to be changed. The notations used in this paper are listed in .
Table 2. Notation of the tier-captive single-aisle AVS/RS
The number of tiers of the area can be calculated as follows (Eder, Citation2020b):
It is the sum of the probabilities that a tote from zone/class is in area
times the relative number of storage locations of class
and this sum times the number of tiers to get the number of tiers in area
. For example, a rack with
and a relative number of storage location
and
and further that all locations from class A are in the first area (
),
of class B are also in the first area (
), and
of class B are in the second area (
). This leads to a number of tiers in area 1
and in area 2
.
The probability of order from area is due to (Eder, Citation2020b):
This is the sum of the probabilities that a tote from class is in area
times the probability of order from zone/class
.
The number of slot for articles from zone in the area
is as follows. This is the distribution of the articles from the classes over the length of a tier (Eder, Citation2020b).
This expression contents the probability that a tote from zone is in area
times the number of storage slots from zone/class
times the number of slots on each side per aisle times the number of tiers and all these terms divided by the number of tiers in area
.
The probability of order within a tier in the respective area converts the overall probability of order
into the probability of order within a tier to calculate the service time of the respective tier (Eder, Citation2020b).
This conversion is done by the multiplication of the probability of order from zone/class with the probability that a tote from zone/class
is in area
. This multiplication is divided by the sum over all zones of the same multiplication.
4.1. Inter-arrival time
The first part needed in the throughput’s determination is the inter-arrival time of the tote into the different tiers. This calculation has to be made once for the entire aisle across all the different zones. In the end of this section, the assuming inter-arrival time has to be differentiated to the different zones in vertical direction.
Single command cycle The first equation treats the mean cycle time of the lifts. It contents the meantime for the ride to the respective tiers and the time needed to transfer the tote to and from the lift.
The following equation describes the time needed for the ride to the different tiers combined with the probabilities for inter-arrivals to the respective tiers. The term corresponds to the factor
for the different areas; for the area 0, the term
.
Taking into account the different speed profiles of the lifts/shuttles depending on the distances, the function must be split into two sections: one part for distances below
, viz.,
The last equation in this section describes the transformation of the cycle time of the lift in the inter-arrival times into the different tiers, according to the different zones. This equation has to be made in the same number of times as different zones are in vertical direction.
4.2. Service time for a multiple-depth storage rack
The calculation of the service times of the shuttle for two-dimensional zoning is based on the approach of Eder (Citation2020b) with the extension to multiple-deep storage.
Single command cycle For single command cycle, the equations are as follows.
The meantime for the ride within the tier for single command cycles of the shuttles is as follows (Eder, Citation2020b). The term corresponds to the factor
for the different zones.
Dual command cycle For dual command cycle, the equations are as follows.
The meantime for the ride within the tier for dual command cycles of the shuttles is as follows (Eder, Citation2020b). The terms ,
,
, and
correspond to the factor
for the different zones and
/
corresponds to
.
To calculate the missing terms in the equations above, the following points have to be determined first:
• Meantime to transfer the tote to and from the shuttle () (see 4.2.1).
• Probability that a relocation cycle is needed () (see 4.2.2).
• Meantime needed for the ride for relocation () (see 4.2.3).
• Meantime necessary to transfer the tote to and from the shuttle in the relocation cycle () (see 4.2.4).
• Meantime for the relocation cycle () (see 4.2.5).
4.2.1. Meantime needed to transfer the tote to and from the shuttle
The first part is the meantime for the transfer from and to the shuttle. This equation is based on the paper from Eder (Citation2020a). This approach has to be adopted to consider the different zones within one tier. Therefore, the probability of order from a zone () and the different filling degrees of the respective zones (
) are accumulated to the approach.
For multiple-deep storage with the storage depth (), the meantime for the transfer of a zone in the vertical direction is
4.2.2. Probability of relocation
The probability of relocation strengthens around the fact that the ordered tote is not directly retrievable. The approach from Eder (Citation2020a) has been adopted similarly as above. For greater storage depths, the probability of a relocation cycle is
4.2.3. Meantime necessary for the ride in the relocation cycle
The distances in the relocation cycle depend on the free storage locations next to a storage slot in which the ordered tote is placed. This parameter does not describe the distance for a single relocation cycle, but through a few relocation cycles, it provides an accurate approximation to reality. The mean distance describes the average length that must be covered during the relocation cycle. This cycle sequence can also be described in the following equation that is also valid for greater storage depths (Eder, Citation2020b).
The upper range of the sum is set to the length of the zone within the respective tier. This is the longest possible distance if the slot containing the wanted tote is in the end of a zone. This equation has to be solved for every zone in vertical direction.
4.2.4. Meantime needed to transfer the tote to and from the shuttle in the relocation cycle
The meantime needed to transfer the tote from and to the shuttle in the relocation cycle is like the meantime necessary to transfer the tote from and to the shuttle, EquationEquation (14)(14)
(14) . The difference being the absence of a transfer from and to the buffer. For greater storage depths, the following equation was developed:
4.2.5. Meantime needed in the relocation cycle
The meantime needed in the relocation cycle is the summation of the meantime necessary for the ride (4.2.3) and the meantime needed to transfer the tote to and from the shuttle during the relocation cycle
(4.2.4). This equation with all the parameters inside has to be solved for every zone in the vertical direction.
4.3. Open queueing model M/G/1/K
The calculation of the open queueing system with limited capacity at zoning can be made by the following procedure. The equations for the determination of the throughput of one tier are as follows, and is the number of the area (Eder, Citation2020b). This equation of Eder (Citation2020b) is based on the calculation of the throughput of an open queueing system with limited capacity through Smith (Citation2004).
The throughput of an aisle is the sum over the different areas of the throughput of one tier in the respective area multiplied by the number of tiers in this area (Eder, Citation2020b):
The blocking probability of a queueing system has to be calculated for each area (Eder, Citation2020b).
The same refers to the utilization rate of the shuttle, which also has to be calculated for the different areas (Eder, Citation2020b).
Also the capacity of the queueing system has to be calculated for each area of the SBS/RS (Eder, Citation2020b).
The third argument is , and the coefficient of variation of the service process has to be evaluated by a numerical simulation of the service times.
The probability of emptiness contents the same arguments as the blocking probability and can be calculated as follows for each zone of the SBS/RS (Eder, Citation2020b):
5. Numerical study and discussion
The aim of this part is to show the possibilities of the invented analytical approach in the design of storage systems based on tier-captive shuttles. Hereby, the numerical optimization due to highest throughput is the key feature of this approach. The assumptions for this numerical study are that there are always totes waiting in front of the input lift. The second assumption is that all retrieved tote can leave the storage system unhindered. The parameters for the depicted optimization example are shown in . These parameters are given by a European material-handling provider.
Table 3. Tested parameter configurations of the tier-captive single-aisle SBS/RS
Using this numerical optimization is in the design process of such storage systems or in the upgrading of an existing storage system. In both the processes, the knowledge of the influence of the different parameters is important. Thus, at the upgrading of an existing system without changing the physical storage system, only the implementation of the class-based storage strategy is relevant. Here, the spread of the different storage classes and the number of storage classes can be varied. The influence of implementing class-based storage strategy can be seen in and in . The number of storage slots in this example is at approx 8,000 storage location. In this figure, the impact of the implementation can be seen through one column. The distinct lines represent here the different number of classes. The influence of the spread of a class itself was depicted earlier in the publication of Eder (Citation2020b) for single deep storage. This publication quantifies the loss through a non-optimal spread of a two-class storage strategy to around on the throughput of an aisle.
Figure 2. Example of different storage racks with around 8000 storage locations and different storage strategies.

Table 4. Optimization example
The results were determined using a calculation algorithm which calculated all rack configurations for a fixed number of storage locations. The only restriction here was that the areas of the different zones can only be rectangular. From these results, those with the maximum throughput were extracted, and these results are depicted in . Because the results from this calculation method have an obvious pattern and there were no errors in the calculation algorithm, it can be assumed that the approach is stable.
In the design process of a new storage system, there are more parameters that can be varied. To quantify the impact of the class-based storage strategy and of the multiple-deep storage, only these two parameters are varied in the discussed example. The results are depicted in . The results are also visualized in . The increase in the storage depth leads to decreasing length of the aisle. Up to a storage depth of three, the number of tiers decreases, with further increase of storage depth, it lightly increases, too. The throughput increases up to a storage depth of 3, and it sightly decreases at higher storage depths. The influence of the class-based storage strategy is also not to be neglected. Here, the throughput of an aisle increases with implementing a two-zone storage strategy. The further increase of the throughput through a three zone policy is less than the change from one-zone to a two-zone policy. Also, the main tendency of the throughput due to the storage depth is the same. The only difference is that in this example, the highest throughput is with a storage depth of 2. Another trend of implementing a class-based policy is that with an increase of the number of zones, the rack length decreases and increases in height. The increase of the throughput from the simplest system with single deep storage and no class-based storage policy to the most powerful system in this example with double deep storage and class-based storage policy with 3 classes is around with the same number of storage slots. Also, the application of a 2-class-based storage policy increases the throughput to a maximum of
at double deep storage.
Finally, the increase in the throughput is even higher if there are limitation, for example in the length of an aisle. Hereby, the implementation of higher storage depth in combination with a class-based storage strategy can be much higher as in the depicted example where there are no limitations of any parameters. For example, if the the length is limited to a number of 105 slots for a single depth storage, this leads to a number of 38 tiers. The throughput of an aisle with a uniform storage policy is , and with the same limitation, the throughput of an aisle with 3 storage classes is
. The increase is so around
.
The impact is even more if the storage depth is changed and the class-based storage strategy is implemented. Hereby the length is limited to around 60 slots. This leads to a rack at single depth storage that has 67 tiers and 60 slots in the length. The throughput of such a system is around . The optimized rack has a double depth storage and a 3-class storage strategy. This configuration leads to a throughput of around
. This is an increase of about
towards the simple configuration.
The effect of class-based storage is even more if the time for the ride is a big part of the inter-arrival time and of the service time. The system discussed in this example is powerful in the ride, so the handling of the totes takes a big part of the cycle time of the shuttles and the lifts. This is also because class-based storage strategies only influence the mean length of the ride of shuttles and lifts. If this part of the calculation accounts for a high proportion, then the influence of the storage strategy is even higher.
6. Conclusion
The high performance of the SBS/RS has increased the use of such systems in the industry. Only few decision tools are available to evaluate the performance of such systems. Existing tools discuss systems with only multiple-deep storage or systems with a different storage policy. This paper deals instead with an approach that discusses the combination of multiple-deep storage and a class-based storage policy. The impact and the correlation of these two ways to improve the storage system are depicted. Another feature of the invented approach is that it is continuous in time and spatial discrete. That means that there are no differences to the proper system due to the discretization over time. Further, the proper distances and its leading time to the transporters can be achieved through the spatial discrete approach. This approach is used in the upgrading of an existing storage system to evaluate the reachable throughput by applying a class-based storage strategy. On the other hand, it also can be used in the design process of new storage system to achieve highest throughput and optimal dimensions of the rack to meet the requirements.
The accuracy of the invented approach is given by the combination of two validated approaches. The one is for the multiple-deep storage in Eder (Citation2020a), and the second for the class-based storage policy is from Eder (Citation2020b). This is due to the fact that the two parts are independent in the analytical approach. The sensitivity analysis of the invented approach shows that the change of parameters is stable.
Finally, this study presents how the invented approach can be used in the design process of SBS/RS to meet the requirements, such as storage capacity, storage throughput, retrieval throughput, and throughput for symmetrical storage/retrieval. This approach can now evaluate the distribution of the classes through the aisle. This will lead to an increase of the throughput. The increase through implementing a storage policy is depicted to around for no limitations in any direction. Besides, if the length of an aisle is limited to a certain value, the influence of the storage policy and the multiple-deep storage can be much higher. As shown in the example, the increase from a uniform distributed single-deep storage aisle to a double-depth storage aisle with three classes of storage goods and a limited length is around
. So the implementation of a class-based storage policy in the combination with multiple-deep storage cannot be neglected in the design process of SBS/RS. This approach can also be used as a further decision tool to evaluate the gain on throughput in relation to the effort required for a class-based storage strategy.
Further work should be dedicated to SBS/RS with a higher number of lifts and the influences on the throughput due to the interactions on the different lifts. The zone spread discussed in this article is set to rectangular zones. In further work, it must be examined if this is the best spread or if other types of areas, such as shell-shaped spreads of the classes, provide a higher throughput. An economic consideration was not the aim of this paper, but for further research, it will be interesting.
Acknowledgments
This work was supported by the TU Wien University Library, through its Open Access Funding Programme.
Disclosure statement
No potential conflict of interest was reported by the author(s).
References
- Azadeh, K., Roy, D., & De Koster, R. (2019). Design, modeling, and analysis of vertical robotic storage and retrieval systems. Transportation Science, 53(5), 1213–1234. https://doi.org/10.1287/trsc.2018.0883
- Boysen, N., De Koster, R., & Weidinger, F. (2019). Warehousing in the e-commerce era: A survey. European Journal of Operational Research, 277(2), 396–411. https://doi.org/10.1016/j.ejor.2018.08.023
- Carlo, H. J., & Vis, I. F. A. (2012). Sequencing dynamic storage systems with multiple lifts and shuttles. International Journal of Production Economics, 140(2), 844–853. https://doi.org/10.1016/j.ijpe.2012.06.035
- Eder, M., & Kartnig, G. (2016). Throughput analysis of S/R shuttle systems and ideal geometry for high performance. FME Transactions, 44(2), 174–179. https://doi.org/10.5937/fmet1602174E
- Eder, M. (2019). An analytical approach for a performance calculation of shuttle-based storage and retrieval systems. Production & Manufacturing Research, 7(1), 255–270. https://doi.org/10.1080/21693277.2019.1619102
- Eder, M. (2020a). An approach for a performance calculation of shuttle-based storage and retrieval systems with multiple-deep storage. The International Journal of Advanced Manufacturing Technology, 107, 859–873. https://doi.org/10.1007/s00170-019-04831-7
- Eder, M. (2020b). Analytical model to estimate the performance of shuttle-based storage and retrieval systems with class-based storage policy. The International Journal of Advanced Manufacturing Technology, 107, 2091–2106. https://doi.org/10.1007/s00170-020-04990-y
- Ekren, B. Y., Sari, Z., & Lerher, T. (2015). Warehouse design under class-based storage policy of shuttle-based storage and retrieval system. IFAC-PapersOnLine, 48(3), 1152–1154. https://doi.org/10.1016/j.ifacol.2015.06.239
- Ekren, B. Y., et al. 2017. “A queuing network approach for performance estimation of shuttle based storage and retrieval system design.” In B. Y. Ekren, A. Akpunar, Z. Sari, T. Lerher (Eds.), XXII international conference on material handling constructions and logistics - MHCL 2017.
- Epp, M., Wiedemann, S., & Furmans, K. (2017). A discrete-time queueing network approach to performance evaluation of autonomous vehicle storage and retrieval systems. International Journal of Production Research, 55(4), 960–978. https://doi.org/10.1080/00207543.2016.1208371
- Heragu, S. S., Cai, X., Krishnamurthy, A., & Malmborg, C. J. (2011). Analytical models for analysis of automated warehouse material handling systems. International Journal of Production Research, 49(22), 6833–6861. https://doi.org/10.1080/00207543.2010.518994
- Jerman, B., Yetkin Ekren, B., Küçükyaşar, M., & Lerher, T. (2021). Simulation- based performance analysis for a novel avs/rs technology with movable lifts. Applied Sciences, 11(5), 2283. https://doi.org/10.3390/app11052283
- Kriehn, T., Schloz, F., Wehking, K.-H., & Fittinghoff, M. (2018). Impact of class-based storage, sequencing of retrieval requests and warehouse reorganisation on throughput of shuttle-based storage and retrieval systems. FME Transactions, 46(3), 320–329. https://doi.org/10.5937/fmet1803320K
- Kuo, P.-H., Krishnamurthy, A., & Malmborg, C. J. (2008). Performance modelling of autonomous vehicle storage and retrieval systems using class-based storage policies. International Journal of Computer Applications in Technology, 31(3–4), 238–248. https://doi.org/10.1504/IJCAT.2008.018160
- Lehmann, T., & Hußmann, J. (2021). Travel time model for multi-deep automated storage and retrieval system with a homogeneous allocation structure. Logistics Research, 14, 5. https://doi.org/10.23773/2021_5
- Lerher, T. (2016). Travel time model for double-deep shuttle-based storage and retrieval systems. International Journal of Production Research, 54(9), 2519–2540. https://doi.org/10.1080/00207543.2015.1061717
- Lin, Y., Wang, Y., Zhu, J., & Wang, L. (2021). A model and a task scheduling method for double-deep tier-captive SBS/RS with alternative elevator-patterns. IEEE Access, 9, 146378–146391. https://doi.org/10.1109/ACCESS.2021.3120418
- Liu, Z., Wang, Y., Jin, M., Hao, W., & Dong, W. (2021). Energy consumption model for shuttle-based storage and retrieval systems. Journal of Cleaner Production, 282, 124480. https://doi.org/10.1016/j.jclepro.2020.124480
- Manzini, R., Accorsi, R., Baruffaldi, G., Cennerazzo, T., & Gamberi, M. (2016). Travel time models for deep-lane unit-load autonomous vehicle storage and retrieval system (AVS/RS). International Journal of Production Research, 54(14), 4286–4304. https://doi.org/10.1080/00207543.2016.1144241
- Marchet, G., Melacini, M., Perotti, S., & Tappia, E. (2012). Analytical model to estimate performances of autonomous vehicle storage and retrieval systems for product totes. International Journal of Production Research, 50(24), 7134–7148. https://doi.org/10.1080/00207543.2011.639815
- Marolt, J., Kosanić, N., & Lerher, T. (2022). Relocation and storage assignment strategy evaluation in a multiple-deep tier captive automated vehicle storage and retrieval system with undetermined retrieval sequence. The International Journal of Advanced Manufacturing Technology, 118(9), 3403–3420. https://doi.org/10.1007/s00170-021-08169-x
- Schloz, F., Kriehn, T., Wehking, K.-H., & Fittinghoff, M. (2017). Entwicklung situationsabhängiger Lagerstrategien für Hochregallager mit autonomen Fahrzeugen. Logistics Journal: Proceedings, 2017(10), 1–8 https://doi.org/10.2195/lj_Proc_schloz_de_201710_01
- Silva, A., Coelho, L. C., Darvish, M., & Renaud, J. (2020). Integrating storage location and order picking problems in warehouse planning. Transportation Research Part E: Logistics and Transportation Review, 140, 102003. https://doi.org/10.1016/j.tre.2020.102003
- Smith, J. M. (2004). Optimal design and performance modelling of M/G/1/K queueing systems. Mathematical and Computer Modelling, 39(9–10), 1049–1081. https://doi.org/10.1016/S0895-7177(04)90534-1
- Tappia, E., Roy, D., De Koster, R., & Melacini, M. (2017). Modeling, analysis, and design insights for shuttle-based compact storage systems. Transportation Science, 51(1), 269–295. https://doi.org/10.1287/trsc.2016.0699
- Zhan, X., Liyun, X., & Ling, X. (2021). Task scheduling problem of double-deep multi-tier shuttle warehousing systems. Processes, 9(1), 41. https://doi.org/10.3390/pr9010041