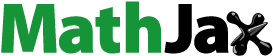
Abstract
This study aimed to validate an Indonesian version of the Mobile Phone Problem Use Scale (MPPUS) in a sample of high school students from South Tangerang City, Banten Province,, Indonesia. Throughout this process, we evaluated the scale’s psychometric properties and examined the differential item functioning (DIF) across schools with and without a smartphone ban policy. The study used a cross-sectional survey design with a sample of 622 high school students (Mage = 16.10; SDage = 1.41). There were 312 respondents from schools with a smartphone ban and 310 from schools with no smartphone ban. We used confirmatory factor analysis (CFA), multiple indicators multiple causes (MIMIC) model, and Rasch Rating Scale Model (RSM) to analyze the data. The CFA and MIMIC model results indicated a good fit for the overall model using the pooled sample. Based on MIMIC model, one item was flagged as DIF. Furthermore, RSM analysis demonstrated that the Indonesian MPPUS has adequate psychometric properties. RSM-based DIF analysis identified four DIF items. Therefore, 23 of 27 items on the scale have the same meaning across schools with two different policies. Our findings provide empirical evidence that MPPUS performed well across students from schools with and without smartphone restrictions, despite the fact that the MPPUS was originally designed for the general population. The findings of this study can be used to develop an evidence-based policy for implementing smartphone policies in Indonesian schools.
1. Background of the study
It goes without doubt that the growth of mobile phones or smartphones has recently been incredibly vast. According to a report made in 2020, the number has reached up to 3.5 billion (Statista, Citation2020a). As a huge country in Southeast Asia with 210 million users, Indonesia ranks number one as smartphone users (Statista, Citation2020b). Furthermore, approximately 21% of smartphone users are between the ages of 12 and 19 or of school age (Digital Influence Lab, Citation2020). However, the constant availability of smartphones combined with the addictiveness of popular applications can become problematic when people use them in unproductive or even harmful ways (Van Velthoven et al., Citation2018). Also, high school students were found to be very vulnerable to smartphone use because they spend a lot of time with smartphones (Cha & Seo, Citation2018; Fischer-Grote et al., Citation2021; Rachmayani et al., Citation2020).
When new technologies, such as smartphones, are introduced into schools, they pose a number of challenges. As a result, some governments and schools enact various smartphone ban policies and regulations (Gao et al., Citation2014). In Indonesia, for example, some schools have policies prohibiting students from using smartphones in school (Baginda et al., Citation2020; Machmud, Citation2018a, Citation2018b). Previous findings from Mollman (Citation2015) stated that the smartphone ban policy in Indonesia was implemented to allow students to focus on their learning while providing ample time for students to interact directly with their peers and teachers. Furthermore, the arguments for a potential ban on smartphones in schools in Indonesia came primarily from teachers, parents, and school administrators who were concerned about the risks that students faced as a result of problematic smartphone use (Machmud, Citation2018a).
Previous findings indicating that a large number of Indonesian high school students are classified as problematic users have emphasized the negative consequences of problematic smartphone use in Indonesian schools (Nufus et al., Citation2020; Rachmayani et al., Citation2020). Another study of Indonesian high school students discovered that roughly 60% of respondents were moderate to severely addicted to their smartphones (Taufik et al., Citation2020). According to the most recent research, problematic smartphone use has a negative impact and will have serious psychological consequences (see, Busch & McCarthy, Citation2021). Unfortunately, few studies have been conducted to investigate the smartphone ban policy and its direct link to problematic smartphone use. Current research on smartphone ban policies and problematic smartphone use is frequently conducted in isolation rather than as part of an integrated study.
To name a few, one study investigated students’ perceptions of the smartphone ban using descriptive methods to explain the phenomenon (Machmud, Citation2018a). Another is a mixed-method study that investigated school principals’ and teachers’ perceptions of the smartphone ban policy, which discovered that the policy’s implementation was not driven by empirical evidence and was inconsistent across schools and areas (Machmud, Citation2018b). The most recent study by Baginda et al. (Citation2020), suggested that the equality of such a policy across schools should be promoted. Furthermore, the majority, if not all, of the preceding studies were conducted after the smartphone ban was implemented. When this is the case, such studies cannot provide adequate evidence to support smartphone policy in schools.
In contrast to Indonesia, other countries (e.g., China) conducted research before enacting and widely implementing smartphone bans. A comprehensive survey was conducted to compare two school clusters, one of which had a smartphone ban policy and the other did not. The samples were drawn from a variety of school levels, including elementary and secondary schools (Gao et al., Citation2014). In addition, related research that underpinned the implementation of the smartphone ban policy was also carried out (for example, Zuo, Citation2021).
One reason for implementing the smartphone policy was to keep students from becoming addicted to harmful smartphone-based activities (Machmud, Citation2018a, Citation2018b). In support of this claim, a study conducted by the Australian state of Victoria revealed that the policy’s implementation effectively created a safe and conducive learning environment free of disruptions caused by inappropriate smartphone use (Baker, Citation2018; Victoria State Government, Citation2020). However, the study on the implementation of the smartphone ban policy should ideally be preceded by a measurement of the condition and severity of problematic smartphone use (Lepkowska, Citation2019). In other words, research into problematic smartphone use is critical for strengthening the conceptual framework for enacting the smartphone-ban policy (Wiederhold, Citation2019). Unfortunately, there have been no such studies conducted in Indonesia to date.
The smartphone-ban policy has recently received increased attention as a key construct in the field of psychology (Bianchi & Phillips, Citation2005; Busch & McCarthy, Citation2021; Lepkowska, Citation2019; Mach et al., Citation2020). A systematic review of problematic smartphone use can be conducted to decide on a smartphone ban policy (Lepkowska, Citation2019). In other words, a study like this is critical for solidifying the conceptual framework for enforcing a smartphone ban policy (Wiederhold, Citation2019).
2. Literature review
2.1. Problematic smartphone use: theoretical framework and measures
Problematic smartphone use is defined as a psychological and behavioral dependence on smartphones characterized by an inability to control one’s mobile phone use, which eventually leads to negative consequences in daily life (Billieux, Citation2012). Since the late 1990s, this emerging public health issue has been growing (Harris et al., Citation2020). Furthermore, problematic smartphone use addressed issues such as intolerance, escape from other problems, withdrawal, craving, and negative life consequences in various contexts (Bianchi & Phillips, Citation2005).
The construct of problematic smartphone use has several conceptual similarities with addiction, including overuse, failure of impulse control, dependence, use in risky and dangerous situations, and potential negative effects of not using smartphones (Harris et al., Citation2020). However, smartphone addiction falls within a broader category of behavioral addiction. As a relatively new conceptual addition to American psychiatry, behavioral addiction was first recognized by the Diagnostic and Statistical Manual of Mental Disorders in 2010 (DSM-V; Rosenberg & Feder, Citation2014). Like substance addiction, behavioral addiction is characterized by excessive salience, withdrawal symptoms, conflict, and relapse behaviors (Allred & Atkin, Citation2020).
Some researchers believe that technological addictions are a subset of behavioral addictions and operationally define technological addictions as non-chemical behavioral addictions involving human-machine interaction. However, regardless of whether excessive use of various forms of technology can or should be labeled an “addiction,” researchers have discovered some evidence for excessive use of technology that can be deemed problematic (Bianchi & Phillips, Citation2005; Griffiths, Citation1996; Citation1998).
Busch and McCarthy (Citation2021) conducted a systematic literature review from 2008 to 2019 on the antecedents and consequences of problematic smartphone use and proposed seven key research questions for the future agenda of research on this topic. One of the questions is “how do different demographics experience problematic smartphone use?”. In parallel, in the context of measurement instruments, a recent systematic review has identified 78 instruments and their underlying conceptual framework on excessive or problematic smartphone use (Harris et al., Citation2020). Nonetheless, almost none of the instruments measuring problematic smartphone use in Indonesia have undergone adequate reviews (Baginda et al., Citation2020; Machmud, Citation2018a, Citation2018b). The dangers of this behavior are most likely to occur in Indonesia. Unfortunately, such research reports are hard to find, especially with well-established instruments to measure problematic smartphone use. Therefore, the problematic use of smartphones is an important construct to be studied in Indonesia’s context of the smartphone ban policy. This will provide information on whether the use of smartphones by Indonesian students is problematic or not.
In recent years, various instruments for problematic mobile phone use have been developed, and related research was conducted across continents resulting, as noted, in 78 instruments (Harris et al., Citation2020; Vally & Hichami, Citation2019). The Mobile Phone Problem Use Scale (MPPUS; Bianchi & Phillips, Citation2005) is one of the prevailing instruments that are most widely used. Henceforth, research related to adaptation as well as modification of MPPUS has been done in many countries across the globe, such as in China (Hongjuan et al., Citation2017), Germany (Foerster et al., Citation2015), Iran (Kalhori et al., Citation2015), Lebanon (Nahas et al., Citation2018), Poland (Mach et al., Citation2020), Spain (de-Sola et al., Citation2017), and United Arab Emirates (Vally & Hichami, Citation2019). Unfortunately, none has been made in Indonesia, although this country is categorized as the third of the top three smartphone users in Asia and first in Southeast Asia. In addition, it is important to check whether MPPUS can function well when administered to a specific sample, such as students from schools with a smartphone-banned policy.
2.2. Differential item functioning (DIF) analysis
When validating an adapted instrument, it is critical to consider the absence of bias. Assume bias exists; the item performs differently in different groups with the same latent trait level. In other words, people from different groups will respond to the items differently due to factors other than the latent trait being measured, such as group identity (Baylor et al., Citation2014; Saggino et al., Citation2020).
In this context, item bias was referred to as differential item functioning (DIF). Items marked as DIF have the potential to jeopardize the validity of scores if they are not investigated further (Martinkova et al., Citation2017). Different smartphone policies at school, for example, can be a source of bias. According to the review of literature, no study on this topic has ever been conducted, particularly in Indonesia.
There are currently several methods for detecting DIF. DIF detection methods are classified into two philosophical views: statistical (e.g., factor analysis-based) and measurement models (e.g., Rasch model-based), with each model having different assumptions (Hayat et al., Citation2021). However, the Rasch model has uniqueness in terms of specific objectivity, additivity, and parameter separation (Wu et al., Citation2016), where these features assume an absence of DIF (Mesbah & Kreiner, Citation2012). Given the importance of DIF testing for validating measuring instruments, we believe that in the context of the current study, the DIF analysis procedure should be performed as part of testing the psychometric properties to determine whether the measurement instrument of problematic smartphone use can function well across different groups (school with vs. without smartphone banned policy), even though the instrument was developed for the general population. DIF analysis findings will provide empirical evidence of measurement invariance across different groups. This means that the Indonesian MPPUS is valid to be used regardless of different smartphone-banned policy implementations.
2.3. Rationale of the study
As previously stated, research on problematic smartphone use in the Indonesian context, which underpins the policy’s implementation, is limited. Furthermore, no studies have been conducted on the adaptation of the problematic smartphone use scale. This study reports on the use and adaptation of the problematic smartphone use instrument, the findings of which are expected to benefit future researchers on smartphone use in Indonesia significantly. MPPUS is preferred over all other commonly used scales for measuring smartphone use, as noted previously. The significance of this study stems from the following factors: (1) it is the first validation of MPPUS conducted in Indonesia, (2) it is the first contribution of the Rasch measurement model to validating MPPUS, and (3) it employs DIF analysis.
If the adapted MPPUS is proven to be free from DIF, of course, it can be used for further research to examine differences in the use of problematic smartphones in the sample with and without the smartphone ban policy. This can be done because the instrument has been shown to function well in populations with different group members.
3. Methods
3.1. General background
This study employed a cross-sectional survey research design, with data collected on a single occasion. This design adheres to the fundamental principles of explanatory research by employing a confirmatory approach to test whether differences in school policy affect the measurement of problematic smartphone use. We examined whether MPPUS functions consistently across different School policies regarding smartphone bans. Quota sampling, a non-probability sampling technique, was used to select participants for this study. The ethical approval was granted by the Institute for Research and Community Services (LPPM), Universitas Negeri Jakarta, Jakarta, Indonesia (No. 676/UN39.14/PJ.00/2020). Participants agreed to take part in the study and to have the results published in accordance with ethical approval. Permission was granted from the principal of the school to obtain ethical clearance from the relevant institution.
3.2. Participants
The respondents of this research comprised 622 students (356 male, 266 female) from 20 secondary schools in South Tangerang City, Banten Province, Indonesia. The respondents’ ages ranged from 14 to 18 years (mean age = 16.10; SD = 1.41). There were 312 respondents from schools that banned smartphones and 310 from schools that did not. We expected a balanced sample of 350 respondents from schools with smartphone restrictions and 350 from schools without. However, only 622 people volunteered to participate in this study, with an 82.93% response rate.
3.3. Instrument
In this study, the Mobile Phone Problem Use Scale (MPPUS; Bianchi & Phillips, Citation2005) was used as the main instrument consisting of 27 items abbreviated as MPPUS-27. The scale was, as noted, initially developed in response to a comprehensive picture of the levels of problematic smartphone use and to understand the factors that influence it (Bianchi & Phillips, Citation2005; Harris et al., Citation2020). The main instrument in this study was the Mobile Phone Problem Use Scale (MPPUS; Bianchi & Phillips, Citation2005), which consisted of 27 items abbreviated as MPPUS-27. A professional translator translated MPPUS-27 into Indonesian for this study. In addition, lecturers in psychology thoroughly reviewed the translated version of MPPUS-27 (henceforth MPPUS-27 INA) to ensure its readability and content accuracy. In contrast to the original version, which used a 10-point Likert scale, MPPUS-27 INA used a five-point Likert scale (“strongly disagree” to’strongly agree’). The five-point Likert scale was also used in an Iranian version of the MPPUS-27 (Kalhori et al., Citation2015). Cronbach’s alpha (α) of MPPUS-27 INA in this study is 0.88, indicating high internal consistency.
3.4. Data analyses procedure
3.4.1. Confirmatory factor analysis (CFA)
The data analyses of this study were conducted in a two-step process: (1) Confirmatory Factor Analysis (CFA) and (2) Rasch analysis. CFA was used to investigate whether items are unidimensional and appropriate for calibration using a unidimensional IRT model (Hayat et al., Citation2021). CFA was performed in Mplus 8.4 on the hypothesized 1-factor model. Following that, we conducted DIF testing using the multiple indicators multiple causes model (MIMIC; Cheng et al., Citation2016; Muthén, Citation1989).
The robust maximum likelihood estimator was used to estimate the parameters of the MIMIC models. Model fit was evaluated in several ways, including the chi-square () statistics, comparative fit index (CFI), Tucker-Lewis Index (TLI), and root mean square error of approximation (RMSEA). The following cut-offs were used to analyse goodness-of-fit of the model: a CFI > 0.90, TLI > 0.90, SRMR < 0.08, and values of RMSEA less than 0.05, respectively (Schumaker & Lomax, Citation2016). Because chi-square (
) statistics are susceptible to sample size; small samples might present significant results even when the fit is acceptable in another fit statistic (i.e., RMSEA and SRMR; Wang & Wang, Citation2019).
3.4.2. Rasch model
Rasch measurement model (Rasch, Citation1960) recently received more attention from researchers in various fields dealing with test designs, construct validation, and addressing item bias (Hayat et al., Citation2021). The basic concept of the Rasch models is that psychological constructs are not directly observable and can only be observed through the manifest responses of persons to a set of items that define the latent construct (DiStefano et al., Citation2019). Several models in the family of Rasch measurement can accommodate different types of response formats, such as polytomous, dichotomous, or mixed formats (Suryadi et al., Citation2020). Since the response data of MPPUS-27 INA was in the form of a Likert scale, the rating scale model (RSM; Andrich, Citation1978) procedure was appropriate for this study. RSM estimates the probability that a person endorses a specific category of the rating scale, given both person’s trait level () and the difficulty of the item (DiStefano et al., Citation2019).
RSM shares three assumptions: (a) unidimensionality, which refers to the assumption that a single trait underlies a collection of items of a measuring instrument; (b) local independence, which means that the response to one item will be independent of the response to another item; and (c) parallel ICCs, which states that the discrimination is uniform across items (Mair, Citation2018). The RSM was used to analyze the items of the MPPUS-27 INA using the WINSTEPS statistical program. Person and item parameters were estimated using an unconditional maximum likelihood (UCON) estimator.
To examine the psychometric properties of the scales, the following procedures were performed: (1) unidimensionality assessment using the principal component analysis of standardized residual; (2) an analysis of the local independence using the standardized residual correlation; (3) inspecting item properties, including item location, item-fit statistics using infit and outfit MNSQ statistics, item-to-total correlation, and DIF analysis, and (4) rating scale diagnostics of MPPUS.
4. Results
4.1. Factor analysis
Based on CFA, we found that 1-factor model fitted the data [χ2 (324) = 772.327, p < 0.001; RMSEA = 0.047 (90% CI = 0.043, 0.051), CFI = 0.929, TLI = 0.923, SRMR = 0.035] with five fit statistics met the predefined criteria. Although the p-value of the χ2 for the model was significant (p < 0.01), this likely happened due to the large sample size. We concluded that the goodness-of-fit for the unidimensional factor model of MMPUS was satisfactory. Loadings on the Indonesian MPPUS ranged from 0.506 to 0.727 at a p < 0.01 significance level. After the 1-factor CFA model fitted the data, we regressed one dichotomous covariate (score 1 for students from schools with restriction and 0 for school without restriction) on the latent factor using MIMIC model.
Results of the MIMIC model indicated that the model fit the data reasonably well [χ2 (324) = 796.923, p < 0.001; RMSEA = 0.045 (90% CI = 0.041, 0.050), CFI = 0.930, TLI = 0.924, SRMR = 0.035]. We found improvement in RMSEA, which is smaller than the 1-factor model. Loadings on MIMIC model ranged from 0.496 to 0.727 at a p < 0.01 significance level. Smartphone-banned policy as a covariate has a significant impact on the problematic smartphone use ( = −0.269, p < 0.01). Considering that the significant impact has a negative direction, students from schools with code 0 (school without restriction) have higher problematic smartphone use than those with code 1 (school with restriction). Furthermore, the study results showed a significant difference in the mean score of problematic smartphone use of students from schools with or without smartphone restrictions, where students without restrictions (code 0) had a higher mean.
Taking a closer look at the results of the DIF analysis of the items, we found one of the 27 items (item 24) flagged as DIF. Item 24 “Saya telah diperingatkan bahwa saya menghabiskan waktu terlalu banyak untuk bermain handphone. [I have been told that I spend too much time on my mobile phone.]” has a significantly associated with smartphone-banned policy in negative direction ( = −0.120, p < 0.01). This implies that participants from schools without smartphone restriction policy (coded as 0) get scores higher on Item 24 than those from schools with smartphone restriction (coded as 1), controlling for the factor of problematic smartphone use.
4.2. Rasch analysis
4.2.1. Unidimensionality
In this study, we used Principal Component Analysis on Standardised Residual (PCAR; Linacre, Citation1998) to test the unidimensionality of MPPUS-27 INA. Based on this analysis, we found evidence of unidimensionality since the minimum variance explained by the measure was 45.1% (22.1 in eigenvalues unit) above the minimum recommendation of 40% (Holster & Lake, Citation2016). In addition, we also found that the eigenvalue in the first contrast is 1.6 (in items unit), less than the predefined criteria of 2.0. The eigenvalue less than 2.0 indicates the presence of secondary factors with no more than two items. Based on this evidence, the MPPUS-27 INA showed to be unidimensional.
4.2.2. Local independence
The assumption of local independence states that any set of items does not significantly correlate except by latent trait measured by the items (Edwards et al., Citation2018). This study used the standardized residual correlation (Linacre, Citation1998) to examine possible locally dependent items. When using the residual correlation between item pairs, it is specified that the standardized residual correlation between item pairs is never > 0.30 (Saggino et al., Citation2020). With this criteria, we found one item pair exhibits a local dependence (Item 12 with Item 26) with a standardized residual correlation of 0.35. Considering that only one pair of items is greater than the criteria, the locally dependent item is not strong enough to violate unidimensionality. This is in line with the PCAR result that the secondary factor (multidimensionality) is insignificant (below two items).
4.2.3. Rasch item fit statistics, location, and DIF effect size
To determine how well each item fits the Rasch model, the “infit” and “outfit” mean-squares statistics (MNSQ) were calculated to investigate the extent to which each item follows the model expectation. An infit or outfit MNSQ values in the range of 0.6–1.4 are categorized as acceptable for the Likert scale format (Bond & Fox, Citation2015). In addition to Rasch fit statistics, an item-to-total correlation was used, which is similar to CTT’s item discrimination indices, with positive values indicating that the items behave as expected (Suryadi et al., Citation2020).
Using “infit” and “outfit” MNSQ statistics, all MPPUS-27 items were found to be in the acceptable range (0.6–1.4). Furthermore, as shown in , the item-to-total correlation was found to be in the range of 0.51 to 0.69, indicating that all of the items had positive correlations, as expected. Based on item measure (location), item 9: “Kesibukan saya pada percakapan di handphone menyebabkan saya tidak memperhatikan apa yang sedang saya kerjakan [Sometimes, when I am on the mobile phone and I am doing other things, I get carried away with the conversation and I don’t pay attention to what I am doing.]” was the hardest item to be endorsed (most “difficult”). In contrast, item 24 was the easiest item to be endorsed (most “easy”). The mean person measure was −1.33 logit (SD = 1.18), suggesting that the mean of students’ problematic smartphone use was lower than the mean of item difficulty (zero). Furthermore, the person score distribution of problematic smartphone use ranged from −4.07 to 4.63 logit, exceeding the item difficulty range (−0.84 to 0.82 logit). This indicates a wide range of problematic smartphone use levels among students, ranging from the least problematic to the most problematic.
Table 1. Item parameter and fit statistics of MPPUS-INA
DIF analysis was used to determine whether MPPUS is free of item bias. Despite the fact that different groups (e.g., the school with or without a smartphone ban) in the context of this study are at the same levels of the underlying trait, they may respond to an item differently, indicating the presence of an item bias between groups (Saggino et al., Citation2020). To detect the presence of item bias, the item-trait chi-square was used. A chi-square significance difference between groups (with and without smartphone restriction) and a DIF effect size greater than 0.50 indicate that there is item bias (Yan & Mok, Citation2012).
Using DIF analysis, we discovered four items with DIF: items 9, 15, 23, and 24. Despite being statistically significant, none of the DIF effect sizes exceeded 0.50. The largest DIF effect sizes are found in item 24 (DIF contrast = −0.27). This means that students with smartphone restrictions will find it more difficult to support item 24 (coded as 1). This finding was consistent with the MIMIC model results, which showed that students who were not restricted from using their smartphones scored higher.
4.2.4. Category endorsement
For each item, the number of endorsements, the observed averages, and the MNSQ statistics of each response for the items of MPPUS-27 INA were inspected (see, ). To interpret the information in , we use criteria where for each response category, the MNSQ Infit and Outfit values are between 0.5 to 2.0 were acceptable, and no response category which not selected by the respondent indicates well functioning response category (Linacre, Citation1999).
Table 2. Response categories functioning
The observed response frequencies had positively skewed distributions, as shown in Table , with only about 3% of total endorsements falling into the’strongly agree’ category. However, because none of the “infit” and “outfit” MNSQ statistics were greater than 2.0, all response categories fit the model, indicating that the MPPUS-27 INA response set functioned well based on the Rasch measurement model. In other words, the assumption of a uniform threshold range across items is maintained when using RSM.
5. Discussion
The primary goal of this study was to assess the psychometric properties of the MPPUS-27 INA, followed by DIF analysis across schools with and without smartphone restrictions to see if MPPUS worked well across different groups. CFA was also used as a separate analysis from the RSM application for construct validation. We concluded that the MPPUS-27 INA was unidimensional because the 1-factor CFA model fited the data well. This finding was consistent with the original factor structure of MPPUS (see, Bianchi & Phillips, Citation2005) and the Spanish version of MPPUS (de-Sola et al., Citation2017), both of which discovered a unidimensional factor structure of MPPUS, despite the fact that other studies discovered a multidimensional structure of MPPUS (e.g., Foerster et al., Citation2015). We conducted an RSM analysis because the unidimensionality requirement of RSM was met. We discovered significant evidence of a single latent trait measuring problematic smartphone use by using PCAR to test unidimensionality. Based on the Rasch model, we confirm the unidimensionality of the Indonesian version of MPPUS.
Based on local independence testing, we discovered one pair of items with a standardized residual correlation greater than 0.30 (e.g., Saggino et al., Citation2020), which is Item 12 “Saya telah berusaha mengurangi waktu untuk bermain handphone tetapi gagal” [I have attempted to spend less time on my mobile phone but am unable to] with Item 26 ““Teman-teman saya tidak suka kalau handphone saya mati” [My friends don’t like it when my mobile phone is switched off] (residual correlation = 0.35) According to this finding, the highest correlation (0.35) indicated that those two items shared only about 12% (0.35 0.35) of their variance. As a result, no critical evidence of a violation of the assumption of local independence was discovered (Yan & Mok, Citation2012).
The Rasch analysis of the MPPUS-27 has several advantages over previous psychometric validation methods based on classical test theory (CTT). The current analysis, in particular, provides item-level information that supplements information from the CTT (Hayat et al., Citation2021). In addition, we discovered that all of the items fitted the RSM and had a positive item-to-total correlation. As a result, MPPUS-27 INA has a Rasch measurement advantage, such as allowing a non-specialist researcher to use the total MPPUS score because the raw score is a sufficient statistic for the Rasch models (Saggino et al., Citation2020).
The highest item measure (0.82 logit) is item 9 MPPUS-INA. Because of its content, these items are difficult for high school students to endorse. This is consistent with previous research findings that smartphone use can impact various aspects of daily life, including educational and learning activities (eg, Misra & Stokols, Citation2012; Shin et al., Citation2011).
For MPPUS-27 INA, item 24 had the lowest item measure (−0.84 logit). Because teachers, parents, and friends frequently remind students about spending too much time on their smartphones, the item-measured behavior was simple to endorse based on the item content. According to previous research, 42.45 percent of Indonesian students used a smartphone 1–5 hours per day, while 33.06 percent used it 6 hours per day. According to the data, the most significant percentage of smartphone users spent 1–5 hours per day on their smartphones (Machmud, Citation2018a).
We discovered DIF in four items using Rasch-based DIF analysis: items 9, 15, 23, and 24. They were all statistically significant, and none had DIF effect sizes greater than 0.50. The largest DIF effect sizes were found in item 24 (DIF contrast = −0.27). This implies that Item 24 is more difficult to endorse by students who do not have smartphone restrictions (coded 0). This finding was linked to the MIMIC model based on factor analysis results, which indicated that students who did not have smartphone restrictions would score higher.
This study’s findings add to the theoretical and practical implications of the validated MPPUS-27 INA. First, a smartphone-ban policy can be implemented following a thorough investigation into whether smartphone use is problematic. Second, prior to the implementation of the smartphone-ban policy, a comparative analysis should be conducted. The comparison can be made based on student achievement, grade level, and gender (e.g., Zuo et al., Citation2021). This suggestion is consistent with the findings of recent studies (Baginda et al., Citation2020; Machmud, Citation2018a, Citation2018b). Finally, the findings of this study can help to strengthen the underlying framework for enacting and enforcing the smartphone ban policy.
As previously stated, research on problematic smartphone use has been conducted in many countries, with various limitations. This study also has several limitations. First, we make no claim that the samples are representative based on probability sampling. Second, no convergent or discriminant validity was demonstrated in the current study. Future research can overcome the limitations before using the 27-item version of the MPPUS. Third, it should be noted that the problematic use is not limited to smartphones but also includes other devices such as iPads and laptop computers. As a result, our findings cannot be applied to the problematic use of other devices. Fourth, although most previous studies using MPPUS included the measurement of user intensity of smartphone use and the purpose of use, this study did not. Another limitation of this study is that the sample was limited to senior high school students. As a consequence, it cannot be generalized beyond the sampled group. This issue is hoped to be considered in future research.
6. Conclusion
In conclusion, applying the RSM, factor analysis, and the MIMIC model to the MPPUS helped confirm the validity and utility of the MPPUS-27 INA. This method provided detailed information about the scale’s psychometric properties. Some DIF items account for a small proportion of total items. The findings of this study should help non-specialists (e.g., students, teachers, and psychologists) in future research to examine the relationship between problematic smartphone use and other psychological constructs. However, future research can look into the MPPUS’s test-retest reliability and convergent validity. Although the MPPUS-27 INA was the focus of this study, the same Rasch analysis procedures that produced sample-free measures could be used to evaluate other psychological measurement scales. Overall, this is Indonesia’s first study comparing samples of students from schools with and without a smartphone ban policy. Moreover, the findings of this study show that, despite being designed for the general population, MPPUS can function well on a specific sample of students from schools with a smartphone ban policy.
Disclosure statement
No potential conflict of interest was reported by the author(s).
Additional information
Funding
Notes on contributors
Muchlas Suseno
Muchlas Suseno, Senior lecturer of English for Master Program of English Language Education, State University of Jakarta, Jakarta, Indonesia [PhD, Educational Research and Evaluation, State University of Jakarta, 2010].
Bahrul Hayat
Bahrul Hayat, Lecturer at the Faculty of Psychology, Syarif Hidayatullah State Islamic University, Jakarta, Indonesia [PhD, Measurement, Evaluation and Statistical Analysis (MESA), University of Chicago, United States, 1992].
Muhammad Dwirifqi Kharisma Putra
Muhammad Dwirifqi Kharisma Putra, Doctoral student at the Faculty of Psychology, Gadjah Mada University, Yogyakarta, Indonesia [Master’s degree in Psychometrics, Syarif Hidayatullah State Islamic University Jakarta, Jakarta, 2016].
Jonna Karla Bien
Jonna Karla Bien, Director, Office of International Affairs, Polytechnic University of the Philippines, Manila, Philippines [Master’s degree in Clinical Psychology, Polytechnic University of the Philippines, Manila, 2013].
R. Rachmawati
R. Rachmawati, Lecturer at the Faculty of Medicine, Sriwijaya University, Palembang, Indonesia [Master’s degree in Psychology, Gadjah Mada University, Yogyakarta, 2011].
H. Hartanto
H. Hartanto, Lecturer at the Faculty of Psychology, Widya Dharma University, Klaten, Indonesia [Master’s degree in Psychology, Gadjah Mada University, Yogyakarta, 2012].
References
- Allred, R. J., & Atkin, D. (2020). Cell phone addiction, anxiety, and willingness to communicate in face-to-face encounters. Communication Reports, 33(3), 95–14. https://doi.org/10.1080/08934215.2020.1780456
- Andrich, D. (1978). A rating formulation for ordered response categories. Psychometrika, 43(4), 561–573. https://doi.org/10.1007/BF02293814
- Baginda, Sihotang, D. O., Batu, J. S. L., & Purba, S. (2020). Analysis of smartphone use policy: A case study in SMA Negeri Subulusalam. In B. Sinaga, R. Husein, & J. Rajagukguk (Eds.), Proceedings of the 5th annual international seminar on transformative education and educational leadership (AISTEEL 2020). Atlantis Press. https://doi.org/10.2991/assehr.k.201124.053
- Baker, J. (2018 May 28). ‘Schools need to react quickly’: Education expert urges smartphone ban. The Sydney Morning Herald. https://www.smh.com.au/national/nsw/schools-need-to-react-quickly-education-expert-urges-smartphone-ban-20180525-p4zhm4.html
- Baylor, C., McAuliffe, M. J., Hughes, L. E., Yorkston, K., Anderson, T., Kim, J., & Amtmann, D. (2014). A differential item functioning (DIF) analysis of the communicative participation item bank (CPIB): Comparing individuals with parkinson’s disease from the United States and New Zealand. Journal of Speech, Language, and Hearing Research, 57(1), 90–95. https://doi.org/10.1044/1092-4388(2013/12-0414)
- Bianchi, A., & Phillips, J. G. (2005). Psychological predictors of problem mobile phone use. Cyberpsychology & Behavior, 8(1), 39–52. https://doi.org/10.1089/cpb.2005.8.39
- Billieux, J. (2012). Problematic use of the mobile phone: A literature review and a pathways model. Current Psychiatry Reviews, 8(4), 299–307. https://doi.org/10.2174/157340012803520522
- Bond, T., & Fox, C. (2015). Applying the rasch model: Fundamental measurement in the human sciences (3rd) ed.). Lawrence Erblaum & Associates. https://doi.org/10.4324/9781315814698
- Busch, P. A., & McCarthy, S. (2021). Antecedents and consequences of problematic smartphone use: A systematic literature review of an emerging research area. Computers in Human Behavior, 114, 106414. https://doi.org/10.1016/j.chb.2020.106414
- Cauffman, E., & MacIntosh, R. (2006). A rasch differential item functioning analysis of the massachusetts youth screening instrument: Identifying race and gender differential item functioning among juvenile offenders. Educational and Psychological Measurement, 66(3), 502–521. https://doi.org/10.1177/0013164405282460
- Cha, -S.-S., & Seo, B.-K. (2018). Smartphone use and smartphone addiction in middle school students in Korea: Prevalence, social networking service, and game use. Health Psychology Open, 5(1), 205510291875504. https://doi.org/10.1177/2055102918755046
- Cheng, Y., Shao, C., & Lathrop, Q. N. (2016). The mediated MIMIC model for understanding the underlying mechanism of DIF. Educational and Psychological Measurement, 76(1), 43–63. https://doi.org/10.1177/0013164415576187
- de-Sola, J., Talledo, H., de Fonseca, F. R., Rubio, G., & Weinstein, A. M. (2017). Prevalence of problematic cell phone use in an adult population in Spain as assessed by the mobile phone problem use scale (MPPUS). PLoS ONE, 12(8), e0181184. https://doi.org/10.1371/journal.pone.0181184
- Digital Influence Lab. (2020). Indonesia digital marketing statistics 2018. Digitalinflueancelab (.dil). https://digitalinfluencelab.com/indonesia-digital-marketing-stats-2018/
- DiStefano, C., Greer, F. W., & Dowdy, E. (2019). Examining the BASC-3 BESS parent form–preschool using Rasch methodology. Assessment, 26(6), 1162–1175. https://doi.org/10.1177/1073191117723112
- Edwards, M. C., Houts, C. R., & Cai, L. (2018). A diagnostic procedure to detect departures from local independence in item response theory models. Psychological Methods, 23(1), 138–149. https://doi.org/10.1037/met0000121
- Fischer-Grote, L., Kothgassner, O. D., & Felnhofer, A. (2021). The impact of problematic smartphone use on children’s and adolescents’ quality of life: A systematic review. Acta Paediatrica, 110(5), 1417–1424. https://doi.org/10.1111/apa.15714
- Foerster, M., Roser, K., Schoeni, A., & Röösli, M. (2015). Problematic mobile phone use in adolescents: Derivation of a short scale MPPUS-10. International Journal of Public Health, 60(2), 277–286. https://doi.org/10.1007/s00038-015-0660-4
- Gao, Q., Yan, Z., Zhao, C., Pan, Y., & Mo, L. (2014). To ban or not to ban: Differences in mobile phone policies at elementary, middle, and high schools. Computers in Human Behavior, 38, 25–32. https://doi.org/10.1016/j.chb.2014.05.011
- Griffiths, M. D. (1996). Gambling on the internet: a brief note. Journal of Gambling Studies, 12(4), 471–473. https://doi.org/10.1007/BF01539190
- Griffiths, M. D. (1998). Internet addiction: Does it really exist?. In J. Gackenbach (Ed.), Psychology and the Internet: Intrapersonal, interpersonal and transpersonal applications (pp. 61–75). Academic Press.
- Harris, B., Regan, T., Schueler, J., & Fields, S. A. (2020). Problematic mobile phone and smartphone use scales: A systematic review. Frontiers in Psychology, 11, 672. https://doi.org/10.3389/fpsyg.2020.00672
- Hayat, B., Hidayat, R., & Putra, M. D. K. (2021). Exploring the factor structure of environmental attitudes measure in a sample of Indonesian college students. Revista CES Psicologia, 14(1), 112–129. http://dx.doi.org/10.21615/cesp.14.1.9
- Holster, T. A., & Lake, J. (2016). Guessing and the Rasch model. Language Assessment Quarterly, 13(2), 124–141. https://doi.org/10.1080/15434303.2016.1160096
- Hongjuan, Z., Rude, L., & Ying, L. (2017). The impact of peer attachment on problematic mobile phone use among adolescents: Moderated mediation effect of loneliness and self-construal. Journal of Psychological Science, 40(1), 89–95. http://www.psysci.org/EN/Y2017/V40/I1/89
- Kalhori, S. M., Mohammadi, M. R., Alavi, S. S., Jannatifard, F., Sepahbodi, G., Reisi, M. B., Sajedi, S., Farshchi, M., Khodakarami, R., & Kasvaee, V. H. (2015). Validation and psychometric properties of mobile phone problematic use scale (MPPUS) in university students of Tehran. Iranian Journal of Psychiatry, 10(1), 25–31. https://ijps.tums.ac.ir/index.php/ijps/article/view/48
- Lepkowska, D. (2019). To ban or not to ban? Mobile phone use in schools. British Journal of School Nursing, 14(10), 510–511. https://doi.org/10.12968/bjsn.2019.14.10.510
- Linacre, J. M. (1998). Detecting multidimensionality: Which residual data-type works best? Journal of Outcome Measurement, 2(3), 266–283. https://pubmed.ncbi.nlm.nih.gov/9711024/
- Linacre, J. M. (1999). Investigating rating scale category utility. Journal of Outcome Meaasurement, 3(2), 103–122. https://pubmed.ncbi.nlm.nih.gov/10204322/
- Mach, A., Demkow-Jania, M., Klimkiewicz, A., Jakubczyk, A., Abramowska, M., Kuciak, A., Serafin, P., Szczypinski, J., & Wojnar, M. (2020). Adaptation and validation of the Polish version of the 10-item mobile phone problematic use scale. Frontiers in Psychiatry, 11, 427. https://doi.org/10.3389/fpsyt.2020.00427
- Machmud, K. (2018a). The smartphone use in Indonesian schools: The high school students’ perspectives. Journal of Arts & Humanities, 7(3), 33–40. http://dx.doi.org/10.18533/journal.v7i3.1354
- Machmud, K. (2018b). Model kebijakan integrasi pemanfaatan mobile technology di sekolah menengah atas dan kejuruan. Deepublish Publisher.
- Mair, P. (2018). Modern psychometrics with R. Springer International Publishing AG. https://doi.org/10.1007/978-3-319-93177-7
- Martinkova, P., Drabinova, A., Liaw, Y.-L., Sanders, E. A., McFarland, J. L., Price, R. M., & Nehm, R. (2017). Checking equity: Why differential item functioning analysis should be a routine part of developing conceptual assessments. CBE Life Sciences Education, 16(2), rm2. https://doi.org/10.1187/cbe.16-10-0307
- Mesbah, M., & Kreiner, S. (2012). Rasch models for ordered polytomous items. In K. B. Christensen, S. Kreiner, & M. Mesbah (Eds.), Rasch models in health (pp. 27–42). John Wiley & Sons, Inc. https://doi.org/10.1002/9781118574454.ch2
- Misra, S., & Stokols, D. (2012). A typology of people–environment relationships in the digital age. Technology in Society, 34(4), 311–325. https://doi.org/10.1016/j.techsoc.2012.10.003
- Mollman, S. (2015). An Indonesian official wants to ban cell phone use for kids—to make them smarter and more social. Quartz. https://qz.com/415089/an-indonesian-official-wants-to-ban-cell-phone-use-for-kids-to-make-them-smarter-and-more-social/
- Muthén, B. O. (1989). Latent variable modeling in heterogeneous populations. Psychometrika, 54(4), 557–585. https://doi.org/10.1007/BF02296397
- Nahas, M., Hlais, S., Saberian, C., & Antoun, J. (2018). Problematic smartphone use among Lebanese adults aged 18-65 years using MPPUS-10. Computers in Human Behavior, 87, 348–353. https://doi.org/10.1016/j.chb.2018.06.009
- Nufus, N. P., Fitri, S., & Wirasti, M. K. (2020). Penggunaan smartphone bermasalah pada siswa SMA serta implikasinya bagi pelayanan bimbingan dan konseling di sekolah. Enlighten: Jurnal Bimbingan Konseling Islam, 3(2), 96–105. http://dx.doi.org/10.32505/enlighten.v3i2.1941
- Rachmayani, D., Kurniawati, Y., & Hikmiah, Z. (2020). Permasalahan penggunaan smartphone pada remaja dan hubungannya dengan gangguan kecemasan. Prosising Senantias, 1(1), 861–870. http://openjournal.unpam.ac.id/index.php/Senan/article/view/9067/5759
- Rasch, G. (1960). Probabilistic models for some intelligence and attainment tests. Danish Institute for Educational Research.
- Rosenberg, K. P., & Feder, L. C. (Eds.). (2014). An introduction to behavioral addictions. In Rosenberg, K. P. & Feder, L. C. (Eds.), Behavioral addictions: Criteria, evidence, and treatment (pp. 1–17). Elsevier Academic Press. https://doi.org/10.1016/B978-0-12-407724-9.00001-X
- Saggino, A., Molinengo, G., Rogier, G., Garofalo, G., Loera, B., Tommasi, M., & Velotti, P. (2020). Improving the psychometric properties of the dissociative experiences scale (DES-II): A Rasch validation study. BMC Psychiatry, 20(1), 8. https://doi.org/10.1186/s12888-019-2417-8
- Schumaker, R. E., & Lomax, R. G. (2016). A beginner’s guide to structural equation modeling (4th) ed.). Routledge.
- Shin, D.-H., Shin, Y.-J., Choo, H., & Beom, K. (2011). Smartphones as smart pedagogical tools: Implications for smartphones as u-learning devices. Computers in Human Behavior, 27(6), 2207–2214. https://doi.org/10.1016/j.chb.2011.06.017
- Statista. (2020a). Smartphone users worldwide 2016-2021. https://www.statista.com/statistics/330695/number-of-smartphone-users-worldwide/
- Statista. (2020b). Smartphone users in Indonesia 2015-2025. https://www.statista.com/statistics/266729/smartphone-users-in-indonesia/
- Suryadi, B., Hayat, B., & Putra, M. D. K. (2020). Evaluating psychometric properties of the Muslim daily religiosity assessment scale (MUDRAS) in Indonesian samples using the rasch model. Mental Health, Religion & Culture, 23(3–4), 331–346. https://doi.org/10.1080/13674676.2020.1795822
- Taufik, E., Dewi, S. Y., & Muktamiroh, H. (2020). Hubungan kecanduan smartphone dengan kecenderungan perilaku phubbing pada remaja di SMAN 34 Jakarta Selatan. Prosiding Seminar Nasional Riset Kedokteran (SENSORIK 2022), 1(1), 321–330. https://conference.upnvj.ac.id/index.php/sensorik/article/view/479/612
- Vally, Z., & Hichami, F. E. (2019). An examination of problematic mobile phone use in the United Arab Emirates: Prevalence, correlates, and predictors in a college-aged sample of young adults. Addictive Behaviors Reports, 9, 100185. https://doi.org/10.1016/j.abrep.2019.100185
- van Velthoven, M. H., Powell, J., & Powell, G. (2018). Problematic smartphone use: Digital approaches to an emerging public health problem. Digital Health, 4, 1–19. https://doi.org/10.1177/2055207618759167
- Victoria State Government. (2020 October 8). Mobile phone in schools. https://www.education.vic.gov.au/parents/going-to-school/Pages/Mobile-phones-in-schools.aspx
- Wang, J., & Wang, X. (2019). Structural equation modeling: Applications using mplus (2nd) ed.). John Wiley & Sons Ltd. https://doi.org/10.1002/9781119422730
- Wiederhold, B. K. (2019). Should smartphone use be banned for children? Cyberpsychology, Behavior and Social Networking, 22(4), 235–236. https://doi.org/10.1089/cyber.2019.29146.bkw
- Wu, M., Tam, H. P., & Jen, T.-H. (2016). Educational measurement for applied researchers: Theory into practice. Springer Nature Singapore Pte Ltd. https://doi.org/10.1007/978-981-10-3302-5
- Yan, Z., & Mok, M. M. C. (2012). Validating the coping scale for Chinese athletes using multidimensional Rasch analysis. Psychology of Sport and Exercise, 13(3), 271–279. https://doi.org/10.1016/j.psychsport.2011.11.013
- Zuo, M. (2021, February 3). Mobile phone ban ordered for schools in China to improve students’ focus on study and fight addiction to the internet and games. South China Morning Post. https://www.scmp.com/news/people-culture/trending-china/article/3120270/mobile-phone-ban-ordered-schools-china-improve