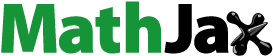
Abstract
There is a stellar upsurge in the number of persons pursuing a master’s level of education as well as the institutions offering it in the current generation. Nevertheless, an explicit theoretical and empirical implication of how the tutelages, at this level, shape the well-being of learners—such that it could help individuals overcome career decision-making difficulties remains to be elucidated. The present study addressed two major objectives. Firstly, we investigated the well-being of master’s degree students along with career decision-making difficulties in India. Secondly, apart from exploring the possible influence of nationality of the respondents on career decision difficulty, the study expanded the literature on career decision-making difficulties to under-researched populations in developing countries. Through a cross-sectional research design, we recruited a sample of 136 master’s degree respondents. The result reveals that while the composite well-being resources significantly influenced Career Decision Difficulties, the nationality of respondents appeared not as a germane factor in this context. Following the evaluation of the direct effect of individual well-being resources; Self-acceptance and Personal growth proved to have a statistically significant effect on career decision-making difficulties. Also, among the constituents of career decision-making difficulties studied, lack of readiness appears to be the major concern among the respondents. The findings expand the literature on cognitive, vocational, and organization science vis-a-vis career decision-making difficulties and provide useful insights for educational institutions and practitioners.
PUBLIC INTEREST STATEMENT
Master’s degree education continues to attract varied interests among different stakeholders. Notwithstanding its crucial significance, several factors seem to contribute to the aberrantly diminished quality of learning outcomes among learners at this level. Research on career decision-making difficulties focusing on factors within the individual in this context appears limited. Based on an extensive appraisal of the literature on career decision-making difficulties and well-being, we propose that improved well-being of master’s degree students attenuates their career decision-making difficulties. Specifically, the current study reveals that improved self-acceptance and personal growth significantly reduce career decision-making difficulties among master’s degree respondents. Additionally, the lack of readiness appears to be the major source of career decision-making difficulties among the master’s students surveyed. The theoretical and practical implications of the study are provided for learners, stakeholders, and practitioners.
1. Introduction
Throughout human history, there has never been a period where crusading for higher education is so pronounced than in the present generation (Barrineau & Education., A. for C. H, Citation2003), even among individuals and societies with less veneration for higher education. Indeed, advanced learning has been reported to have multifaceted relevance in many countries (Garrett, Citation2003) including but not limited to shaping lives, enhanced cognitive processes, and most importantly overall improved career prospects. Although career readiness and its outcomes are the focal measures for programs and student success (Castellano et al., Citation2017; Soulé & Warrick, Citation2015), career decision-making difficulties appear ubiquitous and unemployment continues skyrocketing among master’s degree students (Khambayat, Citation2015; Kumuda, Citation2014). Career decision-making difficulties are defined as the inability to specify an educational or occupational choice (Kelly & Pulver, Citation2003). Osipow (Citation1999) defines career decision-making difficulties as the inability to specify an educational or occupational choice, accompanied by a delay in bringing a conclusion to the decision-making process. Historically, career decision-making difficulties remains a topical issue of discussion in career counseling and career development (Hacker et al., Citation2013; Lipshits-Braziler et al., Citation2016; Osipow, Citation1999), underscoring the consistent theoretical proposition that career decision-making problem is an important but challenging developmental task across life-span (Super, Citation1994). Osipow (Citation1999) summarized the literature concerning decision-making difficulties models more than a decade ago, yet there has been little significant theoretical and empirical advancement of research on factors impacting career decision-making difficulties (Brown et al., Citation2012; Hacker et al., Citation2013; Xu & Tracey, Citation2014, Citation2017) especially among master’s degree respondents. Just as life does not seem to follow a linear track, career pathways also seem not to be following a linear trajectory.
Emerging trends indicate several countries including Australia, Brazil, Chile, China, Egypt, France, Germany, Ghana, Great Britain, India, Japan, Russia, USA are vigorously expanding their higher education (Altbach, Citation2009; Altbach et al., Citation2017). However, studies conducted to assess outcomes of postsecondary education appear to focus on factors outside the individual such as the influence of parents, culture, socio-political dynamics, and immigration on career decision-making difficulties (Juntunen & Cline, Citation2010; Roysircar et al., Citation2010) and undergraduate sample. Studies conducted on graduate samples seem to focus on factors other than well-being as predictors.
Therefore, present-day counselors, educationists, and policymakers need an updated comprehension of decision-making difficulties structure and factors affecting it across different categories of learners, especially, at the master’s level with a focus on the learner. Given the complexities associated with career choice, it is evident that the career decision-making difficulties problem requires a multifaceted research approach and intervention strategies, including online interventions (Pordelan & Hosseinian, Citation2020), and no universally effective approach appears applicable for different forms of career decision-making difficulties (Gati et al., Citation1996; Osipow, Citation1999).
2. Theoretical and empirical background
Earlier research by Osipow (Citation1999) highlights two major theoretical approaches to study decision-making difficulties: Firstly, a theory-driven approach that relies on the predetermined factor structure of decision-making theory; and secondly, a data-driven approach that emphasizes an empirical investigation of data as the main source for decision-making difficulties. These theoretical and empirical foundations have hardly been expanded to include the possibility of examining the problem of the decision-making difficulties process as a cognitive process for self-directed decisions and positive outcomes.
Global uncertainties and competitions are impacting job opportunities, transforming the world economy, and creating an unprecedented war for talents (Batt & Colvin, Citation2011), a caveat for graduates (master’s students) and educational institutions. For master’s degree education to serve the purpose it is expected in society, we might want to see more protean workforce and entrepreneurs than graduates with varying dimensions of career decision-making difficulties. Previous studies seem to focus heavily on factors affecting vocational decision-making among adolescents, teenagers, young adults (Crişan & Turda, Citation2015; Feldman, Citation2003; Stǎricǎ, Citation2012) and undergraduates (Talib & Tan, Citation2009) in advanced countries. Some researchers argue that career decision-making difficulties among graduates are influenced by the social class they belong to (Ostrove et al., Citation2011). There is a paucity of research on the elements that affect career decision-making difficulties among master’s students which is attributable to their well-being. Conceivably, master’s degree students are projected to have reached the pinnacle of education and would, therefore, be equipped with skills and competencies indispensable for informed career decisions by default. However, with an increasing rise of unemployment, misplaced decisions, priorities, and identities; especially among master’s students (Khambayat, Citation2015; Kumuda, Citation2014), it raises a serious concern about the sort of training and capacity building given to students at this level. Recent studies report that university students suffer vastly from career decision-making difficulties (Di Fabio et al., Citation2015), and meaningless career life (Miller & Rottinghaus, Citation2014).
Research in India and the USA reveal the significant influence of family (Fouad et al., Citation2016), others found a significant impact of parental or friends on graduates’ career decisions (Emmanuelle, Citation2009; Mudhovozi & Chireshe, Citation2012). Career decision-making difficulties are a major factor affecting graduates of today, and such persons seem deficient in self-concept; lack appropriate career information, skills, competencies, as well as pragmatic knowledge about the world of work (Gati et al., Citation1996). Furthermore, personality interests (Burns et al., Citation2013), socio-political conditions, job prospects, school-related factors, and career counseling have also been reported to play crucial roles in career exploration and decision-making process (Ukil, Citation2016). The career decision-making problem has been described as a classical topic of theoretical debate in vocational psychology research (Osipow, Citation1999; Osipow & Gati, Citation1998; Santos & Ferreira, Citation2012). It is important to note that operational career decisions are required not only during graduate studies or graduation stage but also whenever a revision of career plans is needed at a variety of important life transitions as each of these alterations exemplifies a potential scenario for career decision-making difficulties to occur. On the one hand, career decision-making difficulties can be viewed as a normal developmental experience some individuals go through for a brief period at certain stages of their life and career. On the other hand, it may become chronic career decision-making difficulties, which can seriously destabilize an individual’s career decision-making process (Guay et al., Citation2006). According to the rational choice theory (RCT) model, agents in an effective decision-making process seek to maximize their expected utility based on weighted alternatives, anticipated outcomes, and preferences (Sen, Citation2011). Bringing all this down to career decision-making difficulties context, we argue that it is not the process, but the innate determinants of the individual which cause the dysfunctional thinking and approach to problem-solving accompanied by a maladaptive level of anxiety, as was stated previously (Gordon, Citation1998; Peterson et al., Citation1991).
The growing uncertainties associated with jobs, markets, and a diversified global economy make the topic of career choice and master’s students’ career well-being central to parents, educators, and organizations. Unfortunately, there seems to be a paucity of studies on career decision-making difficulties among master’s students. To the best of our knowledge, no study has examined the impact of master’s students’ well-being on career decision-making difficulties in India. It is based on this background that this paper seeks to explore the conundrums of career decision-making difficulties among master’s students along the dimensions of Personal Growth; Purpose in Life; Autonomy and Self-Acceptance.
2.1. Psychological well-being
2.1.1. Career well-being
The classical explanation of well-being could be traced back to the two ancient Greece (Connerley & Wu, Citation2016) generic philosophy of well-being: Firstly, the hedonic which emphasize on maximizing pleasure as a source of happiness and purposeful life (Ryff, Citation1989). It depicts a person’s subjective well-being as embodying the emotional and cognitive appraisal of one’s life. Secondly, the eudaimonic perspective hinges on growth and personal fulfillment which is strongly associated with psychological well-being (Ryff, Citation1989; Ryff & Keyes, Citation1995). Unlike other constructs such as career success which have gained much attention in the literature, studies on how well-being affects career decision-making difficulties appear limited.
Career well-being is operationalized as the experiences associated with a positive effect on an individual’s career in both the present and future terms (Kidd, Citation2008). It epitomizes the ongoing subjective feeling about an individual’s career. Researchers such as Creed and Blume (Citation2013) view career well-being as a person’s subjective satisfaction about a particular career choice. Indeed, effective well-being intervention has been reported to result in the prudent cultivation of human flourishing (Dahl et al., Citation2020). Investigators like Chen and Haller (Citation2015) linked career well-being to the well-being modeled by Ryff and colleagues involving self-acceptance, autonomy, personal growth, purpose in life, environmental mastery, and positive relationship with others (Ryff, Citation1989; Ryff & Keyes, Citation1995). Therefore, psychological well-being, well-being, and career well-being are used interchangeably in this study to mean the same thing. Multiple studies underscore the role of well-being in shaping different aspects of a person’s life (Reker et al., Citation1987; Sen, Citation2011). However, its exact influence on career decision-making difficulties remains unclear. Therefore, we posit that;
Hypothesis 1 (H1): Career well-being positively predicts career decisions.
2.1.2. Personal growth
tudies indicate that individuals who can effectively evaluate, identify themselves as growing, and aspiring to maximize their potential exhibit improved patterns of choices (Robitschek et al., Citation2012; Strauser et al., Citation2008). Personal growth entails mental, physical, and spiritual personal growth. The main focus, concerning personal growth herein has to do with mental personal growth relating to Cognitive Information Processing (Peterson et al., Citation2002). Personal growth entails the possibility that an individual can evaluate, and realizes his/her potential based on one of the main goals of life, attainment of self-actualization (Ivtzan et al., Citation2013). Ultimately, this can go a long way to boost a person’s capability to confidently plan an appropriate career as well as meaningful career search (Silvia et al., Citation2010). Individuals with positive deposition on personal growth should be more proactive on choices, intentionally capitalizing on personal development opportunities, and exhibit a clear sense of career choice (Robitschek & Cook, Citation1999; Ryff, Citation2013). Knowledge of the explicit impact of personal growth on career decision-making difficulties is less known. Grounded on these backgrounds, we postulate that master’s degree respondents with impaired personal growth patterns will exhibit significant career decision-making problems irrespective of their advanced education:
Hypothesis 2 (H2): Personal growth positively predicts career decisions.
2.1.3. Purpose in life
Classical studies describe lack of purpose in life as a malaise, which if left unchecked could metamorphose into other psychological conditions such as anxiety, depression, helplessness, hopelessness, and worthlessness (Frankl, Citation1978; Klinger, Citation1977; Ruffin, Citation1984). Some investigators found purpose in life to have a noteworthy association with the significance of living, meaningful choices, directed behavior, as well as overall well-being (Diener et al., Citation1998). Purpose in life is operationalized as the purposive intention backed with action to accomplish a defined objective. Reflecting on the theory of “will to meaning” (see Frankl, Citation1978) for instance, Reker and colleagues constructed a multidimensional basis for measuring meaning and purpose in life (Reker et al., Citation1987). Their studies show the attribute to be consistent with career well-being: meaning, goal-seeking, and future meaning. Perceived purpose and meaning should be expected to facilitate coping with multiple developmental crises, namely developmental decision-making difficulties. Further, theorists on life development and meaning for example, found a significant role of stage-wise life progression to purposiveness (Buhler, Citation1959; E. Erikson, Citation1968; E. H. Erikson, Citation1964) including contextual values to predict developmental accomplishments (E. H. Erikson, Citation1964). Notwithstanding, the obvious impact of purposeful life on career decision-making difficulties is yet to be explored thoroughly. Therefore, we posit that master’s degree learners (holders) with low sense of purpose exhibit a significant dysfunctional career decision-making outlook.
Hypothesis 3 (H3): Purpose in life positively predicts career decisions.
2.1.4. Self-acceptance
Humanists such as Maslow and Rogers centered on the innate human capacity to evaluate, recognize meaningful experience, as well as the distinctive capacity for self-directed behavioral change and personal transformation (Saul, Citation2014; Thalberg & Maslow, Citation1964). Self-acceptance, one of the key focuses of the humanists, is operationalized as a long-term self-evaluation, including a positive attitude toward the self, harmonizing and incorporating the presence of both good and bad outcomes (Ryff, Citation2013). Studies among South African graduates and youths show that participants with a healthy sense of self-acceptance, competency for adaptation to new and challenging environments exhibit a better employability profile (Chetty, Citation2012; Coetzee, Citation2012; Ismail, Citation2017). Moreover, acceptance of oneself has been found to represent an important construct in positive psychology and it is known to not only positively improve well-being, but also the global attitude towards self (Furnham, Citation2019; Seligman & Csikszentmihalyi, Citation2000).
Self-acceptance is seen to predict a person’s ability in performing dynamic activities such as career planning and improve job searching (Bardagi & Hutz, Citation2010; Silvia et al., Citation2010). Recently, results of studies among 45 Dutch respondents highlight the significant association between self-acceptance and the development of career clarity (Kunnen, Citation2014). Most of the studies on self-acceptance and other outcomes were centered around young individuals, adolescents, and undergraduates and on constructs other than career decision-making difficulties: less is known about the extent to which self-acceptance of the individual influences career decision-making difficulties. Therefore, we posit that master’s degree learners with a low sense of self-acceptance will exhibit significant difficulty concerning career choice:
Hypothesis 4 (H4): Self-acceptance positively predicts career decisions.
2.1.5. Autonomy and nationality (Multicultural perspective)
Moreover, research in positive psychology highlights the significance of an individual’s autonomy to overall well-being. A higher level of autonomy has been associated with a major sense of self-efficacy. Autonomy has been operationalized as a person’s ability to evaluate oneself according to personal values and standards and not looking for the approval of others (Ryff, Citation1989; Ryff & Keyes, Citation1995). This variable has proven to be positively related to young adults’ confidence in their ability to plan search for work (Guay et al., Citation2006). For instance, research by Guay et al. (Citation2006) revealed that young individuals with lower levels of autonomy were at risk of experiencing chronic life challenges. However, to the best of our knowledge, there is a dearth of research that has empirically examined the impact of autonomy on career decision-making difficulties among master’s degree respondents. In this paper, we argue that master’s degree respondents with higher scores on autonomy should be self-determined, capable of taking initiative, and independent. Such individuals can also be designated as possessing the skills and resources to resist social and political pressure as well as being able to think and act in some efficacious way. On the other hand, those with a poor profile on autonomy could be deficient in regulating their behaviors from within and have challenges with evaluating “self” from personal standards.
Although research on the influence of nationality or other multicultural variables on career decision-making difficulties remains limited, emerging lines of investigations highlight the contrasting influence of nationality of respondents on career choices. For instance, studies conducted in Switzerland reveal socio-economics factors and nationality of respondents to likely predispose a person to a state of procrastination related to career choices (Masdonati, Citation2010). Research by Xu and Bhang (Citation2019) found a meaningful cultural variance in measuring decision-making difficulties. Xu et al. (Citation2016) examined variations in career decision ambiguity tolerance based on culture and development stages among Chinese and U.S. college students and between Chinese high school and college students. The study revealed that while the tolerance factor was not upheld in both Chinese samples, there was structural invariance concerning preference and aversion factors. The study further highlighted that U.S. College students had a higher preference and aversion when compared to Chinese high school students. However, Chinese College students had a higher level of aversion in comparison to Chinese High school students (Xu et al., Citation2016). Researchers such as (Lopez, Citation1989) argue that socio-economic and or nationality-related factors, per se, do not directly influence a person’s career choice, but instead could indirectly inhibit individuals’ career exploration and suitable support systems. Given these conflicting findings, this study seeks to verify the association of nationality with career well-being and its impact on career decision difficulties of master’s degree respondents. Therefore, we hypothesize that:
Hypothesis 5 (H5): Autonomy positively predicts career decisions.
Hypothesis 6(H6): Nationality positively relates to well-being and predicts career decision difficulties.
The summary of the framework of the research is shown in :
. depicts our proposed two-stage model for the hypothesized relationships and predictions. It epitomizes how respondents’ well-being resources (Purpose in life, personal growth, self-acceptance, and autonomy) embodying the consequences of career well-being, affect career decision-making difficulty in the direct model, as well as indirect effect fashion. Career Decision-making Difficulties represent our proposed ultimate latent endogenous construct whose R2 could be accounted for by the variations due to the other variables in the model. The measurement model shows the proposed exogenous and endogenous manifest variables respectively. Essentially, the researchers hope that this measurement model will help in evaluating the extent to which the latent exogenous, Career Well-being and endogenous, Career Decision Difficulty constructs could be represented by the aforementioned manifest variables, through confirmatory factor analysis (CFA). The confirmation here is expected to cover the underlying structure of indicators considered to measure the constructs. These measurements are crucial in establishing the construct validity of our scales as well as in making sense for further structural equation analysis: aberrantly low measurement model indices nullify or invalidate structural model testing. The path between our proposed latent exogenous and latent endogenous constructs represents the structural model: regression. The Error terms (εs), point to the estimated variables while the disturbances (Ds) point to latent constructs and hypotheses (1–6) are represented by H1 to H6. Our hypothetical model, therefore, aims to estimate a set of underlying parameters corresponding to regression coefficients as well as variances and covariance matrices of the proposed independent variables (P M. Bentler, Citation2006). In this model, our exogenous and endogenous constructs could be viewed as reflective constructs. Thus, we assume measuring the factors by indicators over which they influence (Hair et al., Citation2014).
The proposed structural and mathematical models which guided this paper, as in (Kumar, Citation2015), are presented below: Structural model:
where ɳ denotes endogenous construct, ɱ represents a vector of the exogenous variable, the error term (disturbance) is the ɛ, the regression coefficients of the endogenous and exogenous constructs are the B and L. The estimated mathematical (measurement) model is:
where ^y, ^x represents regression coefficients of the manifest variables, and the residual error terms are represented by δ, ℮.
3. Method
3.1. Sample and data collection
The study was conducted at a large University in South India following the guidelines of the Institutional Ethics Committee (IEC) and in line with the 1964 Helsinki Declaration of research with human participants. To enhance representativeness, we selected affiliated institutions and colleges where both Indian and foreign master’s students study varying programs. An official letter specifying the intent of the study was first submitted to Heads of selected institutions for permission to recruit participants for the study. A set of inventories was distributed among 207 English-speaking master’s students, who consented to participate in the study. The participants included Indians, and foreign students (from Afghanistan, Congo, Sudan, Ghana, Ethiopia, Nigeria, Kenya, Togo, Uganda, Tanzania, Mali, Somalia, Eretria, Maldives, and Côte d’Ivoire), pursuing or just completed Master’s Degree. The participants were assured of privacy and confidentiality of data at the time of getting their consent for participation in the study. The researchers were available for any clarification to any items in the tests during the administration. The respondents were made aware of their right to freely discontinue at any point without any consequences. One hundred and seventy-four respondents took all the tests, but only 136 of the items were found fully completed and suitable for further analysis. The final sample of 136 could be considered appropriate for the minimal sample size and power requirement required for structural equation modeling (SEM) as reported previously (Anderson & Gerbing, Citation1988; Bentler & Yuan, Citation1999; Ding et al., Citation1995; Tabachnick & Fidell, Citation2012; Tinsley & Tinsley, Citation1987). The sample size of 136 also fulfills the criteria to achieve a power of .80 at .05 alpha level as well as the alternate root-mean-square error of approximation (RMSEA) = .08 (MacCallum et al., Citation1996).
As shown in , Female respondents constituted 61.8% of the survey, and 62.5% of the total respondents were of Indian nationality. Regarding their age (M = 24; SD = 4), 74.3% were aged between 19 and 25 years. Other characteristics of the respondents are shown in .
Table 1. Biodata of respondents
Despite acknowledging the suitability of cross-sectional design and questionnaire administration for this study, we felt that these might also give rise to a concern for self-reporting bias, Common Method Bias (CMB) was therefore examined. When CMB occurs, it might result in significant measurement approach differential coefficients (Jakobsen & Jensen, Citation2015; Podsakoff et al., Citation2012). This was controlled for and checked procedurally as well as statistically. Through a procedural approach, we ensured that the measures for the predictors and criterion variables were administered to master’s students from different universities. To control for social desirability, a statement about “there are no right or wrong answers” was written and communicated clearly, items measuring specific constructs were separated psychologically and temporally for each questionnaire as was demonstrated in similar previous studies (Podsakoff et al., Citation2012). Furthermore, after data collection, we conducted a post hoc statistical procedure, Harman’s Single Factor test, to check for concern for CMB (Chang et al., Citation2010; Podsakoff et al., Citation2012). This test is among the most widely used and acceptable measures of CMB in research (Podsakoff et al., Citation2003). The observed Principal Component Analysis (PCA) output for the first non-rotated factor captured only 14.78 % of the variance in the data (far less than 50% covariance), which shows minimal concern for CMB (Podsakoff et al., Citation2003).
3.2. Measures
3.2.1. Well-being components
For measuring our proposed exogenous construct, we adopted the sub-scales of psychological well-being (Ryff & Keyes, Citation1995) which have been found to reliably measure career well-being and overall well-being. The main components used to measure the profile of the proposed career well-being (CWB) herein include autonomy, self-acceptance, purpose in life, and personal growth. Excerpt of items measuring these included: I am not afraid to voice my opinions; even when they are in opposition to the opinions of most people; I am not interested in activities that will expand my horizons, I live life one day at a time and don’t think about the future; When I look at the story of my life; I am pleased with how things have turned out (Ryff & Keyes, Citation1995). Respondents express their degree of agreement with the statements on a six-point Likert scale item ranging from 1 = Strongly Disagree to 6 = Strongly Agree. Items that were negatively expressed were reverse coded before analysis.
This study confirms the following reliability coefficients: personal growth α = 0.87, purpose in life α = 0.87, self-acceptance α = 0.85, Autonomy α = 0.87. The reliability statistics reported previously on these constructs (Ryff & Keyes, Citation1995) are presented in .
Table 2. Descriptive and cronbach alpha reliability statistics
3.2.2. Career decision difficulty
We adopted the Gati and colleagues’ Career Decision Difficulty Questionnaire (CDDQ) (Gati et al., Citation1996) for assessing the career decision difficulty of the respondents. The CDDQ emanated from the taxonomy of decision-making difficulties as a result of an adaptation of a decision-making theory in the context of career decision-making (Gati et al., Citation1996). The questionnaire has been used widely in career decision-making difficulty studies such as in Morgan and Ness (Citation2003). The major sources of career decision-making difficulties measured herein are Lack of Readiness, Lack of Information, and Inconsistent information about the career. Extract of items measuring the CDD included: I usually feel that I need confirmation and support for my decisions from a professional or somebody I trust; It is usually difficult for me to make decisions; I find it difficult to make a career decision because I am not sure about my career preferences yet (Gati et al., Citation1996). Respondents express their degrees of agreement on a nine-point Likert scale ranging from; 1 = Does not describe me, to 9 = Describes me well. All questions measuring the CDD were stated in a positive format, hence required no reverse coding. depicts the psychometric properties of the instruments in the previous and the current study.
Table 3. Descriptive and cronbach alpha reliability statistics
4. Results
The Preliminary descriptive and correlation matrix among the variables are shown in .
Table 4. Descriptive statistics and correlation matrix
depicts the correlation matrix between the variables under investigation. The correlations among the variables were examined to ascertain whether there is a concern for Multicollinearity, a condition where independent variables are strongly correlated with each other (Tabachnick & Fidell, Citation2012). The coefficients were found to be within the acceptable range. These were further supported by the suitable Tolerance values and Variance inflation factors (VIF), as shown in .
Table 5. Coefficientsa
4.1. Analysis
The R Statistical Software (Version 4.0.0) with “lavaan” and other packages including “haven”, “semPlot”, “semTools”, “psych” was used for our analysis. When examining Harman’s Single factor test for Common Method Bias (CMB), we used the IBM SPSS Statistical software (Version 20) as was reported recently (Tehseen et al., Citation2017). Master’s students’ career decision difficulty reflects complex real-life phenomena involving multiple variables, and since structural equation modeling (SEM) takes into account multiple variables, equations, and relationships at the same time with error terms, we considered it to be appropriate for testing our hypothesized relationships (Meade, Citation2006). The fitting function of the “CFA” in SEM with Lavaan requires the choice of ideal estimator, which takes into account not only the sample size (Schermelleh-Engel et al., Citation2003; Vandenberg & Lance, Citation2000) but also the type of data collected, as well as multivariate normality. To account for such features, we ran the Mardia Test (Mardia, Citation1970). The resultant output shows multivariate kurtosis coefficient (kurtosis = b2d) = 79.724, standardized multivariate kurtosis coefficient (z-score) = −0.127 and p-value (p) = 0.899 as exemplified in (Yuan et al., Citation2005). Based on these observations, we opted to use the Robust estimator (MLR) as it seems more appropriate for data obtained using a Likert scale, and with fairly small sample size.
4.1.1. Output description and evaluation
We employed a confirmatory factor analysis (CFA) to specify our measurement model. The resultant output with its associated adjustments, along with other factor loadings, was found to be within an acceptable range. shows the overall goodness-of-fit (GOF) and the local fit indexes relevant for this study. The lavaan 0.6–6 ended normally after 64 iterations, resulting in an appropriate solution.
Table 6. Model goodness-of-fit and local fit indexes
4.1.2. Overall goodness-of-fit
As shown in , a summary of the overall goodness-of-fit indices is provided for both the ML and Robust ML (MLR), using the Yuan-Bentler correction (Bentler & Yuan, Citation1999). The Absolute fit indices for the χ2 Minimum Function Test Statistics of the variant estimators only differ slightly: χ2ML (15) = 26.983 while for χ2Robust(MLR) (15) = 26.494(26.983/1.018), with the scaling correction factor for the Yuan-Bentler correction (YBχ2) = 1.018, indicating a moderate level of correction. A value significantly greater than 1.00 will mean a huge deviation from normality. The p-values of the two variant χ2(15) are not statistically significant (p > .05) for both the ML (.093) and MLR (0.273), suggesting an excellent harmony between our data and the model. Also, an SRMR value of .054, which is less than .08, further concurs with the harmony of the model fit (Hu & Bentler, Citation1999). However, it must be emphasized that chi-square (χ2) values should be interpreted with caution due to their extreme sensitivity to sample size. Additionally, an inspection of the incremental fit indices in , the χ2 for both of the variant estimators: χ2ML = 424.699; and χ2Robust(MLR) = 396.202, additionally support the fitting of the model with the data, as well as the robust CFI and TLI, where the corrected incremental indices are at least 0.95, further favors parsimony (Marsh et al., Citation2004; Sharma et al., Citation2005).
With regards to the other parsimonious fit indices, we looked at the RMSEA (robust-RMSEA) = .076, along with a 90% Cl [0.021–0.122]. The RMSEA (.076) value, which is expected to be less than .07, as reported elsewhere in (Steiger, Citation2007) coupled with the upper limit of the confidence interval exceeding 0.1 may raise some suspicion that the observed model fitting may be due to some lack of parsimony instead of the underlying theoretical substratum that it may represent. In a nutshell, it can be concluded that the overall goodness-of-fit (GOF) indices, as indicated in , offer the minimally expected fit of the model.
4.1.3. Local fit indices of the output
For the local fit indices, we examined the parameter estimates and the R2. Firstly, after scrutinizing the “parameter estimates”: the factor loadings of the “estimate” reveals the absence of the Heywood case or inadmissible parameter as well as the abnormal standard error. Once the acceptability of the model cannot be doubted up to this stage, we can be confident to proceed further in reading and analyzing the detailed results. The output () of the two path coefficients (Regression 1); Career Well-being (β = 0.580, p < .001) is statistically significant while Nationality of respondents (β = −0.102, p > .05) is not.
Table 7. Estimates, significances, and R-squared
The predictive effect of Career Well-being on Career Decision-making difficulties is positively significant, demonstrating that the higher the career well-being resources (well-being) of persons, the less likely they will hold a dysfunctional career decision profile. Thus, a unit improvement in career well-being will lead to approximately 58% effective career choice, upholding Hypothesis1(H1). Quite revealing of the output is the observation that nationality plays no significant role in predicting Career Decision Difficulties, resulting in failing to support Hypothesis (H6). To account for the direct predictive effects of the career well-being resources on CCD (see ), the results (Regression 2) reveals Self-acceptance (β = 0.395, p < .001) and Personal growth (β = 0.239, p < .05) as having a significant positive predictive effect on Career Decision Difficulties. Thus, every one-unit advancement in Self-acceptance and personal growth will result in approximately 40% and 24% progress in effective and efficient career decisions, supporting hypothesis 3 (H3) and hypothesis 4 (H4) respectively. Meanwhile, Autonomy (β = −.098, p > .05) and purpose in life (β = .003, p > .05) show no significant effects in predicting CDD, leading to the failure to support H2 and H5.
The implication here is that individuals with a high sense of self-acceptance and personal growth, are less likely going to experience difficulties with career decision-making. With the case of R2, essential evidence is provided about the quality of an indicator as a symbol of the latent variable on which it hinges (validity coefficient). Our exogenous manifest variable, Self-acceptance 59%(0.588) as well as the endogenous manifest indicators, Lack of readiness 75% (0.751), and Lack of Information 70%(0.711) have the highest R2. Only Autonomy 29% (0.288) and Purpose in life 38%(0.384) appear to have gone contrary to earlier reported expected R2 value of at least 0.40 as recommended in (Gudgeon et al., Citation1994; Tabachnick & Fidell, Citation2012).
It should further be emphasized that an excellent solution to a CFA model must take into account not only the overall fit indices (structural validity) but also its reliability estimate. As such, apart from estimating reliability through the R2, we further measured other coefficients through the factorial loadings and composite reliability (‘omega”) as proposed by (Raykov, Citation2001). As can be seen in , the resultant composite reliability coefficients, Cronbach alpha (Cronbach, Citation1951), the Raykov’s omega (Raykov, Citation2001), the Bentler’s omega2 (Peter M. Bentler, Citation2009), as well as the McDonald’s omega3 (McDonald, Citation1999) are all close to each other and within an acceptable range, likewise, the Average Variance Extracted (AVE), “avervar” as recommended in (Fornell & Larcker, Citation1981). The unexplained v
Table 8. Reliability(alpha), Omega and Average Variance Extracted(AVE)
5. Discussion
The continuous exploration of the divergent nature of career decision-making difficulties elements, including its varying etiologies is clinically relevant at present time. Previous studies have consistently focused on management and prevention of decision-making difficulties among teenagers, undergraduates, and with the decision-maker passively considered: there seems to be a poverty of knowledge about the relationships between the aspects of the decision-maker and the career decision-making difficulties itself. This research highlights some of the under-reported factors affecting career decision-making difficulties, especially relating to master’s students’ well-being. The output of this study shows a positive correlation between career decision-making difficulties components and all the components of well-being examined.
Quite revealing from the result of this study is the impact of self-acceptance on career decision-making difficulties. As shown from the results, self-acceptance by respondents proved to be an effective influence on career decision-making difficulties. This corroborates with the findings of several studies (e.g., Di Fabio & Fabio, Citation2014; Di Fabio et al., Citation2015; Kunnen, Citation2014; Seligman, Citation2002) about the relevance of self-acceptance in facilitating career progression. The positive relationship of autonomy with career decision-making difficulties components also suggests its directional relevance to career decision-making difficulties as reported elsewhere (Guay et al., Citation2006; Silvia et al., Citation2010). Overall, the components of well-being correlated positively with career decision difficulties components. When looking at their predictive capacities, self-acceptance and personal growth emerged as the strongest factors to significantly influence the career decision-making difficulties of respondents in this study. This could imply that master’s degree respondents with a high sense of personal growth and self-acceptance are more likely to be proactive in their life, intentionally seeking out or capitalizing on development opportunities. They are more likely to have a clear sense of what career paths they should pursue, similar to earlier reported studies (Robitschek et al., Citation2012; Robitschek & Cook, Citation1999; Ryff, Citation2013; Strauser et al., Citation2008).
Following the evaluation of the major constituents of career decision difficulty—namely lack of readiness, lack of Information, and Inconsistency of Information: lack of readiness was seen to have a very stronger connection with career decision difficulties among the respondents surveyed. This depicts the poor attention given to this domain even by higher degree-granting institutions, to master’s degree respondents as shown in this study. Perhaps, the low mean scores as reported herein (see ) on lack of information and inconsistent information might be characterized by a floor effect. This might imply that the participants surveyed might not be facing major career decision challenges on these two domains at the time of measurement. Additionally, Self-acceptance and lack of readiness appear to have a stronger connection than the other components of the constructs examined, which might further suggest their distinctive directional relationship. Meanwhile, the nationality of the respondents seems to play no significant role in predicting Career Decision Difficulties. Conceivably, this may be attributable to the mixed cultural background of the participants: about 37% of the survey respondents are foreigners. Perhaps, the outcome of this study might result in differing outcomes if participants are recruited from a single nationality.
5.1. Theoretical implications
Education up to at least a master’s degree level is generally expected to be a significant catalyst in shaping the overall behavioral and functional abilities of learners. However, with an increasing proportion of master’s degree graduates superficially exhibiting multifaceted challenges about career decision-making with its attendant problems, it raises a thoughtful question: what could be the state of their well-being?
In this study, through a multi-level equation modeling (SEM), we investigated the likely predictors of career decision difficulties among master’s degree learners (holders), putting the individual as the focus, and utilizing the dimension of well-being resources (Ryff & Keyes, Citation1995): autonomy, personal growth, self-acceptance and purpose in life, and with nationality as another prospective predictor. The results of the study point to some significant theoretical and practical contributions to the field of cognitive science, positive psychology, organizational and Behavioral science as well as vocational psychology as discussed below.
Firstly, the findings not only confirm the significant role of well-being in shaping career decision-making difficulties but also expanded the literature to cover under-researched populations in developing countries. From a theoretical perspective, out of the four main well-being components examined, purpose in life and autonomy appear not to be significant constituents for concern of well-being among the surveyed master’s degree respondents. Perhaps, future research in positive psychology and well-being might consider ways of re-examining components of well-being to reflect different stages of learners for enhanced life-long learning. Alternatively, we could also be tempted to argue that it is the master’s level of education that has improved the autonomy and sense of purpose among the respondents surveyed.
Notwithstanding, self-acceptance and personal growth corroborated with previous studies about the relevance of these well-being resources not only for other constructs but also essential for improved career choice. Overall, the combinations of the well-being resources (Indirect effect) concur with earlier studies on how these could positively impact the overall functional and behavioral abilities of individuals ().
Figure 2. Showing composite (Indirect) effects and relationships of the model

Secondly, among other things, the findings reveal that parameters used to gauge career decision-making difficulties, as established in multiple studies (Osipow, Citation1999; Osipow & Gati, Citation1998; Santos & Ferreira, Citation2012) need not be generalized across all areas of learners and populations. Certainty, this study upholds the crucial role of, especially, learners’ lack of readiness, as key components associated with career decision-making difficulties (see & .) not only among youth, adolescence, and undergraduates but also across different categories of learners including master’s students. The observed directional relationship between self-acceptance and lack of readiness might imply that when learners are groomed to be more career-ready, their sense of self-acceptance will likely improve and vice versa.
This study could be viewed as an important augmentation to literature for its emphasis on the investigation of aspects of the individual, well-being, as likely predictors of career decision-making difficulties among master’s degree students from different developing countries in India. Furthermore, most of the earlier researches in the field of vocational science, cognitive science, career counseling, and well-being appear to mainly focus on the top-down approach, where institutions and practitioners are expected to plan and design a career path for learners (Oldham & Richard Hackman, Citation2010) seemingly across all levels of learning. As evinced by the results of the current study, respondents appear to have a sense of purpose and are more autonomous to craft their career path. Master’s students should therefore be well-groomed, made career ready, and be provided with relevant as well as consistent career information to help them craft their career, resulting in proactive bottom-up career crafting (Hornung et al., Citation2010).
5.2. Practical implications
Apart from the aforementioned theoretical implications derived from the current study, we highlight some plausible practical implications. Firstly, lack of readiness, lack of information, and inconsistent information about career embodied career decision-making difficulties even among master’s degree respondents, especially, lack of readiness. This observation perhaps goes to affirm the seemingly poor attention given to these domains even among higher degree-granting institutions: universities, and colleges. Perhaps, the observed outcomes might be due to the age categories of the participants: almost 75% of the survey respondents are millennials age. Notwithstanding, going forward, universities and practitioners might want to give special attention to career readiness and career information of master’s students; suitable outlets and opportunities should be put into practice and or communicated efficiently and effectively. Master’s degree learners must also be encouraged to be active information seekers beyond the confines of institutions and colleges. As observed from the findings of this study, universities and institutions should place greater emphasis on helping individuals improve upon their level of Self-acceptance and personal growth for improved career and general life choices. It is crucial to recognize that, unlike other information sources, self-acceptance and personal growth are not something that is acquired or given randomly, rather they must be built within and developed over time in learners through a well-structured and pragmatic approach and training. As observed from this study, improving the career readiness of individuals could be an effective way to successfully increase their sense of self-acceptance. Considering the values of the R square, other variables that are beyond the scope of the current study could also possibly contribute to the career decision difficulties of the surveyed respondents.
5.3. Limitations and future research
Despite the apparent strengths of this study, it is equally essential we point to some probable limitations that should be noted while interpreting the result. Firstly, our sample size appears small, considering that SEM usually requires large samples. Notwithstanding, we were able to provide a reasonable basis for using this sample (Bentler & Yuan, Citation1999). Besides, we chose a suitable model estimator for the measurement of our CFA, Robust (MLR) estimator, which seems compatible with a fairly small sample size. Secondly, the tendency for social desirability and the potential for common method bias (CMB) was identified beforehand and reasonably controlled for, procedurally, and statistically. Additionally, recognizing the potential restrictions of chi-square for depicting our goodness-of-fit (GOF), we relied on multiple fit indices (Schermelleh-Engel et al., Citation2003) for elucidating the extent to which our model fit with the data. Therefore, while interpreting the results of this study, it should be noted that these and other potential limitations have been recognized and reasonably controlled for as was demonstrated previously (Gati et al., Citation1996; Jakobsen & Jensen, Citation2015; Podsakoff et al., Citation2012; Ryff, Citation2013). Future research might want to consider investigating the role of higher education in the context of career decision-making difficulties as well as the remodeling of educational institutions for productive career gains. Extended research on the influence of multicultural dynamics and how that shapes career choice is also needed. In this study, the extent to which the well-being components influence career decision-making difficulties among master’s students was examined. Perhaps, it is plausible for future research to model a study with career decision difficulties as a predictor of well-being.
6. Conclusions
To summarize, a master’s degree education has been reported to result in multi-faceted behavioral and cognitive changes, including productive goal-directed outcomes in learners. The number of individuals pursuing a master’s degree and the institutions offering it keeps increasing. Nonetheless, master’s degree students seem to exhibit profound challenges regarding career choices and there seems to be a poverty of research putting aspects of the individual learner in context. This study investigated the possible influence of well-being on career decision-making difficulties among master’s degree participants in India. The results reveal the well-being of master’s degree respondents, especially self-acceptance and personal growth significantly shape their subsequent career decisions. The findings of this study advanced the literature on well-being, organizational science, and also, it is useful for practitioners in educational institutions, policymakers, and graduates.
Credit authorship contribution statement
Zaidan Mohammed: Conceptualization, Investigation, Methodology, Software, Funding acquisition, Data Curation, Visualization, Writing-original draft preparation, Formal analysis.
Sampath Kumar: Supervision, Methodology, Validation, Writing-Reviewing and Editing, Formal analysis.
Prakash Padakannaya: Validation, Methodology, Software, Writing-Reviewing, and Editing, Formal analysis
Acknowledgements
We wish to express our profound gratitude to all the institutions that accepted our request to collect data from their students. We also appreciate the cooperation and support of all the students who voluntarily participated in the study. Additionally, we thank all the faculties at the Department of studies in Psychology, the University of Mysore for the support given throughout the study. Finally, a special thanks go to the Indian Council for Cultural Relations (ICCR) for the funding support.
Disclosure statement
No potential conflict of interest was reported by the author(s).
Additional information
Funding
Notes on contributors
Zaidan Mohammed
Zaidan Mohammed is a Ph.D. student at the Department of Psychological science, The University of Vermont, Burlington, United States. He holds a B.Sc. Psych. and M.Sc. Psych. (Clin). His research interest includes Behavioral Neuroscience, Neuropsychology, Neuroinflammation, Behavioral Engineering, Population health, Preventive Medicine, Decision-making, and Organizational Science.
Sampath Kumar
Sampath kumar is a Professor of Psychology at the Department of Psychology, University of Mysore. His research interests include Organizational Behavior, Counselling Psychology, Positive Psychology, Health Psychology, Social Psychology.
Prakash Padakannaya
Prakash Padakannaya is a Professor of Psychology, Christ University, Bangalore, India. He is a Fellow of the Association of Psychological Science (APS), USA, and a Fellow of the National Academy of Psychology (NAOP), India. His research interests include Cognition, Reading in different orthographies, Psycholinguistics, and Language & Brain.
References
- Altbach, P. G. (2009). One-third of the globe: The future of higher education in China and India. Prospects, 39(1), 11–31. https://doi.org/10.1007/s11125-009-9106-1
- Altbach, P. G., Reisberg, L., & De Wit, H. (Eds.). (2017). Responding to massification: Differentiation in postsecondary education worldwide. Springer.
- Anderson, J. C., & Gerbing, D. W. (1988). Structural equation modeling in practice: A review and recommended two-step approach. Psychological Bulletin, 103(3), 411–423. https://doi.org/10.1037/0033-2909.103.3.411
- Bardagi, M. P., & Hutz, C. S. (2010). Undergraduate students’ life satisfaction, career commitment and career exploration. Arquivos brasileiros de psicologia, 62(1), 159–170. https://psycnet.apa.org/record/2012-16109-014
- Barrineau, I. T., & Education., A. for C. H. (2003). Continuing education in the era of quantum change. 2003 ACHE proceedings. (65th Annual Meeting, Charlottesville, VA, November 8- 12,2003). Association for Continuing Higher Education.
- Batt, R., & Colvin, A. J. S. (2011). An employment systems approach to turnover: Human resources practices, quits, dismissals, and performance. Academy of Management Journal, 54(4), 695–717. https://doi.org/10.5465/AMJ.2011.64869448
- Bentler, P. M. (2009). Alpha, dimension-free, and model-based internal consistency reliability. Psychometrika, 74(1), 137–143. https://doi.org/10.1007/s11336-008-9100-1
- Bentler, P. M. (2006). EQS 6 structural equations program manual. BMDP Statistics Software.
- Bentler, P. M., & Yuan, K. H. (1999). Structural equation modeling with small samples: Test statistics. Multivariate Behavioral Research, 34(2), 181–23. https://doi.org/10.1207/S15327906Mb340203
- Brown, S. D., Hacker, J., Abrams, M., Carr, A., Rector, C., Lamp, K., Telander, K., & Siena, A. (2012). Validation of a four-factor model of career decision-making difficulties. Journal of Career Assessment, 20(1), 3–21. https://doi.org/10.1177/1069072711417154
- Buhler, C. (1959). Theoretical observations about life’s basic tendencies. American Journal of Psychotherapy, 13(3), 561–581. https://doi.org/10.1176/appi.psychotherapy.1959.13.3.561
- Burns, G. N., Morris, M. B., Rousseau, N., & Taylor, J. (2013). Personality, interests, and career decision-making difficulties: A multidimensional perspective. Journal of Applied Social Psychology, 43(10), 2090–2099. https://doi.org/10.1111/jasp.12162
- Castellano, M., Ewart Sundell, K., & Richardson, G. B. (2017). Achievement outcomes among high school graduates in college and career readiness programs of study. Peabody Journal of Education, 92(2), 254–274. https://doi.org/10.1080/0161956X.2017.1302220
- Chang, S. J., Van Witteloostuijn, A., & Eden, L. (2010). From the Editors: Common method variance in international business research. Journal of International Business Studies, 41(2), 178–184. https://doi.org/10.1057/jibs.2009.88
- Chen, C. P., & Haller, S. (2015). The role of career counselling in supporting career well-being of nurses. Australian Journal of Career Development, 24(1), 15–26. https://doi.org/10.1177/1038416214555772
- Chetty, Y. (2012). Graduateness and employability in the higher education sector: A focused review of the literature. In Developing student graduateness and employability: Issues, provocations, theory and practical application (pp. 5–24). https://silo.tips/download/graduateness-and-employability-in-the-higher-education-sector-a-focused-review-o
- Coetzee, M. (2012). A framework for developing student graduateness and employability in the economic and management sciences at the University of South Africa. In Developing student graduateness and employability: Issues, provocations, provocations, theory and practical guidelines(pp. 119–152).
- Connerley, M. L., & Wu, J. (2016). Handbook on well-being of working women. London: Springer. https://doi.org/10.1007/978-94-017-9897-6
- Creed, P. A., & Blume, K. (2013). Compromise, well-being, and action behaviors in young adults in career transition. Journal of Career Assessment, 21(1), 3–19. https://doi.org/10.1177/1069072712453830
- Crişan, C., & Turda, S. (2015). The connection between the level of career decision-making difficulties and the perceived self-efficacy on the career decision-making among teenagers. Procedia - Social and Behavioral Sciences, 209, 154–160. https://doi.org/10.1016/j.sbspro.2015.11.271
- Cronbach, L. J. (1951). Coefficient alpha and the internal structure of tests. Psychometrika, 16(3), 297–334. https://doi.org/10.1007/BF02310555
- Dahl, C. J., Wilson-Mendenhall, C. D., & Davidson, R. J. (2020). The plasticity of well-being: A training-based framework for the cultivation of human flourishing. Proceedings of the National Academy of Sciences, 117(51), 32197–32206. https://doi.org/10.1073/pnas.2014859117
- Di Fabio, A., & Fabio, A. D. (2014). Career counseling and positive psychology in the 21st century: New constructs and measures for evaluating the effectiveness of intervention. Journal of Counsellogy,1, 193–213. https://www.scinapse.io/papers/2550877373
- Di Fabio, A., Palazzeschi, L., Levin, N., Gati, I., Levin, N., & Gati, I. (2015). The role of personality in the career decision-making difficulties of Italian young adults. Journal of Career Assessment, 23(2), 281–293. https://doi.org/10.1177/1069072714535031
- Diener, E., Sapyta, J. J., & Suh, E. (1998). Subjective well-being is essential to well-being. Psychological Inquiry, 9(1), 33–37. https://doi.org/10.1207/s15327965pli0901_3
- Ding, L., Velicer, W. F., & Harlow, L. L. (1995). Effects of estimation methods, number of indicators per factor, and improper solutions on structural equation modeling fit indices. Structural Equation Modeling: A Multidisciplinary Journal, 2(2), 119–143. https://doi.org/10.1080/10705519509540000
- Emmanuelle, V. (2009). Inter-relationships among attachment to mother and father, self-esteem, and career decision-making difficulties. Journal of Vocational Behavior, 75(2), 91–99. https://doi.org/10.1016/j.jvb.2009.04.007
- Erikson, E. (1968). Youth: Identity and crisis. WW. https://doi.org/10.1002/yd.29
- Erikson, E. H. (1964). Childhood and society (2nd ed.). New York: Norton.
- Feldman, D. C. (2003). The antecedents and consequences of early career decision-making difficulties among young adults. Human Resource Management Review, 13(3), 499–531. https://doi.org/10.1016/S1053-4822(03)00048-2
- Fornell, C., & Larcker, D. F. (1981). Evaluating structural equation models with unobservable variables and measurement error. Journal of Marketing Research, 18(3), 382–388. https://doi.org/10.2307/3151312
- Fouad, N. A., Kim, S. Y., Ghosh, A., Chang, W. H., & Figueiredo, C. (2016). Family influence on career decision making: Validation in India and the United States. Journal of Career Assessment, 24(1), 197–212. https://doi.org/10.1177/1069072714565782
- Frankl, V. E. (1978). The unheard cry for meaning: Psychotherapy and humanism. The unheard cry for meaning: Psychotherapy and humanism. Simon and Schuster.
- Furnham, A. (2019). Personality and individual differences. In The psychology of behaviour at work (pp. 159–230). Psychology Press. https://doi.org/10.4324/9780203506974-4
- Garrett, R. (2003). The observatory on borderless higher education. New Review of Information Networking, 9(1), 113–122. https://doi.org/10.1080/1361457042000186967
- Gati, I., Krausz, M., & Osipow, S. H. (1996). A taxonomy of difficulties in career decision making. Journal of Counseling Psychology, 43(4), 510–526. https://doi.org/10.1037/0022-0167.43.4.510
- Gordon, V. N. (1998). Career decidedness types: A literature review. The Career Development Quarterly, 46(4), 386–403. https://doi.org/10.1002/j.2161-0045.1998.tb00715.x
- Guay, F., Ratelle, C. F., Senécal, C., Larose, S., & Deschnes, A. (2006). Distinguishing developmental from chronic career decision-making difficulties: Self-efficacy, autonomy, and social support. Journal of Career Assessment, 14(2), 235–251. https://doi.org/10.1177/1069072705283975
- Gudgeon, A. C., Comrey, A. L., & Lee, H. B. (1994). A first course in factor analysis. The Statistician, 43(2), 332. https://doi.org/10.2307/2348352
- Hacker, J., Carr, A., Abrams, M., & Brown, S. D. (2013). Development of the career decision-making difficulties profile: Factor structure, reliability, and validity. Journal of Career Assessment, 21(1), 32–41. https://doi.org/10.1177/1069072712453832
- Hair, J. F., Jr., Hult, G. T. M., Ringle, C. M., & Rstedt, M. S. (2014). A prımer on partıal least squares structural equation modelling. Practical assessment, research and evaluation. https://doi.org/10.1108/ebr-10-2013-0128
- Hornung, S., Rousseau, D. M., Glaser, J., Angerer, P., & Weigl, M. (2010). Beyond top-down and bottom-up work redesign: Customizing job content through idiosyncratic deals. Journal of Organizational Behavior, 31(2–3), 187–215. https://doi.org/10.1002/job.625
- Hu, L. T., & Bentler, P. M. (1999). Cutoff criteria for fit indexes in covariance structure analysis: Conventional criteria versus new alternatives. Structural Equation Modeling: A Multidisciplinary Journal, 6(1), 1–55. https://doi.org/10.1080/10705519909540118
- Ismail, S. (2017). Graduate employability capacities, self-esteem and career adaptability among South African young adults. SA Journal of Industrial Psychology, 43(1), 1–10. https://doi.org/10.4102/sajip.v43i0.1396
- Ivtzan, I., Gardner, H. E., Bernard, I., Sekhon, M., & Hart, R. (2013). Well-being through self-fulfilment: Examining developmental aspects of self-actualization. The Humanistic Psychologist, 41(2), 119–132. https://doi.org/10.1080/08873267.2012.712076
- Jakobsen, M., & Jensen, R. (2015). Common method bias in public management studies. International Public Management Journal, 18(1), 3–30. https://doi.org/10.1080/10967494.2014.997906
- Juntunen, C. L., & Cline, K. (2010). Culture and self in career development: Working with American Indians. Journal of Career Development, 37(1), 391–410. https://doi.org/10.1177/0894845309345846
- Kelly, K. R., & Pulver, C. A. (2003). Refining measurement of career decision-making difficulties types: A validity study. Journal of Counseling & Development, 81(4), 445–453. https://doi.org/10.1002/j.1556-6678.2003.tb00271.x
- Khambayat, R. P. (2015). Addressing skilling & youth employability: An Indian perspective. In Inted2015: 9th international technology, education and development conference.
- Kidd, J. M. (2008). Exploring the components of career well-being and the emotions associated with significant career experiences. Journal of Career Development, 35(2), 166–186. https://doi.org/10.1177/0894845308325647
- Klinger, E. (1977). Meaning & void: Inner experience and the incentives in people’s lives. Oxford England U Minnesota Press.
- Kumar, S. (2015). Structure equation modeling basic assumptions and concepts: A novices guide. Asian Journal of Management Sciences,3(07), 25–28. https://www.eajournals.org/journals/international-journal-of-quantitative-and-qualitative-research-methods-ijqqrm/
- Kumuda, D. D. (2014). Educated youth and un-employment: An Indian perspective. IOSR Journal of Humanities and Social Science, 19(8), 09–11. https://doi.org/10.9790/0837-19870911
- Kunnen, E. S. (2014). The effect of a career choice guidance on self-reported psychological problems. Frontiers in Psychology, 5, 547. ISSN1664-1078. https://doi.org/10.3389/fpsyg.2014.00547
- Lipshits-Braziler, Y., Gati, I., & Tatar, M. (2016). Strategies for coping with career decision-making difficulties. Journal of Career Assessment, 24(1), 42–66. https://doi.org/10.1177/1069072714566795
- Lopez, F. G. (1989). Current family dynamics, trait anxiety, and academic adjustment: Test of a family-based model of vocational identity. Journal of Vocational Behavior, 35(1), 76–87. https://doi.org/10.1016/0001-8791(89)90049-3
- MacCallum, R. C., Browne, M. W., & Sugawara, H. M. (1996). Power analysis and determination of sample size for covariance structure modeling. Psychological Methods, 1(2), 130–149. https://doi.org/10.1037/1082-989X.1.2.130
- Mardia, K. V. (1970). Measures of multivariate skewness and kurtosis with applications. Biometrika, 57(3), 519–530. https://doi.org/10.1093/biomet/57.3.519
- Marsh, H. W., Hau, K. T., & Wen, Z. (2004). In search of golden rules: Comment on hypothesis-testing approaches to setting cutoff values for fit indexes and dangers in overgeneralizing Hu and Bentler’s (1999) findings. Structural Equation Modeling: A Multidisciplinary Journal, 11(3), 320–341. https://doi.org/10.1207/s15328007sem1103_2
- Masdonati, J. (2010). The transition from school to vocational education and training: A theoretical model and transition support program. Journal of Employment Counseling, 47(1), 20–29. https://doi.org/10.1002/j.2161-1920.2010.tb00087.x
- McDonald, R. P. (1999). Test theory: A unified approach. Lawrence Earlbaum.
- Meade, A. W. (2006). A beginner’s guide to structural equation modeling (2nd ed.). Organizational Research Methods.
- Miller, A. D., & Rottinghaus, P. J. (2014). Career decision-making difficulties, meaning in life, and anxiety: An existential framework. Journal of Career Assessment, 22(2), 233–247. https://doi.org/10.1177/1069072713493763
- Morgan, T., & Ness, D. (2003). Career decision-making difficulties of first-year students. Canadian Journal of Career Development, 2(1), 33–39. https://cjcd-rcdc.ceric.ca/index.php/cjcd/article/view/311
- Mudhovozi, P., & Chireshe, R. (2012). Socio-demographic factors influencing career decision-making among undergraduate psychology students in South Africa. Journal of Social Sciences, 31(2), 167–176. https://doi.org/10.1080/09718923.2012.11893025
- Oldham, G. R., & Richard Hackman, J. (2010). Not what it was and not what it will be: The future of job design research. Journal of Organizational Behavior, 31(2–3), 463–479. https://doi.org/10.1002/job.678
- Osipow, S. H. (1999). Assessing career decision-making difficulties. Journal of Vocational Behavior, 55(1), 147–154. https://doi.org/10.1006/jvbe.1999.1704
- Osipow, S. H., & Gati, I. (1998). Construct and concurrent validity of the career decision-making difficulties questionnaire. Journal of Career Assessment, 6(3), 347–364. https://doi.org/10.1177/106907279800600305
- Ostrove, J. M., Stewart, A. J., & Curtin, N. L. (2011). Social class and belonging: Implications for graduate students’ career aspirations. Journal of Higher Education,82(6), 748–774. https://doi.org/10.1353/jhe.2011.0039
- Peterson, G. W., Sampson, J. P., Lenz, J. G., & Reardon, R. C. (2002). A cognitive information processing approach to career problem solving and decision making. In Career choice and development,48(1), 3–18.
- Peterson, G. W., Sampson, J. J., & Reardon, R. C. (1991). Career development and services: A cognitive approach. Thomson Brooks/Cole Publishing Co.
- Podsakoff, P. M., MacKenzie, S. B., Lee, J. Y., & Podsakoff, N. P. (2003). Common method biases in behavioral research: A critical review of the literature and recommended remedies. Journal of Applied Psychology, 88(5), 879–903. https://doi.org/10.1037/0021-9010.88.5.879
- Podsakoff, P. M., MacKenzie, S. B., & Podsakoff, N. P. (2012). Sources of method bias in social science research and recommendations on how to control it. Annual Review of Psychology, 63(1), 539–569. https://doi.org/10.1146/annurev-psych-120710-100452
- Pordelan, N., & Hosseinian, S. (2020). Design and development of the online career counselling: A tool for better career decision-making. Behaviour and Information Technology, 26(3), 3445–3457. https://doi.org/10.1080/0144929X.2020.1795262
- Raykov, T. (2001). Estimation of congeneric scale reliability using covariance structure analysis with nonlinear constraints. British Journal of Mathematical and Statistical Psychology, 54(2), 315–323. https://doi.org/10.1348/000711001159582
- Reker, G. T., Peacock, E. J., & Wong, P. T. P. (1987). Meaning and purpose in life and well-being: A life-span perspective. Journals of Gerontology, 42(1), 44–49. https://doi.org/10.1093/geronj/42.1.44
- Robitschek, C., Ashton, M. W., Spering, C. C., Geiger, N., Byers, D., Schotts, G. C., & Thoen, M. A. (2012). Development and psychometric evaluation of the personal growth initiative scale-II. Journal of Counseling Psychology, 59(2), 274–287. https://doi.org/10.1037/a0027310
- Robitschek, C., & Cook, S. W. (1999). The influence of personal growth initiative and coping styles on career exploration and vocational identity. Journal of Vocational Behavior, 54(1), 127–141. https://doi.org/10.1006/jvbe.1998.1650
- Roysircar, G., Carey, J., & Koroma, S. (2010). Asian Indian college students’ science and math preferences: Influences of cultural contexts. Journal of Career Development, 36(4), 324–347. https://doi.org/10.1177/0894845309345671
- Ruffin, J. E. (1984). The anxiety of meaninglessness. Journal of Counseling & Development, 63(1), 40–42. https://doi.org/10.1002/j.1556-6676.1984.tb02678.x
- Ryff, C. D. (1989). Happiness is everything, or is it? Explorations on the meaning of psychological well-being. Journal of Personality and Social Psychology, 57(6), 1069–1081. https://doi.org/10.1037/0022-3514.57.6.1069
- Ryff, C. D. (2013). Psychological well-being revisited: Advances in the science and practice of eudaimonia. Psychotherapy and Psychosomatics,83(1), 10–28. https://doi.org/10.1159/000353263
- Ryff, C. D., & Keyes, C. L. M. (1995). The structure of psychological well-being revisited. Journal of Personality and Social Psychology, 69(4), 719–727. https://doi.org/10.1037/0022-3514.69.4.719
- Santos, P. J., & Ferreira, J. A. (2012). Career decision statuses among Portuguese secondary school students: A cluster analytical approach. Journal of Career Assessment, 20(2), 166–181. https://doi.org/10.1177/1069072711420853
- Saul, M. (2014). Carl Rogers | Simply psychology. https://www.simplypsychology.org/carl-rogers.html
- Schermelleh-Engel, K., Moosbrugger, H., & Müller, H. (2003). Evaluating the fit of structural equation models: Tests of significance and descriptive goodness-of-fit measures. Methods of Psychological Research Online, 8, 23–74.
- Seligman, M. E., & Csikszentmihalyi, M. (2000). Positive psychology. An introduction. The American Psychologist, 55(1), 5–14. https://doi.org/10.1037/0003-066X.55.1.5
- Seligman, M. E. P. (2002). Positive psychology, positive prevention, and positive therapy. In Handbook of positive psychology. https://doi.org/10.1017/CBO9781107415324.004
- Sen, A. (2011). Rational behaviour. In The New Palgrave Dictionary of economics. London: Palgrave Macmillan. https://doi.org/10.1057/9780230226203.3389
- Sharma, S., Mukherjee, S., Kumar, A., & Dillon, W. R. (2005). A simulation study to investigate the use of cutoff values for assessing model fit in covariance structure models. Journal of Business Research, 58(7), 935–943. https://doi.org/10.1016/j.jbusres.2003.10.007
- Silvia, P., Maria Luisa, F., Francesco, A., & Michele, V. (2010). Work self-efficacy scale and search for work self-efficacy scale: A validation study in Spanish and Italian cultural contexts. Revista de Psicología Del Trabajo y de Las Organizaciones, 26(3), 201–210. https://doi.org/10.5093/tr2010v26n3a4
- Soulé, H., & Warrick, T. (2015). Defining 21st century readiness for all students: What we know and how to get there. Psychology of Aesthetics, Creativity, and the Arts, 9(2), 178–186. https://doi.org/10.1037/aca0000017
- Stǎricǎ, E. C. (2012). Predictors for career decision-making difficulties in adolescence. Procedia - Social and Behavioral Sciences, 33, 168–172. https://doi.org/10.1016/j.sbspro.2012.01.105
- Steiger, J. H. (2007). Understanding the limitations of global fit assessment in structural equation modeling. Personality and Individual Differences, 42(5), 893–898. https://doi.org/10.1016/j.paid.2006.09.017
- Strauser, D. R., Lustig, D. C., & Çiftçi, A. (2008). Psychological well-being: Its relation to work personality, vocational identity, and career thoughts. Journal of Psychology: Interdisciplinary and Applied, 142(1), 21–35. https://doi.org/10.3200/JRLP.142.1.21-36
- Super, D. E. (1994). A life span, life space perspective on convergence. In Convergence in career development theories: Implications for science and practice (pp. 63–74). CPP Books. https://psycnet.apa.org/record/1994-97757-005
- Tabachnick, B. G., & Fidell, L. S. (2012). Using multivariate statistics (6th ed.). Harper and Row. https://doi.org/10.1037/022267
- Talib, M. A., & Tan, K. A. (2009). Predictors of career decision-making difficulties among Malaysian undergraduate students. European Journal of Social Sciences,8(2), 215–224. http://psasir.upm.edu.my/7024/
- Tehseen, S., Ramayah, T., & Sajilan, S. (2017). Testing and controlling for common method variance: A review of available methods. Journal of Management Sciences, 4(2), 142–168. https://doi.org/10.20547/jms.2014.1704202
- Thalberg, I., & Maslow, A. H. (1964). Toward a psychology of being. Philosophy and Phenomenological Research, 25(2), 288. https://doi.org/10.2307/2105414
- Tinsley, H. E. A., & Tinsley, D. J. (1987). Uses of factor analysis in counseling psychology research. Journal of Counseling Psychology, 34(4), 414–424. https://doi.org/10.1037/0022-0167.34.4.414
- Ukil, M. I. (2016). Career barriers to career decision-making difficulties: A final-year Bba students view. Polish Journal of Management Studies, 13(1), 192–205. https://doi.org/10.17512/pjms.2016.13.1.18
- Vandenberg, R. J., & Lance, C. E. (2000). A review and synthesis of the measurement invariance literature: Suggestions, practices, and recommendations for organizational research. Organizational Research Methods, 3(1), 4–70. https://doi.org/10.1177/109442810031002
- Xu, H., & Bhang, C. H. (2019). The structure and measurement of career decision-making difficulties: A critical review. The Career Development Quarterly, 67(1), 2–20. https://doi.org/10.1002/cdq.12159
- Xu, H., Hou, Z. J., Tracey, T. J. G., & Zhang, X. (2016). Variations of career decision ambiguity tolerance between China and the United States and between high school and college. Journal of Vocational Behavior, 93, 120–128. https://doi.org/10.1016/j.jvb.2016.01.007
- Xu, H., & Tracey, T. J. G. (2014). The role of ambiguity tolerance in career decision making. Journal of Vocational Behavior, 85(1), 18–26. https://doi.org/10.1016/j.jvb.2014.04.001
- Xu, H., & Tracey, T. J. G. (2017). Career decision ambiguity tolerance and its relations with adherence to the RIASEC structure and calling. Journal of Career Assessment, 25(4), 715–730. https://doi.org/10.1177/1069072716665874
- Yuan, K. H., Bentler, P. M., & Zhang, W. (2005). The effect of skewness and kurtosis on mean and covariance structure analysis: The univariate case and its multivariate implication. Sociological Methods & Research, 34(2), 240–258. https://doi.org/10.1177/0049124105280200