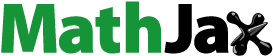
Abstract
Adopting sustainable intensification technologies improves the productivity, incomes, and livelihoods of small farm households; however, their adoption remains disproportionately low. Although soil, climate and vegetative cover are important factors in determining the viability of sustainable intensification technologies in agronomic trials or field experiments, they are rarely considered in socio-economic analyses of smallholder adoption decisions. Using agroecological zones as proxies for similar soil, climate, and vegetation conditions in two agroecological zones in northern Ghana, we examined their effects on smallholder farmers’ decisions to adopt sustainable intensification technologies. We applied a multistage sampling technique to obtain data from 461 small farm households in the Sudan savannah and Guinea savannah agroecological zones. We observed a statistically significant difference in the covariates of adopters and non-adopters of sustainable intensification technologies in terms of age (6.389, p < 0.010), education (0.106, p < 0.010), group membership (0.648, p < 0.010), access to extension services (1.274, p < 0.010) and access to credit (GH¢52.436, p < 0.010). The odds of sustainable intensification technologies adoption depend on age, number of agricultural extension visits, group or farmer-based organisation membership, education and agroecological factors (2.140, p < 0.050). The study provides an opportunity for future research to consider the inclusion of farm-level measurement of agroecological variables in explaining the adoption behaviour of small farm households.
REVIEWing EDITOR:
1. Introduction
Global food demand is rapidly increasing as are agricultural expansion economic and environmental costs (Kopittke et al., Citation2019; van Dijk et al., Citation2021). To meet the food and nutrition requirements of an additional two billion people by 2050 will necessitate an increase in global food production. While it is important to increase the food supply, it is critical to do so in a sustainable manner (Klöffel et al., Citation2022). If the current production practices persist, a significant proportion of land will be lost to agricultural production, with associated increase in greenhouse gas emissions in the form of carbon monoxide and nitrogen (IPCC, Citation2019, Citation2023; Tilman et al., Citation2011). The role of smallholder farmers in ensuring sustainable food system cannot be overstated, especially in developing countries where they produce about 80% of the food supply (FAO, Citation2014).
In the context of agroecology,Footnote1 sustainable intensification technologies (SITs) provide a path to increase food and animal production with minimal social, economic, human, and environmental costs (Altieri, Citation2018). Sustainable agricultural intensification technologies are critical for ensuring the long-term viability of agricultural production systems in various agroecological zones (Bernard & Lux, Citation2017). The justification for the development of SITs is to increase production in a more sustainable manner. Considerable strides have been taken to improve access to sustainable agricultural technology for small farm households, with the backing of international organizations, national governments, and NGOs/CSOs, including the United States Agency for International Development (USAID) and the International Institute for Tropical Agriculture (IITA).
This paper focuses on the role of agroecological differences (in soil, rainfall, temperature, and vegetation cover) in determining small farm households’ adoption of SITs. It also suggests policy implications for a developing country, specifically Ghana. Crop and livestock production takes place in different agroecological zones (AEZs). Within these zones are similar conditions of soil, rainfall, temperature, and vegetation cover. However, these factors or conditions differ across the zones. This means that each agroecological zone is different and would require a tailor-made intervention to address the challenges it faces in agricultural production. Would a unified technology solution for the different zones be beneficial? If not, what are the implications of ignoring these agroecological differences in understanding small farm households’ adoption decisions? The study, therefore, addresses the question: does a farmer’s agroecological zone influence his or her choice of SI technologies?
Significant efforts have been made to improve access to new technologies to smallholder farmers, particularly in sub-Saharan Africa. However, adoption of these technologies remains disproportionately low (Gabriel & Gandorfer, Citation2022; Grabowski et al., Citation2016; Kuyah et al., Citation2021), and the factors influencing adoption decisions remain contested (Arslan et al., Citation2022). Meanwhile there are persistent challenges of low productivity and food insecurity (Takahashi et al., Citation2020). Consequently, smallholders’ technology adoption remains an active area of research, with the scientific community investigating the factors and conditions that contribute to farmers’ behaviour. Recent studies (e.g. Ayantunde et al., Citation2020; Pham et al., Citation2021; Sebatta et al., Citation2019) have explored factors influencing the adoption of sustainable intensification technologies.
Notwithstanding the contribution of these studies, they have focused on the technology attributes, socio-economic and institutional factors such as age, income, education, extension, and social capital factors affecting the adoption of these technologies (also Feyisa, Citation2020; Guo et al., Citation2020; Jones-Garcia & Krishna, Citation2021; Manyanga et al., Citation2023; Ruzzante et al., Citation2021; Smidt & Jokonya, Citation2022). This is because these factors can be easily measured. Although Jinbaani & Wale (Citation2023) incorporated agroecological zones into their model, they did not extend the analysis to the regions within these zones. Agroecological conditions of soil, climate and vegetation conditions are critical in agronomic or field trials of SITs (Michalscheck et al., Citation2018). But because these variables are difficult to measure at the farm level, they are mostly overlooked in the social and applied economics literature when determining the factors influencing smallholder adoption decisions (Anik et al., Citation2023; Jones-Garcia & Krishna, Citation2021). The present study aims to fill this gap by using proxies as the initial step towards highlighting their significance.
Therefore, in this study, we represented the conditions of soil, rainfall, temperature and vegetation cover with northern Ghana’s Sudan and Guinea savannah AEZs to understand how these differences affect sustainable intensification technology (SIT) adoption. This we did because these conditions vary across the two agroecological zones (see Section 2.2). By introducing AEZs, this is a step towards expanding the applied socio-economic literature in SIT adoption, as it is in the field of agronomic trials. The findings of the study should aid in understanding, developing, and disseminating SITs to smallholder farmers across various AEZs. This paper contributes to literature in other ways.
First, it shows that agroecological zones (because of differences in soil, vegetation, temperature, and rainfall patterns across the various zones) play an important role in farmers’ choice of sustainable intensification technologies. Second, it demonstrates that even within the same agroecological zone, regional differences, arising from administrative, socio-economic and culture are critical in determining farmers’ adoption of SITs. Third, it offers insights to policymakers and researchers who develop and disseminate SITs that the elements that affect farmers’ behaviour are intricate and multifaceted. Hence, technologies for small-scale farmers must cater to their specific demands since a one-size-fits-all approach may not be effective in various circumstances. In other words, SITs that may work in one AEZ may not necessarily be adopted in another AEZ due to differences in soil, rainfall, temperature and vegetation conditions.
The next section of the paper describes the study materials, study design and justification for selected variables. Section 3 provides information on concept, empirical estimation strategy, and analysis procedure and limitations. Section 4 presents and discusses the findings of the methodology. Summary statistics and mean differences in covariates between SIT adopters and non-adopters are presented, as are the factors influencing SIT adoption; agroecological conditions, education, age, FBO membership and extension service. The final section contains the conclusion and lessons, which highlight the importance of considering differences that exist across AEZs when delivering SITs to smallholder farmers, including future research directions.
2. Materials
2.1. Study area and project
Northern Ghana is about 143,119 km2 in size, with two major AEZs - Sudan savannah and Guinea savannah (https://eros.usgs.gov/westafrica/ecoregions-and-topography/ecoregions-and-topography-ghana), and a population of about 5,549,000 people (Ghana Statistical Service [GSS], Citation2021). Rainfall is unimodal, with a long dry period. The area is good for growing grains and cereals, as well as tubers (yam). The savannah nature of the area makes animal production prevalent and a source of revenue during the lean season. Furthermore, when crop income is depleted, income from animal sales is used to finance farm operations. The density of the population is lower in northern Ghana than in southern Ghana. Both men and women work in crop and animal production. Farm operations, on the other hand, are highly gendered. Females, for example, are primarily responsible for planting and harvesting, whereas males are responsible for other farm activities such as weeding. Females also work off-farm in industries such as groundnut and shea processing, as well as retail trade. The Sudan savannah covers largely the Upper East and Upper West regions, while the Guinea savannah covers the Northern, North East and savannah regions (see ).
Figure 1. Map of Ghana showing the regions and the agroecological zones.
Source: adapted and modified from Hashmiu et al. (Citation2022).

2.2. Characteristics of the Sudan and Guinea savannah AEZs of northern Ghana
The various AEZs in Ghana have different soil, climate and vegetative cover, which may influence production and practices. The Sudan savannah AEZ has a unimodal rainfall distribution, with an annual total of 956.55 mm and a mean annual temperature of 29.20 °C (Asare-Nuamah & Botchway, Citation2019). The rainy season starts from May to October, followed by a period of drought until the next rainy season. The landscape consists of continuous grassland with scattered shrubs, high soil acidity, low fertility and organic matter content, and coarse-textured, rocky and undulating terrain (Agyin-Birikorang et al., Citation2022; Issahaku et al., Citation2016). Compared to Guinea savannah, the area is highly vulnerable to climate change and desertification.
The Guinea savannah AEZ (Northern Region) receives more rain than the Sudan savannah, with an average annual rainfall of approximately 1079.10 mm although it also has a unimodal rainfall pattern. The rainy season lasts from April to October, with the rest of the year being dry. The annual average temperature is 27 °C (Asare-Nuamah & Botchway, Citation2019). The soils are naturally moderately acidic, low in organic matter, friable and with a thin topsoil that is prone to erosion. Iron concretions can be found a few centimetres below the surface of the soil in some areas (Amoako & Gambiza, Citation2019; Braimoh & Vlek, Citation2004). The major crops cultivated are millet, guinea corn, maize, groundnut, beans and sorghum, and livestock production and poultry production are common in the two AEZs. However, in the Sudan AEZ, dry-season tomato and onion production is more common (Amoako & Gambiza, Citation2019).
2.3. The sampling approach
The data for this study comes from six districts in northern Ghana, where the phase II of the Africa research in sustainable intensification for next generation (Africa RISING) project was implemented between 2017 and 2023. The districts are Tolon-Kumbumgu and Savelugu in the Northern Region, Nadowli-Kaleo and Wa West in the Upper West Region, and Bongo and Kassina-Nankana in the Upper East Region. Some of the communities are Tingoli, Lange, Zanko, Goli, Nyangua and Sambolugo. The data was collected between January and February 2021. Small farm holdings were introduced to SITs as a measure to mitigate the impact of climate change and low productivity. Farming communities in different AEZs in northern Ghana have been taken through the same trial plots of the same technologies in so-called ‘technology parks’ before adopting them on their farms. The project was a collection of technologies or practices in which the International Institute for Tropical Agriculture (IITA) provided technical and material assistance to smallholder farmers in the two AEZs to increase productivity. SITs introduced to farmers included integrated soil management (e.g. row planting and drill fertiliser), conservation agriculture (e.g. improved varieties and cowpea living mulch), crop-livestock integration (e.g. maize leaf stripping and livestock production), and postharvest management (e.g. maize sellers and storage facilities). The farmers received initial input support, such as fertiliser and improved maize seed. We contacted the IITA in Tamale, which informed us about the history of the project and the communities where it was implemented. Prior to conducting the field survey in the communities, we reached out to our previous research district and community networks, specifically agricultural extension agents, community opinion leaders and community-based enumerators.
The data was collected using a multistage sample design. First, six districts in northern Ghana, where the project was implemented, were purposefully selected. The intensity of farming and the adoption of sustainable intensification technologies vary by region and district. Twelve (12) communities were involved in the study - two from each district. Smallholder farm households adopting SITs in the communities were identified with the assistance of the Agricultural Extension Officer, the local politician (locally called Assemblyman) and a community opinion leader. There were several farmers in the communities but most of them were not adopting SITs. In the Savelugu district, simple random sampling was applied to select 95 farmers (50 SIT adopters and 45 non-adopters). Non-adopters were included in the sample because using the logistic regression model requires information from both adoptersFootnote2 and non-adopters of SITs to analyse the factors that determine the adoption. We replicated the approach in the remaining districts. Sixty-three (63) respondents were observed for Tolon District (31 adopters and 32 non-adopters of SI). In Nadowli-Kaleo, 91 respondents were interviewed (48 adopters and 43 non-adopters). In Wa-West, a total of 70 respondents (37 adopters and 33 non-adopters) were covered. A total of 79 (38 adopters and 41 non-adopters) and 63 (33 adopters and 30 non-adopters) respondents were interviewed for the Bongo and Kasina-Nankana districts respectively. A total of 237 adopters of SITs and 224 non-adopters were randomly sampled for the study. Data was collected on socio-economic, farm-level characteristics and institutional characteristics of farmers such as access number of extension visit, age, educational level, membership of a farmer-based organisation, etc. The data was gathered through with a computer-assisted personal interview (CAPI), transferred electronically, and analysed with Stata 16.
shows the variables () used in the analysis, their meaning, means of measurement, minimum and maximum values. There appear to be outliers in some of the variables, such as household size and farm size. However, we have no good reason to drop them, but rather correct for possible heteroscedasticity using robust standard errors.
Table 1. Variables and measurement.
2.4. Justification for inclusion of variables in the model
Climate and soil conditions in each AEZ may influence farmers’ technology choices and/or agricultural production practices (Hussain & Velasco-Munoz, Citation2021; Rahman & Anik, Citation2020). Sudan savannah, for example, has lower and erratic rainfall, poor soil fertility, prolonged drought, and higher high temperatures than Guinea savannah, which may influence farmers’ behavioural decisions, choices, and practices. The AEZs considered in the study- Sudan and Guinea savannahs () could affect the adoption of SITs due to differences in climatic and soil conditions and concentration of agrarian activities. Technology adoption is more likely to occur in a zone with higher agricultural potential but is more vulnerable to climate change and poor soil conditions. Similarly, the specific farmland type could impact the type of technologies adopted by farmers. For instance, farmers whose farms are in the lowlands are unlikely to adopt stone-bonding technology. Soil fertility has been found to drive the adoption decisions of farmers for certain technologies as well as the intensity of adoption (Ayenew et al., Citation2020). Farm location positively influences farmers’ investment decisions (Kassie et al., Citation2013). The study expects a positive of farmland location on adoption.
Also, socio-economic variables influence the adoption of agricultural technologies and in most cases mixed effects have been reported in the literature. Age could influence adoption in both positive and negative ways. For example, Quaye et al. (Citation2021) documented the positive effects of age on modern agricultural technology adoption in the cassava value chain in Ghana. The level of education expected farmers with formal education to adopt sustainable intensification technologies faster than those who did not. Education influences the adoption of improved wheat varieties (Kassie et al., Citation2015), and both positive and negative effects are anticipated, depending on the dynamics in local socio-economic characteristics. Household size is particularly important for SIT technologies that are labour intensive. The positive effects of household size on agricultural technology adoption are well documented (Manda et al., Citation2016) and we anticipate both positive and negative effects in the current study.
Gender was included to determine whether the sex of the farmer informs agricultural technology adoption decisions. The likelihood of modern technology adoption in agriculture increases with females relative to males (Quaye et al., Citation2021). Studies show gender influences SIT adoption decisions differently (see Ndiritu et al., Citation2014; Teklewold et al., Citation2013), and so we anticipate a positive or negative effect based on the technologies under consideration. Belonging to a group, for example a farmer-based organisation (FBO), could influence technology adoption due to peer influence. Quaye et al. (Citation2021) reported a positive effect of FBO on the adoption of rice and maize technologies. Membership in farmers’ associations positively affects the speed of adoption of SITs, as it promotes social learning (Ngango & Hong, Citation2021), hence a positive effect on farmer adoption decisions is anticipated. Agricultural technology adoption is also influenced positively by access to credit (Akudugu et al., Citation2012; Ayenew et al., Citation2020; Ngango & Hong, Citation2021). The amount of credit received by a farmer in the form of a loan is relevant, especially for input-intensive technologies.
Where credit is well applied to agricultural production, farmers are better placed to adopt SITs, hence positive effects are expected. Also, access to extension services impacts positively on technology adoption. The number of extension visits received by a farmer during the 2019 production season was used as a measure. Extension service access facilitates the sharing of technology-related information for adoption by farmers. The quality of extension services delivered impacts positively on technology adoption (Kassie et al., Citation2015; Quaye et al., Citation2021) and the incomes of farmers (Akudugu et al., Citation2012; Anang et al., Citation2020). The study, therefore, anticipated a positive effect of access to agricultural extension services on adoption. Farm size could influence technology adoption, and positive effects have been reported (Akudugu et al., Citation2012; Kassie et al., Citation2013). We anticipate that farm size would have positive effects on technology adoption.
3. Methods
3.1. Modelling framework
In this study, we employed the binary logistic regression model proposed by Amemiya (Citation1981) and subsequently by Maddala (Citation1983) to analyse the factors influencing the adoption of SITs. The model has been used extensively in empirical studies (see Akudugu et al., Citation2009; Jumpah et al., Citation2019; Kuwornu et al., Citation2012; Owusu et al., Citation2011). The logistic regression model is adopted because the ‘regressand’ (decision to adopt or not to adopt SITs) is dichotomous, which is a qualitative response variable taking the values of 1 and 0, which is observable. Logistic regression provides a consistent and efficient estimator to analyse the effect of a group of regressors on a dichotomous variable by estimating each independent variable’s unique influence (Stoltzfus, Citation2011). The decision to adopt SITs is based on the knowledge of the existence of the technology.
Assuming a farmer, i is risk-neutral and maximises expected utility which is the result of making choices from
= number of choices available. The utility function is then expressed in EquationEquation 1
(1)
(1) .
(1)
(1)
= vector of relevant deterministic variables; α = the parameters of the deterministic variables to be estimated; ε = the error term, which is assumed to be identical and independently distributed, [
, with a constant variance, i.e.
(2)
(2)
Our data did not satisfy EquationEquation 2(2)
(2) , so we accepted
and reject
. In the circumstance, White (Citation1980), proposed the robust standard error, which corrects for the heteroscedasticity in the error term, expressed in EquationEquation 3
(3)
(3) .
(3)
(3)
Y = 1, if a farmer chooses to adopt sustainable intensification technology (that is the utility of choosing to adopt SITs is greater than choosing another option), otherwise = 0. The utility of choosing to adopt SITs is greater than choosing another option is expressed as:
)
P, the probability, which is a decision to adopt SITs or not is determined by the deterministic or response variables. F (the function) could take the form of a logistic, normal, or probability function. The estimation of P requires the use of the logistic cumulative distributive function for the logistic regression model. The P ( is expressed in EquationEquation 4
(4)
(4) .
(4)
(4)
(5)
(5)
= probability, with individual observations
The probability model, EquationEquation 6(6)
(6) , is the regression of the conditional probability expectation of Y on X.
(6)
(6)
Because the model is not linear, the coefficients are not essentially the marginal values of the different deterministic variables. To obtain the relative effects of the various deterministic variables on the adoption of SITs, EquationEquation 6(6)
(6) should be maximised with respect
(Greene, Citation2018). This results in EquationEquation 7
(7)
(7) .
(7)
(7)
For estimation purposes, the logit model is as specified in EquationEquation 8(8)
(8) .
(8)
(8)
where
is the logistic function.
3.2. Empirical estimation procedure
The agroecological conditions and socio-economic characteristics of the farmer influence the decision to use SITs, and those used in the empirical estimation equation are outlined in EquationEquation 9(9)
(9) . These variables were selected based on the previous research as discussed earlier, and knowledge of the study area. We included as many regressors and their interactions as possible during the modelling process. To avoid inconsistent parameters and reduced generalizability beyond the current data, some policy-relevant variables that were not statistically significant were dropped (Hosmer & Lemeshow, Citation2000; Shipe et al., Citation2019; Stoltzfus, Citation2011; Tabachnick, Citation2023). The decision to adopt SITs is modelled in EquationEquation 9
(9)
(9) .
(9)
(9)
(Refer to for to
definition.)
(sustainable intensification technologies adoption).
3.3. Limitations and direction for further study
The study has limitations because it did not directly measure the specific conditions of the soil, rainfall, temperature, and vegetation cover on each farm. Instead, proxies based on agrological zones of the farmer were used. Although measuring directly would have improved the study, it would have required considerable time and financial resources to do so. Nevertheless, the proxies reflect the similar conditions of soil, temperature, rainfall, and vegetation cover within agroecological zones. We anticipate that these factors will influence how farmers within a given agroecological adopt new technologies. The current study does not capture details of each of the technologies disseminated. Rather, it specifies is an adopter in order to collect data. We also acknowledged that soil conditions might differ slightly within the same agroecological zone. This is the reason why we have extended our analysis to cover the regions. A study that includes farm-level measurement and observation would be an improvement of the current study. Further study is also needed to understand why Upper West and East regions are in the same agroecological zone but differ in adoption rates.
4. Results and discussions
4.1. Descriptive statistics and test of means
The results of the descriptive statistics are reported in . The maximum credit received by non-adopters (GHȼ2000.00) is higher than the adopters (GHȼ1800.00). Also, the adopters appear to be relatively older than the non-adopters. The maximum age for adopters is 82 and 78 for non-adopters. In addition, the minimum age for non-adopters is 18 and that for adopters is 20. The adoption of SITs in the study area is likely to raise the cost of production. This is because the various technologies farmers are exposed to are likely to increase the cost of production. For example, farmers are required to plant in rows for ease of other farm operations and to improve productivity. This, however, is slow and labour-demanding, which implies farmers need to hire extra labour if they are to keep up with the rainy season and the planting time.
Table 2. Summary statistics and test of means of the respondents.
The means of the variables, the differences and the pooled mean are reported in . Except for farm location, farm size, marital status, household size and gender, all the differences in means for the covariates are statistically significant.
Farm size and household size are likely to be homogeneous, and thus there is no statistical significance in the difference in means because these are smallholder farmers living in the same communities, and similarities in farm and household sizes are likely to exist among them.
About 98% of respondents suggested that both males and females have an equal chance of adopting SITs, a plausible reason for the statistically not significant difference in mean for gender. Age, education, credit, extension, hired labour cost, on-farm income, group membership and regional location, on the other hand, have all shown statistically significant differences in means. Adopters are slightly older (49.630 years) than non-adopters (43.240 years). Adopters are 6.390 years older than non-adopters on average, which is statistically significant at 1%. It appears that the older the farmer, the more likely he or she is to adopt SITs. This could be due to the farmer’s years of experience, which could aid him in implementing SITs. More non-adopters are educated (26.230%) than adopters (15.620%). Non-adopters and adopters spend an average of 2.690 years and 1.560 years at school respectively. Adopters, on the other hand, are more likely to be in a group or a member of FBO (93.67%) than non-adopters (28.880%) (). This could be due to peer pressure and the opportunity to learn from one another in groups.
Farmers who choose SITs are expected to use a specific amount of fertilizer to achieve the best yield, which requires the use of monetary resources that rural farmers do not have. Adopters may require more funds for the activities they intend to undertake; it is worth noting that adopters received more credit than non-adopters. shows adopters received more credit (GHȼ112.25) than non-adopters (GHȼ59.822), with a mean difference of GHȼ52.436, which is also statistically significant at 1%. A similar pattern is observed in the number of extension visits, indicating that adopters had greater access to agricultural extension than non-adopters (). Access to extension services is critical for the adoption of SITs because farmers must be exposed to the technologies by scientists or agricultural extension agents. And, without this, such knowledge would be difficult to obtain.
4.2. Determinants of SIT adoption
Results of two logistic regressions are reported in . First, we analysed the data based on the two agroecological zones (refer to Appendix A). We then proceeded to disaggregate the analysis by the regions. The discussions are done on the disaggregated results. The results of logistic regression, Model 2 () show 458 respondents (adopters and non-adopters of SITs), a Chi2 of 0.000 and Pseudo R2 (0.543) from the analysis. The probability > Chi2or p-value of 0.000 suggests that the model is asymptomatically significant at 99%, whereas the Pseudo R2 value indicates that the independent variables explain () approximately 54.3% of the variations in the dependent variable (adoption of SITs).
Table 3. Results of logistic regression.
4.2.1. Age
The age of an individual () is a critical factor in determining the decision the person would take at a given point in time. As explained by Spicka (Citation2020) and (Adams et al., Citation2021), an individual is more risk-seeking when he or she is young but becomes more risk-averse in later years and, therefore, the choices of individuals may be contingent on age. Likewise, farmers are likely to be risk-loving (adopting new technologies) in the production and marketing of farm outputs while young and the reverse would be the case while old. The result () shows that the age of the farmers relates positively to the adoption of SITs. Analysis of the data shows that age had a 1.150 parameter, and it is significant at 10% (p-0.030). The implication is that an increase in age by a year increases the odds of adopting SITs by 1.150. As noted earlier, the average age of the farmers in the study areas is 46.517 years, whereas the national average is 54 years. Relatively, farmers in the study areas are younger (compared to the national average) and, therefore, the tendency to adopt SITs would improve with increasing age. In addition, as the farmer’s age increases, he or she may gain more experience and thus be more willing to adopt SITs. However, because age is a trend variable, it is not expected that the odds of adopting SITs would continue to increase as the farmer grows. There would come a point when the desire and energy to adopt SITs would wane, and thus the likelihood of SITs adoption would begin to decline. It is, therefore, critical to initiate projects and programmes for farmers while they are still young, so that they can benefit from them while they are still young. Like this study, Guo et al. (Citation2020) also found that age has a positive relationship with the adoption of technology when they analysed the determinants of the adoption of SITs in southern African farming systems, using a meta-analysis.
4.2.2. Educational level
The result () shows that education is negatively related to the adoption of SITs with a −2.273 odd ratio. It is statistically significant at 5% (p < 0.050) which is a p-value of 0.033. The implication is that as the number of years spent at school () l increases, the odds of adoption of SITs decrease by 2.273. This could be because as the educational levels of people rise, so will their opportunities for lucrative jobs and, as a result, such farmers will be unwilling to adopt SITs or will opt out of farming entirely. Furthermore, higher levels of education expose people to a broader range of information. Farmers with more knowledge may be less likely to adopt SITs because they are more familiar with technologies that less educated farmers are not. Jumpah et al. (Citation2019) discovered a positive relationship between educational level and participation in agricultural-related credit programmes in their research. Jabbar et al. (Citation2020) found statistically significant and positive associations between SITs (tillage and intercropping) adoption and education, but not with organic manure use, improved seeds, or crop rotation. Guo et al. (Citation2020) also observed a negative but statistically not significant relation between the adoption of SITs and educational level. Variations in studies like these show that research results are dependent on several factors: time of the research, type of research, location, the type of respondents and the volume of data, among others.
4.2.3. Membership of a group/FBO
The result () shows a coefficient of 39.420 and a p-value of 0.000, meaning that the variable, membership of group or FBO () is statistically significant at 1%. The relatively high coefficient of FBO may be due to outliers in the data, or it may be perfectly predicting adoption. The implication is that a farmer, being part of an FBO/group, increases his or her likelihood of adopting SITs almost forty-fold. The result appears to support the notion that development organisations are more comfortable with supporting farmers in groups than on an individual basis. This could be because transferring technologies to farmers in groups is more cost-effective than doing so individually. Group members are also likely to assist one another with knowledge-sharing, especially after the project is completed and there are challenges ahead. Although being part of a group increases the odds of adopting sustainable intensification technologies, this may pose a challenge if community members self-select who should be part of the group to the detriment of other members of the community. Like this finding, Guo et al. (Citation2020) also observed that being a member of a group increases the likelihood of adopting SITs. Pretty et al. (Citation2011) and Bizikova et al. (Citation2020) highlighted the role of building trust among groups and organisations their importance in scaling up SI technologies.
4.2.4. Extension services
The number of extension visits (), which measures the number of times each farmer has been visited by an agricultural extension agent (either a private extension or public extension). The result in shows an odd ratio of 1.373 for access to agricultural extension service and it is statistically significant at 5% (p < 0.010). The implication is that a unit increase in agricultural extension service visits increases the odds of adoption of SITs by 1.373. Ranasinghe et al. (Citation2023) found a similar result in Bangladesh. Access to agricultural extension services is very critical to the transfer of technologies and agricultural production practices. Mostly, smallholder farmers in rural areas rely on traditional methods of farming not because they so wish but because there is little or no access to agricultural extension services. Smallholder farmers typically revert to traditional production systems in response to shocks from new innovations or technologies in the absence of adequate extension supervision. It is, therefore, noteworthy to observe from the analysis that the number of extension visits improves the odds of adopting SITs. In this regard, it is essential for duty bearers and other relevant stakeholders to ensure that smallholder farmers have access to agricultural extension services through AEA or any other means possible.
The farm location, household size, gender, amount of credit received for the cropping season, farm size, cost of hired labour and on-farm income are statistically not significant (p > 0.100). The location of the farm (upland or lowland) was dummied. The odd ratio of 0.826 suggests that given the farm is located on the upland, the odds of adopting SITs improve by 0.826. Household size also relates positively to the adoption of SITs, having a coefficient of 0.993 (). This implies that an increase in the household size by a person reduces the increase of adoption of SITs by 0.993. This is typical of rural areas in northern Ghana where agricultural production is manpower-driven. Like farm location, gender was also dummied. The result suggests that given that the farmer is a female, the odds of adoption of SITs increase by 1.250. The amount of credit received for the cropping season, on-farm income, and farm size all follow a similar pattern. Although gender, household size, and access to credit are statistically not significant in our findings, studies like Theriault et al. (Citation2017), Guo et al. (Citation2020) and Jabbar et al. (Citation2020) found statistically significant results, though the magnitudes of the coefficients differed between studies.
4.2.5. Agroecological zones
The study considers the Sudan and Guinea savannah agroecological zones (AEZs) of northern Ghana and finds a statistically significant effect on the adoption of SITs for the Sudan savannah (see Appendix A). We also extended the analysis into the regions to observe that the odds of adoption were still significant for the regions in the Sudan savannah, but with varying odds of adoption. As noted above, the Sudan savannah is characterised by prolonged drought, poor soil structure and vegetation, and high levels of poverty and illiteracy, making the zone more vulnerable to the effects of climate change. Variations in agroecological conditions appear to influence the choice of production systems. The Sudan savannah AEZ (Upper East and Upper West) has a statistically significant positive effect on SITs adoption. The Upper West Region () has a coefficient of 2.140, the Upper East Region (
) has a coefficient of 1.612 and the Northern Region (
) has a coefficient of –2.770. (p-0.834). The results for the Upper West and East regions are statistically significant (at 5%), but not for the Northern Region (p > 0.100). The adoption of SITs increased by 2.414 and 1.612 in the Upper West and Upper East regions respectively, while it decreased by 2.770 in the Northern Region. Smallholders in the Sudan savannah are more likely to adopt SITs than those in the Guinea savannah. The implication of the finding is that the adoption of SITs is likely to be higher in AEZs with unfavourable soil, climate, and vegetation for agricultural production. For example, the adoption of SITs is significant and higher in the Sudan AEZ but not in the Guinea savannah. The Sudan AEZ has higher temperatures, low humidity, low and short rainfall periods, and a long dry season. It also has poor vegetation cover relative Guinea savannah. In such conditions, farmers are more likely to adopt SITs such as shorter-maturing varieties, even if these varieties increase production costs. This enables them to produce within the short rainy season. Farmers in such AEZs are also more likely to use mulch and compost, which reduce water evaporation and improve soil structure respectively. In fact, the soil in the Sudan AEZ has little or no humus, and the high temperature in the same area leads to high soil water evaporation.
Maize leaf stripping, one of the SITs, allows farmers to strip leaves from the crop to feed animals without affecting the crop. This technology appears to be helpful for farmers in the Sudan AEZ, where livestock production is high due to large flat fields, but vegetation cover is poor and there are long dry spells. The use of these SITs also appears to support the concept of circular farming, where farmers use animal manure and crop residues to fertilise the soil, and in turn use plant by-products and leaves to feed livestock. This ensures efficient use of local resources in areas with unfavourable production conditions and limited production resources. Further, the Sudan savannah is more agrarian and less developed. Sudan savannah is more vulnerable to the effects of climate change, such as desertification and rainfall variability than the Guinea savannah (Baffour-Ata et al., Citation2021; Kermah et al., Citation2017). This could explain why SIT adoption is positive and statistically significant in Sudan savannah but not in Guinea savannah. It is possible that people or farmers living in relatively developed areas will be less enthusiastic about agricultural projects. SIT projects need to target poor rural farmers and AEZs that are more vulnerable to climate change. Guo et al. (Citation2020) found a statistically significant relationship between the location of farmers who practised tillage and intercropping and the adoption of sustainable intensification technologies. However, while Guo et al. (Citation2020) found a positive relationship, our study found mixed results across zones.
In summary, the Sudan savannah AEZ has poorer soil conditions, higher temperatures, less rainfall, and less vegetative cover than the Guinea savannah AEZ, making the adoption of SITs there more likely.
5. Conclusions and policy implication
The use of sustainable intensification technologies (SITs) is seen as a method of using increasingly scarce natural resources such as land and water to increase crop and livestock productivity while minimising economic, social, human, and environmental costs. In this context, SITs have been promoted by policymakers and the scientific community over the past two decades. When it comes to promoting SITs at the farm household level, Ghana is no exception. However, the factors influencing the decisions of farm households to adopt these technologies or practices, particularly in the agroecological dimension, are largely unexplored. As a result, the current study investigated the factors that influence the use of SITs, taking agroecological differences into account for policy recommendations. Using the multistage sampling procedure and logistic regression model, the study finds that farmers’ educational levels, membership in a group or farmer-based organisation, age and differences in agroecological conditions, all have statistically significant effects on the adoption of SITs. While age, access to agricultural extension services, membership in farmer-based organisations and agroecological variations all have a positive impact on the adoption of SITs, educational level has the opposite effect.
Agroecological conditions such as soil, climate and vegetation cover, like agronomic trials or field experiments, are important in small farm households’ decision to adopt a given technology, according to our research. So, small farm households in agroecological zones with high soil acidity, low and short rainfall duration, high temperatures, and poor vegetation cover are more likely to use SITs. Policymakers and scientists who advocate the development and dissemination of SITs must consider this. We propose interventions by stakeholders, particularly government and NGOs, to improve the delivery of extension services and education using digital technologies to reach as many people as possible, as well as to promote group formation for peer learning, information sharing, and networking while taking agroecological dynamics into account when delivering new technologies to farmers. Finally, adoption of SITs is contingent on the availability of financial resources as well as other ancillary services such as timely and cost-effective input supply, both of which must be addressed.
Public interest statement.docx
Download MS Word (12.7 KB)Acknowledgements
We are grateful to two anonymous reviewers whose comments helped improve this paper. And to Africa RISING Ghana Project, funded by USAID under grant# AID-BFS-G-11-00002. Through the project data used for the analysis was collected.
Disclosure statement
No potential conflict of interest was reported by the author(s).
Data availability statement
Data for the analysis are available from the corresponding author and may be provided on reasonable request.
Additional information
Notes on contributors
Emmanuel Tetteh Jumpah
Emmanuel Tetteh Jumpah is a doctoral student in the Department of Economics and Development, Faculty of Tropical AgriSciences, Czech University of Life Sciences, Prague (FTZ-CZU). He is also a researcher with the Council for Scientific and Industrial Research-Science and Technology Policy Research Institute, Ghana. With over 10 years of experience in research and development. Abdulai Adams is a Senior Lecturer in the Department of Economics at SD Dombo University of Business and Integrated Development University, Ghana. He possesses over 20 years of experience in research, lecturing, and international development programme management. He serves as a co-supervisor to Emmanuel Tetteh Jumpah. Tomas Ratinger is an Associate Professor in Econometrics in the Department of Economics and Development at FTZ-CZU and a Senior Researcher at the Technology Centre, Prague. With over 30 years of experience in lecturing, EU project management, and student supervision, he acts as the doctoral supervisor of Emmanuel Tetteh Jumpah. Bernard Kwamena Cobbina Essel is a doctoral student in the Department of Economics and Development at FTZ-CZU. Forzia Ibrahim is a doctoral student in the Faculty of Forestry and Wood Sciences at CZU. This research is the result of collaboration among the Department of Economics at FTZ-CZU, SD Dombo University of Business and Integrated Development Studies, and the Faculty of Forestry and Wood Sciences at CZU.
Notes
1 The term agroecology has been broadened and defined in many ways (Altieri, Citation2018). But, in this study, it is used to mainly refer to conditions of soil, climate and vegetative cover as outlined in Section 2.2.
2 Adopters, as used in this study, refer to smallholder maize and cowpea farmers who chose to participate in the Africa RISING project and were implementing at least three of the proposed technologies at the time of the data collection.
References
- Adams, A., Jumpah, E. T., & Caesar, L. D. (2021). The nexuses between technology adoption and socioeconomic changes among farmers in Ghana. Technological Forecasting and Social Change, 173, 1. https://doi.org/10.1016/j.techfore.2021.121133
- Agyin-Birikorang, S., Tindjina, I., Adu-Gyamfi, R., Fugice, J., Jr, Dauda, H. W., Angzenaa, A. B., & Sanabria, J. (2022). Optimizing fertilizer use efficiency for sustainable maize production in strongly acid soils of the Sudan Savanna agroecological zone of Northern Ghana. Journal of Plant Nutrition, 45(17), 2578–15. https://doi.org/10.1080/01904167.2022.2064291
- Altieri, A. M. (2018). Agroecology: The science of sustainable agriculture. CRC Press, Taylor and Francis Group.
- Akudugu, M. A., Guo, E., & Dadzie, S. K. (2012). Adoption of modern agricultural production technologies by farm households in Ghana: What factors influence their decisions? Journal of Biology, Agriculture and Healthcare, 2(3), 1–13.
- Akudugu, M. A., Egyir, I. S., & Mensah-Bonsu, A. (2009). Women farmers’ access to credit from rural banks in Ghana. Agricultural Finance Review, 69(3), 284–299. https://doi.org/10.1108/00021460911002671
- Amemiya, T. (1981). Qualitative response model: A survey. Journal of Economic Literature, 19, 1483–1536.
- Amoako, E. E., & Gambiza, J. (2019). Effects of anthropogenic fires on some soil properties and the implications of fire frequency for the Guinea savanna ecological zone, Ghana. Scientific African, 6, e00201. https://doi.org/10.1016/j.sciaf.2019.e00201
- Anang, B. T., Bäckman, S., & Sipiläinen, T. (2020). Adoption and income effects of agricultural extension in northern Ghana. Scientific African, 7, e00219. https://doi.org/10.1016/j.sciaf.2019.e00219
- Anik, A. R., Eory, V., Begho, T., & Rahman, M. M. (2023). Determinants of nitrogen use efficiency and gaseous emissions assessed from farm survey: A case of wheat in Bangladesh. Agricultural Systems, 206, 103617. https://doi.org/10.1016/j.agsy.2023.103617
- Arslan, A., Floress, K., Lamanna, C., Lipper, L., & Rosenstock, T. S. (2022). A meta-analysis of the adoption of agricultural technology in Sub-Saharan Africa. PLOS Sustainability and Transformation, 1(7), e0000018. https://doi.org/10.1371/journal.pstr.0000018
- Asare-Nuamah, P., & Botchway, E. (2019). Understanding climate variability and change: Analysis of temperature and rainfall across agroecological zones in Ghana. Heliyon, 5(10), e02654. https://doi.org/10.1016/j.heliyon.2019.e02654
- Ayenew, W., Lakew, T., & Kristos, E. H. (2020). Agricultural technology adoption and its impact on smallholder farmers’ welfare in Ethiopia. African Journal of Agricultural Research, 15(3), 431–445.
- Ayantunde, A. A., Oluwatosin, B. O., Yameogo, V., & van Wijk, M. (2020). Perceived benefits, constraints and determinants of sustainable intensification of mixed crop and livestock systems in the Sahelian zone of Burkina Faso. International Journal of Agricultural Sustainability, 18(1), 84–98. https://doi.org/10.1080/14735903.2019.1698494
- Baffour-Ata, F., Antwi-Agyei, P., Nkiaka, E., Dougill, A. J., Anning, A. K., & Kwakye, S. O. (2021). Effect of climate variability on yields of selected staple food crops in northern Ghana. Journal of Agriculture and Food Research, 6, 100205. https://doi.org/10.1016/j.jafr.2021.100205
- Bernard, B., & Lux, A. (2017). How to feed the world sustainably: An overview of the discourse on agroecology and sustainable intensification. Regional Environmental Change, 17(5), 1279–1290. https://doi.org/10.1007/s10113-016-1027-y
- Bizikova, L., Nkonya, E., Minah, M., Hanisch, M., Turaga, R. M. R., Speranza, C. I., Karthikeyan, M., Tang, L., Ghezzi-Kopel, K., Kelly, J., Celestin, A. C., & Timmers, B. (2020). A scoping review of the contributions of farmers’ organizations to smallholder agriculture. Nature Food, 1(10), 620–630. https://doi.org/10.1038/s43016-020-00164-x
- Braimoh, A. K., & Vlek, P. L. G. (2004). The impact of land‐cover change on soil properties in northern Ghana. Land Degradation & Development, 15(1), 65–74. https://doi.org/10.1002/ldr.590
- FAO. (2014). The state of food and agriculture 2014: Innovation in family farming. Food and Agriculture Organization of the United Nations.
- Feyisa, B. W. (2020). Determinants of agricultural technology adoption in Ethiopia: A meta-analysis. Cogent Food & Agriculture, 6(1), 1855817. https://doi.org/10.1080/23311932.2020.1855817
- Gabriel, A., & Gandorfer, M. (2022). Adoption of digital technologies in agriculture—An inventory in a European small-scale farming region. Precision Agriculture, 24(1), 68–91. https://doi.org/10.1007/s11119-022-09931-1
- Greene, W. H. (2018). Econometric analysis (8th Ed.). Pearson Education Inc.
- Guo, Q., Ola, O., & Benjamin, E. O. (2020). Determinants of the adoption of sustainable intensification in southern African farming systems: A meta-analysis. Sustainability, 12(8), 3276. https://doi.org/10.3390/su12083276
- Ghana Statistical Service. (2021). Population and housing census. GSS.
- Hussain, C. M., & Velasco-Munoz, J. F. (Eds.). (2021). Sustainable resource management: Modern approaches and contexts. Elsevier.
- Hosmer, D. W., & Lemeshow, S. (2000). Applied logistic regression. Wiley.
- IPCC. (2023). Summary for policymakers. In Climate change 2023: Synthesis report. Contribution of Working Groups I, II and III to the sixth assessment report of the IPCC (pp. 1–34). IPCC. https://doi.org/10.59327/IPCC/AR6-9789291691647.001
- IPCC. (2019). Climate change and land: an IPCC special report on climate change, desertification, land degradation, sustainable land management, food security, and greenhouse gas fluxes in terrestrial ecosystems [P. R. Shukla, J. Skea, E. Calvo Buendia, V. Masson-Delmotte, H.-O. Pörtner, D. C. Roberts, P. Zhai, R. Slade, S. Connors, R. van Diemen, M. Ferrat, E. Haughey, S. Luz, S. Neogi, M. Pathak, J. Petzold, J. Portugal Pereira, P. Vyas, E. Huntley, K. Kissick, M. Belkacemi, J. Malley, (Eds.)]. IPCC. In press.
- Grabowski, P. P., Kerr, J. M., Haggblade, S., & Kabwe, S. (2016). Determinants of adoption and disadoption of minimum tillage by cotton farmers in eastern Zambia. Agriculture, Ecosystems & Environment, 231, 54–67. https://doi.org/10.1016/j.agee.2016.06.027
- Hashmiu, I., Agbenyega, O., & Dawoe, E. (2022). Cash crops and food security: Evidence from smallholder cocoa and cashew farmers in Ghana. Agriculture & Food Security, 11(1), 12. https://doi.org/10.1186/s40066-022-00355-8
- Issahaku, A. R., Campion, B. B., & Edziyie, R. (2016). Rainfall and temperature changes and variability in the Upper East Region of Ghana. Earth and Space Science, 3(8), 284–294. https://doi.org/10.1002/2016EA000161
- Jabbar, A., Wu, Q., Peng, J., Zhang, J., Imran, A., & Yao, L. 1. (2020). Synergies and determinants of sustainable intensification practices in Pakistani Agriculture. Land, 9(4), 110. https://doi.org/10.3390/land9040110
- Jinbaani, A. N., & Wale, E. (2023). How does participation in Ghana’s fertilizer subsidy program (GFSP) affect the adoption of sustainable intensification practices (SIPs) and gross farm inputs? Development Studies Research, 10(1), 2180047. https://doi.org/10.1080/21665095.2023.2180047
- Jones-Garcia, E., & Krishna, V. V. (2021). Farmer adoption of sustainable intensification technologies in the maize systems of the Global South. A review. Agronomy for Sustainable Development, 41(1), 8. https://doi.org/10.1007/s13593-020-00658-9
- Jumpah, E. T., Osei-Asare, Y., & Tetteh, E. K. (2019). Do farmer and credit specific characteristics matter in microfinance programmes’ participation? Evidence from smallholder farmers in Ada West and East districts. Agricultural Finance Review, 79(3), 353–370. https://doi.org/10.1108/AFR-05-2018-0044
- Kassie, M., Teklewold, H., Jaleta, M., Marenya, P., & Erenstein, O. (2015). Understanding the adoption of a portfolio of sustainable intensification practices in eastern and southern Africa. Land Use Policy, 42, 400–411. https://doi.org/10.1016/j.landusepol.2014.08.016
- Kassie, M., Jaleta, M., Shiferaw, B., Mmbando, F., & Mekuria, M. (2013). Adoption of interrelated sustainable agricultural practices in smallholder systems: Evidence from rural Tanzania. Technological Forecasting and Social Change, 80(3), 525–540. https://doi.org/10.1016/j.techfore.2012.08.007
- Kermah, M., Franke, A. C., Adjei-Nsiah, S., Ahiabor, B. D. K., Abaidoo, R. C., & Giller, K. E. (2017). Maize-grain legume intercropping for enhanced resource use efficiency and crop productivity in the Guinea savanna of northern Ghana. Field Crops Research, 213, 38–50. https://doi.org/10.1016/j.fcr.2017.07.008
- Klöffel, T., Young, E. H., Borchard, N., Vallotton, J. D., Nurmi, E., Shurpali, N. J., Urbano Tenorio, F., Liu, X., Young, G. H. F., & Unc, A. (2022). The challenges fraught opportunity of agriculture expansion into boreal and Arctic regions. Agricultural Systems, 203, 103507. https://doi.org/10.1016/j.agsy.2022.103507
- Kopittke, P. M., Menzies, N. W., Wang, P., McKenna, B. A., & Lombi, E. (2019). Soil and the intensification of agriculture for global food security. Environment International, 132, 105078. https://doi.org/10.1016/j.envint.2019.105078
- Kuwornu, J. K. M., Ohene-Ntow, I. D., & Asuming-Brempong, S. (2012). Agricultural credit allocation and constraint analyses of selected maize farmers in Ghana. British Journal of Economics, Management & Trade, 2(4), 353–374. https://doi.org/10.9734/BJEMT/2012/2270
- Kuyah, S., Sileshi, G. W., Nkurunziza, L., Chirinda, N., Ndayisaba, P. C., Dimobe, K., & Öborn, I. (2021). Innovative agronomic practices for sustainable intensification in sub-Saharan Africa. A review. Agronomy for Sustainable Development, 41(2), 1–21. https://doi.org/10.1007/s13593-021-00673-4
- Maddala, G. S. (1983). Limited dependent and qualitative variables in econometrics. Cambridge University Press.
- Manda, J., Alene, A. D., Gardebroek, C., Kassie, M., & Tembo, G. (2016). Adoption and impacts of sustainable agricultural practices on maize yields and incomes: Evidence from rural Tanzania. Journal of Agricultural Economics, 67(1), 130–153. https://doi.org/10.1111/1477-9552.12127
- Manyanga, M., Pedzisa, T., & Hanyani-Mlambo, B. (2023). Adoption of agroecological intensification practices in Southern Africa: A scientific review. Cogent Food & Agriculture, 9(1), 2261838. https://doi.org/10.1080/23311932.2023.2261838
- Michalscheck, M., Groot, J. C., Kotu, B., Hoeschle-Zeledon, I., Kuivanen, K., Descheemaeker, K., & Tittonell, P. (2018). Model results versus farmer realities. Operationalizing diversity within and among smallholder farm systems for a nuanced impact assessment of technology packages. Agricultural Systems, 162, 164–178. https://doi.org/10.1016/j.agsy.2018.01.028
- Ndiritu, S. W., Kassie, M., & Shiferaw, B. (2014). Are there systematic gender differences in the adoption of sustainable agricultural intensification practices? Evidence from Kenya. Food Policy. 49, 117–127. https://doi.org/10.1016/j.foodpol.2014.06.010
- Ngango, J., & Hong, S. (2021). Speed of adoption of intensive agricultural practices in Rwanda: A duration analysis. Agrekon, 60(1), 43–56. https://doi.org/10.1080/03031853.2021.1883448
- Owusu, V., Abdulai, A., & Abdul-Rahman, S. (2011). Non-farm work and food security among farm households in northern Ghana. Food Policy. 36(2), 108–118. https://doi.org/10.1016/j.foodpol.2010.09.002
- Pham, H. G., Chuah, S. H., & Feeny, S. (2021). Factors affecting the adoption of sustainable agricultural practices: Findings from panel data for Vietnam. Ecological Economics, 184, 107000. https://doi.org/10.1016/j.ecolecon.2021.107000
- Pretty, J. N., Toulmin, C., & Williams, S. B. (2011). Sustainable intensification in African agriculture. International Journal of Agricultural Sustainability, 9(1), 5–24. https://doi.org/10.3763/ijas.2010.0583
- Quaye, W., Onumah, J. A., Boimah, M., & Mohammed, A. (2021). Gender dimension of technology adoption: The case of technologies transferred in Ghana. Development in Practice, 32(4), 434–447. https://doi.org/10.1080/09614524.2021.2000588
- Ruzzante, S., Labarta, R., & Bilton, A. (2021). Adoption of agricultural technology in the developing world: A meta-analysis of the empirical literature. World Development, 146, 105599. https://doi.org/10.1016/j.worlddev.2021.105599
- Rahman, S., & Anik, A. R. (2020). Productivity and efficiency impact of climate change and agroecology on Bangladesh agriculture. Land Use Policy, 94, 104507. https://doi.org/10.1016/j.landusepol.2020.104507
- Ranasinghe, R. D. N. K., Korale-Gedara, P. M., & Weerasooriya, S. A. (2023). Climate change adaptation and adaptive capacities of dairy farmers: Evidence from village tank cascade systems in Sri Lanka. Agricultural Systems, 206, 103609. https://doi.org/10.1016/j.agsy.2023.103609
- Sebatta, C., Mugisha, J., Bagamba, F., Nuppenau, E. A., Domptail, S. E., Kowalski, B., Hoeher, M., Ijala, A. R., & Karungi, J. (2019). Pathways to sustainable intensification of the coffee-banana agroecosystems in the Mt. Elgon region. Cogent Food & Agriculture, 5(1), 1611051. https://doi.org/10.1080/23311932.2019.1611051
- Shipe, M. E., Deppen, S. A., Farjah, F., & Grogan, E. L. (2019). Developing prediction models for clinical use using logistic regression: an overview. Journal of Thoracic Disease, 11(Suppl 4), S574–S584. https://doi.org/10.21037/jtd.2019.01.25
- Smidt, H. J., & Jokonya, O. (2022). Factors affecting digital technology adoption by small-scale farmers in agriculture value chains (AVCs) in South Africa. Information Technology for Development, 28(3), 558–584. https://doi.org/10.1080/02681102.2021.1975256
- Spicka, J. (2020). Socio-demographic drivers of the risk-taking propensity of micro farmers: Evidence from the Czech Republic. Journal of Entrepreneurship in Emerging Economies, 12(4), 569–590. https://doi.org/10.1108/JEEE-09-2019-0143
- Stoltzfus, J. C. (2011). Logistic regression: A brief primer. Academic Emergency Medicine, 18(10), 1099–1104. https://doi.org/10.1111/j.1553-2712.2011.01185.x
- Tabachnick, B. G. (2023). Using multivariate statistics. Pearson India.
- Takahashi, K., Muraoka, R., & Otsuka, K. (2020). Technology adoption, impact, and extension in developing countries’ agriculture: A review of the recent literature. Agricultural Economics, 51(1), 31–45. https://doi.org/10.1111/agec.12539
- Teklewold, H., Kassie, M., & Shiferaw, B. (2013). Adoption of multiple sustainable agricultural practices in rural Ethiopia. Journal of Agricultural Economics, 64(3), 597–623.
- Theriault, V., Smale, M., & Haider, H. (2017). How does gender affect sustainable intensification of cereal production in the West African Sahel? Evidence from Burkina Faso. World Development, 92, 177–191. https://doi.org/10.1016/j.worlddev.2016.12.003
- Tilman, D., Balzer, C., Hill, J., & Befort, B. L. (2011). Global food demand and the sustainable intensification of agriculture. Proceedings of the National Academy of Sciences of the United States of America, 108(50), 20260–20264. https://doi.org/10.1073/pnas.1116437108
- Van Dijk, M., Morley, T., Rau, M. L., & Saghai, Y. (2021). A meta-analysis of projected global food demand and population at risk of hunger for the period 2010–2050. Nature Food, 2(7), 494–501. https://doi.org/10.1038/s43016-021-00322-9
- White, H. (1980). A heteroskedasticity-consistent covariance matrix estimator and a direct test for heteroskedasticity. Econometrica, 48(4), 817–838. https://doi.org/10.2307/1912934