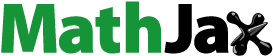
Abstract
The early optimal crop age for harvest is a desirable characteristic in cassava. Many cassava accessions have been disregarded due to their late maturity. In Ethiopia, there is a lack of data on cassava maturity studies, highlighting the need for further research. The study aimed to determine the optimal crop age for harvest in cassava using stored root yield over time. A total of 64 accessions were evaluated using a lattice design from 10 to 22 months after planting (MAP). Results of the analysis of variance showed a highly significant (p < 0.001) difference in the optimal crop ages for harvest in cassava. The fresh storage root yield (FSRY) ranged from 5.5 t ha-1 at 10 MAP to 72 t ha-1 at 18 MAP crop age. Clustering analysis categorized 64 accessions into three clusters with varied crop ages. The highest genetic coefficient of variation (35.2%) was at 10 MAP crop ages, while the lowest genetic coefficient of variation (21.8%) was at 18 MAP crop age. This indicates that the evaluated accessions had high genetic variability in the optimal FSRY over time. Furthermore, the FSRY had high broad-sense heritability and a genetic advance percent mean at all crop ages, indicating the presence of an additive gene effect. G30 at 10 MAP, G11, G17, G31, G55, and G56 at 12 MAP crop ages are selected as promising candidates for utilization. The study indicates that cassava cultivars can be developed below the national recommended crop age for harvest in the evaluated population.
PUBLIC INTERST STATEMENT
Cassava is one of the main subsistence crops grown for food by a large proportion of farmers. It has great potential to diversify the food base of families in Africa. Despite the crop’s importance, it is underutilized and less researched in terms of genetic variability. The early optimal crop age for harvest is a desirable characteristic in cassava. Many cassava accessions have been disregarded due to their late maturity. In Ethiopia, there is a lack of data on cassava maturity studies, highlighting the need for further research. Thus, the study aimed to determine the optimal crop age for harvest in cassava using stored root yield over time, and the identified accessions can be utilized for production.
REVIEWING EDITOR:
1. Introduction
Cassava is a perennial dicotyledonous and vegetatively propagated shrub grown throughout the tropics and subtropics for its starchy and thickened roots (Anikwe & Ikenganyia, Citation2018; FAOSTAT, Citation2021). It is grown in more than 90 countries worldwide and is the fourth most important food crop in developing countries after rice, wheat, and maize (FAOSTAT, Citation2021; Jansson et al., Citation2009). Cassava is thought to be the primary source of daily energy for more than 800 million people worldwide, with over 500 million of these individuals living in sub-Saharan Africa (Bilate Daemo et al., Citation2023c; FAOSTAT, Citation2021; Tan, Citation2015). After sugarcane and sugar beets, the cassava plant produces the third-highest amount of carbohydrates per unit of area among crop plants (Joint, Citation2018). In comparison to 176,000 for rice, 110,000 for wheat, and 200,000 for maize, it may yield food calories at rates reaching 250,000 per hectare per day (Joint, Citation2018; Bilate Daemo et al., Citation2022). Cassava is an excellent crop to withstand the detrimental effects of climate change due to its relative tolerance to droughts and even brief flooding. Thus, it is crucial for fighting hunger in a world of climate change, ensuring food security when and where other crops fail, generating cash through cassava processing and sales, boosting local agro-industries, lowering the cost burden of food imports on national governments, serving as a source of starch for a variety of industrial uses, and as a potential export crop, particularly for use as livestock and chicken feed (Akinbola & Ikuemonisan, Citation2021; Ikuemonisan et al., Citation2020). As a result, cassava is becoming more crucial in sub-Saharan Africa and some regions of Asia for ensuring food security, fostering the growth of rural agro-industry, and creating jobs and income (Joint, Citation2018).
Cultural practices, pest control, and harvesting at the right stages are all necessary for a genotype to attain its full potential (Soares et al., Citation2017). Additionally, the optimal harvesting period must be integrated with relationships among agronomic parameters for genotype recommendation in production systems (Lessa et al., Citation2019). According to Mtunguja et al. (Citation2016), the best harvest stage for cassava has not been established because the crop varies in optimal crop age for harvest. For instance, cassava roots of the Yacé variety were considered to have the optimal root yield when they were twelve months old. However, in Thailand, it was frequently left in the ground until sixteen months, the optimal crop age for harvest (Chipeta et al., Citation2016), and in Nigeria, the optimal harvest for the Yace variety was at 24 months old, and other varieties occasionally lasted up to 48 months old (Okogbenin et al., Citation2013; Soares et al., Citation2017). According to Vitor et al. (Citation2019), limited information about the cycle of a cassava cultivar and harvesting at an inappropriate time may cause financial loss to farmers. Because of the strong demand, some producers prefer to harvest early, neglecting the impact of the harvest period on agronomic and biochemical quality. Hence, inappropriate crop age at harvest can have a negative effect on agronomic and biochemical quality (Oriola & Raji, Citation2013).
Cassava is typically considered a long-duration crop, and several cassava genotypes have been abandoned over the years due to prolonged optimal storage root yield for harvest (Tumuhimbise et al., Citation2015). However, recent research has revealed the existence of optimal cassava genotypes for harvest at 6 MAP (Kamau et al., Citation2011; Suja et al., Citation2010). Souza (Citation2017) reported that the degree of variability in the optimal crop ages for harvest among cassava germplasm confirms the importance of research work for the evaluation and selection of new and/or improved genotypes. Suja et al. (Citation2010) and Ntawuruhunga and Dixon (Citation2010) stated that the optimal crop age for harvest in cassava is influenced by both genetic and environmental factors. Thus, a better understanding of the interaction between these variables is necessary for successfully selecting the optimal crop age for harvest in cassava. Because it is thought that an earlier optimal crop age for harvest would transform cassava from a traditional to an industrial crop, it has become an increasingly important characteristic (Okogbenin et al., Citation2013). Cassava genotypes differ in their rate and pattern of assimilation and hence in their earliness, which explains some of the variances in the optimal crop age for harvest in cassava (Lessa et al., Citation2019). Furthermore, introducing early-bulking genotypes with reduced growth periods is one strategy to improve cassava productivity in terms of fresh storage root yield (FSRY) per unit of time (Tumuhimbise et al., Citation2015). However, in Ethiopia, there is relatively little evidence assessing the genetic variability of cassava accessions for the optimal crop age for harvest. This highlights the need for research to assess the optimal crop age for harvest in cassava. This information can be exploited in crop improvement for desirable cassava accessions by reducing crop ages along with the optimal storage root yield, which could benefit smallholder farmers by providing opportunities for the effective utilization of their land. Therefore, the objective of this study was to assess the genetic variability and determine the optimal crop age for harvest in cassava based on accumulated storage root yield through periodic crop ages at harvest.
2. Materials and methods
2.1. Description of the study area
The study was carried out in accordance with guidelines provided by the national research thematic focus. It was conducted at the Wara and Disa research sites in the Dawuro zone of the Southwest Ethiopia people’s Regional State. Both sites have nitosols types with weathered red soil. Wara is located at a latitude of 7° 34’ N and a longitude of 37° 44’ E, with an altitude of 1500 meters above sea level (Bilate Daemo et al., Citation2023a). The mean annual rainfall is 1400 mm, and the mean annual temperature is 23.5 °C. Disa is located at latitude of 7° 09’ 45" N and a longitude of 37° 03’ 16" E, with an altitude of 1244 meters above sea level (Bilate Daemo et al., Citation2023a). The average total annual rainfall is 1151 mm, and the average annual temperature is 24.43 °C. In the study period, the climate data of research sites are presented in .
Table 1. Wara and Disa trial sites received total rainfall and temperature across seven crop ages.
2.2. Plant materials
The plant materials were collected in accordance with guidelines provided by national research legislation and standards. The study used 64 cassava accessions, with 15 from Nigeria and 49 from the Jimma and Hawassa Agricultural Research Center ().
Table 2. The list of cassava accessions used in the study and their some of morphological characteristics.
2.3. Experimental design and management
The field experiment was laid out an 8x8 simple lattice with two replications. The cassava cuttings were planted with a spacing of 1 m by 1 m between rows and plants, using a single-row plot of 23 m in length. The experiment was conducted from March 1, 2020, to December 30, 2021, across cropping seasons. The seven different crop ages at harvest were 10, 12, 14, 16, 18, 20, and 22 months after planting (MAP), and crop ages at harvest were used as treatments for the study. In total, 21 plants within a row were marked in the middle, and 3 consecutive plants per plot were sampled for each crop age at harvest. Weeding and cultivation were performed four times in each location.
2.4. Data collection
The data were collected in accordance with guidelines provided by the national cassava crop research standard. Sequential harvesting was done at 10, 12, 14, 16, 18, 20, and 22 months after planting (MAP) from two locations. At each crop age at harvest, fresh storage root yield data from five consecutive plants per plot were collected, weighed in kg using a balance, and then converted to t ha-1.
2.5. Data analysis
The study used R and SAS software packages for statistical analyses, testing individual location data for homogeneity and normality of error variance, and merging data for statistical analysis (SAS, Citation2019; Team R. D. C., 2020). Additionally, the homogeneity of the individual crop age at harvest experiment error variance was assessed using Bartlett’s tests of variance homogeneity. Then, the combined analysis of variance for crop age at harvest was performed after confirming the homogeneity of the variances. The following model was used to generate a combined ANOVA (Gomez & Gomez, Citation1984):
Where is the observed value of genotype
in block
, replication
of crop age at harvest
and evaluated at location
, μ is the grand mean,
is the effect of genotype i,
is the effect location
,
is the effect of crop age at harvest
,
is the interaction effect genotype
with location
,
is the interaction effect of genotype
with crop age at harvest j,
is the interaction effect of location
with crop age at harvest
,
is the interaction effect of genotype
with location
and crop age at harvest
,
is the effect of replication
,
is the effect of block
in replication
is the error (residual) effect of genotype
in block
, replication k of crop age at harvest
and location
.
Clustering analysis for fresh storage root yield was performed based on the unweighted pair group method with arithmetic average (UPGMA). The number of clusters was determined using the values of pseudo F and pseudo T², as described by Mohammadi and Prasanna (Citation2003). The study evaluated variations among accessions using mean, range, phenotypic variance, genotypic variance, genotypic coefficient of variation (GCV), phenotypic coefficient of variation (PCV), broad-sense heritability, and GAM, employing formulas presented in .
Table 3. The formulas used to determine genetic parameters for fresh storage root yield traits.
3. Results
3.1. Analysis of variance
The two-way ANOVA used to assess genetic variability for harvest in cassava, and the results showed that fresh storage root yield was significantly (p < 0.001) affected by crop age, accession, and their interactions (). This indicates that the optimal crop age for harvest varied among the evaluated cassava accessions. Thus, there is a high chance of selecting desirable accessions for the optimal crop age for harvest in cassava from the studied population.
Table 4. The two-way ANOVA for fresh storage root yield from 64 cassava accessions over seven crop age.
3.2. The mean performance accessions in response to crop age at harvest
The descriptive statistics for fresh storage root yield in response to crop ages at harvest are presented in , and the fresh storage root yield was significantly affected by crop age at harvest among accessions. Wide ranges of differences were observed for fresh storage root yield across crop age at harvest (). Accessions G30 (47.5), G55 (68.0), G40 (64.0), G59 (64.5), G38 (72.0), G62 (65.7), and G53 (61.0) had the highest yield (t ha-1) at 10 MAP, 12 MAP, 14 MAP, 16 MAP, 18 MAP, 20 MAP, and 22 MAP crop ages, respectively (). The highest accession mean storage root yield (t ha-1) averaged across crop ages was G53 (51.1), followed by G40 (48.8), G54 (47.3), and G38 (47.0 t ha-1), while the lowest yield was G16 (20.3 t ha-1), with an overall grand mean of 37.0 t ha-1 (). The mean crop age for storage root yield (t ha-1) averaged across accessions ranged from 24.2 t ha-1 at 10 MAP to 43.56 t ha-1 at 18 MAP (). The lowest storage root yield (5.5 t ha-1) was for G22 at 10 MAP crop ages, while the highest storage root yield (72.0 t ha-1) was found for G38 at 18 MAP crop ages (). Among the evaluated accessions, the G30 (47.5 t ha-1) at 10 MAP crop age, the G11 (55.5 t ha-1), the G17 (49.8 t ha-1), the G31 (30.3 t ha-1), the G55 (68.0 t ha-1), and the G56 (60.0 t ha-1) at 12 MAP crop age were shown to have earlier attained the optimal storage root yield for harvest (). On the other hand, in 21.9%, 23.44%, 35.9%, and 3.13%. of the evaluated accessions, the peak storage root yield was harvested at 14 MAP, 16 MAP, 18 MAP, and 20 MAP crop ages, respectively ().
Table 5. Descriptive statistics fresh storage root yield of 64 accessions evaluated at the seven crop ages.
Table 6. Mean performance of 64 cassava accessions fresh storage root yield (t ha-1) across seven crop ages.
Table 7. Clusters of cassava accessions based on fresh storage root yield t ha-1 across seven crop ages.
3.3. Cluster analysis
The clustering analysis revealed three distinct clusters formed using pseudo-F (25.2) and pseudo-t-squared (2.9) criteria, with the maximum increase occurring at this point (Figure S1). The 64 accessions were distributed into three clusters: cluster I (26 accessions), cluster II (35 accessions), and cluster III (3 accessions). The accessions grouped under cluster I were mainly identified by the optimal fresh storage root yield harvested at 16 MAP crop ages, followed by 14 MAP crop ages. Furthermore, accessions expressing their optimal storage root yield for harvest at 10 MAP (G30) and 12 MAP (G31) crop ages were grouped under this cluster ( and ). Under cluster II, 35 accessions were grouped together by having the optimal storage root yield for harvest at 18 MAP crop ages, followed by 16 MAP crop ages. Also, accessions with an undefined optimal crop age for harvest, like G1 and G14, were grouped in cluster II. Finally, three accessions were classified in cluster III due to the optimal storage root yield for harvest at 12 MAP crop ages, and these accessions were selected for the breeding program.
3.4. Variances, heritability, and the coefficient of variance component
Individual crop ages were estimated separately for the genetic parameters study. The highest genetic variance for fresh storage root yield was 108.40 at 12 MAP crop ages, followed by 105.50 at 16 MAP and 102.50 at 22 MAP crop ages, while the lowest genetic variance for fresh storage root yield was 72.80 at 10 MAP crop ages (). Similarly, the phenotypic variance for fresh storage root yield ranged from 80.0 at 10 MAP crop ages to 132.3 at 20 MAP crop ages. The highest genetic coefficient of variation for fresh storage root yield (GCV = 35.2%) was at 10 MAP crop ages, followed by 32.5% at 12 MAP, 26.4% at 20 MAP, and 22 MAP, while the lowest genetic coefficient of variation for fresh storage root yield (GCV = 21.8%) was at 18 MAP crop ages (). The phenotypic coefficients of variation (PCV) for fresh storage root yield ranged from PCV = 24.1% at 18 MAP crop ages to PCV = 37.2% at 10 MAP crop ages (). presents the estimated heritability and genetic advance as a percent mean of fresh storage root yield across seven crop ages at harvest. The broad-sense heritability values for fresh storage root yield ranged from 77.3% at 20 MAP crop ages to 93.4% at 12 MAP crop ages, while genetic advance as a percentage mean of fresh storage root yield ranged from 36.0% at 18 MAP crop ages to 63.2% at 12 MAP crop ages ().
Table 8. Variances, heritability, and coefficient of variances of fresh storage root yield of 64 accessions of cassava assessed on seven separate crop ages in southwest Ethiopia.
4. Discussion
In the present study, the interaction between crop age at harvest and accession was significant for fresh storage root yield among the evaluated accessions over time. This indicates that the evaluated accessions exhibit significant variability in their optimal crop age for harvest. Thus, genetic progress could be achieved for the optimal crop age for harvest through phenotypic selection. This finding is consistent with a previous study by Mulualem and Ayenew (Citation2012), who reported that the interaction between crop age at harvest and genotype had a remarkable effect on yield and yield components. Similarly, Akpan and Udoh (Citation2017) and Lessa et al. (Citation2019) reported that the interaction of crop age at harvest and genotype was significant for fresh storage root yield.
The timing of planting and harvesting in cassava is a crucial decision for farmers, as environmental factors like rainfall significantly influence the crop’s starch content and fresh storage root yield. In the present study, accessions with the optimal crop age for harvest at 10 MAP, 12 MAP, 20 MAP, and 22 MAP were harvested after the end of the heavy rain seasons and during the dry seasons. Hence, the study hypothesized that these accessions would be harvested with full root yield weight since harvesting in the dry season could result in low root yield losses; whereas their root yield weight could be expected to decrease if harvested during the rainy season or at the onset of rain. In contrast, accessions with the optimal crop age for harvest at 14 MAP, 16 MAP, and 18 MAP were harvested after a prolonged dry season, at the onset of rain, and during the peak rainy season. Consequently, their root yield weight could be expected to decline due to starch remobilization from the roots to regenerate a new canopy and losses from root rot. Therefore, the study suggests that cassava harvesting should be conducted during the dry season to maximize root yield. This aligns with previous studies by Enesi et al. (Citation2022a), Enesi et al. (Citation2022b), and Ologunde et al. (Citation2023), which reported variations in storage root yield weight during harvesting in the dry and rainy seasons. The conclusion that harvesting storage roots at the onset of rain or during the rainy season leads to a decrease in both starch content and fresh storage root yield. This decline is attributed to both physiological factors (related to the natural processes within the plant) and biotic factors (such as interactions with other living organisms like pests or pathogens) that occur during these periods. In essence, the conditions during the onset of rain or the rainy season seem to negatively impact both the quality and quantity of the harvested storage roots.
At each crop age at harvest, the performance of each accession varied, and the time it took to reach peak storage root yield was significantly different. Consequently, one, five, eleven, fifteen, and twenty-nine accessions reached the maximum storage root yield for harvest at 10 MAP, 12 MAP, 14 MAP, 16 MAP, and 18 MAP crop ages, respectively (). This suggests that the findings reveal variations among accessions regarding the best or most suitable ages at which to harvest crops. In other words, different accessions show preferences for specific ages at which they exhibit optimal yield for harvesting. This implies that factors such as genetic differences or environmental conditions might influence the ideal timing for harvesting crops across various accessions. Hence, the presence of genetic variation among accessions provides ample opportunity for selection to identify and exploit the optimal crop age for harvest, leading to the development of cultivars with enhanced productivity, quality, and adaptability. However, accessions represented by G1, G14, and G22 were not known for their optimal crop age for harvest, and they were steadily growing by resisting dry spell periods throughout the end-of-crop age at harvest (). Nearly all accessions showed that the storage root yield increased steadily from 10 MAP to 18 MAP crop ages, while their growth was reduced during the 20 MAP and 22 MAP crop ages (). This suggests that accessions may be reaching their optimal crop age for harvest within a specified period of time.
In Ethiopia, the national recommended crop age for cassava, including improved cultivars, is harvested > 18 MAP, with a national average yield of 10.9 t ha-1 (Joint, Citation2018; Bilate Daemo et al., Citation2023b). In this regard, in the present study, all evaluated accessions apart from G1, G16, G18, and G22 produced > 10.9 t ha-1 at 10 MAP crop ages (). Except for the mentioned accessions, regardless of the peak attainment of storage root yield, all evaluated accessions could be selected as having the optimal fresh storage root yield for harvest at 10 MAP crop ages for production, demonstrating the genetic potential of accessions in terms of storage root yield. However, among the evaluated accessions, based on the maximum accumulated storage root yield, G30 at 10 MAP crop ages, and G11, G17, G31, G55, and G56 at 12 MAP crop ages were shown to have earlier attained the optimal crop age for harvest. Thus, these accessions can be selected as desirable for breeding programs since they reached the optimal age for harvest earlier at 10 MAP and 12 MAP compared to the rest of the evaluated accessions. Except for G31, all desirable accessions produced storage root yields greater than the grand mean of 18 MAP harvested accessions and were harvested earlier (6–8 months) compared to 18 MAP accessions. This demonstrates that using these desirable accessions could be a good opportunity to boost smallholder farmers’ production and income.
This finding is in line with previous work by Adjebeng-Danquah et al. (Citation2016b), who evaluated 20 cultivars using four harvesting intervals to develop the optimal crop age for harvest in the Guinea Savannah ecology. They found variation in accumulated root yield across crop age at harvest among the evaluated cultivars, and one cultivar was identified as optimal for harvest at 10 MAP. Tumuhimbise et al. (Citation2015) investigated 12 genotypes over five harvest intervals and discovered the maximum fresh storage root yield harvested at 9 MAP crop ages. Akpan and Udoh (Citation2017) harvested at 8 MAP crop ages for maximum storage root yield; Lessa et al. (Citation2019) and Gnamien et al. (Citation2020) achieved optimal storage root yield at 12 MAP crop ages. Furthermore, a similar study was conducted by Nzola et al. (Citation2022) in the DR Congo using seven cultivars with 9 MAP to 24 MAP crop ages evaluated at each three-month interval. They reported that the best fresh and dry storage root yield was harvested at 15 MAP crop ages. In Nigeria, Ologunde et al. (Citation2023) evaluated two genotypes at three crop ages (9 MAP, 11 MAP, and 13 MAP) and reported that harvesting at 13 MAP crop ages attains higher cassava root yields.
Cluster analysis is used to categorize similar accessions into one group and different ones into separate groups. As a result, the 64 accessions were classified into three clusters, with varying numbers of accessions represented in each cluster. Distinct clusters are formed from accessions originating from the same or different sources. For instance, in cluster I, the distribution of accessions showed that there were 26 (40.63%) accessions, with 17 from Hawassa and 9 from Nigeria, indicating differences in the genetic makeup of the accessions. This finding suggests that cluster II contains a large number of accessions with the optimal crop age for harvest at 18 MAP, while cluster I possesses a significant number of accessions with the optimal crop age for harvest at 14–16 MAP, along with some accessions with the optimal crop age for harvest below 14 MAP. Cluster III contains accessions with the optimal crop age for harvest at 12 MAP.
The genotypic and phenotypic coefficients of variation were used to quantify genetic variability across crop ages (Mulualem et al., Citation2013). The phenotypic coefficient of variation (PCV), which demonstrates the proportional influence of environmental variation on total variance, was nearly equal to that of the genotypic coefficient of variation (GCV). In previous studies by Tumuhimbise et al. (Citation2015), Ntawuruhunga and Dixon (Citation2010), and Akinwale et al. (Citation2010), the PCV value was significantly greater than the GCV for storage root yield. This indicates that the total variation in the attributes was caused by environmental factors as well as genetic factors. However, in the present study, the difference between GCV and PCV was small. For instance, fresh storage root yield showed the highest ratio of GCV/PCV values at 12 MAP (0.97), followed by 22 MAP (0.96), 10 MAP (0.95), and 14 MAP (0.94). The high GCV to PCV ratio suggests that fresh storage root yield variability is genetically influenced, with minimal environmental influence, making it inherited. This suggests that substantial genetic gain in the attribute would be possible through selection. Peprah et al. (Citation2020) and Jiwuba (Citation2020) found a similar report, where fresh storage root yield had a high ratio of GCV to PCV among the evaluated cassava genotypes. However, this finding disagrees with that reported by Mulualem et al. (Citation2013), who stated that fresh storage root yield was found to have a low ratio of GCV to PCV values in cassava.
Bhateria et al. (Citation2006) classified heritability estimates as high (> 50%), medium (30–50%), and low (< 30%), whereas Johnson et al. (Citation1955) classified the genetic advance percent mean as high (20%), moderate (10–20%), and low (0–10%). Based on this categorization, the fresh storage root yield had high broad-sense heritability and a genetic advance percent mean at all crop ages. This indicates the presence of an additive gene effect. Hence, phenotypic selection at each crop age for fresh storage root yield could yield possible genetic gain. The study suggests that rapid progress in fresh storage root yield could be achieved with high heritability and genetic advance percent mean, even with a simple phenotypic selection procedure.
5. Conclusion
The study identified significant variation in accumulated storage root yield among accessions over time, suggesting that genetic progress could be achieved through simple phenotypic selection. The time taken by accessions to reach their maximum storage root yield varied across crop ages, indicating a genetic background. Environmental factors such as rainfall significantly influence cassava fresh storage root yield, underscoring the importance of optimal harvest timing for farmers to maximize profits. Therefore, the study recommends that growers should avoid harvesting cassava during periods when rains begin after extended dry periods or during rainy seasons. The fresh storage root yield exhibits a high ratio of GCV to PCV, indicating genetic influence and an additive gene effect. The study found that cassava accessions producing optimal storage root yield when harvested at 10 MAP and 12 MAP crop ages were selected as desirable for the breeding program. Promising candidates for the breeding program include G30 at 10 MAP, G11, G17, G31, G55, and G56 at 12 MAP, as they produce optimal storage root yield when harvested at these crop ages. These accessions could prove profitable for smallholder farmers, offering an early return on investment. The study confirmed high genetic variability in storage root yield across crop ages, suggesting its potential use in developing cultivars with optimal crop ages for harvest below the nationally recommended crop age.
Authors’ contributions
Berhanu Bilate Daemo: Conceptualization, data curation, formal analysis, funding acquisition, investigation, project administration, methodology, writing original draft, writing review and editing. Derbew Belew: Conceptualization, project administration, supervision, writing review and editing. Tewodros Mulualem: Conceptualization, project administration, supervision, writing review and editing. Wosene Gebreselassie: Conceptualization, project administration, supervision, writing review and editing.
Supplemental Material
Download MS Word (42.8 KB)Acknowledgments
The authors acknowledge the universities of Jimma and Wolita Sodo for providing funding for the study. The authors express gratitude to Jimma and Hawassa Agricultural Research Centers and the International Institute for Tropical Agriculture for providing the necessary planting materials for the study.
Disclosure statement
No potential conflict of interest was reported by the author(s).
Data availability statement
Datasets will be made available upon request.
Additional information
Funding
Notes on contributors
Berhanu Bilate Daemo
Berhanu Bilate Daemo is a PhD candidate at Jimma University, serving as a lecturer and researcher in the Plant Science departments at Wolaita Sodo University’s Dawuro Tarcha campus in Tarcha, Ethiopia. His research interest lies in generating and enhancing crop varieties for improved yield, resilience to biotic and abiotic stresses, and nutritional quality.
Derbew Belew Yohannes
Derbew Belew Yohannes, PhD (Professor) is a researcher, lecturer, and advisor to PhD students at the Department of Horticulture and Plant Sciences, Jimma University College of Agriculture and Veterinary Medicine in Jimma. Ethiopia. His research interest lies in generating and enhancing crop varieties for improved yield, resilience to biotic and abiotic stresses, and nutritional quality.
Tewodros Mulualem Beyene
Tewodros Mulualem Beyene is a PhD, a senior researcher, advisor to PhD students, and head of the Horticultural Crop Research Department at the Jimma Agricultural Research Centre in Jimma, Ethiopia. His main research interest is in plant breeding, focusing on generating and enhancing crop varieties for improved yield, resilience to biotic and abiotic stresses, and nutritional quality.
Wosene Gebreselassie Abtew
Wosene Gebreselassie Abtew, PhD (Associate Professor) is a researcher, lecturer, and advisor to PhD students at the Department of Horticulture and Plant Sciences, Jimma University College of Agriculture and Veterinary Medicine in Jimma, Ethiopia.His main research interest lies in leveraging genetic diversity and modern breeding techniques such as marker-assisted selection and genomic selection to accelerate crop improvement.
References
- Adjebeng-Danquah, J., Gracen, V. E., Offei, S. K., Asante, I. K., & Manu-Aduening, J. (2016b). Genetic variability in storage root bulking of cassava genotypes under irrigation and no irrigation. Agriculture & Food Security, 5(1), 1–12. https://doi.org/10.1186/s40066-016-0055-7
- Akinbola, A. E., & Ikuemonisan, E. S. (2021). Future trends in cassava production: indicators and its implications for food supply in Nigeria. Asian Journal of Agricultural Extension, Economics & Sociology, 39(3), 60–74. https://doi.org/10.9734/ajaees/2021/v39i330546
- Akinwale, M. G., Akinyele, B. O., Dixon, A. G. O., & Odiyi, A. C. (2010). Genetic variability among forty-three cassava genotypes in three agro-ecological zones of Nigeria. Plant Breeding and Crop Science, 2(5), 104–109.
- Akpan, E. A., & Udoh, V. S. (2017). Evaluation of Cassava (Manihot esculenta Crantz) genotype for yield and yield component, tuber bulking, early maturity in Cross River basin flood plains, Itu, Akwa Ibom State, Nigeria. Canadian Journal of Agriculture and Crops, 2(2), 68–73. https://doi.org/10.20448/803.2.2.68.73
- Allard, R. W. (1960). Principles of Plant Breeding. John Willy and sons. Inc. 485p.
- Anikwe, M. A. N., & Ikenganyia, E. E. (2018). Ecophysiology and production principles of Cassava (Manihot species). In Southeastern Nigeria Cassava. Intechopen. https://doi.org/10.5772/intechopen.70828
- Bhateria, S., Sood, S. P., & Pathania, A. (2006). Genetic analysis of quantitative traits across environments in linseed (Linum usitatissimum L.). Euphytica, 150(1-2), 185–194. https://doi.org/10.1007/s10681-006-9106-7
- Bilate Daemo, B., Belew Yohannes, D., Mulualem Beyene, T., & Abtew, W. G. (2023c). Phenotypic characterization, evaluation, and classification of cassava (Manihot esculenta crantz) accessions in Ethiopia. International Journal of Agronomy, 2023, 1–12. https://doi.org/10.1155/2023/1559070
- Bilate Daemo, B., Belew Yohannes, D., Mulualem Beyene, T., & Gebreselassie Abtew, W. (2022). Biochemical analysis of cassava (manihot esculenta Crantz) accessions in southwest of Ethiopia. Journal of Food Quality, 2022, 1–13. https://doi.org/10.1155/2022/9904103
- Bilate Daemo, B., Belew Yohannes, D., Mulualem Beyene, T., & Gebreselassie Abtew, W. (2023a). AMMI and GGE biplot analyses for mega environment identification and selection of some high-yielding cassava genotypes for multiple environments. International Journal of Agronomy, 2023, 1–13. https://doi.org/10.1155/2023/6759698
- Bilate Daemo, B., Belew Yohannes, D., Mulualem Beyene, T., & Gebreselassie Abtew, W. (2023b). Assessment of cassava utilization patterns, postharvest handling practices, and productivity influencing factors in South and Southwest Ethiopia. Journal of Food Quality, 2023, 1–12. https://doi.org/10.1155/2023/9914370
- Burton, G. W., & Devane, D. E. (1953). Estimating heritability in tall fescue (Festuca arundinacea) from replicated clonal material 1. Agronomy Journal, 45(10), 478–481. https://doi.org/10.2134/agronj1953.00021962004500100005x
- Chipeta, M. M., Shanahan, P., Melis, R., Sibiya, J., & Benesi, I. R. (2016). Early storage root bulking index and agronomic traits associated with early bulking in cassava. Field Crops Research, 198, 171–178. https://doi.org/10.1016/j.fcr.2016.09.004
- Enesi, R. O., Hauser, S., Pypers, P., Kreye, C., Tariku, M., & Six, J. (2022a). Understanding changes in cassava root dry matter yield by different planting dates, crop ages at harvest, fertilizer application and varieties. European Journal of Agronomy, 133, 126448. https://doi.org/10.1016/j.eja.2021.126448
- Enesi, R. O., Pypers, P., Kreye, C., Tariku, M., Six, J., & Hauser, S. (2022b). Effects of expanding cassava planting and harvesting windows on root yield, starch content and revenue in southwestern Nigeria. Field Crops Research, 286, 108639. https://doi.org/10.1016/j.fcr.2022.108639
- FAOSTAT. (2021). Food and agriculture organization of the united nations databases agricultural production; FAO Statistics Databases: Rome, Italy, Available online: http://www.fao.org/faostat/en/(accessed on 20 June, 2022),2021.
- Gnamien, A. C. A., Martial-Didier, A. K., Martin, D. K., Messou, T., Honora, T. B. F., & Kablan, T. (2020). Effect of harvest date on technological yield and evolution of hydrocyanic acid loss rate after transformation of cassava root (Manihot Esculenta CRANTZ) from Yacé variety by Placali and Attiéké Consumed in Côte d’Ivoire. International Journal of Advances in Scientific Research and Engineering, 06(01), 147–155. https://doi.org/10.31695/IJASRE.2020.33671
- Gomez, K. A., & Gomez, A. A. (1984). Statistical procedures for agricultural research. John wiley & sons.
- Ikuemonisan, E. S., Mafimisebi, T. E., Ajibefun, I., & Adenegan, K. (2020). Cassava production in Nigeria: trends, instability and decomposition analysis (1970–2018). Heliyon, 6(10), e05089. https://doi.org/10.1016/j.heliyon.2020.e05089
- Jansson, C., Westerbergh, A., Zhang, J., Hu, X., & Sun, C. (2009). Cassava, a potential biofuel crop in (the) People’s Republic of China. Applied Energy, 86, S95–S99. https://doi.org/10.1016/j.apenergy.2009.05.011
- Jiwuba, L. C. (2020). Genetic variability and genetic advance of elite varieties of cassava (Manihot esculenta Crantz). Nigeria Agricultural Journal, 51(3), 81–84.
- Johnson, H. W., Robinson, H. F., & Comstock, R. E. (1955). Estimates of genetic and environmental variability in soybeans. Agronomy Journal, 47(7), 314–318. https://doi.org/10.2134/agronj1955.00021962004700070009x
- Joint, F. A. O. (2018). Cassava production guidelines for food security and adaptation to climate change in Asia and Africa. (No. IAEA-TECDOC--1840). Joint FAO/IAEA Division of Nuclear Techniques in Food and Agriculture.
- Kamau, J., Melis, R., Laing, M., Derera, J., Shanahan, P., & Ngugi, E. C. (2011). Farmers’ participatory selection for early bulking cassava genotypes in semi-arid Eastern Kenya. Journal of Plant Breeding and Crop Science, 3(3), 44–52.
- Lessa, L. S., Ledo, C. A. S., & Santos, V. S. (2019). Effect of harvesting times on agronomic characteristics of industrial cassava genotypes. Revista Brasileira de Ciências Agrárias - Brazilian Journal of Agricultural Sciences, 14(2), 1–6. https://doi.org/10.5039/agraria.v14i2a5647
- Mohammadi, S. A., & Prasanna, B. M. (2003). Analysis of genetic diversity in crop plants—salient statistical tools and considerations. Crop Science, 43(4), 1235–1248. https://doi.org/10.2135/cropsci2003.1235
- Mtunguja, M. K., Laswai, H. S., Kanju, E., Ndunguru, J., & Muzanila, Y. C. (2016). Effect of genotype and genotype by environment interaction on total cyanide content, fresh root, and starch yield in farmer-preferred cassava landraces in Tanzania. Food Science & Nutrition, 4(6), 791–801. https://doi.org/10.1002/fsn3.345
- Mulualem, T., & Ayenew, B. (2012). Cassava (Mannihot esculenta Crantz) varieties and harvesting stages influenced yield and yield related components. Journal of Natural Sciences Research, 2(10), 122–128.
- Mulualem, T., WeldeMichael, G., Benti, T., & Walle, T. (2013). Genetic diversity of cassava (Manihot esculenta Crantz) genotypes in Ethiopia. Greener J. Agric. Sci, 3, 636–642.
- Ntawuruhunga, P., & Dixon, A. G. (2010). Quantitative variation and interrelationship between factors influencing cassava yield. Journal of Applied Biosciences, 26(4), 1594–1602.
- Nzola, M., Ndonda, A., Bidiaka, S., Gwanyebit Kehbila, A., Tata-Hangy, W., Tambu, E., & Binzunga, M. (2022). Screening cassava for time of maturity to respond to various market needs: Case study in African sub-tropical zones. Journal of the Science of Food and Agriculture, 102(8), 3169–3178. https://doi.org/10.1002/jsfa.11660
- Okogbenin, E., Setter, T. L., Ferguson, M., Mutegi, R., Ceballos, H., Olasanmi, B., & Fregene, M. (2013). Phenotypic approaches to drought in cassava. Frontiers in Physiology, 4, 93. https://doi.org/10.3389/fphys.2013.00093
- Ologunde, O. H., Busari, M. A., Adebayo, O. E., Olowokere, F. A., Kreye, C., Salako, F. K., & Hasser, S. (2023). Effect of planting date and crop age at harvest on cassava root and starch yield in the derived Savanna of southwestern Nigeria. Advances in Agriculture, Horticulture and Entomology, 1(1), 1–13.
- Oriola, K., & Raji, A. (2013). Effects of tuber age and variety on physical properties of cassava [Manihot esculenta (Crantz)] roots. Innovative Systems Design and Engineering, 4(9), 15–25.
- Peprah, B. B., Parkes, E., Manu-Aduening, J., Kulakow, P., Van Biljon, A., & Labuschagne, M. (2020). Genetic variability, stability and heritability for quality and yield characteristics in provitamin A cassava varieties. Euphytica: Netherlands Journal of Plant Breeding, 216(2), 31. https://doi.org/10.1007/s10681-020-2562-7(0123456789().,-volV()0123456789().,-volV)
- SAS. (2019). Institute Inc, SAS® 9.4 System Options: Reference. SAS Institute Inc. 2019.
- Singh, R. K., & Chaudhary, B. D. (1977). Biometrical methods in quantitative genetic analysis. Biometrical methods in quantitative genetic analysis. Kalyani Publishers.
- Soares, M. R. S., do Nascimento, R. D. M., Viana, A. E. S., Cardoso, A. D., Braga, G. C. M., & Júnior, J. N. L. F. (2017). Componentes agronômicos qualitativos e caracterização morfológica de variedades de mandioca (Manihot esculenta Crantz) em seis épocas de colheita. Scientia Plena, 13(6) https://doi.org/10.14808/sci.plena.2017.061201
- Souza, P. F. D. S. (2017). Impactos dos anos climáticos extremos no rendimento da lavoura temporária de mandioca na região rural da metrópole de Belém–Pará. Brazilian Journal of Climatology, 21, 94–112.
- Suja, G., John, K. S., Sreekumar, J., & Srinivas, T. (2010). Short-duration cassava genotypes for crop diversification in the humid tropics: Growth dynamics, biomass, yield and quality. Journal of the Science of Food and Agriculture, 90(2), 188–198. https://doi.org/10.1002/jsfa.3781
- Tan, S. L. (2015). Cassava–silently, the tuber fills. Utar Agriculture Science Journal, 1(2), 1–13.
- Team, R. D. C. (2020). R: A language and environment for statistical computing. R Foundation for Statistical Computing, Vienna, Austria. http://www. R-project. org
- Tumuhimbise, R., Shanahan, P., Melis, R., & Kawuki, R. (2015). Genetic variation and association among factors influencing storage root bulking in cassava. The Journal of Agricultural Science, 153(7), 1267–1280. https://doi.org/10.1017/S0021859614000999
- Vitor, A. B., Diniz, R. P., Morgante, C. V., Antônio, R. P., & de Oliveira, E. J. (2019). Early prediction models for cassava root yield in different water regimes. Field Crops Research, 239, 149–158. https://doi.org/10.1016/j.fcr.2019.05.017