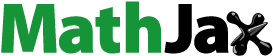
Abstract
How pedestrians find and choose routes in buildings is a fundamental research topic that is immediately relevant to building design and safety. However, few studies have explored the relationship between pedestrian route choice and building layout in a systematic way. Here, we introduce a method based on spatial network theory for generating buildings with various layout properties. We conduct a virtual experiment with over 200 participants and many generated buildings to investigate how layout properties influence different aspects of pedestrian route choice. Our findings suggest route recall is worse in buildings that have more connections and possible routes, even when the overall size of buildings and length of routes is kept constant. Pedestrians also prefer more regular building layouts and are more likely to adopt the heuristic of walking along the outer edges of buildings the less regular they are and the more connections they have.
1. Introduction
Pedestrian route choice is one of the most interesting and challenging problems for research into pedestrian behaviour (Hoogendoorn and Bovy Citation2004). Route choice behaviour of pedestrians inside buildings, especially in complex facilities, such as airports and hospitals, is often directly relevant to building design, crowd management and evacuations in emergencies. It has long been a question of great interest in a wide range of fields including engineering, mathematics, psychology, and safety science.
In general, pedestrians are assumed to perceive and process environmental spatial information through a subjective cognitive process and then choose routes based on preferences, such as the shortest distance and the fewest turns (Andresen, Chraibi, and Seyfried Citation2018). This process can depend on individual characteristics. Previous studies have established that age, gender, culture and vision ability can affect how pedestrians choose a route (Bernhoft and Carstensen Citation2008; Lawton and Kallai Citation2002; Han, Liu, and Li Citation2021). However, the factors deemed to be most important in route choice behaviour are environmental factors. Static information (time-independent), such as signs and building layout, has been distinguished from dynamic information (time-dependent), such as the level of congestion along different routes (Bode, Wagoum, and Codling Citation2014). The influence of both types of information on route choice has been widely investigated using experiments and simulations (Haghani and Sarvi Citation2017; Lovreglio et al. Citation2014). The effect of dynamic information is often tested by updating the information shown to participants in well-controlled experiments or by setting simulated rules based on assumptions in simulations. For example, Lin et al. (Citation2020) investigated how pedestrians choose their routes when presented with different split levels of crowd flow via an immersive virtual metro station experiment. In contrast, static information is more likely to be tested by comparing the route choice of pedestrians in different pre-designed buildings with specific structures. For example, Zhu et al. (Citation2020) investigated how likely pedestrians were to follow signs with different designs during evacuations. Furthermore, different information use strategies influence pedestrian route choice. For example, Lin, Zhang, and Hang (Citation2022) identified reactive pedestrians who only rely on current information to make decisions and predictive pedestrians who choose routes based on the predictive travel cost.
In addition to individual characteristics, previous research has established that pedestrian route choice depends on the context. Motivations, social influence and familiarity have been identified as essential factors affecting pedestrian route choice. Motivation indicates the travel purposes of pedestrians and affects their route choice strategies. For example, compared to commuters who prefer the shortest possible route without inclines (Sarjala Citation2019), tourists are more likely to choose routes with more pleasant visual attractions (Davies Citation2018). Social influence describes how pedestrians change their route choice in a social environment (Nicolas and Hafinaz Hassan Citation2021). For example, some studies found pedestrians in a social group that are connected via social relationships such as friendship or work relationships, prefer to stay close to each other and share the same destination (Yamaguchi et al. Citation2011; Hu et al. Citation2020). Familiarity reflects the spatial knowledge of pedestrians about the environment surrounding them (Andresen, Chraibi, and Seyfried Citation2018). Some work reveals that pedestrians are more likely to choose familiar exits even when other available exits are closer (Benthorn and Frantzich Citation1999). In contrast, pedestrians who are not familiar with the environment have to seek other information for their route choices such as the movements of others (Tong and Bode Citation2021) or signs (Ronchi, Nilsson, and Gwynne Citation2012).
A crucial but understudied aspect of pedestrian environments is the layout of buildings, that is to say, the spatial arrangement of rooms, corridors, doors, and walls. Previous work already provides evidence that building layout properties, such as curved and angled corridors, influence pedestrian movement characteristics (Jiang et al. Citation2022). Two types of research can be distinguished: first research that considers only part of the building layout and second research that considers the entire building layout. The former type of research has received much attention and focuses on specific structures, such as obstacles in front of doors, the number of doors, and exit widths (Li et al. Citation2019). In contrast, the latter type of research is concerned with investigating the influence of the entire building layout on pedestrian route choice. It has received much less attention and is the focus of our work presented here.
One of the essential concepts of investigating the influence of building layout on pedestrian route choice can be described as building layout complexity. This term reflects the condition of the geometric elements in a building and the forms of relationships among these elements. As it is a broad concept, different approaches to quantifying it exist (Hölscher and Conroy Dalton Citation2008; Stankiewicz, Legge, and Schlicht Citation2001). An important measure is the Inter Connection Density (ICD), defined by O'Neill (Citation1991) as the total number of choices at decision points divided by the number of decision points in the building. ICD measures the density of available paths between places in an environment, such as a building. Findings suggest that as the average ICD of buildings increases, individuals construct less accurate cognitive maps of buildings and their wayfinding performance, measured by the number of wrong turns and backtracking events, decreases (O'Neill Citation1991). Other work also found that people spend more time and have higher errors in wayfinding tasks in environments with higher ICD values (Slone et al. Citation2015). Going beyond the ICD, the effect of other building layout measures, such as geometrical misalignment (Werner and Schindler Citation2004), a spatial relation that measures whether the current perspective is parallel to the perspective of pedestrians when they obtain map information, and intersections of corridors with various angles (Jansen-Osmann, Schmid, and Heil Citation2007) have been investigated but no statistically significant results were found.
While the influence of ICD on pedestrian route choice has been confirmed in several studies, Werner and Schindler (Citation2004) argue that many buildings may have the same ICD values but different other geometrical attributes and pedestrians perform differently in these cases. Thus, there might be some other factors affecting pedestrian behaviour. Importantly, most previous research has only considered a limited number of manually constructed building layouts (fewer than 10). Therefore, more empirical data that involve a large number of building layouts are needed to ensure a broader range of possible layout properties is investigated.
Previous research indicates that how pedestrians interpret route information from their own subjective perspective is essential for pedestrian route choice (Shatu, Yigitcanlar, and Bunker Citation2019). Cognitive maps, the mental representations of external environments constructed by pedestrians, can capture the cognitive factors that might affect pedestrian route choice. This concept is termed by Tolman who found evidence that rats possess a clue about specific objects and their spatial relation obtained from previous visiting experiences, and that hippocampal formation is involved in the establishment of such a cognitive map (Tolman Citation1948). They found that specific cells, such as grid cells (Moser et al. Citation2008) and border cells (Solstad et al. Citation2008), play a role in spatial information perception. Similar cells that provide environmental information have also been discovered in the human brain (Ekstrom et al. Citation2003). Five elements of cognitive maps are identified: paths, nodes, districts, edges and landmarks (Lynch et al. Citation1964). Paths refer to the shared corridors, edges are limiting or enclosing features, districts are larger spaces sharing some common characters, nodes are the intersections of major paths or places, and landmarks distinctive features that people use to reference and locate themselves.
Network theory is a convenient and successful approach to represent the relations between discrete objects and has been widely used (Parkhe, Wasserman, and Ralston Citation2006). Networks can be used to represent spatial relations. When representing building layouts via networks, each node represents an intersection point, and each edge that links nodes represents the space pedestrians can walk through, such as corridors (Barthélemy Citation2011). Measures, such as the average path length (average length of connections between intersection points), have been developed to characterise properties of networks. For example, the ICD discussed above is exactly the concept of average degree – the average number of edges per node in a network. This suggests concepts in network theory are suitable for measuring building layout properties.
Previous studies have adopted various measures to create different levels of simulated stress for participants in experiments, such as imposing time pressure, giving financial incentives and presenting motivational instructions. However, studies on the effect of stress have produced diverse results. The work by Haghani, Sarvi, and Shahhoseini (Citation2020) shows stress may increase the vigilance of participants and thus decrease their reaction time, leading to a short evacuation time. In contrast, the work by Bode and Codling (Citation2013) found stress may impair pedestrian information processing ability and leads participants to choose routes that are far from optimal. Moreover, it is difficult to achieve consistent and desired participant responses or stress levels in virtual experiments. Therefore, in this work, we only present participants with motivational messages and argue that this is sufficient to test participants' responses to different building layouts.
Pedestrian route choice can be regarded as a decision-making process in terms of spatial navigation in psychological research. Tong and Bode (Citation2022) identify four processes of pedestrian route choice: ‘information perception’ considers how pedestrians perceive information in a selective and purposeful way, ‘information integration’ deals with how pedestrians subjectively integrate environmental spatial information into mental representations, ‘responding to information’ describes how pedestrians tend to respond to information individually and collectively and ‘decision-making mechanisms’ are concerned with how pedestrians trade-off the evidence and make final route choice. In each process, pedestrian behaviours change across contexts. It is almost impossible to consider all potential factors that will result in the subjectivity of pedestrian route choice. Therefore, we focus on the processes of ‘responding to information’ and ‘decision-making mechanisms’ in this work. We abstract complex buildings into networks and investigate the relationship between properties of building layouts and pedestrian route choice without considering heterogeneous cognitive maps in data analysis.
In this contribution, we first develop a method for automatically generating building layouts and then investigate the influence of building layout properties on pedestrian route recall and route choice behaviour in a virtual experiment that involves over 200 human participants. Using a virtual experiment means we can easily expose participants to a wide variety of buildings with different layout features. The paradigm of virtual experiments is established and widely used in research on pedestrian decision-making (Kinateder et al. Citation2014; Drury et al. Citation2009), as they are safe, cheap and allow exposing participants to carefully controlled environments (Lovreglio and Kinateder Citation2020). Different types of virtual reality technologies can be classified: desktop VR, head-mounted display, and cave automatic virtual environment (Feng Citation2021). In this work, we use desktop VR because it is cheaper and allows participants to attend remotely. While desktop VR provides a less immersive environment, previous work has established participants make similar route choices in different types of virtual environments (Ruddle and Péruch Citation2004; Feng Citation2021), which indicates the validity of desktop VR. While it has not yet been determined to what extent human decisions in virtual environments extend to the real world, they nevertheless provide an ideal starting point to explore what characterises building complexity and how it affects route choice. In our experiment, participants can navigate a virtual avatar to complete several route choice tasks in a large number of automatically generated buildings with different layout properties. We analyse the route choice strategies of participants and the effects of the building layout properties on pedestrian route choice behaviour.
The key novelty of this work lies in two aspects. On the one hand, the method we developed allows us to automatically generate building layouts whose properties (e.g. the average degree of their network representations) can be controlled by a few variables. This research method can be applied in other research into building design and pedestrian behaviour. On the other hand, we use large numbers of automatically generated buildings with different layout properties to test pedestrian route choice mechanisms. Our research greatly expands the available data on pedestrian behaviour in buildings with different layouts, compared to previous research that normally considers a limited number of manually constructed building layouts.
2. Experiment
2.1. Generation of spatial networks representing building layouts
The layout of a building can be represented as a spatial network composed of nodes representing intersections and lines representing paths that connect nodes (see Figure for an example). There are many methods to generate complex networks with selected properties for experiments or simulations (see Prettejohn, Berryman, and McDonnell Citation2011 for a review). In this work, we refer to previous work (Mireles de Villafranca, Connors, and Eddie Wilson Citation2017) to construct network generation algorithms but make some modifications to meet the following requirements: (1) The generated networks should be planar graphs, meaning their edges only meet at nodes. (2) The generated networks should have a wide range of properties, such as the average number of connections between nodes, to ensure the possible variability in building layouts is captured. (3) The generated networks should be comparable in spatial extent, seeing that we want to compare the route choice behaviour of people across networks.
Taking these criteria into consideration, we generate spatial networks stochastically using the following three steps. First, we generate a grid of nodes. Second, we adjust the regularity of the grid of nodes, and third, we generate two networks based on the nodes with different average degrees: Gabriel graphs and reduced Gabriel graphs, as shown in Figure .
Figure 2. Generation of spatial networks: (a) a regular grid with 25 nodes arranged; (b) adjusting the regularity of the grid of nodes; (c) the Gabriel graph based on the nodes; (d) reduced Gabriel graphs with a lower average degree.

2.1.1. Randomising the node-set
We generate a regular grid of 25 nodes arranged equally spaced in a grid (see Figure (a)) to avoid the influence of different aspect ratios of building layouts on pedestrian route choice. The distance between any two nodes is given by d units. To reduce the regularity of the grid, we implement the parameter ‘randomness’, which is defined as the maximum ratio of the coordinate offset of each node to the original coordinate and d. For example, if d = 1 unit and randomness = 0.02, for each node in the grid, its horizontal and vertical coordinates will randomly increase or decrease by no more than 0.02 units (see Figure (b) for an example). As the randomness increases, the regularity of the network decreases and overlaps of nodes may occur. To avoid this from happening, we set the randomness to be between 16% and
of d.
2.1.2. Generation of Gabriel graphs
We generate Gabriel graphs based on the grid of nodes generated in the previous step. In Gabriel graphs, two nodes are linked if a circle centred on their midpoint with diameter equal to the distance between the nodes contains no other nodes (see Figure (c)). We use a well-established algorithm to construct Gabriel graphs for a given set of nodes (Jaromczyk and Toussaint Citation1992).
2.1.3. Generation of reduced Gabriel graphs
As the average degree of Gabriel graphs has a narrow distribution, we select some generated Gabriel graphs to reduce their average degree by randomly deleting the links between some nodes (see Figure (d)). By doing this, we can obtain a certain number of networks with similar randomness but a wide range of average degrees.
The algorithm is as follows:
Calculate the average degree of the spatial network.
Calculate the degree of each node.
For each node, if its degree is greater than the average degree and no less than 2 randomly remove one of the links to other nodes.
Repeat steps 2 to 3 until no link meets the above conditions (i.e. the original average degree is used throughout).
The steps described above define the process for how one spatial network representing a building layout is generated. To obtain a wide range of network and therefore layout properties, the ‘randomness’ parameters is uniformly randomly selected from the interval [0.16,0.32], and each generated Gabriel graph has a 50% probability of being reduced (see Section 2.1.3). Using this methodology, we generate 1200 building layouts. The distribution of selected network/layout properties is shown in Figure .
Figure 3. Distributions of building layout properties (the definition of properties can be found in Table ).

Table 1. Summary of the measures of building layout and route properties.
2.2. Virtual experiment
2.2.1. Overview
To investigate the influence of building layout properties on pedestrian route choice, we conduct a virtual experiment with human participants. Participants could move on building layouts that were randomly selected from the 1200 networks we generated and they were asked to complete two different tasks twice. The first task was designed to test route recall and the second task was designed to test route choice behaviour. The experiment is described in detail below.
In our experiment, participants were shown a top-down view of a virtual environment in which a building was displayed in the form of a network (see Figure ). Participants could control an avatar represented by a red circle in two ways: by using the arrow keys on the keyboard to move forward and backwards, turn left and right, or by selecting the position they wanted the avatar to move to with the mouse, ensuring that participants who have different preferences of computer input habits can control the virtual pedestrian in their preferred way. In each task, participants were asked to move the avatar to a designated or preferred destination. We did not record any identifying information about participants. We only recorded the movements of participants inside the experiment and (optionally) the age and gender of participants. The virtual environment was implemented in Unity 3D (Version 2019.3). Ethical approval for our experiment was granted by the Ethics Committee of the Faculty of Engineering at the University of Bristol.
Figure 4. Still images of the virtual experiment as seen by participants on screen for the first task (a) and the second task (b).

At the start of the experiment, participants were shown a building floor plan and the corresponding network used to represent it to demonstrate the link between networks and buildings and to introduce the experiment (see Figure in appendix). In addition to this introduction, participants received the information that ‘In each task, task information will be shown at the bottom right of the screen. You are a person (represented by a red circle) in a building. There is no correct answer to the route choice, so please decide according to how you think you would choose in reality’.
In the first task of the experiment, participants were asked to move to a designated destination via a route of their choice (see Figure (a)). The instructions for this part were ‘Please move to the destination at the top right as soon as possible’. Once they reached the destination, they were asked to retrace their route to return to the starting point: ‘Please try to go back to where you started through the same route you came in’. The task was completed once participants reached the starting point. This task was designed to test the influence of building layout properties on route choice strategy and route recall.
In the second task, participants were asked to a designated destination in the same way as in the first task. However, when they reached the destination, another building layout was displayed (see Figure (b)). Participants were at the intersection of these two buildings, equidistant from their starting point and a new alternative destination. They were asked to move to either of these destinations via a route of their choice. The instructions were: ‘Please move to your preferred destination either at the top right or the left bottom’. The task was completed once participants reached their preferred destination. This task was designed to test the influence of building layout properties on route and destination choice.
In our experiment, participants were asked to complete a total of four tasks, two replicates each of the two tasks described above. The tasks were placed in a randomised order, ensuring that the first two tasks were different and thus making participants not do the same task in succession. To investigate learning or habituation effects arising from participants completing the same task more than once, we tested for an effect of task order on the behaviour shown by participants. For each participant, we selected six different networks at random from the 1200 networks we generated: two networks for the first task and four networks for the second task. We recorded all movements of participants inside the virtual environment.
2.2.2. Data collection
We recruited participants on the online platform ProlificFootnote1 between the 22nd and the 23rd of July 2021. Participants were paid an amount equivalent to 7.5 per hour based on the estimated time to completion (this equated to
1.7 per person). All participants were briefed on the broad purpose of the experiment and were asked to only take part once. Participants had to download an executable file for the virtual experiment onto their computer and return an output file via email upon completion of the experiment. The experiment file could only be executed once.
A total of 506 participants signed up for our experiment on Prolific: 272 participants completed their submission, 216 participants decided to leave the experiment early and 18 participants exceeded the maximum time allowed without completing their submission. Of the 272 participants who completed the experiment, 6 participants uploaded incorrect data files, 10 participants took part in the experiment twice and 55 participants failed to submit the output file. Therefore, the data from 201 participants were analysed.
Reported ages ranged from 18 to 64, with a median and average age of 23 years and 25 years, respectively (5 participants did not disclose their age). The gender distribution included 77 female participants (39.5%), 118 male participants (60.5%) and 6 participants (3%) not identifying with either of these categories or choosing not to disclose a gender. In terms of how participants moved the avatar inside the virtual experiment, 88 participants (43.78%) used the keyboard, 70 participants used the mouse (34.83%) and 43 participants (21.39%) used a mix of both controls.
2.2.3. Data analysis
We summarise the properties of routes and building layouts used in our data analysis in Table . For the first task, in which participants were asked to retrace their route, we used a measure for spatial similarity between two routes (Abraham and Lal Citation2012).
For route A and route B
, the spatial similarity between them (
) can be described as the ratio of common nodes and the total of nodes across both routes, as shown in Equation (Equation1
(1)
(1) ).
(1)
(1) Thus,
and
.
For our statistical analysis, we use generalised linear models (GLMs) with a Binomial error structure and a logit link function. We provide details on the model structure below and we confirmed the appropriateness of these models by examining residual plots. All data analysis was conducted in Matlab R2021a (MATLAB Citation2021).
3. Results
3.1. Pedestrian route choice strategies
We first investigate factors influencing the route choice of participants in the experiment. At the start of each task, participants were asked to choose their preferred route to a designated destination. Only when they reached the destination, they were informed of the following tasks. Therefore, we use their route choice data from this part of the experiment. Figure shows the distributions of the route properties introduced in Table . We find that distance, the number of turns and accumulated angle changes of routes were important factors influencing the route preferences of participants. Many participants selected the shortest routes with the smallest accumulated angle change and the smallest number of turns (see Figure (a–c)). Although overall participants were not inclined to routes with many turns greater than , there were still more people choosing routes with only one big turn than those without big turns (see Figure (d)). No one selected a route with a turn greater than
(see Figure (e)).
We found a large number of participants preferred a route with many nodes on the periphery of the network (see Figure (f)). It could be expected that using the keyboard to steer the avatar in the experiment may affect this preference. Walking in straight lines is the least effort in this case and the routes around the periphery of the network are mostly along straight lines with one big turn in a corner of the network (compare to Figure ). However, we found no evidence for an effect of the steering mechanism participants used on their edge-seeking behaviour ( =1.4769, p=0.2243) by comparing the proportion of the path on the edge of data from participants using different steering mechanism. Our statistical analysis suggests that on average, participants tended towards walking far away from the edge of the building (intercept in Table ). One possible explanation for this is that many participants preferred both the shortest route and the route with the least accumulated angle change. These routes track the leading diagonal through the network and are thus not close to the periphery of the network. For increased randomness or the average degree of the building layout, participants became more likely to select a route along the periphery of the network (parameter estimate in Table ). This implies that when participants were faced with more uncertainty caused by a less regular layout or a higher number of connections in the network leading to more possible route choices, they preferred the route on the edge of the building that is easy to remember, even though other routes are optimal in terms of distance and accumulated angle change.
Table 2. Statistical analysis of the behaviour of pedestrians walking along the periphery of spatial networks.
3.2. Route recall
As shown in Figure (a), most participants could retrace their route accurately in the first task of our experiment (route ). We test what factors influence the similarity between the original and retraced route of participants. We use our measure for route similarity which indicates the proportion of common nodes between the two routes. Importantly, we find no evidence for a difference in results between the two replicates of this experimental task using the Wilcoxon Test (p = 0.9218) and we thus combine the data from two repeated tasks in the following analysis. As explanatory variables for route similarity, we consider the factors in Table and assess if they help explain our data using likelihood-ratio tests. Based on this analysis, we exclude the randomness (Likelihood-ratio test,
, p = 0.4087) and the proportion of the path on the periphery of the network (Likelihood-ratio test,
, p = 0.5004). In addition, the correlation between the average path length and the average degree (R = −0.7019,
), between the relative number of turns and the relative distance (R = 0.7943,
), and between the number of large turns over
and the relative accumulated angle change (R = 0.5593,
), suggest one factor of each of these pairs of factors should be included into our statistical analysis to avoid multicollinearity. Therefore, the explanatory variables included in our statistical analysis are average degree, relative distance, and relative accumulated angle change, as shown in Table .
Figure 6. Distributions of measures for route recall in the first task of the experiment. (a) shows the route similarity measure, (b) the difference in length, and (c) shows the difference in accumulated angle change between the initial route and the recalled route. Negative values in (b,c) indicate that the initial route was longer or had a higher value of the accumulated angle change, respectively.

Table 3. Statistical analysis of route similarity.
Our statistical analysis shows that on average, participants tend to select a similar route to return their starting point (large positive intercept in Table implying a route similarity close to one). The average degree, relative distance and angle change all have a non-zero effect on route similarity. As all three parameter estimates were negative, the route similarity score decreases both when the building layout has a larger average degree and when the initial route chosen by participants is longer or has more turns compared to the optimal route in the network (larger relative distance and accumulated angle change, respectively; Table ). As discussed above, participants prefer the shortest route with the smallest accumulated direction changes. For many building layouts only one or very few routes that are optimal according to these factors are available. So, participants may choose this most direct route consistently. However, as the average degree of the building layout increases, the number of the possible routes also increases, which might make it more difficult for participants to choose the same route, even if they are looking for the most direct route. This could explain why increasing average degrees of building layouts had a negative effect on the route recall of participants.
In addition to route similarity, we investigate two further measures for the difference between the two routes chosen by participants: the difference in length and the difference in accumulated changes in direction between the two routes. We consider the same predictors for these measures as for route similarity but use Linear Models, to capture the positive and negative values we find. Most retraced routes are similar to the initial route participants choose (see Figure ( b,c)). This is also reflected in our statistical analysis (see low values for intercepts in Tables and ). When participants select a longer route than the shortest route to come in, they are more likely to choose a shorter retraced route (see parameter estimate for relative distance in Table ). Similarly, when participants choose a less direct route, they are more likely to choose a more direct route subsequently (see parameter estimate of accumulated angle change in Table ).
Table 4. Statistical analysis of distance using a standard linear model.
Table 5. Statistical analysis of the accumulated angle change.
3.3. Building layout preference
In the second task, participants are asked to move to a designated destination and another building layout is displayed when they reach the destination. Therefore, participants are faced with a choice: either choose the destination in the building they have walked through or select the destination in the new alternative building. We study how the properties of the building layout influence participant preference by comparing the layouts of these two buildings. We find that on average, participants prefer the destination in the first building that they are already familiar with (positive intercept in Table ) and are more likely to choose the destination in the building with a smaller randomness parameter (negative parameter estimate for the difference in Table ). In other words, participants prefer more regular building layouts. We find no evidence for an influence of average degree on destination selection (p = 0.6414). The average path length is not considered, as it is highly correlated with the average degree.
Table 6. Statistical analysis of building layout preference.
For participants who chose the destination in the new alternative building, they may make this decision because they found a preferred route in the new alternative building rather than because they preferred the building layout. To clarify this, we compare the properties of the two routes: one is the participants' previous preferred route in the first building and the other is chosen route in the new alternative building. We find that the two routes are similar in terms of relative distance and relative accumulated angle change (see Figure in the Appendix). This suggests that the preference of participants for a building layout depends on the properties of the building layout itself rather than on whether they can choose a better route in either building layout.
4. Discussion
We develop a method to generate buildings with random layout properties, conduct a virtual experiment with over 200 participants and use statistical models to explore the influence of building layout properties on pedestrian route choice behaviour. We find that increases in the average degree of building layouts represented as networks negatively affect the route recall of participants. Participants prefer the destination they are familiar with and more regular building layouts. We also observe edge-seeking behaviour of participants in that they follow the periphery of the networks representing buildings. Similar behaviour has previously been found in buildings with low or limited visibility where people walk along walls to evacuate, because it is a safe way to avoid the obstacles and find the exit when there is no visible directional information (Guo, Huang, and Wong Citation2012; Jansen-Osmann, Schmid, and Heil Citation2007). There is also considerable evidence for edge-seeking behaviour in animals such as ants (Dussutour, Deneubourg, and Fourcassié Citation2005) and mice (Saloma et al. Citation2003). The causes and mechanisms for this behaviour are likely to differ across contexts but a common aspect is that edges can be used as structural guidelines to orient and navigate in an environment. In our experiment, edge-seeking behaviour occurs more frequently with decreases in regularity and increases in average degree of building layouts. One possible explanation for this is that in the experimental environment, participants only control the movement of the virtual pedestrian, in a way that is far less labour-intensive than in reality, so they are less sensitive to distance differences between routes and thus tend to follow a specific heuristic for route choice. Another possible reason is that it presents a simple, repeatable, and low-risk route choice or even heuristic for pedestrians that avoids the effort or need to carefully evaluate alternative routes in less regular environments with many options. Although the reasons for pedestrians walking along edges may differ across a low-visibility context and abstracted route choice, for example, the fact that it occurs repeatedly suggests it may be a fundamental behaviour that may be worthy of further research.
Our findings reveal pedestrian route preferences in buildings: participants tend to select the shortest routes with the smallest accumulated direction change and number of turns. This preference for the most direct route has also been found in previous work (Hochmair and Frank Citation2000; Stigell and Schantz Citation2011). Building layouts are generated automatically in our research, which means we cannot disentangle the relative effects of route length and direction changes, as the smallest values for these factors may coincide in many buildings. Nevertheless, our experiment helps to establish a general understanding of pedestrian strategies through the data and expands the empirical database on the role of building layout in pedestrian route choice.
In the first task of our experiment, we find that as the average degree of the building layout increases, participants retrace their route less accurately. As discussed in the introduction, the average degree has also been described as Inter Connection Density (ICD) and our work confirms the role of this measure on pedestrian route recall and route choice. The consistency between this work and the results of previous studies suggests the potential and feasibility of the network method in how building layout affects pedestrian route choice. The network method allows not only to generate building layouts with controlled properties effectively but also to apply the well-established knowledge in the field of network science to explore how to capture building layout properties that have immediate relevance to pedestrian spatial decisions. For example, while the average degree in this work can measure the connections between nodes in a network, the degree distribution, the probability distribution of these degrees over the whole network, can measure the connections in another way (Yuan et al. Citation2015). Pedestrians may have more predictable route choices in a network that follows a power-law degree distribution, because in this type of network, only a few nodes have many more connections than others (Warren, Sander, and Sokolov Citation2002), probably making them have a high probability of being on the path of pedestrians. Therefore, other network summary statistics may also help to explain pedestrian route choice strategies and this could be an interesting topic for further investigation.
In the second task of our experiment, we find that participants tend to choose the route in the original building they are familiar with. The preference of pedestrians for familiar places has been suggested to be an essential factor in pedestrian route choice (Sime Citation1983). One possible explanation for this is that the uncertainty in unfamiliar places may result in spatial anxiety, which is a situation pedestrians try to avoid (Phillips et al. Citation2013). In addition, participants in our experiment prefer the more regular building layout (generated with a smaller randomness value). This is an entirely novel finding, the mechanism for which is yet to be studied. One explanation for this could centre on the perceptual fluency, the subjective feeling of ease or difficulty while processing perceptual information (Reber, Winkielman, and Schwarz Citation1998), which has been widely studied in the field of cognitive psychology (McKean et al. Citation2020). Compared with disorganised information, people prefer regular information that leads to a higher perceptual fluency (Bloch Citation1995). A limitation of this task in our experiment is that the difference in the layout properties of two buildings is limited to a range due to the constraints imposed in the layout generation. Therefore, the conclusion we draw about pedestrians choosing destinations based on layout properties rather than route properties is only valid within the range of layouts we studied and it may not be valid when there are extreme differences between building layouts. A new building generation method that can generate a wider range of layout properties that are still meaningful would be useful to explore the trade-offs between the layout and route preferences.
There are many other building layout properties (e.g. network orientation or direction) and potential factors that affect pedestrian route choice (e.g. movements of other pedestrians). However, we argue that our work is not an exhaustive examination and still can provide a starting point for future investigations.
Control over extraneous variables is essential in our experiments. We have investigated the influences of several variables on experiment results. First, we provided two types of steering mechanism participant could use to control the movements of the virtual avatar and tested whether the steering mechanism affected their behaviours. Second, participants were asked to complete several tasks in their experiment, so we randomly assigned the task order and investigated the effect of task order on participant route choice. However, there were other factors that possibly affected pedestrian route choice but were not considered. For example, the orientation of the 2D maps shown to participants implies the main movement direction is along the diagonal from bottom left to top right. This might potentially play a role in pedestrian route choice, especially for participants who had a specific orientation preference. Therefore, more empirical work on factors affecting pedestrian route choice remains to be done.
The method of generating building layouts we use is primarily controlled by three parameters: the randomness (related to the regularity of the grid of nodes), the distance between nodes and the minimum degree of nodes when generating reduced Gabriel graphs. This method allows us to automatically create a large number of networks representing buildings with different layout properties but cannot ensure the authenticity of the generated buildings, because the building layout is not abstracted from real building floor plans. We suggest that our approach is sufficient for a preliminary investigation on the role of building layout properties on pedestrian route choice. Our approach can help identify relevant factors that can then be compared with real or planned building layouts, and with pedestrian behaviour in the real world.
One of the limitations of our study comes from the method we used. We conduct our experiment in a virtual environment and participants interact with abstracted building layouts for decision-making, raising questions of the extent to which our findings extend to pedestrian behaviour (Lovreglio and Kinateder Citation2020). Our experiment also presents participants with a top-down view of an entire building layout. This could be compared to choosing a route on a map and the difference between this situation and human visual cognition in real-world settings, as well as the effects of human–computer interaction, could influence our findings. There is work that directly demonstrates the validity of the virtual experiment paradigm for pedestrian route choice and decision making (Li et al. Citation2019) and the route choice of participants in abstracted buildings can still capture their preferences (Feng, Duives, and Hoogendoorn Citation2021; Feng et al. Citation2018). Moreover, the discussion above shows that elements of our findings on route choice confirm the findings of previous research, suggesting our approach is valid. Moreover, the 2D representation of the building allows participants to have global knowledge about the building simply and quickly. This could produce new features affecting pedestrian spatial perception such as the orientation of maps. Therefore, further research on how spatial representations can affect pedestrian route choice would be useful (e.g. comparison of pedestrian route choice in 2D and 3D virtual environments).
Participants for our experiment were recruited using a dedicated platform for scientific research and the experiment was conducted online. While online recruitment allows us to collect data cheaply, effectively and flexibly, there may be issues with this type of data collection. For example, the self-selected pool of participants may not be representative for the general population and participants who are not directly supervised by researchers may show different behaviours. Research on this issue has suggested that in principle this data collection paradigm is valid, but caution is warranted (Crump, McDonnell, and Gureckis Citation2013). A possible measure that can address this issue is to conduct online and offline experiments simultaneously, aiming to obtain a truly representative sample.
5. Conclusion
Pedestrians are assumed to make route choice decisions based on the static information obtained from prior knowledge of buildings and dynamic information during walking. The role of static information, especially building layouts, has to date not received much attention. Our study investigates for the first time how building layout properties can influence the route choice of pedestrians using large numbers of automatically generated buildings with different layout features. Our work reveals that more connections in buildings negatively affect pedestrian route recall and a more regular building layout is preferred by pedestrians. The edge-seeking behaviour occurs more frequently as regularity decreases and the number of connections increases. These findings not only provide a deeper insight into how building layout affects pedestrian spatial behaviour that may be of assistance to building design but also identify several metrics for quantifying ‘building layout complexity’, an essential concept but is not clearly defined. Furthermore, this work suggests the potential and feasibility of network methods in pedestrian route choice inside buildings. Future research should be carried out to determine the role of building layout in human behaviour using real building samples and to explore the application of research results in building design and pedestrian management.
Disclosure statement
No potential conflict of interest was reported by the author(s).
Additional information
Funding
Notes
1 Prolific: Online participant recruitment for surveys and market research URL: https://prolific.co/, accessed 25 September 2021
References
- Abraham, Sajimon, and P. Sojan Lal. 2012. “Spatio-temporal Similarity of Network-constrained Moving Object Trajectories Using Sequence Alignment of Travel Locations.” Transportation Research Part C: Emerging Technologies 23: 109–123.
- Andresen, Erik, Mohcine Chraibi, and Armin Seyfried. 2018. “A Representation of Partial Spatial Knowledge: a Cognitive Map Approach for Evacuation Simulations.” Transportmetrica A: Transport Science 14 (5–6): 433–467.
- Barthélemy, Marc 2011. “Spatial Networks.” Physics Reports 499 (1): 1–101.
- Benthorn, Lars, and Håkan Frantzich. 1999. “Fire Alarm in a Public Building: How Do People Evaluate Information and Choose An Evacuation Exit?” Fire and Materials 23 (6): 311–315.
- Bernhoft, Inger Marie, and Gitte Carstensen. 2008. “Preferences and Behaviour of Pedestrians and Cyclists by Age and Gender.” Transportation Research Part F-Traffic Psychology and Behaviour 11 (2): 83–95.
- Bloch, Peter H. 1995. “Seeking the Ideal Form: Product Design and Consumer Response.” Journal of Marketing 59 (3): 16–29.
- Bode, Nikolai W. F., and Edward A. Codling. 2013. “Human Exit Route Choice in Virtual Crowd Evacuations.” Animal Behaviour 86 (2): 347–358.
- Bode, N. W. F., A. U. Kemloh Wagoum, and E. A. Codling. 2014. “Human Responses to Multiple Sources of Directional Information in Virtual Crowd Evacuations.” Journal of the Royal Society Interface 11 (91): 20130904.
- Crump, Matthew J. C., John V. McDonnell, and Todd M. Gureckis. 2013. “Evaluating Amazon's Mechanical Turk As a Tool for Experimental Behavioral Research.” PloS One 8 (3): e57410.
- Davies, Nick. 2018. “Who Walks, Where and Why? Practitioners' Observations and Perspectives on Recreational Walkers At UK Tourist Destinations.” Annals of Leisure Research 21 (5): 553–574.
- Dobson, Annette J., and Adrian G. Barnett. 2018. An Introduction to Generalized Linear Models. Boca Raton: Chapman and Hall/CRC.
- Drury, John, Chris Cocking, Steve Reicher, Andy Burton, Damian Schofield, Andrew Hardwick, Danielle Graham, and Paul Langston. 2009. “Cooperation Versus Competition in a Mass Emergency Evacuation: A New Laboratory Simulation and a New Theoretical Model.” Behavior Research Methods 41 (3): 957–970.
- Dussutour, Audrey, Jean-Louis Deneubourg, and Vincent Fourcassié. 2005. “Amplification of Individual Preferences in a Social Context: The Case of Wall-following in Ants.” Proceedings of the Royal Society B: Biological Sciences 272 (1564): 705–714.
- Ekstrom, Arne D., Michael J. Kahana, Jeremy B. Caplan, Tony A. Fields, Eve A. Isham, Ehren L. Newman, and Itzhak Fried. 2003. “Cellular Networks Underlying Human Spatial Navigation.” Nature425 (6954): 184–188.
- Feng, Yan. 2021. “Do Different Types of VR Influence Pedestrian Route Choice Behaviour? A Comparison Study of Desktop VR and HMD VR.” In Extended Abstracts of the 2021 CHI Conference on Human Factors in Computing Systems, 1–7.
- Feng, Yan, Dorine C. Duives, and Serge P. Hoogendoorn. 2021. “Using Virtual Reality to Study Pedestrian Exit Choice Behaviour During Evacuations.” Safety Science 137: 105158.
- Feng, Zhenan, Vicente A. González, Robert Amor, Ruggiero Lovreglio, and Guillermo Cabrera-Guerrero. 2018. “Immersive Virtual Reality Serious Games for Evacuation Training and Research: A Systematic Literature Review.” Computers & Education 127: 252–266.
- Guo, Ren-Yong, Hai-Jun Huang, and S. C. Wong. 2012. “Route Choice in Pedestrian Evacuation Under Conditions of Good and Zero Visibility: Experimental and Simulation Results.” Transportation Research Part B: Methodological 46 (6): 669–686.
- Haghani, Milad, and Majid Sarvi. 2017. “Stated and Revealed Exit Choices of Pedestrian Crowd Evacuees.” Transportation Research Part B: Methodological 95: 238–259.
- Haghani, Milad, Majid Sarvi, and Zahra Shahhoseini. 2020. “Evacuation Behaviour of Crowds Under High and Low Levels of Urgency: Experiments of Reaction Time, Exit Choice and Exit-choice Adaptation.” Safety Science 126: 104679.
- Han, Yanbin, Hong Liu, and Liang Li. 2021. “An Evacuation Guidance Model for Pedestrians with Limited Vision.” Transportmetrica A: Transport Science in press: 1–22. doi:10.1080/23249935.2021.1977867.
- Hochmair, Hartwig, and Andrew U. Frank. 2000. “Influence of Estimation Errors on Wayfinding-decisions in Unknown Street Networks – Analyzing the Least-angle Strategy.” Spatial Cognition and Computation2 (4): 283–313.
- Hölscher, Christoph, and Ruth Conroy Dalton. 2008. Comprehension of Layout Complexity: Effects of Architectural Expertise and Mode of Presentation. In: Gero, J.S., Goel, A.K. (eds) Design Computing and Cognition '08.Springer, Dordrecht.
- Hoogendoorn, S. P., and P. H. L. Bovy. 2004. “Pedestrian Route-choice and Activity Scheduling Theory and Models.” Transportation Research Part B: Methodological 38 (2): 169–190.
- Hu, Yanghui, Jun Zhang, Hanyi Xiao, Shuchao Cao, Xiangxia Ren, Xuanwen Liang, and Hongliu Li Weiguo Song. 2020. “Experimental Study and Analysis on Behaviours and Strategies of Social Groups and Individuals.” Safety Science 127: 104736.
- Jansen-Osmann, Petra, Juliane Schmid, and Martin Heil. 2007. “Wayfinding Behavior and Spatial Knowledge of Adults and Children in a Virtual Environment: The Role of the Environmental Structure.” Swiss Journal of Psychology 66 (1): 41–50.
- Jaromczyk, Jerzy W., and Godfried T. Toussaint. 1992. “Relative Neighborhood Graphs and Their Relatives.” Proceedings of the IEEE 80 (9): 1502–1517.
- Jiang, Kechun, Jun Zhang, Shuchao Cao, Long Xia, Xiangxia Ren, Hongliu Li, Xudong Li, Han Xu, and Weiguo Song. 2022. “Experimental Study on the Movement Characteristics of Individuals Through Angled Corridors with Different Speeds and Directions.” Transportmetrica A: Transport Science in press: 1–34. doi:10.1080/23249935.2022.2075952.
- Kinateder, Max, Enrico Ronchi, Daniel Gromer, Mathias Müller, Michael Jost, Markus Nehfischer, Andreas Mühlberger, and Paul Pauli. 2014. “Social Influence on Route Choice in a Virtual Reality Tunnel Fire.” Transportation Research Part F: Traffic Psychology and Behaviour 26: 116–125.
- Lawton, Carol A., and Janos Kallai. 2002. “Gender Differences in Wayfinding Strategies and Anxiety About Wayfinding: A Cross-Cultural Comparison.” Sex Roles 47 (9): 389–401.
- Li, Hongliu, Jun Zhang, Long Xia, Weiguo Song, and Nikolai W. F. Bode. 2019. “Comparing the Route-choice Behavior of Pedestrians Around Obstacles in a Virtual Experiment and a Field Study.” Transportation Research Part C: Emerging Technologies 107: 120–136.
- Lin, Z. Y., P Zhang, and H. L. Hang. 2022. “A Dynamic Continuum Route Choice Model for Pedestrian Flow with Mixed Crowds.” Transportmetrica A: Transport Science in press: 1–26. doi:10.1080/23249935.2022.2075951.
- Lin, Jing, Runhe Zhu, Nan Li, and Burcin Becerik-Gerber. 2020. “Do People Follow the Crowd in Building Emergency Evacuation? A Cross-cultural Immersive Virtual Reality-based Study.” Advanced Engineering Informatics 43: 101040.
- Lovreglio, Ruggiero, Dino Borri, Luigi dell'Olio, and Angel Ibeas. 2014. “A Discrete Choice Model Based on Random Utilities for Exit Choice in Emergency Evacuations.” Safety Science 62: 418–426.
- Lovreglio, Ruggiero, and Max Kinateder. 2020. “Augmented Reality for Pedestrian Evacuation Research: Promises and Limitations.” Safety Science 128: 104750.
- Lynch, Kevin. 1964. The Image of the City. Massachusetts: MIT Press.
- MATLAB. 2021. MATLAB Version 9.10.0.1602886 (R2021a). Natick, MA: The MathWorks Inc.
- McKean, Bryony, Jonathan C. Flavell, Harriet Over, and Steven P. Tipper. 2020. “Three Minutes to Change Preferences: Perceptual Fluency and Response Inhibition.” Royal Society Open Science 7 (10): 200766.
- Mireles de Villafranca, Alonso Espinosa, Richard D. Connors, and R. Eddie Wilson. 2017. “Static Traffic Assignment on Ensembles of Synthetic Road Networks.” In International Conference on Traffic and Granular Flow, 29–36. Springer.
- Moser, Edvard I., Emilio Kropff, and May-Britt Moser. 2008. “Place Cells, Grid Cells, and the Brain's Spatial Representation System.” Annual Review of Neuroscience 31 (1): 69–89.
- Nicolas, Alexandre, and Fadratul Hafinaz Hassan. 2021. “Social Groups in Pedestrian Crowds: Review of Their Influence on the Dynamics and Their Modelling.” Transportmetrica A: Transport Science in press: 1–26. doi:10.1080/23249935.2021.1970651.
- O'Neill, Michael J. 1991. “Evaluation of a Conceptual Model of Architectural Legibility.” Environment and Behavior 23 (3): 259–284.
- Parkhe, Arvind, Stanley Wasserman, and David A. Ralston. 2006. “New Frontiers in Network Theory Development.” Academy of Management Review 31 (3): 560–568.
- Phillips, Judith, Nigel Walford, Ann Hockey, Nigel Foreman, and Michael Lewis. 2013. “Older People and Outdoor Environments: Pedestrian Anxieties and Barriers in the Use of Familiar and Unfamiliar Spaces.” Geoforum 47: 113–124.
- Prettejohn, Brenton J., Matthew J. Berryman, and Mark D. McDonnell. 2011. “Methods for Generating Complex Networks with Selected Structural Properties for Simulations: A Review and Tutorial for Neuroscientists.” Frontiers in Computational Neuroscience 5: 11.
- Reber, Rolf, Piotr Winkielman, and Norbert Schwarz. 1998. “Effects of Perceptual Fluency on Affective Judgments.” Psychological Science 9 (1): 45–48.
- Ronchi, Enrico, Daniel Nilsson, and S. M. V. Gwynne. 2012. “Modelling the Impact of Emergency Exit Signs in Tunnels.” Fire Technology 48 (4): 961–988.
- Ruddle, Roy A., and Patrick Péruch. 2004. “Effects of Proprioceptive Feedback and Environmental Characteristics on Spatial Learning in Virtual Environments.” International Journal of Human-Computer Studies 60 (3): 299–326.
- Saloma, Caesar, Gay Jane Perez, Giovanni Tapang, May Lim, and Cynthia Palmes-Saloma. 2003. “Self-organized Queuing and Scale-free Behavior in Real Escape Panic.” Proceedings of the National Academy of Sciences 100 (21): 11947–11952.
- Sarjala, Satu. 2019. “Built Environment Determinants of Pedestrians' and Bicyclists' Route Choices on Commute Trips: Applying a New Grid-based Method for Measuring the Built Environment Along the Route.” Journal of Transport Geography 78: 56–69.
- Shatu, Farjana, Tan Yigitcanlar, and Jonathan Bunker. 2019. “Objective Vs. Subjective Measures of Street Environments in Pedestrian Route Choice Behaviour: Discrepancy and Correlates of Non-concordance.” Transportation Research Part A: Policy and Practice 126: 1–23.
- Sime, Jonathan D. 1983. “Affiliative Behaviour During Escape to Building Exits.” Journal of Environmental Psychology 3 (1): 21–41.
- Slone, Edward, Ford Burles, Keith Robinson, Richard M. Levy, and Giuseppe Iaria. 2015. “Floor Plan Connectivity Influences Wayfinding Performance in Virtual Environments.” Environment and Behavior47 (9): 1024–1053.
- Solstad, Trygve, Charlotte N. Boccara, Emilio Kropff, May-Britt Moser, and Edvard I. Moser. 2008. “Representation of Geometric Borders in the Entorhinal Cortex.” Science 322 (5909): 1865–1868.
- Stankiewicz, Brian J., Gordon E. Legge, and Erik Schlicht. 2001. “The Effect of Layout Complexity on Human and Ideal Navigation Performance.” Journal of Vision 1 (3): 189.
- Stigell, Erik, and Peter Schantz. 2011. “Methods for Determining Route Distances in Active Commuting – Their Validity and Reproducibility.” Journal of Transport Geography 19 (4): 563–574.
- Tolman, E. C. 1948. “Cognitive Maps in Man and Animals.” Psychological Review 55: 189–208.
- Tong, Yunhe, and Nikolai W. F. Bode. 2021. “The Value Pedestrians Attribute to Environmental Information Diminishes in Route Choice Sequences.” Transportation Research Part C: Emerging Technologies 124: 102909.
- Tong, Yunhe, and Nikolai W. F. Bode. 2022. “The Principles of Pedestrian Route Choice.” Journal of the Royal Society Interface 19 (189): 20220061.
- Warren, Christopher P., Leonard M. Sander, and Igor M. Sokolov. 2002. “Geography in a Scale-free Network Model.” Physical Review E 66 (5): 056105.
- Werner, Steffen, and Laura E. Schindler. 2004. “The Role of Spatial Reference Frames in Architecture: Misalignment Impairs Way-Finding Performance.” Environment and Behavior 36 (4): 461–482.
- Yamaguchi, Kota, Alexander C. Berg, Luis E. Ortiz, and Tamara L. Berg. 2011. “Who Are You with and Where Are You Going?” In CVPR 2011, 1345–1352. IEEE.
- Yuan, Xin, Shuai Shao, H. Eugene Stanley, and Shlomo Havlin. 2015. “How Breadth of Degree Distribution Influences Network Robustness: Comparing Localized and Random Attacks.” Physical Review E 92 (3): 032122.
- Zhu, Yu, Tao Chen, Ning Ding, Mohcine Chraibi, and Wei-Cheng Fan. 2020. “Follow the Evacuation Signs Or Surrounding People During Building Evacuation, An Experimental Study.” Physica A: Statistical Mechanics and Its Applications 560: 125156.
Appendix.
Details of statistical models
The statistical models used in Tables are Generalized Linear Models (GLMs). GLMs are a standard tool in statistics (Dobson and Barnett Citation2018), which aim to explain variability in a response variable y in terms of explanatory variables, (where
). For our models, we assume y follows a Binomial distribution and we also assume that the expectation of y,
, satisfies the following relationship:
, where
is the intercept and
are the regression coefficients corresponding to the explanatory variables.