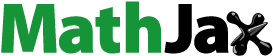
Abstract
Chinese agriculture grapples with contemporary challenges involving resource scarcity, environmental concerns, and transformations in agricultural labor structures. This work endeavors to address these challenges and foster sustainable agricultural development by advocating the principles of agricultural green development and sustainability in China. The application principles of digital technology are first expounded alongside a discussion on the foundational concepts of fuzzy systems. Subsequently, an in-depth analysis is undertaken on the composition and trajectory of China’s agricultural total factor productivity (ATFP), culminating in the construction of a Green ATFP model. The findings illuminate the heavy reliance on labor and resources in current agricultural development, with the transformation of labor structure emerging as a pivotal factor influencing progress. Notably, the trends of feminization, aging, and urbanization in rural labor have precipitated a substantial shortage, impeding agricultural development. Furthermore, under the guidance of digital technology and fuzzy systems, this paper establishes the theoretical framework of green ATFP. Evaluation results disclose a noteworthy decrease in the contribution rates of various indicators under the Green ATFP model, with the highest contribution rate approximating 1.23. This implies that the existing measures for China’s green ATFP are still imperfect, signaling a need for greater emphasis on environmental considerations in agricultural development. This work furnishes valuable insights for advancing agricultural development and contributes significantly to the ongoing efforts in promoting the green evolution of Chinese agriculture.
1. Introduction
Technological progress, as the linchpin of social development, emphasizes the imperative of research across various sectors of social production supported by science and technology (Vargo et al., Citation2021). This strategic approach propels comprehensive societal development and conserves valuable social resources. Agriculture, fundamental to people’s livelihoods and a nucleus of social progress, necessitates meticulous scientific and technological investigation to foster sustainable development and optimize the utilization of agricultural resources. Despite the growing importance of science and technology in various sectors of social production, a conspicuous research gap persists in the domain of green agricultural total factor productivity (ATFP), particularly concerning the analysis of temporal and spatial variations within the context of Chinese green agriculture. In light of these considerations, this work seeks to unveil the temporal and spatial differentiation characteristics of Chinese green ATFP and its influencing factors to provide a scientific foundation for the formulation of targeted policy measures (Hu et al., Citation2021). To achieve this objective, this work employs the Data Envelopment Analysis (DEA) method to assess green ATFP in China. DEA, a non-parametric method for evaluating relative efficiency, excels in handling complex systems with multiple inputs and outputs, rendering it particularly suited for evaluating green ATFP. Furthermore, this work utilizes the Malmquist productivity index method to dissect changes in green ATFP across different temporal and spatial dimensions, shedding light on the sources of variations in this critical field.
Li et al. (Citation2021) reported that 80% of the provinces and autonomous regions in China could rely on local advantages to adjust the agricultural structure in an orderly manner. A new pattern of industrial belts in advantageous agricultural production areas has taken shape, and the growth rate of characteristic agricultural output value has reached 3.1%. However, the differences in regional effects are also prominent. Generally speaking, the north is better than the south, the Yellow River Basin is better than the Yangtze River Basin, and the traditional agricultural area is better than the general area. The provinces with better matching of comparative advantages and adjustment effects of agrarian structure adjustment include Mongolia, Kyrgyzstan, Henan, Shandong, Qiong, Shaanxi, Gan, Ning, Xinhe, and Tibet; the provinces with poor matching include Beijing, Tianjin, Heilongjiang, Hubei, Gui, green. Regional policy regulation plays a particularly important role in adjusting agricultural structure (Li et al., Citation2021). Ahmad et al. (Citation2021) calculated China’s agricultural carbon emissions from 1993 to 2008 based on six primary carbon sources in agricultural production. They found that China’s agricultural carbon emissions have been in a phased upward trend since 1993, which can be divided into four stages of change: fast growth period, slow growth period, rebound period of growth rate, and apparent slowdown period of growth rate. The average annual growth rate of agricultural carbon emissions and intensity was 4.08% and 2.38%, respectively. The areas with high total agricultural carbon emissions were mainly concentrated in large agricultural provinces, and the areas with high agricultural carbon emission intensity were mainly concentrated in developed cities, developed eastern coastal provinces, and central agricultural provinces. At the same time, there were still big misunderstandings in the control of non-point source pollution in major river basins in China. The main problem is that there was no classification control for point and non-point source pollution. At present, even in the basin where agricultural non-point source pollution has become the primary source of water pollution; the construction of terminal control projects such as estuaries and river areas is still the mainstay. Since the basic principles of agricultural non-point source pollution control were not followed, the entire basin was distributed uniformly, with full consideration given to local rural economic conditions and existing planting structures, and the interests of farmers were taken into maximum consideration. The total amount of different types of pollution was controlled at the source. The area of vegetable fruit and flower farmland with high nitrogen and phosphorus nutrient consumption was still increasing significantly in many areas, making it more difficult to control river basin pollution in the future (Ahmad et al., Citation2021). Liu et al. (Citation2022) pointed out that source control of different types of non-point source pollution sources should be established as soon as possible in river basins with serious water pollution and areas with high pollution risk according to the current situation in China. Based on the need for water source protection, local climate, hydrogeology, and terrain should be taken into consideration in farmland soil conditions, reasonable delineation of water source protection zones at all levels in the basin, and the corresponding restrictive agricultural production technical standards should be formulated and tried out. Sufficient experimental research is the premise to guarantee the scientificity, rationality and operability of the standard. The authors suggested that the green box policy should be piloted in qualified areas. Besides, farmers should be encouraged and promoted to widely adopt alternative technologies and production models that protect the environment to improve the utilization rate of agricultural resources and promote the upgrading of agricultural technologies (Liu et al., Citation2022).
This work builds upon a robust foundation, delving into the nuanced applications of digital technology and fuzzy systems. It comprehensively examines the state of agricultural development in China, culminating in the construction of the ATFP model tailored for green agriculture in China, grounded in the principles of digital technology and fuzzy systems. Through this research, this work extends technical support to the evolution of agriculture in China, thereby contributing significantly to the conservation of societal resources. Precisely, digital technology is leveraged to analyze diverse elements in agricultural production, encompassing land, labor, and capital. Additionally, the fuzzy systems approach is harnessed to address the inherent uncertainties and ambiguities prevalent in agricultural production. By synergistically integrating these two technological approaches, a model capable of comprehensively assessing the ATFP of green agriculture in China is established, unraveling the spatiotemporal differentiation characteristics of agricultural production efficiency and its influencing factors. This work not only bridges a critical gap in the research landscape of ATFP in Chinese green agriculture but also furnishes valuable references for the formulation and implementation of agricultural policies. Its insights play a pivotal role in propelling Chinese agriculture towards a greener and more sustainable trajectory.
2. Research theories and methods
2.1. Digital technology
Digital technology, a scientific and technological domain executed through computers, holds the capability to convert diverse information types—images, text, and sound—into binary digits, comprehensible by electronic computers. This conversion facilitates computation, processing, storage, transmission, and dissemination, collectively shaping the landscape of digital technology. Commonly known as digital computer technology, it is integral to the processes of operation and storage, necessitating information encoding, compression, and decoding (Li et al., Citation2021). In the backdrop of society’s incessant evolution, the contemporary human milieu has transitioned into the digital era, where daily activities are mediated through the Internet of Things (IoT). This includes interpersonal communication, as well as routine activities such as learning, work, and daily life. Smart home network systems, predominantly orchestrated by computers, have successfully interconnected households, significantly simplifying people’s lives. Digital technology has emerged as the predominant technological force, permeating every facet of human society (Vuorre et al., Citation2021).
In the context of green agriculture development, digital technology assumes a pivotal role. As an illustrative example, precision agriculture technology employs digital technology to provide farmers with precise information about soil conditions and crop growth, facilitating the formulation of rational planting plans. Consequently, the reduction in the use of fertilizers and pesticides helps mitigate adverse environmental impacts. Moreover, the application of digital technology in agricultural IoT enables comprehensive monitoring and management of the entire agricultural production process, thereby augmenting production efficiency and elevating the quality of agricultural products. Consequently, digital technology stands poised as a formidable driving force for the future development of green agriculture.
reveals the main application scenarios of digital technology.
As shown in , with the continuous upgrading and penetration of digital technology, it is becoming a major development direction of modern society. Digital design courses also provide the impetus for this development direction. Through the development of digital design technology, various technical ideas have emerged in an endless stream for decades, but the synergistic effect of time and space has always been the mainstream of development (Nikou & Aavakare, Citation2021). From a macro perspective, the digital design and development process is equivalent to applying the latest information technology from point to line and line to surface to the field of product design. Extensive simulation applications are becoming a major trend in today’s digital design technology development. With the introduction of the concept of virtual prototyping, the application of simulation technology tends to be collaborative and systematic. Carrying out research on virtual prototypes and their key technologies will definitely improve the independent design and development capability of enterprises and accelerate the process of enterprise informatization (Mager & Katzenbach, Citation2021).
2.2. Fuzzy system
Fuzzy systems, rooted in knowledge or rules, derive their essence from a knowledge base constructed through IF-THEN algorithmic rules. Consequently, fuzzy systems can be characterized as amalgamations of IF-THEN rules. Presently, the application methodologies of fuzzy systems predominantly span communication, signal processing, and automatic control (Cai et al., Citation2021). These advanced and theoretical fuzzy methods hold considerable promise for expansive development. Fuzzy theory, serving as the principal guiding tenet of fuzzy systems, encompasses the foundational principles of fuzzy systems and various facets such as fuzzy mathematics, artificial intelligence, fuzzy logic, uncertainty information and theory, and fuzzy decision-making. These elements assume pivotal roles in the evolution of model theory and fuzzy systems. Among them, fuzzy logic, mathematics, and decision-making have the most substantial impact on fuzzy systems (Zheng et al., Citation2021).
The genesis of fuzzy mathematics lies in the replacement of classical sets with fuzzy sets, thereby extending the foundational concept of sets in classical mathematics and giving rise to the discipline of fuzzy mathematics. Within the theoretical framework of classical set concepts, the relationship between two elements adheres to the “either-or” classical thinking, while fuzzy sets delve into fuzzy phenomena and process them with logical nuance (Abedi Gheshlaghi & Feizizadeh, Citation2021). Fuzzy theory, breaking away from the rigid “either-or” paradigm, advances the concept of “both this and that.” The development of approximate reasoning in classical logic plays a pivotal role in the evolution of fuzzy logic, and the integration of fuzzy theory and approximate reasoning gives rise to the development of expert systems. Expert systems, classified under artificial intelligence, demonstrate dynamic performance. The underlying principle of expert systems involves translating a substantial corpus of experiential knowledge, expressible in natural language, into computer algorithms through algorithmic methodologies (Kisi et al., Citation2021).
Founded on rules and knowledge, the computational core of fuzzy systems, grounded in fuzzy theory, is centered on IF-THEN algorithmic rules. These rules effectively articulate system problems expressed in natural language through algorithmic constructs (Huang et al., Citation2021). The application of IF-THEN in fuzzy systems involves amalgamating these rules to construct a hybrid IF-THEN fuzzy system, encompassing fuzzy processing methodologies and theories in communication, signal processing, and automation control. Fuzzy decision-making emerges from the synergy and transformation of fuzzy set theory and decision theory, representing a mathematical framework for addressing problems in a fuzzy environment. Common fuzzy decision-making methods include fuzzy ranking, fuzzy strategy, and fuzzy optimization, with wide-ranging applications and a pivotal role in resolving real-world issues (Liu et al., Citation2021).
In conclusion, fuzzy theory stands as a primary theoretical foundation for addressing societal challenges, providing highly effective avenues for researching and solving social problems. Additionally, spatiotemporal differentiation research holds the potential to drive advancements in the development of Chinese agriculture, rendering the research presented in this paper of significant value and significance.
2.3. Agriculture development in China
The status of agriculture in a country is imperative. It is related to the basic needs of human beings. It is the basis for the survival and development of human society and the fundamental element of human survival. After the market-oriented reform, China’s agriculture has achieved unprecedented development and miraculous results (Singh et al., Citation2021). The growth rate of China’s agricultural output value is close to 6.5%, the growth rate of farmers’ per capita net income is over 7.9%, and the country has achieved a food self-sufficiency rate of over 95%. However, the development of agriculture is still not ideal, and there are many problems in the current agricultural development process (Chaudhuri et al., Citation2021). The main source of the problem is China’s agricultural growth model. The traditional agricultural growth model in China is extensive growth, characterized by high input and high emissions, resulting in high pollution and low efficiency (Adedoyin et al., Citation2021). Therefore, it is an important task of current agricultural development to study the development of China’s agriculture through the concept of green agriculture to improve the production problems and agricultural problems brought by traditional agriculture and to promote its comprehensive sustainable development (Dabkiene et al., Citation2021).
The existing problem of traditional Chinese agriculture is lacking stamina of production factors; that is, the development prospect of traditional Chinese agriculture is limited. Since the establishment of agriculture in China, factors such as land, water resources, and labor have always been necessary conditions for agricultural development, but the current situation is not optimistic (Yao et al., Citation2021). First of all, the current agricultural land in China is decreasing. The land is the main carrier of agricultural planting, so the scale of agricultural arable land is a direct factor reflecting the level of agricultural development. However, China’s agricultural arable land has dropped from 1.95 billion mu (130,000 square kilometers) in 1996 to 1.87 billion mu (124,666.67 square kilometers) in 2020, and the area of agricultural arable land is constantly approaching the red line of China’s agricultural arable land area (Lee et al., Citation2023). Second, agricultural water resource quantity and quality both drop. With the development of social industry and the continuous advancement of urbanization, the current social, and agricultural water consumption has been squeezed to a large extent, so the agricultural water consumption is showing a continuous decline. Therefore, there will be insufficient agricultural users in the future development of agriculture. The phenomenon is an inevitable trend (Campi & Nuvolari, Citation2021). In 2000, the proportion of agricultural users accounted for about 68.9% of China’s total water consumption, but China’s agricultural water consumption in 2020accounted for about 61.0%. At the same time, the current level of water pollution is also deepening, and industrial discharge and agricultural discharge have a great impact on water resources (El-Shirbeny et al., Citation2021). In addition, the waste of water resources has an important impact on China’s agricultural water use. Finally, the change in labor force structure is one of the important factors affecting the development of China’s agriculture. The development of agriculture in China in the early days was based on the demographic dividend, and the current demographic dividend in China is gone forever, and the current structure of China’s agricultural labor force has undergone great changes (Zhao et al., Citation2023). Population aging, feminization, and urbanization are the inevitable trends in the development of China’s agricultural labor force. As of 2021, the proportion of people over 60 years old in China was over 18.9%. However, the development of agriculture needs to rely on a strong labor force, so the trend of aging has an important impact on China’s agriculture. Moreover, the feminization and urbanization of the agricultural labor force are also reducing the development of the agricultural labor force. Thus, the current labor force has become one of the important factors restricting the development of agriculture (Yu, Citation2022).
Moreover, the development of modern agricultural production factors in China is not perfect. The neoclassical economic growth theory considers productivity to be another important factor in improving economic growth and promoting sustainable economic development after labor and capital. Therefore, productivity is usually regarded as a modern factor of production (Tsebee, Citation2021). The improvement of China’s agricultural productivity depends on the investment in mechanical technology and science and technology, but current China’s agricultural technology is still relatively backward compared with developed countries. In addition, China’s agricultural development still lacks agricultural technology talents and lacks the popularization of agricultural technology (Ngoc Huy et al., Citation2021).
Furthermore, environmental capacity is another essential factor limiting agricultural development in China. The development of China’s agriculture is undeniable. Nevertheless, it has significantly impacted China’s environment. Chinese agriculture relies on factors such as pesticides, fertilizers, and plastic film to improve crop yield, but these factors have negligible effects on the environment (Mogborukor, Citation2021). Therefore, reducing environmental pollution and developing green agriculture should be the main goals of China’s agricultural development.
3. Construction of the green ATFP model
3.1. Green ATFP
Factor productivity (FP) is an important indicator to measure performance in economics. Production factor mainly includes single FP and total FP, while productivity mainly includes factors such as labor, capital, and energy (Pandey & Yadav, Citation2021). With the development of society, the important factor of the environment is included in the productivity measurement index system. ATFP concept was born based on this (Karimzadeh, Citation2021). The research model constructed here is a green ATFP model based on the coordinated development of resources, energy, environment, and economy.
There are two main calculation methods of green ATFP: parametric methods and non-parametric methods. Parametric methods include the algebraic index method, the stochastic frontier analysis method, and the Solow residual value method (İsmail & Arisoy, Citation2021). The non-parametric methods contain the Shephard distance function and Malmquist index, data envelopment analysis method, directional distance function, and Malmquist-Luenberger index (Madanayake et al., Citation2021). Time is usually chosen as the main data index in the selection of research indexes. The main factors of variable selection are land, labor, energy, emissions, fertilizer input, and other factors.
3.2. Model construction
This work endeavors to explore the influence of green ATPF on the Chinese agricultural sector, with the overarching objective of advancing the sustainable development of agriculture in China, enhancing rural environments, and expediting the establishment of aesthetically pleasing countryside landscapes. To achieve these goals, this work harnesses panel data encompassing 30 Chinese provinces and municipalities, spanning the period from 2000 to 2020. The data sources are drawn from multiple authoritative channels, including the National Bureau of Statistics, the China Environmental Monitoring Station, statistical yearbooks from various provinces and municipalities, as well as pertinent government reports. This data selection ensures the utmost accuracy and reliability.
Throughout this work, a comprehensive overview of the panel data is presented. Firstly, in consideration of data representativeness and reliability, the present work judiciously cherry-picked the 30 provinces and municipalities in China as the research sample. These regions encompass China’s principal agricultural production zones, ensuring a wide spectrum of representativeness and data reliability.
Secondly, the work covers a substantial temporal expanse from 2000 to 2020, spanning 21 years. This duration encapsulates long-term trends and transformations within the realm of green development in Chinese agriculture. Over this period, China witnessed rapid economic growth and underwent shifts in environmental policies while several noteworthy advancements unfolded in the agricultural domain. This extensive time frame enables this work to comprehensively examine the impact of these alterations on the green ATPF of Chinese agriculture.
In terms of research content, this work thoroughly analyzes the theoretical framework of green ATPF. This framework encompasses a range of indicators across various dimensions, including hydropower energy, non-point source pollution factors in agriculture, and agricultural emissions. Additionally, spatial and temporal difference analyses are executed to shed light on the disparities in green ATPF across diverse regions and timeframes. The insights garnered from these analytical findings significantly contribute to an enhanced comprehension of the present status and future developmental trajectories within the domain of green agricultural development in China.
Moreover, this work employs a spectrum of highly effective statistical analysis methodologies, incorporating literature review, comparative analysis, and qualitative analysis employing fuzzy sets. The strategic use of these methods equips this work to holistically evaluate the current state of green ATPF in China, discern the factors influencing it, and elucidate forthcoming developmental trends. Furthermore, this endeavor aspires to furnish valuable points of reference and support for the prospective modernization of Chinese agriculture through these in-depth analyses.
Within the realm of model construction, this work intricately addresses several key facets:
3.2.1. Model establishment
The work presents the construction of an ATPF model rooted in a production function. This model incorporates crucial input variables, namely hydropower energy, agricultural non-point source pollution factors, and agricultural emissions, amalgamating diverse parameters associated with the environment and time.
3.2.2. Model interpretation
Within the framework of a traditional production function, the model takes agricultural total output as the dependent variable, with hydropower energy, agricultural non-point source pollution factors, and agricultural emissions serving as independent variables. The model introduces a set of control variables, including time-related parameters, and integrates disturbance terms. By employing regression analysis, this framework facilitates the derivation of the elasticity of green ATPF relative to each input variable. This enables an assessment of the contribution of various factors to agricultural total output and the efficiency level of green ATPF.
3.2.3. Data sources and processing
This work draws upon panel data spanning the years 2000 to 2020 from 30 provinces and municipalities in China. Data were meticulously sourced from authoritative institutions, including the National Bureau of Statistics, China Environmental Monitoring Station, statistical yearbooks from various provinces and municipalities, and relevant government reports. Rigorous data preprocessing was conducted, involving the elimination of missing values, outliers, and duplicate entries, ensuring the reliability and accuracy of regression results.
3.2.4. Model estimation and testing
Various regression analysis techniques, including pooled regression, fixed-effects regression, and random-effects regression, are employed to evaluate the stability and reliability of the model. Rigorous assessments for multi-collinearity and autocorrelation are conducted to uphold the effectiveness and credibility of the model.
3.2.5. Research assumptions
The ATPF model is posited to effectively assess the contribution of distinct input variables to agricultural total output and provide insights into green agricultural production’s efficiency.
3.2.6 Research objectives
This work endeavors to construct a production function-based green ATPF model, utilizing panel data from 30 provinces and municipalities in China for empirical analysis. The primary objective is to evaluate the contributions of diverse input variables to agricultural total output and the efficiency level of green ATPF. Through meticulous model estimation and testing, this research aspires to provide a reliable and effective tool for assessing and enhancing the production efficiency of green agriculture.
3.3. Theoretical calculation basis
The main reference factor in the research process is spatial heterogeneity, that is, the different phenomena or things in each spatial location. The fundamental reasons for its emergence are as follows. (1) It is difficult for each phenomenon or thing to maintain a balance in each spatial location. (2) The characteristics of spatial units are different. The Data Envelopment Analysis (DEA) is utilized. It uses data as the driving factor, does not need to set the function form and factor price information, and can analyze and evaluate a variety of cross-period samples. The reference functions include the Shard distance function and the Malmquist productivity index, the directional distance function and the Malmquist-Luenberger productivity index, and the directional distance function and the Luenberger productivity index.
1) EquationEquation (1)(1)
(1) describes the Shard distance function and Malmquist productivity index.
(1)
(1)
2) The direction distance function and Malmquist-Luenberger productivity index can be expressed as:
(2)
(2)
(3)
(3)
(4)
(4)
(5)
(5)
(6)
(6)
(7)
(7)
3) The directional distance function and Luenberger productivity index can be written as:
(8)
(8)
(9)
(9)
(10)
(10)
In the above Equations, represents the input factor;
stands for the expected output;
denotes the sequence of input factors;
refers to the undesired output;
signifies the period;
represents the maximum possible expansion multiple of the output vector;
denotes the probability. Based on this, this work studies the temporal and spatial differentiation of China’s green ATFP to comprehensively analyze the development of China’s agricultural modernization and contribute to the sustainable development of China’s agriculture. The data used here are all from the China Agricultural Yearbook website.
3.4. Theoretical framework of spatial-temporal differentiation of China’s green ATFP
The main factor for the development of green agriculture in China is the environmental problem. Specifically, it is essential to reduce the damage to the environment, ensure the sustainable development of agriculture, and speed up the process of China’s agriculture entering a modern state in the process of agricultural development. Therefore, the agricultural resource and environmental technology set can be described as EquationEquation (11)(11)
(11) .
(11)
(11)
In EquationEquation (11)(11)
(11) , the meaning of all parameters is the same as the previous one. After conversion, EquationEquation (11)
(11)
(11) can be expressed as:
(12)
(12)
(13)
(13)
(14)
(14)
(15)
(15)
where represents the density variable, and its main meaning is the weight of the decision-making unit. Among them, input variables mainly include labor, land, capital, water resources, energy, and other inputs. Output variables mainly include expected output and undesired output. The agricultural non-point source pollution needs to be accounted for first in the environmental assessment. lists the standard of agricultural non-point source pollution.
Table 1. Agricultural non-point source pollution standard.
As shown in , Total Nitrogen (TN), Total Phosphorus (TP), and Chemical Oxygen Demand (COD) are important indicators in sewage detection. They are calculated according to EquationEquation (16)(16)
(16) .
(16)
(16)
In EquationEquation (16)(16)
(16) ,
stands for the total amount of agricultural non-point source pollution emissions;
represents the index of
;
denotes the pollution intensity coefficient;
signifies the resource utilization coefficient;
refers to the amount of pollution;
represents the pollution discharge coefficient;
indicates the spatial feature. presents the measurement index system of green ATFP reported here.
Table 2. Calculation index system of green ATFP.
As illustrated in , the weights, denoted within parentheses following the indicator names, are computed utilizing the entropy weight method. These indicators serve as instrumental tools for scrutinizing the green ATFP over the course of agricultural development in China, offering a means to evaluate the comprehensive developmental status of Chinese agriculture. Notably, the inclusion of water as an output indicator is imperative, given its indispensable and pivotal role as a critical resource in agricultural production.
4. Research on the temporal and spatial differentiation of China’s green ATFP
4.1. Analysis of agricultural production factors in China
Labor force, water resources, and land are all necessary factors in agricultural development. Therefore, this work firstly studies the basic elements in the process of China’s agricultural development to explore the changes in the basic elements of agriculture in the process of China’s agricultural development and analyze China’s agricultural development prospects. shows the changes in the basic elements of China’s agricultural development.
Figure 2. Changes in the basic elements of China’s agricultural development (a): labor force; (b): rural and urban population changes; (c): agricultural product consumption; (d): per-capita agricultural output value).

As depicted in , the proportion of females in rural areas is steadily increasing, accompanied by a simultaneous deepening of population aging. Presently, the agricultural workforce in rural areas is predominantly comprised of women and the elderly. The rapid pace of urbanization has further exacerbated the demographic shift, resulting in a decline in the rural population and a concomitant rise in urban residents, ultimately reducing individuals engaged in agricultural activities. Moreover, the escalating use of agricultural products in production activities has undeniable ramifications for the environment. Concurrently, regional disparities in agricultural development significantly impact overall agricultural progress. A series of strategic measures is imperative to effectively address these multifaceted issues. There is an urgent need to bolster education and training initiatives in rural areas, elevating farmers’ knowledge and skill levels and encouraging their participation in modernized agricultural practices. Secondly, governmental investment in agriculture must be augmented, focusing on promoting advanced agricultural technologies and equipment to enhance production efficiency and quality. Additionally, rigorous quality supervision of agricultural products should be instituted, advocating for green and organic agricultural production methods to mitigate adverse environmental impacts. Finally, fostering collaboration and communication among different regions is essential, facilitating the sharing of agricultural resources and cooperative development to achieve sustainable agriculture.
4.2. Analysis of China’s green ATFP
According to the above theoretical framework, this work first analyzes the green ATFP under different models. presents the changes in agricultural total production factors under different models.
Figure 3. Changes of green agricultural total production factors under different models (a) and (b) are changes under the productivity model; c and d are changes under the green ATFP model).
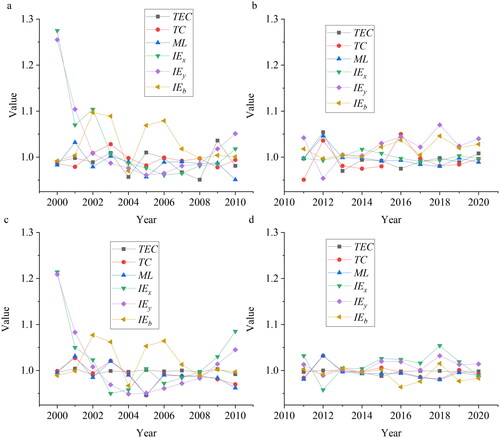
As depicted in , in productivity models that do not account for green resources, the contribution rates of each evaluation indicator maintain a relatively high level, with the highest contribution rate reaching approximately 1.27. However, a significant shift occurs when transitioning to the green ATFP model. In this model, the contribution rates of each indicator experience a noticeable decline, with the highest contribution rate reaching only about 1.23. This shift highlights the current deficiencies in China’s strategies for green agricultural total factor productivity and underscores the imperative for a more balanced approach between agricultural development and environmental protection in China. Evidently, China’s investments and initiatives in green agricultural development have not yielded the anticipated results, potentially attributed to a lack of scientifically effective management and planning or insufficient understanding of the intricate relationship between environmental protection and agricultural development. Hence, China must prioritize green and sustainable principles in future agricultural development plans. This encompasses but is not limited to enhancing environmental awareness among farmers, advocating for environmentally friendly agricultural technologies, optimizing agricultural production structures, and fortifying the protection and restoration of agricultural ecosystems. Only through such comprehensive efforts can China ensure agricultural production while minimizing the adverse impact on the environment, achieving authentic green and sustainable agricultural development.
4.3. Comprehensive analysis of green ATFP development in China
Conducting a comprehensive analysis of green ATFP development in Chinese agriculture necessitates the consideration of multiple factors. Green ATFP is a pivotal metric designed to gauge the capacity for resource utilization, environmental preservation, and economic efficiency enhancement within agricultural production processes. Hence, this work undertakes a comprehensive evaluation of Chinese green ATFP development spanning 2000 to 2020. The developmental status in the years 2000, 2005, 2010, 2015, and 2020 serves as the primary analytical benchmarks. The research findings are illustrated in .
Figure 4. Analysis of the development status of green ATFP in China (a) for the year 2000, (b) for 2005, (c) for 2010, (d) for 2015, and (e) for 2020.
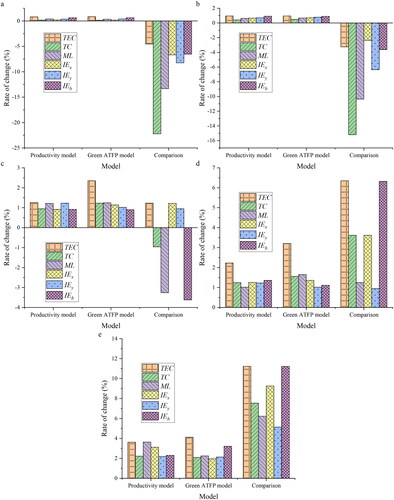
As depicted in , the data spanning from 2000 to 2020 illustrates a consistent upward trajectory in China’s green ATFP. This developmental journey can be classified into the following distinct stages:
First Stage (2000–2005): This phase saw green ATFP advancing at a relatively modest pace. Constrained by the prevailing level of economic development, agricultural inputs remained limited, and initiatives to promote technological innovation and eco-friendly production practices were somewhat sluggish.
Second Stage (2006–2010): green ATFP’s growth rate gained momentum during this interval. Notably, substantial strides were made in technological innovation, and governmental investments in agriculture witnessed annual increments, further facilitating the augmentation of green ATFP.
Third Stage (2011–2015): The growth rate of green ATFP continued its accelerated ascent. Transforming and upgrading the agricultural industry became a national strategic priority. Simultaneously, as urbanization gathered speed and the aging population expanded, labor shortages in agriculture became more pronounced. However, the rapid progression of agricultural mechanization adeptly offset this deficiency. The extensive dissemination and application of green production technologies played a pivotal role in elevating green ATFP.
Fourth Stage (2016–2020): The pace of green ATFP’s growth began to decelerate during this phase. Challenges linked to resource scarcity and environmental contamination assumed increasing prominence. Additionally, mounting agricultural production costs and intensified market competition placed pressure on the enhancement of green ATFP. The Chinese government responded by bolstering support for agriculture, advancing structural reforms on the supply side of agriculture, and fortifying ecological environmental conservation measures, ensuring the sustained growth of green ATFP.
The continuously evolving data spanning from 2000 to 2020 underscores the sustained progress in China’s green ATFP. Each phase encountered distinct challenges, yet the Chinese government implemented a suite of effective policies and measures, resulting in positive outcomes. With the ongoing advancement of technology and the dynamic socioeconomic landscape, China’s green ATFP is anticipated to maintain a stable growth trajectory. The distinctive contribution of this work lies in its thorough research and analysis, revealing that in advancing green ATFP, the Chinese government not only prioritizes technological innovation and application but also places significant emphasis on policy formulation and implementation. These policies encompass support and subsidies for green agricultural technologies, training and education for farmers, and initiatives for the protection and enhancement of agricultural ecological environments. The meticulous implementation of these policies and measures provides robust assurance and support for the development of green ATFP in China. Therefore, it is asserted that the Chinese government has garnered crucial experience and achievements in promoting green ATFP, serving as valuable insights and references for other countries and regions. Furthermore, the anticipation is that China will continue to innovate and make significant contributions to global agricultural sustainability in the future.
5. Conclusion
In the pursuit of societal progress, the prominence of green production principles has intensified, with agriculture leading this transformative paradigm. This work elucidates the intricate relationship between digital technology, fuzzy systems, and the evolution of Chinese agriculture. Crucially, the research introduces a study model for Chinese green ATFP and conducts a comprehensive spatiotemporal assessment through empirical investigation. This work results underscore a significant phenomenon: the agricultural landscape is undergoing profound changes, marked by an increasing proportion of the rural female population and an escalation of population aging. The agricultural workforce, predominantly comprising women and elderly individuals, is diminishing amidst urbanization, resulting in a reduction in agricultural labor. Concurrently, the consumption of agricultural resources for production is escalating, albeit with an imbalanced pattern across different regions. Additionally, this work presents an illustrative green ATFP model. Model analysis reveals that, compared to productivity models, this model has a markedly lower impact, with a maximum contribution rate of only 1.23. This finding underscores the misalignment between the current trajectory of agricultural development and the imperatives of green ATFP, emphasizing the gradual need to enhance the sustainability of agriculture. However, it is crucial to acknowledge that, while this work encompasses the green production paradigm, digital technology in agriculture, and fuzzy systems, and designs a comprehensive model for Chinese green ATFP, it bears certain limitations and uncertainties. Firstly, due to constraints in data acquisition, the dataset may not comprehensively reflect the actual situation. Secondly, despite constructing a relatively comprehensive model, there are limitations in exploring the complexity of specific factors. Additionally, despite emphasizing the necessity of green agricultural development, this work does not delve into the specific details of implementation and improvement. Future research needs to delve deeper into the constituents of the model, enhancing its analytical and applicative capabilities. Simultaneously, an in-depth exploration of strategies and methodologies for achieving green agricultural development will be indispensable in guiding towards a more effective path of sustainable agricultural development.
Authors’ contributions
C.C and B. mainly contribute this work conception and design; C.C, B. and Y.P. (Sun) are mainly responsible for data collection; C.C, B., Y.P. (Sun) and Y.P. (Xiao) mainly contribute to the analysis and interpretation of results; Y.P. (Sun) and Y.P. (Xiao) draft the manuscript. C.C and B. are responsible for manuscript reviewing and editing. All authors reviewed the results and approved the final version of the manuscript.
Disclosure statement
The author(s) declare no competing interests.
Data availability statement
The datasets generated during and/or analyzed during the current study are available from the corresponding author on reasonable request.
Additional information
Funding
References
- Abedi Gheshlaghi, H., & Feizizadeh, B. (2021). GIS-based ensemble modelling of fuzzy system and bivariate statistics as a tool to improve the accuracy of landslide susceptibility mapping. Natural Hazards, 107(2), 1–14. https://doi.org/10.1007/s11069-021-04673-1
- Adedoyin, F. F., Bein, M. A., Gyamfi, B. A., & Bekun, F. V. (2021). Does agricultural development induce environmental pollution in E7? A myth or reality. Environmental Science and Pollution Research International, 28(31), 41869–41880. https://doi.org/10.1007/s11356-021-13586-2
- Ahmad, F., Fouad, H., Liang, S.-Y., Hu, Y., & Mo, J.-C. (2021). Termites and Chinese agricultural system: Applications and advances in integrated termite management and chemical control. Insect Science, 28(1), 2–20. https://doi.org/10.1111/1744-7917.12726
- Cai, X., Wang, J., Zhong, S., Shi, K., & Tang, Y. (2021). Fuzzy quantized sampled-data control for extended dissipative analysis of T–S fuzzy system and its application to WPGSs. Journal of the Franklin Institute, 358(2), 1350–1375. https://doi.org/10.1016/j.jfranklin.2020.12.002
- Campi, M., & Nuvolari, A. (2021). Intellectual property rights and agricultural development: Evidence from a worldwide index of IPRs in agriculture (1961–2018). The Journal of Development Studies, 57(4), 650–668. https://doi.org/10.1080/00220388.2020.1817395
- Chaudhuri, S., Roy, M., McDonald, L. M., & Emendack, Y. (2021). Reflections on farmers’ social networks: A means for sustainable agricultural development? Environment, Development and Sustainability, 23(3), 2973–3008. https://doi.org/10.1007/s10668-020-00762-6
- Dabkiene, V., Balezentis, T., & Streimikiene, D. (2021). Development of agri-environmental footprint indicator using the FADN data: Tracking development of sustainable agricultural development in Eastern Europe. Sustainable Production and Consumption, 27(11), 2121–2133. https://doi.org/10.1016/j.spc.2021.05.017
- El-Shirbeny, M. A., Ali, A. M., Khdery, G. A., et al. (2021). Monitoring agricultural water in the desert environment of New Valley Governorate for sustainable agricultural development: A case study of Kharga. Euro-Mediterr J Envi, 6(2), 1–15.
- Hu, J., Wang, Z., & Huang, Q. (2021). Factor allocation structure and green-biased technological progress in Chinese agriculture. Economic Research-Ekonomska Istraživanja, 34(1), 2034–2058. https://doi.org/10.1080/1331677X.2020.1860795
- Huang, Y., Chen, D., Zhao, W., & Mo, H. (2021). Deep fuzzy system algorithms based on deep learning and input sharing for regression application. International Journal of Fuzzy Systems, 23(3), 727–742. https://doi.org/10.1007/s40815-020-00998-4
- İsmail, A., & Arisoy, H. (2021). International fund for agricultural development and evaluation of Turkey’s practices. Tarım Ekonomisi Dergisi, 27(1), 39–47.
- Karimzadeh, M. (2021). Prioritizing the barriers to agricultural development in rural areas of Saravan. Co-Operation and Agriculture, 10(37), 153–180.
- Kisi, O., Khosravinia, P., Heddam, S., Karimi, B., & Karimi, N. (2021). Modeling wetting front redistribution of drip irrigation systems using a new machine learning method: Adaptive neuro-fuzzy system improved by hybrid particle swarm optimization–Gravity search algorithm. Agricultural Water Management, 256(2), 107067. https://doi.org/10.1016/j.agwat.2021.107067
- Lee, C. C., He, Z. W., & Yuan, Z. (2023). A pathway to sustainable development: Digitization and green productivity. Energy Economics, 124, 106772. https://doi.org/10.1016/j.eneco.2023.106772
- Li, J.-P O., Liu, H., Ting, D. S. J., Jeon, S., Chan, R. V. P., Kim, J. E., Sim, D. A., Thomas, P. B. M., Lin, H., Chen, Y., Sakomoto, T., Loewenstein, A., Lam, D. S. C., Pasquale, L. R., Wong, T. Y., Lam, L. A., & Ting, D. S. W. (2021). Digital technology, tele-medicine and artificial intelligence in ophthalmology: A global perspective. Progress in Retinal and Eye Research, 82(9), 100900. https://doi.org/10.1016/j.preteyeres.2020.100900
- Li, L., Lou, W., Kong, L., & Shen, W. (2021). Hydrogen commonly applicable from medicine to agriculture: From molecular mechanisms to the field. Current Pharmaceutical Design, 27(5), 747–759. https://doi.org/10.2174/1381612826666201207220051
- Liu, S., Deichmann, M., Moro, M. A., Andersen, L. S., Li, F., Dalgaard, T., & McKnight, U. S. (2022). Targeting sustainable greenhouse agriculture policies in China and Denmark: A comparative study. Land Use Policy, 119(7), 106148. https://doi.org/10.1016/j.landusepol.2022.106148
- Liu, F., Sekh, A. A., Quek, C., Ng, G. S., & Prasad, D. K. (2021). RS-HeRR: A rough set-based Hebbian rule reduction neuro-fuzzy system. Neural Computing and Applications, 33(4), 1123–1137. https://doi.org/10.1007/s00521-020-04997-2
- Madanayake, N. H., Hossain, A., & Adassooriya, N. M. (2021). Nanobiotechnology for agricultural sustainability, and food and environmental safety. Quality Assurance and Safety of Crops & Foods, 13(1), 20–36. https://doi.org/10.15586/qas.v13i1.838
- Mager, A., & Katzenbach, C. (2021). Future imaginaries in the making and governing of digital technology: Multiple, contested, commodified. New Media & Society, 23(2), 223–236. https://doi.org/10.1177/1461444820929321
- Mogborukor, J. O. (2021). The physio-chemical characteristics of agbor soils in delta state and the implications for agricultural development. International Journal of Physical and Human Geography, 9(1), 1–12.
- Ngoc Huy, D. T., Van Tuan, P., Dinh Trung, N., Thi Huyen, D., Thi Hang, N., Bich Hong, N., Thu Ha, L., & Thi Sun, B. (2021). Management issues of tea planting and tea crops in Vietnam in the concept of sustainable agricultural development-and recommendations on marketing 4P. Tobacco Regulatory Science, 7(5), 1784–1803. https://doi.org/10.18001/TRS.7.5.102
- Nikou, S., & Aavakare, M. (2021). An assessment of the interplay between literacy and digital technology in higher education. Education and Information Technologies, 26(4), 3893–3915. https://doi.org/10.1007/s10639-021-10451-0
- Pandey, S., & Yadav, D. K. (2021). Role of agricultural credit in Indian agricultural development. Indian Journal of Agricultural Marketing, 35(1), 260–260.
- Singh, H., Sharma, A., Bhardwaj, S. K., Arya, S. K., Bhardwaj, N., & Khatri, M. (2021). Recent advances in the applications of nano-agrochemicals for sustainable agricultural development. Environmental Science. Processes & Impacts, 23(2), 213–239. https://doi.org/10.1039/d0em00404a
- Tsebee, K. A. (2021). Evaluation of communication support materials used for agricultural development projects in selected states in North Central Nigeria. International Journal of Agricultural Extension and Rural Development Studies, 8(4), 1–28.
- Vargo, D., Zhu, L., Benwell, B., & Yan, Z. (2021). Digital technology use during COVID-19 pandemic: A rapid review. Human Behavior and Emerging Technologies, 3(1), 13–24. https://doi.org/10.1002/hbe2.242
- Vuorre, M., Orben, A., & Przybylski, A. K. (2021). There is no evidence that associations between adolescents’ digital technology engagement and mental health problems have increased. Clinical Psychological Science: A Journal of the Association for Psychological Science, 9(5), 823–835. https://doi.org/10.1177/2167702621994549
- Yao, L., Li, Y., & Chen, X. (2021). A robust water-food-land nexus optimization model for sustainable agricultural development in the Yangtze River Basin. Agricultural Water Management, 256(7), 107103. https://doi.org/10.1016/j.agwat.2021.107103
- Yu, B. (2022). The impact of the internet on industrial green productivity: Evidence from China. Technological Forecasting and Social Change, 177, 121527. https://doi.org/10.1016/j.techfore.2022.121527
- Zhao, L., Zhang, Y., Sadiq, M., Hieu, V. M., & Ngo, T. Q. (2023). Testing green fiscal policies for green investment, innovation and green productivity amid the COVID-19 era. Economic Change and Restructuring, 56(5), 2943–2964. https://doi.org/10.1007/s10644-021-09367-z
- Zheng, K., Zhang, Q., Hu, Y., & Wu, B. (2021). Design of fuzzy system-fuzzy neural network-backstepping control for complex robot system. Information Sciences, 546(4), 1230–1255. https://doi.org/10.1016/j.ins.2020.08.110