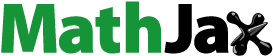
ABSTRACT
Recycling and remanufacturing logistics network affects the scale and efficiency of sustainable development of the manufacturing industry. This paper designs a multi-level closed-loop supply chain network with supplier, manufacturer, recycling centers, preprocessing centers and processing centers. An improved nonlinear grey Bernoulli-Markov model is developed to predict the recycled quantity. The capacity of recycling center and preprocessing center, the demand of manufacturer and the inventory of preprocessing center are formulated as constraints. A dynamic multi-objective model, which is based on scalable logistics facilities, takes into account the minimization of system operating costs and minimization of time costs related to the out-of-stock and inventory in each operating cycle. This model realizes the dynamic selection of the scale of facilities. Objective weighted genetic algorithm is adopted to transform multi-objective optimization problem into a single-objective. A scrap automobile products calculations are analyzed to verify the effectiveness and practicability of this model.
1. Introduction
With the rapid development of economy, the damage to resources and environment is huge and the efficiency of resource utilization is relatively low. These lead to the increasingly prominent contradiction between social and economic development, resource shortage and environmental degradation. Meanwhile, many factors, for instance, the rapid development of Internet technology, the increase in the variety of consumer products, the shortening of product life cycles, and the faster speed of product upgrading, result in a mountain of used products (Fathollahi-Fard et al., Citation2021; Feng et al., Citation2019). Taking automobiles, typical home appliances and electronic products as examples, the quantity of used products is showing an increasing trend. These used products contain metal elements with high utilization value, such as copper, silver, aluminum and gold. Mishandling of the used products in the traditional manufacturing industry for many years has led to the wasting of the resources and severe environmental problems (Ren et al., Citation2017). The requirements for after-sales service of consumers are also rising with the improvement of laws in many countries and the continuous upgrading of market competition. Countries around the world have formulated policies, such as resource conservation, energy conservation and emission reduction, circular economy, ecological economy and green development (Guo & Gao, Citation2015; Nili et al., Citation2021; Tan & Guo, Citation2019). The recycling and remanufacturing of used products not only makes the green transformation and sustainable development of the manufacturing industry, but also helps enterprise to seize the huge opportunities and build a more positive public image (Alkahtani et al., Citation2021). Hence, academic researchers and firm leaders are paying more attention to the research and application of this topic (Tighazoui et al., Citation2019). On the one hand, compared with new products, remanufactured products can save cost, energy, raw materials (Song et al., Citation2016), and minimal solid waste. On the other hand, the quality and performance of remanufactured products reach or exceed the new products. Therefore, the recycling and remanufacturing industry has a bright future.
The logistics network should be designed before carrying out the activities of recycling and remanufacturing. The logistics network of scalable facilities can not only greatly increase the benefits of each node in the supply chain, but also improve the efficiency of supply chain management, which finally affect the scale and efficiency of the sustainable development of the manufacturing industry. In terms of design theories and methods, the recycling and remanufacturing logistics network is different from the traditional forward. It is necessary to improve the existing and establish a targeted model to form a complete set of used products logistics network design theory and solution.
This paper discusses the problem of scalable logistics facilities location for recycling and remanufacturing supply chain networks under uncertainty. The main research contents are as follows:
ⅰ. The improved nonlinear grey Bernoulli-Markov combined prediction model is developed to predict the recycled quantity of used products at each recycling center in each operating cycle. Based on this, according to the scalable principle of facility, supplier, manufacturer, recycling centers, preprocessing centers and processing centers are the participants of closed-loop logistics network. Capacity, the demand of manufacture and the inventory of preprocessing centers are used as constraints with considering the relationship between recycling centers and preprocessing centers. A dynamic multi-objective model not only takes into account the minimization of system operating costs, but also considers minimization of time costs related to the out-of-stock and inventory in each operating cycle.
ⅱ. Aiming at the characteristics of low historical data and high volatility of the recycled quantity of recycling centers, an improved nonlinear grey Bernoulli-Markov combination prediction model suitable for predicting the recycled quantity of used products is proposed by combining with grey prediction theory and Markov chain.
ⅲ. The objective weighted genetic algorithm is adopted to transform the dynamic multi-objective optimization problem into a single-objective optimization problem solving model. Combining scrap automobile products, a calculation example of the location of a recycling and remanufacturing logistics network based on scalable facility is calculated to illustrate the effectiveness and practicability of the model.
The rest of the paper is organized in the following way: Section 2 provides an extensive literature survey on reverse logistics models and prediction models. Section 3 formulates a recycling and remanufacturing logistics network planning model based on scalable facility. Section 4 develops a model based on the improved nonlinear grey Bernoulli-Markov to predicted the recycled quantity of used products in the recycling center, and introduces the objective weighted genetic algorithm to solve the problem. Section 5 presents calculation examples for scrap automobile products to verify the validity and practicability of the model. In final section, the conclusions, as well as the future scope of this paper, are summarized.
2. Related work
Some companies and scholars favor the construction of a single-cycle and single-objective logistics network model. They consider to determine the number, the location, and the distribution of various facilities in the network within a single operating cycle to maximize net income or minimize the total cost (Dat et al., Citation2012; Ghezavati & Nia, Citation2015; Roghanian & Pazhoheshfar, Citation2014; Yu & Solvang, Citation2017). On the basis of maximizing net income or minimizing total cost, some scholars consider other factors in a single operating cycle, such as carbon subsidies (Gao et al., Citation2015), service level (Jiang et al., Citation2020), environmental impact (Ma et al., Citation2016; Nurjanni et al., Citation2017; Zhao et al., Citation2018), etc., and build a multi-objective planning model in the single-cycle. In the solution of the model, the methods, such simulated annealing algorithm (Ghezavati & Nia, Citation2015), genetic algorithm (Roghanian & Pazhoheshfar, Citation2014), and ɛ-constraint method (Jiang et al., Citation2020), seeker optimization algorithm (Subulan et al., Citation2014), etc., are mainly adopted.
The single-cycle logistics network model has many advantages. On the one hand, the model is relatively simple to construct. On the other hand, the model has strong anti-interference ability and good stability. However, from the perspective of the actual production needs of enterprises, the multi-cycle logistics network model is more consistent with actual development. With in-depth research, enterprise and academia have shifted their focus from single-cycle to multi-cycle reverse logistics network optimization. Aiming at a multi-cycle, single-product, and multi-level closed-loop logistics network, Sim et al. (Citation2004) proposed a deterministic MILP model that limited facility capacity. In their study, the minimum sum of product transportation cost and inventory cost was took as a single objective function, and genetic algorithm was adopted to solve the optimal solution. Taking into account the problem of remanufacturing facility location under uncertain environment, Mao et al. (Citation2008) established a multi-cycle, single-objective facility location model with a random parameter expected value programming model. The model was evaluated by the logistics simulation software RALC. Under the condition that the network structure and facility capacity were allowed to change gradually, Alumur et al. (Citation2012) took the maximum profit as the optimization goal and established a dynamic location model of multi-cycle single-objective remanufacturing logistics network. Considering the uncertainty in the logistics network and the selection of the location of the processing center, Wang et al. (Citation2012) constructed a multi-cycle single-objective remanufacturing closed-loop logistics network optimization design model, and used cloud genetic algorithm to solve it. Srinivasan and Khan (Citation2018) proposed a multi-product, multi-cycle, multi-facility capacitated CLSC network. The objective was to minimise the total cost by the trade-off between parts recovered and parts purchased. The model was solved by using CPLEX. On the basis of considering the cost of carbon emissions, Reddy et al. (Citation2020) proposed a mixed integer linear programming model to solve the multi-layer and multi-cycle green RL network location problem. Regarding the optimization of multi-cycle reverse logistics network, most of the previous studies constructed a single objective function model that minimizes the total cost of recycling and remanufacturing logistics network or maximizes the interest of manufacturer. There were few researches considering dynamic multi-objective function models. In fact, the recycling and remanufacturing logistics network, which is driven by multiple factors, involves multiple subjects and levels. Therefore, when a logistics network is constructed, it is not only re-examin the significance of recycling and remanufacturing of used products from the perspective of manufacturer’s interest, demand subjects, time, etc., but also include the operating costs of manufacturer and time effects in the target system.
Min and Ko (Citation2007) set different parameters for different products in an uncertain environment and considered the processing capacity of logistics facilities without distinction. They constructed a multi-cycle and multi-objective two-stage stochastic programming model. Ding et al. (Citation2014) considered the uncertainty of the demand quantity and the influence of product inventory on the location strategy. They established a multi-cycle and multi-objective dynamic location model with the goal of minimizing the total cost of the logistics network and the least negative utility to the residents. Sharifi et al. (Citation2020) provided a multi-objective, multi-period, multi-product integrated sustainable-resilient mixed-integer linear programming model for the optimal design of supply chain network under uncertainty. A novel hybrid stochastic fuzzy-robust approach was utilized to tackle the uncertainty of parameters, and the weighted sum method was applied to deal with the multi-objective problem. Samani and Hosseini-Motlagh (Citation2021) proposed a multi-period, multi-product mathematical model to minimize inefficiency, environmental impacts, and supply chain costs. Furthermore, to cope with uncertainty, a mixed robust-possibilistic programming approach is applied to the model. Hosseini-Motlagh et al. (Citation2021) emphasized the role of uncertainty in the supply chain network model, and then adopted a robust method to deal with the inevitable parameter uncertainty. In these documents that construct multi-cycle and multi-objective models, logistics facilities are not scalable. However, as the operating cycle and the recycled quantity of used products change, in order to improve the efficiency and effectiveness of the supply chain, logistics facilities should also be adjusted accordingly. Therefore, many scholars further consider the scalable facility. Tu et al. (Citation2013) proposed a multi-cycle, multi-product, multi-level closed-loop logistics network model considering facility expansion. It takes minimizing economic cost and maximizing network response speed as the objective function to select the location and distribute material flow of logistics facilities in the network. A two-stage algorithm based on evolutionary algorithm and greedy algorithm was used to solve. Ko and Evans (Citation2005) restricted the capacity of logistics facilities and allowed the expansion of logistics facilities. With the single objective function of minimizing the cost of the entire supply chain, they built a multi-cycle, multi-product, and multi-level dynamic closed-loop logistics network, combined with genetic algorithm and simple two-stage heuristic algorithm solution model composed of type transportation algorithm. Yuan and Li (Citation2008) constructed a dynamic closed-loop supply chain logistics network and established a MINLP model to determine the number of new and expanded facilities in the closed-loop supply chain network in different periods and the optimal flow between facilities.
In the recycling and remanufacturing logistics network, the recycled quantity of used products has a significant impact on the location distribution, scale size and expansion of logistics facilities (Demirel et al., Citation2016; Fleischmann et al., Citation2010; Roudbari et al., Citation2021). Therefore, the establishment of a suitable predictive model to transform uncertain factors into deterministic factors is of great significance to the construction and decision-making of the recycling and remanufacturing logistics network (Aydin et al., Citation2018). Many scholars adopted a single predictive model or method to predict the recycled quantity of used products. Some scholars focused on the relevant principles of Markov chain to predict the recycled quantity of used products. Aiming at the error of Markov forecasting method, Wu (Citation2013) improved the Markov forecasting method by using product life cycle conversion coefficient and state transition matrix. In fact, the grey model is also used more. The applicable range of the model can be expanded by optimizing the background value of the GM(1,1) model (Luo et al., Citation2003), and the initial conditions of the model can also be optimized by minimizing the mean square error to improve the performance of the GM(1,1) model (Madhi & Mohamed, Citation2017). Finally, the goal of improving the prediction accuracy of the GM(1,1) model was achieved. In addition, Jorge et al. (Citation2002) studied a fuzzy neural network method to predict the quantity of recycled products. However, in order to obtain satisfactory accuracy, it is necessary to train a large amount of sample data, so this method is not suitable for the situation of small amount of data. Wang et al. (Citation2019) proposed a DBN prediction model to improve the prediction accuracy in view of the randomness and uncertainty of remanufacturing costs, the limitations of available data samples, and the lack of shallow learning algorithms. Lv and Du (Citation2017) formulated a time-space autoregressive moving average prediction model for waste electrical and electronic products. The error parameter values of the prediction model by the maximum likelihood method were estimated.
Combined forecasting models and methods are also researched more. Toktay et al. (Citation2000) developed maximum likelihood estimation and Bayesian inference to predict the quantity of product recycled by analyzing the relationship between product demand and recycling. In order to solve the problems of a large amount of historical data and a narrow application range for the recycling prediction model, Liu and Fang (Citation2007) proposed a GM(1,1)-transfer function noise two-stage prediction model. For improving the prediction accuracy and applicability, Gao and Gao (Citation2016) analyzed the regularity of the first-order cumulative generation sequence, combined the gray model and the nonlinear regression model, and constructed a gray nonlinear regression model. In order to improve the accuracy and reliability of the recovery prediction. Xu et al. (Citation2020) combined the advantages of the GM(1,1) model and the FTS model to construct a combined prediction model.
There are many researchs on forecasting models and methods, but most of them have strict requirements on the quantity and the quality of historical statistical data. In the recycling and remanufacturing logistics network system, the recycled quantity of the used products has the characteristics of few historical statistics and large random fluctuations. It is difficult to satisfy the requirements of some prediction models and methods. Therefore, it is necessary to find a model that can accurately predict the recycled quantity of the used products through a small amount of data.
3. Multi-objective model description
3.1. Problems and assumptions
This paper is based on the scalable logistics facilities, multi-objective and multi-level recycling and remanufacturing supply chain network. The logistics network structure is illustrated in . In each operating cycle, the recycling center transports the recycled used products to the preprocessing center for preprocessing. The preprocessing process includes classification, cleaning, testing, and dismantling, etc. After the pretreatment is completed, all the waste generated is transported to the processing center for disposal. The parts that can be used for remanufacturing are transported to the manufacturer for remanufacturing. The insufficient remanufacturing demand of manufacturer will be filled by the supplier. Finally, the remanufactured product will enter the positive supply chain.
If the recycled quantity of used products is greater than the capacity of the recycling center, the recycling center is considered to expand at the original site. If the total quantity of the used products recycled by the recycling center is greater than the total capacity of the preprocessing center, the preprocessing center is considered to expand at the original site. If the total quantity of the parts available for remanufacturing in the preprocessing center is greater than the maximum processing capacity of manufacturer, the manufacturer is considered to expand at the different site, that is, a new remanufacturing center. As a result, a scalable logistics facilities location model for recycling and remanufacturing supply chain network under uncertainty is established.
For the accuracy of the model, we make the following reasonable assumptions.
The remanufacturing demand and maximum processing capacity of the manufacturer are foreseen (Gao et al., Citation2015).
The locations of the supplier, manufacturer, recycling centers, and processing centers are known and fixed. The service capabilities of the processing centers are not limited.
The candidate locations and preprocessing capabilities of the preprocessing centers are known.
The operating cycle is fixed (Ding et al., Citation2014).
The logistics between adjacent logistics facilities of the different levels is allowed. The logistics between the same level and the next-level logistics facilities is not allowed (Gao et al., Citation2015).
The remanufacturing waste rate of each preprocessing center is fixed.
The capacity of each recycling center is foreseen, and the used products recycled by the recycling centers are transported to the preprocessing centers in time, that is, each recycling center has no inventory.
The expansion cost of the original site expansion recycling center mainly refers to the management and labor cost of the recycling center. For modeling needs, unit expansion cost is converted into a quantitative expression, and is proportional to the difference between the recycled quantity and the capacity of the recycling center.
The expansion cost of the original preprocessing center mainly refers to the temporary expansion, management, and labor costs of the preprocessing center. For modeling needs, the expansion cost of each preprocessing center is converted into a quantity at a certain level.
Expanded manufacturer at a different location means that the manufacturer builds a remanufacturing center at a different site, and the location is determined. For modeling needs, the open cost of the remanufacturing center is converted into a quantity at a certain level.
3.2. Multi-objective planning model construction
3.2.1. Network model and parameter definition
Based on the scalable facility and combined with practical applications, the model includes two objective functions: (1) minimization of system costs (namely: the transportation costs, the discounted fixed cost of establishing a preprocessing center with basic processing capabilities, and the discounted cost of logistics facility expansion) and (2) minimization of the time costs related to the out-of-stock and inventory in in each operating cycle. The nodes and costs of the recycling and remanufacturing logistics network discussed in this paper are illustrated in . In the logistics network, there are multiple recycling centers, multiple preprocessing centers, a manufacturer, a remanufacturing center that the manufacturer may build, a processing center, and a supplier. When recycled quantity of used products is greater than the capacity limit of the recycling center, the recycling center is considered to expand at the original site. The recycling center classifies the recycled used products, after that, they are sent to the preprocessing center for further disassembly, testing, cleaning, classification, and storage. When the total recycled quantity of used products is greater than the total capacity of the preprocessing center, the preprocessing center is considered to expand at the original site. Remanufactured parts are send to the manufacturer. When the total quantity of remanufactured parts available for the preprocessing center is greater than the manufacturer’s maximum processing capacity, the manufacturer is considered to expand at the different site, that is, build a new remanufacturing center. The waste is send to the processing center. When the demand of manufacturer is greater than the quantity of the products transported from the preprocessing center, the manufacturer will be out of stock to replenish. On the contrary, the manufacturer generates inventory. In the forward supply chain, when the manufacturer is out of stock, the delivery time is the transportation time from the supplier to the manufacturer, which is predictable. In the recycling and remanufacturing logistics network, the delivery time is unpredictable due to the uncertainty of the time for recycling used products in recycling center. In this paper, when the manufacturer is out of stock, the supplier replenishes the manufacturer. Therefore, the two objective functions established in this paper are to ensure the balance between delay and premature remanufacturing of the manufacturer, so that the out of stock and inventory of manufacturer will not occur at the same time, and achieve lean production.
Figure 2. The nodes and costs of the recycling and remanufacturing logistics network based on scalable facility.

The notations used throughout the paper are listed, as shown in .
Table 1. Notation description.
3.2.2. Objective functions
According to the notation definition and description, two objective functions and
of the dynamic multi-objective design model are established, namely:
The objective function (1) means to minimize the transportation cost of the recycling and remanufacturing logistics network (the transportation cost from the recycling center to the preprocessing center, and the transportation cost from the preprocessing center to the manufacturer, remanufacturing center and processing center), the fixed cost of opening the preprocessing center and the cost of expanding logistics facilities. The objective function (2) indicates that based on the principle that remanufactured products can be delivered in time, the replenishment cost and inventory cost of manufacturer in each operating cycle are minimized, as well as the inventory cost of the preprocessing center.
3.2.3. Constraints
In each operation cycle, the recycled quantity of used products in the recycling center
and the capacity of preprocessing center
are considered.
Constraint (3) guarantees that the total quantity transported from a single recycling center to the preprocessing center
is equal to the recycled quantity of a single recycling center. The recycled quantity of used products in the recycling center, which is uncertainty, can be obtained through the prediction model in Section 4.1. Constraint (4) ensures that in each operating cycle, the shipment volume from the recycling center
to the preprocessing center
and the inventory of the preprocessing center
are not greater than the total capacity and expansion of a single preprocessing center
.
In each operation cycle, the demand of manufacturer and the maximum processing capacity are considered.
Constraint (5) ensures that the shipment volume from the preprocessing center to the manufacturer and the out-of-stock quantity of manufacturer are not less than the demand of manufacturer. Constraint (6) ensures that the shipment volume from the preprocessing center
to the manufacturer is not greater than the maximum processing capacity of manufacturer.
In each operation cycle, the inventory of the preprocessing center is considered.
Constraint (7) guarantees the balance of shipment and the inventory of the preprocessing center .
Constraint (8)-Constraint (10) indicate that the upper limit of the expandable recycling center, the preprocessing center, and the preprocessing center that can be opened in each operation cycle are considered.
Trying to satisfy the inventory and out-of-stock at the same time as being non-positive.
If , constraint (11) guarantees that the inventory holdings of manufacturer are not greater than the maximum allowed shortage. If
, constraint (12) guarantees that the shipment from supplier to the manufacturer is not greater than the allowable maximum shortage of manufacturer.
Constraint (13) indicates that both shipment and inventory are non-negative.
The value of binary variables are considered in constraint (14)–(18).
The remanufacturing waste rate of the preprocessing center is considered in constraint (19).
4. Solution algorithm
4.1. Prediction model of the recycled quantity
Considering that the logistics facilities can be expanded, and in view of the uncertainty of the recycled quantity of used products, the prediction model is researched to predict the recycled quantity of used products. Due to the various factors, the recycled quantity of the used products will vary in the different operating cycles of the recycling and remanufacturing logistics system. Therefore, when a predictive model is established, it is not possible to copy the existing model or adopt a certain model, but to improve the model based on the actual situation of the recycling center to make the model more targeted and practical. It is necessary to delete the data with the longest collection time in the forecast time series data and add the latest time series data. The metabolic model is adopted to process and update the time series data. The processed and updated data is more accurate in reflecting the latest characteristics and trends of time series data. The prediction of the future data is also more accurate. The Markov model is combined to correct the residuals and improve the prediction accuracy of the model. The framework of the improved nonlinear grey Bernoulli-Markov prediction model is illustrated in .
The specific steps are as follows:
Step 1: The original time series is built, as shown in Equationequation (20)(20)
(20) .
It can be processed by exponential smoothing to get a new time series as follows in Equationequation (21)(21)
(21) :
The main purpose of Step 1 is to improve the initial value of the time series, eliminate the influence of random factors on the forecast, and achieve the purpose of denoising.
Step 2: A new time series can be obtained by accumulating the data in the time series
(i.e.: 1-AGO), as shown in Equationequation (22)
(22)
(22) .
Step 3: The background value in the prediction model is optimized.
The traditional background value generation coefficient . This paper is based on the background value fitting method of the homogeneous exponential function to reconstruct the optimization model of the background value method. The specific method is as follows:
Abstract the time series as a homogeneous exponential function, namely in Equationequation (23)
(23)
(23) ,
and
are undetermined coefficients. The background value is as follows in Equationequation (24)
(24)
(24) :
The key to the background value fitting method of the homogeneous exponential function is to obtain the undetermined parameter . The specific solution method is as follows in Equationequation (25)
(25)
(25) -Equationequation (27)
(27)
(27) :
Step 4: Determine matrix B, find a, b.
The improved nonlinear Bernoulli model is used to find . After that we calculate the residual.
Step 5: Divide the state according to the residual, calculate the state transition probability, and establish the state transition matrix.
According to Markov theory, the relative residual sequence of the improved model is analyzed, and the relative residual sequence is divided according to the needs. The multiple states that are not the same are obtained. First, calculate the probability from one state to another state, and get the state transition matrix
from this. Taking
, then
shows the possibility of the relative residual error from the state
to
, and only one step is required to complete. Finally, we construct a 1-step transition probability matrix
.
The grey interval for calculating the predicted value of the model is [Ki1, Ki2]. The predicted value of the model is the average of the maximum and minimum values of the gray interval, namely:
Therefore, is got.
Step 6: Improve the initial value based on the metabolism model.
After adding to the original data sequence
and removing the first data in the sequence, the calculations from Step 1 to Step 5 are repeated to obtain
.
4.2. The improved objective weighted genetic algorithm
The objective weighted genetic algorithm is a classic algorithm for solving multi-objective optimization problems. The method is to give all objective functions a certain weight and then sum them, and aggregate them into objective functions with weights as parameters. The problem of multi-objective is transformed into a problem of single-objective optimization. By choosing different weights, different Pareto optimal solutions can be obtained. In this paper, by obtaining different uniform random weight coefficients each time, a variety of weighted objective functions are obtained, and multiple sets of Pareto optimal solutions can be obtained by optimization calculation, thereby obtaining a complete Pareto optimal frontier.
4.2.1. Algorithm coding strategy
The location problem studied in this paper includes a manufacturer, a remanufacturing center expanded by the manufacturer, recycling centers,
preprocessing center candidate points, and two demand points. Using the rand function and the round function, the decision variables
,
, and
are all randomly selected as 0 or 1. The number of variables included in
and
is
, and
is
. The decision variables
,
,
, and
represent the shipment volume, and the average random number generation function is used in conjunction with the constraint coefficient to obtain the random shipment volume that satisfies the shipment volume constraints at each stage. The number of variables included in
is
. The number of variables included in
,
, and
is
. The decision variable
represents the inventory holdings, and the average random number generation function is used with the constraint coefficients to obtain the random inventory holdings that satisfy the shipment constraints at each stage. The number of variables included in
is
. The decision variables
,
,
, and
are single numerical variables, in which the number of variables is all 1.
In the calculation example in Section 5.2, the operating cycle , the number of recycling centers
, the preprocessing center alternative location
, and the total number of decision variables is not large. If binary coding is used, when the number of recycling centers and preprocessing centers increases, the scale of facility location will increase dramatically which lead to rapid expansion of the algorithm search space. The float-encoded has the characteristics, such as shorter encoding string, convenient decoding, and higher operating efficiency. Therefore, in the algorithm, the coding method of random float-encoded is more suitable for decision variables.
If a preprocessing center is selected as a candidate point, each recycling center may ship to the preprocessing center. The preprocessing center can deliver remanufactured products to the manufacturer or the expanded remanufacturing center of manufacturer, and deliver waste to the processing center. If a preprocessing center is not selected, the recycling center cannot ship to it. The preprocessing center cannot deliver remanufactured products to the manufacturer or the expanded remanufacturing center of manufacturer. Nor can waste be transported to the processing center. Therefore, is related to
,
,
,
, and
. The value of
will affect the values of
,
,
,
, and
. Any
corresponds to
variables in
,
variables in
, and one variable in
,
, and
. If
, the variables contained in
,
,
,
, and
are not all 0. If
, the variables contained in
,
,
,
, and
are all 0. If a manufacturer expands a remanufacturing center at a different site, a certain preprocessing center selected can deliver remanufactured products to it, so
and
are related, and the value of
determines the value of
. If
, the variables contained in
are not all 0. If
, the variables contained in
are all 0. In this paper, a random generation method is employed to obtain a group of individuals with mixed codes of length 10–200, and these individuals form the initial population.
4.2.2. Genetic algorithm operation
1) Select operation
Firstly, the best and the worst individual in the current population are found. If the optimal individual of the current time is better than the original optimal individual, the optimal individual of the current time is used to replace the original optimal individual, and the optimal individual of the population can be found by comparing one by one. Similarly, the worst individual of the population can be found. Secondly, the worst individual in the current population is replaced by the original best individual, that is, the data of the best individual is directly copied to replace the worst individual, and then the roulette wheel selection is used to copy the individual into the next generation. The selection probability of each individual is proportional to its fitness value in the roulette wheel selection. Assuming that the population size is , the fitness of individual
is
, and the probability of individual
being selected is
.
2) Cross operation
A single point crossover is performed on the decision variables ,
, and
. Because the decision variable
is related to
,
,
,
, and
, they also need to use the same single point crossover as the decision variable
. The decision variables
,
,
, and
are single data, so these decision variables are not cross-operated. In the calculation example of this paper, the crossover probability is 0.75. A random number between (0,1) is obtained by using the rand function. When it is less than 0.75, among the
gene positions of the decision variable
, a cross position is randomly selected for data exchange between two individuals. Similarly, a single point crossover operation on other decision variables are performed to speed up the global search of the algorithm.
3) Mutation operation
Since the population size is much larger than the number of variable , the diversity of
individuals can be guaranteed. Therefore, if
, mutation operations are only performed on the corresponding decision variables
,
,
,
, and
. In this calculation example, non-uniform mutation is used. The mutation rate is 0.3 and the rand function is used to obtain a random number between (0,1). If it is less than 0.3, a mutation gene point is randomly selected among the genes of decision variables
,
,
,
, and
. The random increase or decrease which satisfies the constraint conditions is performed to accelerate the local search of the algorithm.
4.2.3. Fitness function
It is an important way to solve the multi-objective optimization problem by turning the multi-objective into a simple single-objective. The calculation example in Section 5.2 of this paper adopts the uniform distribution random number generator to convert the weights (uniform random weight coefficients and
) of the two objective functions (
and
).
and
are all between (0,1), and
. A new objective function
is got. Each run of the algorithm can only produce a set of optimal solutions, and the solution must be positioned at the Pareto optimal frontier, which is the image of the pareto optimal solution set in the objective function space. By obtaining different uniform random weight coefficients each time, multiple weighted objective functions are obtained, and multiple sets of Pareto optimal solutions can be obtained by performing optimization calculation. Thereby, the complete Pareto optimal frontier is obtained.
In the calculation example in Section 5.2 of this paper, the constraint condition is used as the first judging factor, and the number of times each individual violates the constraint is counted. Taking the fitness value as the second judging factor, the individual fitness is determined by the size of the fitness function value. For example, if individual A violates constraints less frequently than individual B, then individual A is considered better than individual B. If individual A and individual B violate the constraints the same number of times, but the fitness value of individual A is better than that of individual B, then individual A is better than individual B.
5. Calculation examples and analysis
5.1. Prediction of the recycled quantity of scrap automobile products
The calculation example in this paper assumes that there are eight recycling centers in different geographical locations responsible for recycling a certain used parts/components in each area. Based on the scalable facility, the prediction model constructed in Section 4.1 is adopted to recycle certain types of used from the eight recycling centers. The recycled quantity of used products that can be used for remanufacturing is predicted. According to historical statistics, the recycled quantity of used products (unit: t) in the first four operating cycles is known, as shown in .
Table 2. The recycled quantity of used products in the first four operating cycles of each recycling center.
The recycled quantity of the scrap automobile products in the eight recycling centers is predicted, as shown in .
Table 3. The predicted recycled quantity of a certain type of scrap automobile products.
5.2. Description of the calculation example
Eight recycling centers located in different geographic locations are responsible for the recycling of a certain type of used car products that can be used for remanufacturing in each area. If the recycled quantity of the used products is higher than the capacity of the recycling center, the recycling center is considered to expand at the original site. There are five preprocessing centers located in different geographical locations, and it is planned to select 2–4 preprocessing centers from the five preprocessing centers. If the total recycled quantity of the used products is higher than the total capacity of the preprocessing center, the preprocessing center is considered to expand at the original site. There is only one manufacturer. According to the actual situation, the demand in each operating cycle is different. If the total quantity of the remanufactured parts available for the preprocessing center is greater than the maximum processing capacity of manufacturer, the manufacturer is considered to expand at a different site. There is only one processing center with unlimited processing capacity. The operating cycle is . The unit transportation cost from the supplier to the manufacturer is 150 (RMB/t) in each cycle of operation. The manufacturer builds another remanufacturing center at 2000 t, and the fixed cost per unit-level opening is 90 RMB/t. The parameter settings of each recycling center
are shown in .
Table 4. Parameter settings of the recycling center .
The parameter settings of the preprocessing center are shown in .
Table 5. Parameter settings of preprocessing center
The unit transportation cost from the recycling center to the preprocessing center
is shown in .
Table 6. The unit transportation cost (RMB/t) from the recycling center to the preprocessing center
The relevant parameter settings of the manufacturer are shown in .
Table 7. The relevant parameter settings of the manufacturer.
5.3. Results and analysis
The most approximate optimal solution is obtained from the specific numerical comparison of the solution space. The total cost of the final optimization result is (RMB). The values of binary variables
,
, and
are shown in . Among the eight recycling centers, if
, the recycling center 4, 6, and 7 are expanded. If
, the recycling center 2, 4, 6, 7, and 8 are expanded. Among the five alternative points, if
, the 1, 3, 4, and 5 positions are selected to establish the preprocessing center, and the preprocessing center 5 is expanded. If
, the 1, 3, 4, and 5 positions are selected to establish the preprocessing center, and the preprocessing center 1, 3, and 5 are expanded.
Table 8. The values of binary variables ,
and
.
The shipment volume from the recycling center to the preprocessing center
is shown in for ordered real number pairs
. The former of the ordered real number pair represents the shipment volume at
, and the latter represents the shipment volume at
.
Table 9. Shipment volume from the recycling center to the preprocessing center
.
The shipment volume of the preprocessing center to the manufacturer is shown in for the ordered real number pair
. The former of the ordered real number pair represents the shipment volume at
, and the latter represents the shipment volume at
.
Table 10. Shipment volume from the preprocessing center to the manufacturer.
If , the manufacturer supplied by the supplier is 0, and there is no need to build a new remanufacturing center. If
, the manufacturer supplied by the supplier is 0, and there is no need to build a remanufacturing center in another place.
According to the values in , the total cost is the smallest when the objective function constraints are satisfied. From the value of , the maximum value is
(t), and the minimum value is
(t), which is in line with the actual transportation options. From the value of
, the maximum value is
(t), and the minimum value is
(t), which is in line with the actual transportation options.
5.4. Comparative analysis
In this section, the performance of the proposed model is further shown, especially in the effective solution set compared to non-scalable logistics facilities location for recycling and remanufacturing supply chain networks design model. The effective solution sets are shown in by employing the objective weighted genetic algorithm in Section 4.2 respectively.
The solution set formed by the scattered points is the effective solution set obtained by the algorithm.
In the case that the weighting coefficients
and
are completely randomly and independently selected, the calculation result randomly covers the entire solution set. If the weighting coefficients
and
of objective function
and
vary randomly between (0,1), the situation of
appears more, and the influence coefficient of f1 on the total cost is greater than the influence coefficient of
on the total cost.
The influence coefficient of
on the total cost gradually increases. This is mainly due to the expansion of logistics facilities, which may increase the inventory cost of the preprocessing center.
Some interesting results can be found:
The recycled quantity of used products in the recycling center has increased significantly, and the flow of materials between various logistics facilities has also increased correspondingly. But the total cost of the final optimization result has not increased much. This is conducive to the sustainability and healthy development of the recycling and remanufacturing supply chain, which can improve the enthusiasm and efficiency of the participation of all nodes in the supply chain, and lays a solid foundation for the construction of a green, environmentally friendly and energy-saving society.
The total cost of the final optimization result has increased. Mainly because the capacity of each recycling center, preprocessing center and manufacturer is considered. Meanwhile, the recycled quantity of used products of a certain area that can be used for recycling and remanufacturing scrap car products is predicted. The logistics facilities can be expanded according to the predicted recycled quantity. Although a certain fixed cost will be incurred, it meets the actual situation and needs which can be better applied in production practice.
6. Concluding remarks
6.1. Conclusions
This paper deals with recycling and remanufacturing systems through development of a dynamic multi-objective design network model that combines the scalable logistics facilities and the predicted recycled quantity of used products. The improved nonlinear grey Bernoulli-Markov prediction model is developed to predict the recycled quantity of used products in the recycling center. This model can well overcome the defects of grey GM(1,1) model, nonlinear grey Bernoulli-Markov model and Markov model. Its accuracy and stability are significantly better than GM(1,1), GM(1,1)-Markov and nonlinear grey Bernoulli-Markov models. Based on the predictions, the capacity or maximum processing capacity of each logistics facility is known. Comprehensive consideration is given to the cost of recycling, preprocessing, remanufacturing, and processing of the used product. Combined with the time issue of inventory and out-of-stock replenishment, a single-product, multi-cycle, dynamic multi-objective scalable logistics facility network model is constructed under capacity constraints. The two objective functions established can ensure the balance of delay and premature remanufacturing of the manufacturer in multiple cycles, so that the inventory and out-of-stock of manufacturer will not occur at the same time, and achieve lean production. Meanwhile, this model can realize the dynamic selection of the scale of logistics facilities, and improve the effective utilization rate of the logistics facilities of the recycling and remanufacturing logistics network.
The objective weighted genetic algorithm is adopted to transform the multi-objective optimization problem into a single-objective optimization problem by using MATLAB 9.0. As an example, a scrap automobile products calculations are analysed to further verify the effectiveness and practicability of this model. The validity and practicability of the planning model provide a useful reference for the recycling and remanufacturing logistics network of the used products.
6.2. Future directions
The proposed model has some limitations and can be extended from several viewpoints. For instance, the situation in complex environments (such as uncertain the remanufacturing waste rate of the preprocessing center, uncertain the remanufacturing demand and maximum processing capacity of the manufacturer, uncertain the operating cycle, uncertain the capacity of the recycling center, etc.) is not considered. Thus, we will conduct more in-depth research in the follow-up. Moreover, in the case of the expanded original preprocessing center and manufacturer, the expansion cost of each preprocessing center and manufacturer are converted into a quantity at a certain level. In fact, due to the influence of geographical, economic, environmental and other factors, the expansion cost is also related to them, which is also one of the issues worth studying in the future.
Disclosure statement
No potential conflict of interest was reported by the author(s).
Additional information
Funding
References
- Alkahtani, M., Ziout, A., Salah, B., Alatefi, M., Abd Elgawad, A. E. E., Badwelan, A., & Syarif, U. (2021). An insight into reverse logistics with a focus on collection systems. Sustainability, 13(2), 548. https://doi.org/10.3390/su13020548
- Alumur, S. A., Nickel, S., Saldanha-Da-Gama, F., & Verter, V. (2012). Multi-period reverse logistics network design. European Journal of Operational Research, 220(1), 67–78. https://doi.org/10.1016/j.ejor.2011.12.045
- Aydin, R., Kwong, C. K., Geda, M. W., & Okudan Kremer, G. E. (2018). Determining the optimal quantity and quality levels of used product returns for remanufacturing under multi-period and uncertain quality of returns. International Journal of Advanced Manufacturing Technology, 94(9), 4401–4414. https://doi.org/10.1007/s00170-017-1141-0
- Dat, L. Q., Linh, D. T. T., Chou, S. Y., & Yu, V. (2012). Optimizing reverse logistic costs for recycling end-of-life electrical and electronic products. Expert Systems with Applications, 39(7), 6380–6387. https://doi.org/10.1016/j.eswa.2011.12.031
- Demirel, E., Demirel, N., & Gokcen, H. (2016). A mixed integer linear programming model to optimize reverse logistics activities of end-of-life vehicles in Turkey. Journal of Cleaner Production, 112, 2101–2113. https://doi.org/10.1016/j.jclepro.2014.10.079
- Ding, Y. S., Li, X., & Dao, Y. (2014). Multi-period and multi-objective dynamic location model for remanufacturing logistics network. Chinese Journal of Management, 11(3), 428–433. https://doi.org/10.3969/j.1672-884x.2014.03.017
- Fathollahi-Fard, A. M., Dulebenets, M. A., Hajiaghaei-Keshteli, M., Tavakkoli-Moghaddam, R., & Mirzahosseinian, H. (2021). Two hybrid meta-heuristic algorithms for a dual-channel closed-loop supply chain network design problem in the tire industry under uncertainty. Advanced Engineering Informatics, 50(4), 101418. https://doi.org/10.1016/j.aei.2021.101418
- Feng, D. Z., Yu, X. H., Mao, Y. B., Ding, Y. K., Zhang, Y., & Pan, Z. Y. (2019). Pricing decision for reverse logistics system under cross-competitive take-back mode based on game theory. Sustainability, 11(24), 6984. https://doi.org/10.3390/su11246984
- Fleischmann, M., Beullens, P., Bloemhof-Ruwaard, J. M., & Wassenhove, L. N. (2010). The impact of product recovery on logistics network design. Production and Operations Management, 10(2), 156–173. https://doi.org/10.1111/j.1937-5956.2001.tb00076.x
- Gao, T. S., & Gao, B. (2016). Failure rate prediction of substation equipment combined with grey linear regression combination model. IEEE International Conference on High Voltage Engineering & Application, Chengdu, China, 1–5. https://doi.org/10.1109/ICHVE.2016.7800850
- Gao, J. H., Wang, R., & Wang, H. Y. (2015). Closed-loop supply chain network design under carbon subsidies. Computer Integrated Manufacturing Systems, 21(11), 3033–3040. https://doi.org/10.13196/j.cims.2015.11.024
- Ghezavati, V., & Nia, N. S. (2015). Development of an optimization model for product returns using genetic algorithms and simulated annealing. Soft Computing, 19(11), 3055–3069. https://doi.org/10.1007/s00500-014-1465-8
- Guo, J. Q., & Gao, Y. (2015). Optimal strategies for manufacturing/remanufacturing system with the consideration of recycled products. Computers & Industrial Engineering, 89(C), 226–234. https://doi.org/10.1016/j.cie.2014.11.020
- Hosseini-Motlagh, S. M., Samani, M., & Saadi, F. A. (2021). Strategic optimization of wheat supply chain network under uncertainty: A real case study. Operational Research, 21(3), 1487–1527. https://doi.org/10.1007/s12351-019-00515-y
- Jiang, G., Wang, Q., Wang, K., Zhang, Q., & Zhou, J. (2020). A novel closed-loop supply chain network design considering enterprise profit and service level. Sustainability, 12(2), 544. https://doi.org/10.3390/su12020544
- Jorge, M. G., Claus, R., Andreas, N., & Kruse, R. (2002). Neuro-fuzzy approach to forecast returns of scrapped products to recycling and remanufacturing. Knowledge-Based Systems, 15(1/2), 119–128. https://doi.org/10.1016/S0950-7051(01)
- Ko, H. J., & Evans, G. W. (2005). A genetic algorithm-based heuristic for the dynamic integrated forward/reverse logistics network for 3PLs. Computers & Operations Research, 34(2), 346–366. https://doi.org/10.1016/j.cor.2005.03.004
- Liu, W. J., & Fang, Z. G. (2007). Application of IGM(1,1)-TFN model in the forecasting of returns. Control Theory & Applications, 24(3), 459–464. https://doi.org/10.3969/j.1000-8152.2007.03.025
- Luo, D., Liu, S. F., & Dang, Y. G. (2003). The optimization of grey model GM(1,1). Engineering Science, 5(8), 50–53. https://doi.org/10.3969/j.1009-1742.2003.08.007
- Lv, J., & Du, S. C. (2017). Time-space modeling of waste electrical and electronic equipment return prediction. Industrial Engineering & Management, 22(6), 92–98. https://doi.org/10.19495/j.cnki.1007-5429.2017.06.013
- Ma, R. M., Yao, L. F., Jin, M. Z., Ren, P. Y., & Lv, Z. H. (2016). Robust environmental closed-loop supply chain design under uncertainty. Chaos. Chaos, Solitons & Fractals, 89, 195–202. https://doi.org/10.1016/j.chaos.2015.10.028
- Madhi, M., & Mohamed, N. (2017). An initial condition optimization approach for improving the prediction precision of a GM(1,1) model. Mathematical and Computational Applications, 22(1), 21–26. https://doi.org/10.3390/mca22010021
- Mao, H. J., Ji, X. Z., Li, X. H., He, J., & Qiao, X. N. (2008). Research on remanufacturing reverse logistics network facility location projects simulation evaluation. IEEE International Conference on Management Science and Engineering, Long Beach, CA, USA, 488–494. https://doi.org/10.1109/ICMSE.2007.4421895
- Min, H., & Ko, H. J. (2007). The dynamic design of a reverse logistics network from the perspective of third-party logistics service providers. International Journal of Production Economics, 113(1), 176–192. https://doi.org/10.1016/j.ijpe.2007.01.017
- Nili, M., Seyedhosseini, S. M., Jabalameli, M. S., & Dehghani, E. (2021). A multi-objective optimization model to sustainable closed-loop solar photovoltaic supply chain network design: A case study in Iran. Renewable and Sustainable Energy Reviews, 150. https://doi.org/10.1016/j.rser.2021.111428
- Nurjanni, K. P., Carvalho, M. S., & Costa, L. (2017). Green supply chain design: A mathematical modeling approach based on a multi-objective optimization model. International Journal of Production Economics, 183(Part B), 421–432. https://doi.org/10.1016/j.ijpe.2016.08.028
- Reddy, K. N., Kumar, A., Sarkis, J., & Tiwari, M. K. (2020). Effect of carbon tax on reverse logistics network design. Computers & Industrial Engineering, 139(1), 1–13. https://doi.org/10.1016/j.cie.2019.106184
- Ren, Y. P., Yu, D. Y., Zhang, C. Y., Tian, G. D., Meng, L. L., & Zhou, X. Q. (2017). An improved gravitational search algorithm for profit-oriented partial disassembly line balancing problem. International Journal of Production Research, 55(23–24), 7302–7316. https://doi.org/10.1080/00207543.2017.1341066
- Roghanian, E., & Pazhoheshfar, P. (2014). An optimization model for reverse logistics network under stochastic environment by using genetic algorithm. Journal of Manufacturing Systems, 33(3), 348–356. https://doi.org/10.1016/j.jmsy.2014.02.007
- Roudbari, E. S., Ghomi, S., & Sajadie, H. M. S. (2021). Reverse logistics network design for product reuse, remanufacturing, recycling and refurbishing under uncertainty. Journal of Manufacturing Systems, 60(14), 473–486. https://doi.org/10.1016/j.jmsy.2021.06.012
- Samani, M., & Hosseini-Motlagh, S. M. (2021). A mixed uncertainty approach to design a bioenergy network considering sustainability and efficiency measures. Computers & Chemical Engineering, 149(4), 107305. https://doi.org/10.1016/j.compchemeng.2021.107305
- Sharifi, M., Hosseini-Motlagh, S. M., Samani, M., & Kalhor, T. (2020). Novel resilient-sustainable strategies for second-generation biofuel network design considering neem and eruca sativa under hybrid stochastic fuzzy robust approach. Computers & Chemical Engineering, 143(1), 107073. https://doi.org/10.1016/j.compchemeng.2020.107073
- Sim, E., Jung, S., Kim, H., & Park, J. (2004). A generic network design for a closed-loop supply chain using genetic algorithm. Genetic & Evolutionary Computation Conference, Seattle, WA, USA, 1214–1225. https://doi.org/10.1007/978-3-540-24855-2_129
- song, S. X., Liu, M., Liu, G. F., & Ke, Q. D. (2016). Theories and design methods for proactive remanufacturing of modern products. Journal of Mechanical Engineering, 52(7), 133–141. https://doi.org/10.3901/JME.2016.07.133
- Srinivasan, S., & Khan, S. H. (2018). Multi-stage manufacturing/re-manufacturing facility location and allocation model under uncertain demand and return. International Journal of Advanced Manufacturing Technology, 94(14), 2847–2860. https://doi.org/10.1007/s00170-017-1066-7
- Subulan, K., Baykasoglu, A., & Saltabaş, A. (2014). An improved decoding procedure and seeker optimization algorithm for reverse logistics network design problem. Journal of Intelligent & Fuzzy Systems: Applications in Engineering and Technology, 27(6), 2703–2714. https://doi.org/10.3233/IFS-141335
- Tan, Y., & Guo, C. X. (2019). Research on two-way logistics operation with uncertain recycling quality in government multi-policy environment. Sustainability, 11(3), 882. https://doi.org/10.3390/su11030882
- Tighazoui, A., Turki, S., Sauvey, C., & Sauern, N. (2019). Optimal design of a manufacturing-remanufacturing-transport system within a reverse logistics chain. International Journal of Advanced Manufacturing Technology, 101(5–8), 1773–1791. https://doi.org/10.1007/s00170-018-2945-2
- Toktay, L. B., Wein, L. M., & Zenios, S. A. (2000). Inventory management of remanufacturable products. Management Science, 46(11), 1412–1426. https://doi.org/10.1287/mnsc.46.11.1412.12082
- Tu, N., Chang, L. F., Mai, H. D., & Dai, W. J. (2013). Multi-objective optimization of closed-loop logistics network with facility expansion. Industrial Engineering Journal, 16(5), 53–61. https://doi.org/10.3969/j.1007-7375.2013.05.009
- Wang, Z. F., Cui, Y., Wang, L., & Xie, M. (2012). Optimization for remanufacturing closed-loop logistics network under uncertain environment. Computer Engineering and Applications, 48(36), 221–226. https://doi.org/10.3778/j.1002-8331.1205-0174
- Wang, L., Xia, X. H., Cao, J. H., & Liu, X. (2019). Modeling and predicting remanufacturing time of equipment using deep belief networks. Cluster Computing, 22(S2), S2677–S2688. https://doi.org/10.1007/s10586-017-1430-2
- Wu, Y. S. (2013). Prediction method of recovery amount for closed-loop supply chain based on production life cycle. Mathematics in Practice and Theory, 43(24), 1–6. https://doi.org/10.3969/j.1000-0984.2013.24.001
- Xu, S. T., Miu, Z. W., Tan, Z., Cai, N. Z., & ShangGuan, L. L. (2020). Electronic products returns forecasting system based on a hybrid algorithm. Journal of Industrial Engineering Management, 34(1),147-153. https://doi.org/10.13587/j.cnki.jieem.2020.01.016
- Yu, H., & Solvang, W. D. (2017). A carbon-constrained stochastic optimization model with augmented multi-criteria scenario-based risk-averse solution for reverse logistics network design under uncertainty. Journal of Cleaner Production, 164(15), 1248–1267. https://doi.org/10.1016/j.jclepro.2017.07.066
- Yuan, F., & Li, H. (2008). Optimization design of a closed-loop supply chain network based on remanufacturing. Manufacture Information Engineering of China, 37(23), 1–5. https://doi.org/10.3969/j.1672-1616.2008.23.001
- Zhao, X., Xia, X. H., Wang, L., & Yu, G. D. (2018). Risk-averse facility location for green closed-loop supply chain networks design under uncertainty. Sustainability, 10(11), 4072. https://doi.org/10.3390/su10114072