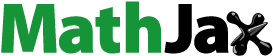
Abstract
The number of students with disabilities served under the federal law of the Individuals with Disabilities Education Act (IDEA) in public schools increased from 6.4 million to 7.0 million students from 2011 to 2017. Current curriculum offered to special needs students is eclectic and inconsistent as they vary across students, classrooms, districts, and throughout the nation. The current study examined the use of Visual Communication Analysis (VCA) within moderate to severe special education classes. Researchers found that through the use of VCA, children with various diagnoses of Autism Spectrum Disorder, Intellectual Disability, and/or Speech and Language Impairment were taught to type independently and thereby improved their learning and functional communication skills, while also showing significant decreases in maladaptive behaviors.
PUBLIC INTEREST STATEMENT
The number of students with disabilities served under the federal law of the Individuals with Disabilities Education Act (IDEA) in public schools increased from 6.4 million to 7.0 million students from 2011 to 2017. Current curriculum offered to special needs students is eclectic and inconsistent as they vary across students, classrooms, districts, and throughout the nation.
The aim of this study was to test the effectiveness of Visual Communication Analysis (VCA) as a method to teach communication and to reduce maladaptive behaviors in children with Autism, Intellectual Disability, and/or Speech and Language Impairment. VCA is the application of a well-established theory of motivation: The Self-Determination Theory. Results indicated statistically significant improvements in communication and the reduction of maladaptive behaviors. This study highlights the need for continued research and application of well-established theories of motivation to the special needs population.
1. Introduction
The rise in the number of students with disabilities served under the federal law of the Individuals with Disabilities Education Act (IDEA) in public schools increased between 2011 and 2017, from 6.4 million to 7.0 million students (National Center for Education Statistics, Citation2019). Within this population, diagnoses such as Autism Spectrum Disorder (ASD) and Intellectual Disability (ID) have been increasing in prevalence. Per the Diagnostic and Statistical Manual of Mental Disorders, Fifth Edition (DSM-5), Autism Spectrum Disorder, and Intellectual Disability affects approximately one percent of the population (American Psychiatric Association, Citation2013). However, both prevalence rates and heritability estimates vary across studies. In their most recent biennial update, the Centers for Disease Control (CDC) indicated a 15% increase in prevalence of ASD, nationally to 1 in 59 children, from 1 in 69 two years prior (Baio et al., Citation2018). In the same report, the CDC indicates that about 31% of children with ASD were classified as intellectually disabled. Speech or Language Impairment (SLI) also accounts for a significant portion of students receiving services under IDEA. In the United States, SLI’s prevalence rate is approximately 5–8% of children ages three to seventeen (U.S. Department of Health and Human Services, Citation2017).
New and veteran special education teachers may often feel overwhelmed with the broad range of subjects, grade levels, and numerous teaching strategies expected to address students’ various academic, social, physical and communication needs (Billingsley et al., Citation2009). Students with moderate to severe disabilities, such as Autism Spectrum Disorder (ASD) and Intellectual Disabilities (ID) may be learning to write their name, to speak, or other functional or academic skills much different than the typical curriculum of students at approximately the same age or grade level. The curriculums are eclectic and inconsistent as they vary across students, classrooms, districts, and throughout the nation (Agran et al., Citation2002; Ayres et al., Citation2011; Petersen, Citation2016). By law, special education teachers are required to individualize the curriculum and provide supports for each student based on their needs and abilities. As such, there can be no standardized curriculum as each student is unique in their abilities and needs. The added burden of creating materials per student coupled with the need to manage the maladaptive behaviors that are inherent in a self-contained special needs classroom leaves many special education teachers overwhelmed (Agran et al., Citation2002). Consequently, these classrooms are usually very structured and rigid and the majority are managed using token systems. There is very little autonomy in a special needs classroom even though there are many empirically based studies showing the positive relations of more autonomous forms of classroom motivations with academic outcomes (e.g., Grolnick & Ryan, Citation1989; Grolnick et al., Citation1991; Guay et al., Citation2010; Howard et al., Citation2017; Katz et al., Citation2014).
Visual Communication Analysis (VCA) is an experiential therapy that is used to teach communication and can also be used to teach academics, while building confidence and self-esteem, and ultimately decreasing maladaptive behaviors. VCA has yielded results such as significant decreases in maladaptive and self-injurious behaviors, an increase in verbalizations, and toilet training (Shkedy et al., Citation2020). Visual Communication Analysis is an application of Self-Determination Theory (SDT). SDT is a theory of intrinsic motivation that is founded on three main tenets: autonomy, competence, and relatedness, and has been used in both the general population and the special needs population, respectively (Deci & Ryan, Citation1985). Researchers Deci and Ryan (Citation1985a & Citation2000) found conditions that support an individual’s experience of autonomy, competence, and relatedness tend to foster motivation and engagement for activities, including enhanced performance, persistence, and creativity. Creating an environment where an individual perceives these three needs are met and can subsequently internalize this experience, predicts an array of positive outcomes (Ryan & Deci, Citation2020). As a result, instead of using an external reward to get a student to perform a task, VCA creates conditions that support the student’s experience of autonomy, competence, and relatedness as well as introjected, identified and integrated regulation in order to elicit motivation to complete tasks. In fact, within the VCA framework, the use of external rewards or punishment is heavily disfavored and never utilized. VCA gives students a variety of choices and perceived control when learning in order to develop intrinsic motivation, reinforced through presumed competence and the presentation of optimal challenges.
Each student receives a personalized plan in order for them to work at an optimal level of difficulty, which helps to maintain engagement and satisfies their need for competence. Relatedness support includes providing unconditional positive regard, consistent with the Rogerian approach, by withholding negative feedback and utilizing technology that provides a method of errorless learning in accordance with SDT principles. Students are met with empathy, their needs are recognized, and the instruction is given in a consistently warm and interpersonal environment. The technology utilized during VCA therapy incorporates the teachings of SDT to further meet the child’s needs for autonomy, competence, and relatedness while enhancing their intrinsic motivation.
VCA combines SDT with visual supports, prompting, and technology-aided instruction and intervention; all evidence-based strategies in supporting students, especially those with a diagnosis of Autism (Wong et al., Citation2015; Xin & Leonard, Citation2015). Technology-aided Instruction and Intervention is an evidence-based practice shown to support social, communication, behavior and school-readiness in students with Autism Spectrum Disorder (Odom et al., Citation2015). Several studies have found individuals with ASD preferred the use of technology to teacher led-instruction or pen and paper, thereby increasing attention and engagement and decreasing problem behavior (Sankardas & Rajanahally, Citation2017; Travers et al., Citation2011). Researchers note the interactive nature and stimulation inherent to technology may assist this process. From an SDT standpoint, well-structured technology can amplify that satisfaction of the need for competence by providing a very large number of iterations of optimal challenges that provide positive feedback and the opportunity for growth. Students who are taught self-determination skills early on have shown to have fewer behaviors problems (Pierson et al., Citation2008). Research indicates computer-assisted instruction in this area can help improve these skills while reducing problem behavior in students with disabilities (Mazzotti et al., Citation2020, Citation2012). The purpose of this study was to examine the efficacy of using VCA in teaching children with ASD, ID, Speech and Language Impairment to type independently as a means of expressive and functional communication. By improving this skill, researchers hypothesize an increase in psychological well-being resulting in a decrease in maladaptive behaviors as the main therapeutic benefit.
2. Method
2.1. Participants
Participants for the study were recruited from three moderate to severe special day classrooms from two different elementary school campuses in the South Bay Union School District, located in San Diego County, California. As a typical special day class represents a random assortment of diagnoses and abilities, every student in each of these classes was included in the study. Researchers obtained consent for services, data collection, and video recording from legal guardians. Every student’s legal guardian was given a consent form and they all consented to their students’ participation in the study. Review and ethics approval was obtained from Point Loma Nazarene University. The students were also provided with information regarding the study in understandable language, and all students gave their assent. All participants had a diagnosis of Autism Spectrum Disorder, Speech Language Impairment, or Intellectual Disability, and some participants had comorbid diagnoses of any combination of the three. Additionally, 13 of the students’ home language was something other than English. At the end of the study, one participant (a male) was not able to be studied due to a combination of high dosage of medications and severe behavioral issues which interfered with their time spent receiving VCA, ultimately producing a dearth of data points, which resulted in an effective amount of 27 total participants. The remaining participants’ ages ranged from 5.5 to 11.5 years; six participants were female and 21 were male (). summarizes the distribution of the disabilities of the participants who completed the study per classroom. Even though English as a second language is not a disability, it may impede learning in the English language and may contribute to maladaptive behaviors due to communication issues, and is thus included for reference.
Table 1. Demographics of participants of study
Table 2. Summary statistics of disabilities of participants of study
summarizes the distribution of the grade levels of the participants who completed the study per classroom. Class one consists primarily of the lower grades, and the other two classes have relatively older students. indicates the behavior supports required by the participants. Approximately 33% of the participants had behavior goals, 11% had a behavior intervention plan, and 11% had a Special Circumstances Instructional Assistant.
Table 3. Summary statistics of grade levels of participants of study
Table 4. Summary statistics of behavioral situation of participants of study
IEP records were used to examine the cognitive psychological testing of each of the students. The students were tested with a variety of tests with the majority tested using the Kaufman Assessment Battery for Children. The achieved scores on this test ranged from 40 to 64 which are all in the lower extreme range. The scores on the other tests (Vineland Adaptive Behavior Scales, Peabody Picture Vocabulary Test, Gilliams Autism Rating Scales) ranged from below the first percentile up to the fifth percentile. These psychological tests measure achievement, cognitive development, adaptive skills, vocabulary, and assess and diagnosis Autism. Scores in these ranges indicate a significant deficit in various cognitive and adaptive domains, and indicate very limited intellectual functioning and a very limited capacity for learning. Common behavioral goals among these group of students were as follows: “refrain from physical aggression (i.e. kicking, hitting, pushing, throwing objects),” “demonstrate appropriate expected behavior (i.e. staying on task, following directions instead of hitting self and throwing himself on the floor),” “less off-task behaviors (i.e. screaming and falling on floor),” and “will utilize positive coping strategies (i.e. take a short break, deep breaths, ask for help).”
The study was conducted over the school year starting in August 2018 and ending in June 2019. On average, a minimum of one class period per school day was allocated to using VCA and data was automatically collected via the software used to implement VCA.
2.2. Procedures
Each teacher, the class Speech Language Pathologist, and all the classroom aides were trained extensively in the implementation of VCA. The teachers allocated the first period, approximately one hour long, in the morning to implement VCA. The goal was for all students studied to participate for at least one hour per day, for five days per week. Each adult present in the classroom was trained in the tenets of SDT and utilized its methodology to give students personalized choices in their learning and not to reward any positive outcomes. One important prediction of SDT is that humans have an innate propensity to perform novel tasks (González-Cutre Et Al., Citation2016). As such, all adults were encouraged to combine the participants’ provision of choices with presentation of novel tasks as part of those choices. VCA leverages intrinsic motivation to encourage students to perform presented tasks and thereby achieves a high level of engagement. As part of this method, novel tasks (e.g., where the object changed to one the student had never seen before, the letter case changed in a way the student had never seen before, the answer changed from a word to a sentence in a way the student had never seen before, etc.) were continually presented to the students. Additionally, as time went on, the tasks presented became progressively more complex.
Each lesson consisted of the teacher or the assigned aide(s) giving the participant a set of tasks to perform using software specifically tailored for VCA, on an iPad. Depending on the severity of the students’ needs and behaviors, they were typically instructed one-on-one or with groups of two to four other students assigned to one teacher. No external rewards or token systems were used, and students were allowed to select what tasks they wanted to work on and for how long they worked on each task/subject. The software used in this study recorded every interaction (button press, key press or matching action) the student made on the iPad—a more efficient method than the typical data collection process; it additionally removed any perceived negative feedback or pressure many children feel when staff manually record data. Research indicates that when a student is cognizant they are being monitored or controlled, intrinsic motivation decreases significantly; so, when the tracking or monitoring subsides, there is very little motivation to minimize undesired behaviors (Ryan & Deci, Citation2000b; Staats et al., Citation2016; Tsai et al., Citation2008). Furthermore, most research indicates individuals display even less intrinsic motivation than before any external pressures for compliance such as tracking were utilized (Ryan & Deci, 2000a; Staats et al., Citation2016; Tsai et al., Citation2008). Therefore, data recorded in this study were covert as it was recorded exclusively by the software. The data were then stored on an external server and analyzed for this study.
All of the tasks presented were a combination of matching tasks and typing tasks where all instructions were presented simultaneously both visually and orally (by staff). The purpose of the matching tasks was twofold. The first was to familiarize the student with the concept of matching in order to understand that in essence typing is a different form of matching where each key matches the letter in the target word. The second was to prime them with the label for each object presented. There were at least four different matching tasks that were utilized depending on the student’s abilities. The first was picture-to-picture matching where five target photos were presented simultaneously on the top and bottom row of the iPad. The student had to drag the matching photo from the bottom row on the iPad up to the corresponding photo in the top row. On Level 1, all the photos lined up in the same order on the top and bottom row. On Level 2, the order of the photos on the bottom row was randomized. On Level 3, the bottom row was changed to word that corresponded with the picture, but the words were placed in the same order as the pictures on the top row. On Level 4, the words on the bottom row were randomized. The software used, automatically randomized which photos from the total pool of available photos were presented on each level, based on a preselected list of desired words to teach, so that each subsequent matching task differs from prior tasks, even though some photos may remain the same.
The typing task consisted of presenting the student with a single photo and having them type the word corresponding to the photo—for instance, the child was prompted to type “apple” when presented with a photo of an apple. On Level 1, the students were shown a silhouette of the correct spelling of the word. The key on the keyboard that needed to be typed would also blink every two seconds. On Level 2, the student was shown a silhouette of the correct spelling of the word, but the keyboard no longer blinked. On Level 3, the permanent silhouette was removed; the children were instead provided with a “help” button that would flash the silhouette of the correct spelling of the word for two seconds. On level 4, the student was given no help and needed to type the answer from memory. Every student started typing at level 1 and depending on performance progressed to Levels 2, 3, and 4.
At the teacher’s discretion, if a task was considered too easy for the child, they were moved up to the next level and if it was too difficult, they went back down a level. All words were initially presented in upper case only. If the student showed improvement with this method, words were additionally presented in lower case. Finally, if improvement was shown in both, the case was randomly selected by the software each time a new task was loaded. As the student progressed, the answer to the typing question was changed to a full sentence, e.g., “this is an apple,” “this is a green apple,” or “the apple is green.” Academic content areas included nouns, calendar, colors, shapes, numbers, counting, life cycles, money identification, following directions, and visual perception. Students were allowed to partake in the selection of a particular content area. The content in many of these applications were customizable to include categories of words that a student tended to prefer working with (such as insects, vehicles, food, etc.), and prompting was individualized to support each student’s level of difficulty and need. Additional choices available to the students were the order of lessons, how long to work on each task, and whether they did matching or typing. Each student was allowed to stand or sit on a chair or on the floor.
2.3. Dependent variable
The use of correct rates as a dependent variable is well known in research. In Eckert et al. (Citation2002) research of reading fluency, they used words correct per minute as the dependent variable. Duhon et al. (Citation2004), used digits correct per minute as the dependent variable in their study of 2 × 2 digit and 3 × 3 digit multiplication. Rhymer et al. (Citation2002) used problems correct per minute as the dependent variable in their study of interventions in increasing math fluency, and McKenna (Citation2011) used digits correct per minute in a study of interventions to teach linear algebra.
Similarly to teaching linear algebra, where the concept remains the same but the task presented is different, teaching typing across different domains involves similar concepts where the tasks presented are different. Therefore, in order to investigate typing fluency, the dependent variable used in this study is letters correct per minute (LCPM). During each session, each student was given the opportunity to type different words, and each eventually progressed to typing partial and full sentences. The number of different words presented varied by student and was changed based on the choices and progress of the student. In order to ensure validity of the data every keystroke made on the iPad was automatically timestamped and stored in a database. This measurement accounts for both rate and accuracy of learning similar to subtests (e.g., Coding, Symbol Search, Block Design) on the Weschler Intelligence Scale for Children (WISC) which calculates Intelligence Quotient (IQ) based on both crystallized intelligence and fluid intelligence indicating the child’s ability to learn, problem-solve, etc.
2.4. Independent variable
The independent variable for this study was the instruction time each student received. Since the software automatically timestamped and stored every interaction with the iPad, the time elapsed between interactions could be calculated. An interaction is any touch on the screen of the iPad made by the participant. If the time elapsed is small enough, then the student was considered to be paying attention. If the time elapsed was large, then the student was considered to be no longer attending and therefore not receiving instruction. The choice of the bound for this calculation is similar to the choice of a step size in numerical integration—i.e. the smaller the value, the smaller the approximation error. However, unlike numerical integration, if the bound gets too small, the error increases since the student may be thinking and still paying attention but not interacting with the iPad.
For this study, the bound was set to 60 seconds or approximately 0.00069 days; so, if the elapsed time between two interactions on the iPad was less than 60 seconds, then the student had attended for that period of time. For comparative purposes, the average elapsed time between interactions was calculated for each student; the minimum average elapsed time was 7.65 seconds and the maximum average elapsed time was 54.8 seconds. The average break time was also calculated for each student. The minimum average break time was 139 seconds and the maximum was 1600 seconds. It is interesting to note that, in general, the students with the minimum average interaction time were the ones with the smallest average break time. Thus, a bound of 60 seconds is accurate for this study. As such, the independent variable for each day was set to the sum of elapsed time changes up to that day, i.e. the cumulative instruction time up to that point.
2.5. Study design
A multiple-baseline across subjects design was used in this study since learning to type is an irreversible condition and is therefore not suitable for an A-B-A type design. Further complicating the design is the fact that during intervention, a stable terminal state can never be reached, as the tasks presented are constantly being modified in order to maintain engagement, and so there will always be fluctuations in the student’s ability to type. As a result, visual analysis of the study data is inappropriate, and thus statistical tests were employed to test results.
Typing is not an innate skill and is typically not part of a school curriculum for children with special needs. Prior to this study, typing was not taught at the participants’ schools and so each student had no previous experience typing. Since learning to type is irreversible, it is not possible to test typing skill without teaching students to type, and any attempt to measure the baseline would influence future measurements and contaminate the willingness of the students to participate in the study. Therefore, it is assumed that at time zero of the study the number of correct letters a student can type per minute is zero.
Since the LCPM are auto correlated due to the connection between day-to-day typing abilities, time series was used to analyze the data. The model used was a variant of ARMAX, which is regression on an exogenous variable with autoregressive moving average (ARMA) errors. The reader is referred to Mills (Citation1990) and Hyndman (Citation2010) for a more comprehensive explanation of the ARMAX statistical method. Since learning is not a smooth process, the series, LC, was set equal to the 5-day moving average of the LCPM that was calculated every day. This series was then regressed against the cumulative instruction time for all tasks received to that day (EquationEquation 1(1)
(1) ) and the intercept was set to zero to reflect the baseline of each participant. The errors were modeled as an ARMA(1,0) process (EquationEquation 2
(2)
(2) ) where LCi is the 5-day moving average of LCPM on day i, Ii is the cumulative instruction time in days as measured on the iPad, ui is the regression error term at day i,
is the autocorrelation coefficient, and
is a normal error term. Using a model of this form allows for the determination of
given that the process is autocorrelated. It should be noted that instruction time also includes the time the student spent on matching tasks.
The advantage of this model is that it allows for the usual interpretation of the regression coefficients, meaning that one can statistically determine the relationship between the dependent and independent variables. At the conclusion of the analysis of the multiple-baseline across subjects, a random effects meta-analysis on correlation was used to aggregate the results of the individual studies to determine the overall effects of the combined study. The same technique was then used on different subsets of students.
3. Findings
By design, the results of each participant were analyzed separately. The data were extracted from the database and an ARMAX analysis was performed on the data for each participant. The first step was to ensure that the time series was stationary for each participant. This was accomplished by examining the absolute values of the complex roots of the resulting AR(1) equation and verifying that it was greater than 1.00. As long as the absolute value of the roots of the equation are greater than 1.00, i.e. outside the unit circle, the process is stationary. The results of each of the ARMAX analysis summary statistics are shown for each class in and , corresponding to each class number. Note that the number of data points differs for each student as they were able to choose the days they wanted to type, match, or both. As can be seen in these tables, each of the studies was stationary and statistically significant.
Table 5-1. ARMAX analysis summary statistics for class 1
Table 5-2. ARMAX analysis summary statistics for class 2
Table 5-3. ARMAX analysis summary statistics for class 3
See below, for the solution coefficients for the regression equation (EquationEquation 1(1)
(1) ) and the coefficients for the ARMA(1,0) process (EquationEquation 2
(2)
(2) ) in separate rows for each participant. In addition to the value, the standard error, calculated t-test, and statistical significance for each parameter is listed on the same row.
Table 6-1. ARMAX analysis summary for variables predicting letters correct per minute for class 1
Table 6-2. ARMAX analysis summary for variables predicting letters correct per minute for class 2
Table 6-3. ARMAX analysis summary for variables predicting letters correct per minute for class 3
Each of the above studies can be considered as investigation of the typing efficiency of each participant when presented with a random task measured against the amount of instruction they have received. A random effects meta-analysis on correlation was performed grouping all participants together, using Hedge’s methods (Hedges & Vevea, Citation1998). Using the correlations for each study listed in and , and, the between study variance was calculated to be 0.18, and using Fisher’s r-Z transformation, the mean effect size was calculated to be 0.481. The standard error of the mean effect size was 0.085. Therefore, a 95% confidence interval [0.27, 0.54] of correlation indicates a consistent positive effect of VCA-based instruction on typing efficiency.
Using the same technique on the subset of students with a diagnosis of intellectual disability, a random effects meta-analysis on correlation was performed. The between study variance was calculated to be 0.18, and using Fisher’s r-Z transformation, the mean effect size was calculated to be 0.515. The standard error of the mean effect size was 0.099. Therefore, a 95% confidence interval [0.25, 0.65] of correlation indicates a consistent positive effect of VCA-based instruction on typing efficiency for students with ID.
Next a random effects meta-analysis on correlation was performed on the subset of students with a diagnosis of autism. The between study variance was calculated to be 0.15, and using Fisher’s r-Z transformation, the mean effect size was calculated to be 0.441. The standard error of the mean effect size was 0.117. Therefore, a 95% confidence interval [0.25, 0.61] of correlation indicates a consistent positive effect of VCA-based instruction on typing efficiency for students with autism.
Using the subset of students with a diagnosis of speech and language impairment, a random effects meta-analysis on correlation was performed. The between study variance was calculated to be 0.267, and using Fisher’s r-Z transformation, the mean effect size was calculated to be 0.392. The standard error of the mean effect size was 0.388. Therefore, a 95% confidence interval [0.10, 0.59] of correlation indicates a consistent positive effect of VCA-based instruction on typing efficiency for students with speech and language impairment.
A random effects meta-analysis on correlation was performed on the subset of students with English as a second language. The between study variance was calculated to be 0.151, and using Fisher’s r-Z transformation, the mean effect size was calculated to be 0.639. The standard error of the mean effect size was 0.153. Therefore, a 95% confidence interval [0.34, 0.74] of correlation indicates a consistent positive effect of VCA-based instruction on typing efficiency for students with English as a second language.
Next, a random effects meta-analysis on correlation was performed on the subset of students with a diagnosis of autism comorbid with intellectual disability. The between study variance was calculated to be 0.150, and using Fisher’s r-Z transformation, the mean effect size was calculated to be 0.457. The standard error of the mean effect size was 0.165. Therefore, a 95% confidence interval [0.14, 0.66] of correlation indicates a consistent positive effect of VCA-based instruction on typing efficiency for students with autism and intellectual disability.
Finally, using the subset of students with a diagnosis of speech and language impairment comorbid with intellectual disability, a random effects meta-analysis on correlation was performed. The between study variance was calculated to be 0.236, and using Fisher’s r-Z transformation, the mean effect size was calculated to be 0.385. The standard error of the mean effect size was 0.178. Therefore, a 95% confidence interval [0.02, 0.61] of correlation indicates a consistent positive effect of VCA-based instruction on typing efficiency for students with speech and language impairment and intellectual disability.
4. Discussion
Researchers hypothesized that with the use of Visual Communication Analysis, participants would learn to type and thereby improve their learning and functional communication skills. As reported above, for each student, this hypothesis was supported by the data. These numbers not only represent learning and accuracy but also the participants’ success with novel tasks. Each participant engaged in new tasks and progressively more difficult tasks, during each lesson, while maintaining a relatively reasonable LCPM. Since students randomly received instruction from different groups of instructors, in different locations with a different set of randomly assigned and chosen tasks, it is reasonable to assume that each of these single studies was independent.
The random effects meta-analysis on the whole group confirms that there is a positive effect of VCA-based instruction on typing efficiency. The examination of each of the subgroups as well as the subgroups with comorbidity of intellectual disability also confirms that there is a positive effect of VCA-based instruction on typing efficiency irrespective of the diagnosis.
This data challenges traditional approaches to testing and teaching children with special needs since results from traditional testing indicated that these students were unable to learn and therefore these outcomes should not have been possible. Typing, especially for special needs students, was dropped from the curriculum in the 1970s and has now proven to be an effective means of promoting expressive communication while decreasing maladaptive behaviors. Overall, this data is meaningful for professionals, parents, and educators alike, as it can inform a child’s actual cognitive abilities, treatment plans and Individualized Education Plans (IEP), and most importantly, give each child a proper means to communicate through typing. Another study utilizing VCA in a purely clinical setting demonstrated an increase in functional communication and a decrease in maladaptive and self-injurious behaviors for children with severe Autism (Shkedy et al., Citation2020). A comparative study utilizing VCA software as an education tool for a “mild to moderate” special education class found significant growth in reading ability compared to their peers who did not receive VCA (CitationSteagall, in review). These studies further support the efficacy of VCA in achieving various goals for children with special needs.
The researchers attribute the children’s willingness to progress through VCA to the application of the Self-Determination Theory, which was embedded in all aspects of the therapy used in this study. The researchers believe that through the use of VCA, which created supportive conditions, the participants were likely able to achieve a sense of autonomy, competence, and relatedness producing motivation to continue to progress and learn the skills necessary to establish their own voice. This belief is based on the more than 30 years of research on SDT indicating these results when all three psychological needs (autonomy, competence, and relatedness) are met in the absence of external rewards and punishments (Deci & Ryan, Citation1985, Citation2000, Citation2010; Ryan & Deci, Citation2000a, Citation2017, Citation2020).
5. Conclusions
5.1. Limitations
In the current study, the time dedicated to the study varied from class to class based on the teachers’ expectations and preferences. Another limitation of this study is that researchers are unable to accurately assess any influence from outside therapies. Participants did not necessarily report any outside therapies used to the school district but researchers are unable to control this. An additional limitation is the lack of standardized assessments used prior to the beginning of the study. Since there is not a standard battery of assessment across school psychologists, not all participants were given the same assessments. Also, there are age limitations on various assessments so that younger participants often were unable to be given the same assessments as older participants.
The demographics of the participants may also be considered limitations. Participant ages varied from 5.5 to 11.6 years of age, with a mean age 9.4 years, and less than 25% of the participants were female. Other anecdotal evidence suggests VCA may be efficacious in preschool-aged children. However, these children were given VCA at another location, outside of the current school district, and so researchers were unable to include this data in the current study.
6. Recommendations
This research proves to be very promising for the special needs community. The use of VCA can serve to help those with various other neurodevelopmental disorders or learning disorders, and even English as a second language (ESL) students. VCA has applied research on brain function and coupled it with Self-Determination Theory in technology as well as SDT in the delivery of services. This innovative experiential therapy could be expanded for use in various regional centers, special education classes, jump start classrooms, etc. Additionally, future studies can attempt to recruit younger children in order to assess efficacy within this age group. Lastly, this research also provides support for the need to utilize well-established theories within the special needs population and the classroom setting.
Compliance with ethical standards
All authors declare no conflict of interest or competing interest.
Funding was not provided for this research.
All authors participated in various roles of this research project.
Data is in depository and available upon request.
Consent to participate was obtained from all participants prior to this study.
Code availability not applicable.
Acknowledgements
We would like to express our deepest gratitude to Bruce Cochrane, Director of Special Education and Tom Bevilacqua, Coordinator of Special Education Services of the South Bay Union School District for their support in the planning and development of this research work.
Additional information
Funding
References
- Agran, M., Alper, S., & Wehmeyer, M. (2002). Access to the general curriculum for students with significant disabilities: What it means to teachers. Education and Training in Mental Retardation and Developmental Disabilities, 37(2), 123-133https://www.jstor.org/stable/23879820
- American Psychiatric Association. (2013) . Diagnostic and statistical manual of mental disorders (DSM-5®). American Psychiatric Publishing.
- Ayres, K. M., Lowrey, K. A., Douglas, K. H., & Sievers, C. (2011). I can identify Saturn but I can’t brush my teeth: What happens when the curricular focus for students with severe disabilities shifts. Education and Training in Autism and Developmental Disabilities.
- Baio, J., Wiggins, L., Christensen, D. L., Maenner, M. J., Daniels, J., Warren, Z., Kurzius- Spencer, M., Zahorodny, W., Robinson, C., Rosenberg, W. T., Durkin, M., Imm, P., Nikolaou, L., Yeargin-Allsopp, M., Lee, L., Harrington, R., Lopez, M., Fitzgerald, R., Hewitt, A., Pettygrove, S., … Durkin, M. S. (2018). Prevalence of autism spectrum disorder among children aged 8 years—Autism and developmental disabilities monitoring network, 11 sites, United States, 2014. MMWR Surveillance Summaries, 67(6), 1. https://doi.org/10.15585/mmwr.ss6706a1
- Billingsley, B. S., Griffin, C. C., Smith, S. J., Kamman, M., & Israel, M. (2009). A review of teacher induction in special education: Research, practice, and technology solutions. NCIIP document number RS-1. National Center to Inform Policy and Practice in Special Education Professional Development. 34(1), 37-43 https://doi.org/10.1177/875687051503400108
- Deci, E. L., & Ryan, R. M. (1985). Cognitive evaluation theory. In Intrinsic motivation and self- determination in human behavior (pp. 43–15). Springer, Boston, MA. https://doi.org/10.1007/978-1-4899-2271-7_3
- Deci, E. L., & Ryan, R. M. (2000). The “what” and “why” of goal pursuits: Human needs and the self-determination of behavior.. Psychological Inquiry, 11(4), 227–268. https://doi.org/10.1207/S15327965PLI1104_01
- Deci, E. L., & Ryan, R. M. (2010). Intrinsic motivation. The Corsini Encyclopedia of Psychology.
- Duhon, G. J., Noell, G. H., Witt, J. C., Freeland, J. T., Dufrene, B. A., & Gilbertson, D. N. (2004). Identifying academic skill and performance deficits: The experimental analysis of brief assessments of academic skills. School Psychology Review, 33(3), 429–443. https://doi.org/10.1080/02796015.2004.12086260
- Eckert, T. L., Ardoin, S. P., Daly, E. J., III, & Martens, B. K. (2002). Improving oral reading fluency: A brief experimental analysis of combining an antecedent intervention with consequences. Journal of Applied Behavior Analysis, 35(3), 271–281. https://doi.org/10.1901/jaba.2002.35-271
- González-Cutre, D., Sicilia, Á., Sierra, A. C., Ferriz, R., & Hagger, M. S. (2016). Understanding the need for novelty from the perspective of self-determination theory. Personality and Individual Differences, 102, 159–169. https://doi.org/10.1016/j.paid.2016.06.036
- Grolnick, W. S., & Ryan, R. M. (1989). Parent styles associated with children’s self-regulation and competence in school. Journal of Educational Psychology, 81(2), 143–154. https://doi.org/10.1037/0022-0663.81.2.143
- Grolnick, W. S., Ryan, R. M., & Deci, E. L. (1991). Inner resources for school achievement: Motivational mediators of children’s perceptions of their parents.. Journal of Educational Psychology, 83(4), 508–517. https://doi.org/10.1037/0022-0663.83.4.508
- Guay, F., Ratelle, C. F., Roy, A., & Litalien, D. (2010). Academic self-concept, autonomous academic motivation, and academic achievement: Mediating and additive effects. Learning and Individual Differences, 20(6), 644–653. https://doi.org/10.1016/j.lindif.2010.08.001
- Hedges, L. V., & Vevea, J. L. (1998). Fixed- and random-effects models in meta-analysis. Psychological Methods, 3(4), 486–504. https://doi.org/10.1037/1082-989X.3.4.486
- Howard, J. L., Gagné, M., & Bureau, J. S. (2017). Testing a continuum structure of Self-determined motivation: A meta-analysis. Psychological Bulletin, 143(12), 1346–1377. https://doi.org/10.1037/bul0000125
- Hyndman, R. J. (2010). The ARIMAX model muddle. Retrieved from https://robjhyndman.com/hyndsight/arimax/.
- Katz, I., Eilot, K., & Nevo, N. (2014). “I’ll do it later”: Type of motivation, self-efficacy and homework procrastination. Motivation and Emotion, 38(1), 111–119. https://doi.org/10.1007/s11031-013-9366-1
- Mazzotti, V. L., Rowe, D. A., Kwiatek, S., Voggt, A., Chang, W. H., Fowler, C. H., Poppen, M., Sinclari, J., & Test, D. W. (2020). Secondary transition predictors of postschool success: An update to the research base. Career Development and Transition for Exceptional Individuals, 44(1), 47-64. 2165143420959793.
- Mazzotti, V. L., Wood, C. L., Test, D. W., & Fowler, C. H. (2012). Effects of computer-assisted instruction on students’ knowledge of the self-determined learning model of instruction and disruptive behavior. The Journal of Special Education, 45(4), 216–226. https://doi.org/10.1177/0022466910362261
- McKenna, B. (2011). Using Alternating Treatment Design to Determine Math Interventions For Linear Equations. ( Masters theses, Eastern Illinois University).
- Mills, T. C. (1990). Time series techniques for economists. Cambridge University Press.
- National Center for Education Statistics. (2019). Children and youth with disabilities. U.S. Department of Education. Retrieved from https://nces.ed.gov/programs/coe/indicator_cgg.asp
- Odom, S. L., Thompson, J. L., Hedges, S., Boyd, B. A., Dykstra, J. R., Duda, M. A., Szidon, K., Smith, L., & Bord, A. (2015). Technology-aided interventions and instruction for adolescents with autism spectrum disorder. Journal of Autism and Developmental Disorders, 45(12), 3805–3819. https://doi.org/10.1007/s10803-014-2320-6
- Petersen, A. (2016). Perspectives of special education teachers on general education curriculum access: Preliminary results. Research and Practice for Persons with Severe Disabilities, 41(1), 19–35. https://doi.org/10.1177/1540796915604835
- Pierson, M. R., Carter, E. W., Lane, K. L., & Glaeser, B. C. (2008). Factors influencing the self- determination of transition-age youth with high-incidence disabilities. Career Development for Exceptional Individuals, 31(2), 115–125. https://doi.org/10.1177/0885728808317659
- Rhymer, K. N., Skinner, C. H., Jackson, S., McNeill, S., Smith, T., & Jackson, B. (2002). The 1- minute explicit timing intervention: The influence of mathematics problem difficulty. Journal of Instructional Psychology, 29(4), 305–311. https://psycnet.apa.org/record/2002-11085-011
- Ryan, R. M., & Deci, E. L. (2000a). Self-determination theory and the facilitation of intrinsic motivation, social development, and well-being. American Psychologist, 55(1), 68–78. https://doi.org/10.1037/0003-066X.55.1.68
- Ryan, R. M., & Deci, E. L. (2000b). Intrinsic and extrinsic motivations: Classic definitions and new directions. Contemporary Educational Psychology, 25(1), 54–67. https://doi.org/10.1006/ceps.1999.1020
- Ryan, R. M., & Deci, E. L. (2017). Self-determination theory: Basic psychological needs in motivation, development, and wellness. Guilford Publications.
- Ryan, R. M., & Deci, E. L. (2020). Intrinsic and extrinsic motivation from a self-determination theory perspective: Definitions, theory, practices, and future directions. Contemporary Educational Psychology, 61, 101860. https://doi.org/10.1016/j.cedpsych.2020.101860
- Sankardas, S. A., & Rajanahally, J. (2017). iPad: Efficacy of electronic devices to help children with Autism spectrum disorder to communicate in the classroom. Support for Learning, 32(2), 144–157. https://doi.org/10.1111/1467-9604.12160
- Shkedy, G., Shkedy, D., Sandoval-Norton, A. H., & Fantaroni, G. (2020). Visual communication analysis (VCA): Applying self-determination theory and research-based practices to autism. Cogent Psychology, 7(1), 1803581. https://doi.org/10.1080/23311908.2020.1803581
- Staats, B. R., Dai, H., Hofmann, D., & Milkman, K. L. (2016). Motivating process compliance through individual electronic monitoring: An empirical examination of hand hygiene in healthcare. Management Science, 63(5), 1563–1585. https://doi.org/10.1287/mnsc.2015.2400
- Steagall, K. (in review). An Exploration of Visual Communication Analysis (VCA) and its effects on elementary aged students with mild to moderate disabilities. Masters Thesis. Point Loma Nazarene University.
- Travers, J. C., Higgins, K., Pierce, T., Boone, R., Miller, S., & Tandy, R. (2011). Emergent literacy skills of preschool students with Autism: A comparison of teacher-led and computer-assisted instruction. Education and Training in Autism and Developmental Disabilities, 46(3), 326–338. https://www.jstor.org/stable/23880589
- Tsai, Y., Kunter, M., Lüdtke, O., Trautwein, U., & Ryan, R. M. (2008). What makes lessons interesting? The role of situational and individual factors in three school subjects. Journal of Educational Psychology, 100(2), 460–472. https://doi.org/10.1037/0022-0663.100.2.460
- U.S. Department of Health and Human Services. (2017). Quick statistics about voice, speech, language. National Institute on Deafness and Other Communication Disorders. Retrieved from https://www.nidcd.nih.gov/health/statistics/quick-statistics-voice-speech-language
- Wong, C., Odom, S. L., Hume, K. A., Cox, A. W., Fettig, A., Kucharczyk, S., Brock, M., Plavnik, J., Fleury, V., & Schultz, T. R. (2015). Evidence-based practices for children, youth, and young adults with Autism spectrum disorder: A comprehensive review. Journal Of Autism And Developmental Disorders, 45(7), 1951–1966. https://doi.org/10.1007/s10803-014-2351-z
- Xin, J., & Leonard, D. (2015). Using iPads to teach communication skills of students with Autism. Journal of Autism & Developmental Disorders, 45(12), 4154–4164. https://doi.org/10.1007/s10803-014-2266-8