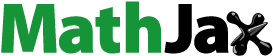
Abstract
The sustainable business model is critical in designing a performance model for logistics service companies by taking into account the dominant factors in managing all the resources needed to carry out operational processes, including the implementation of the logistics system as an effort to increase organizational competitiveness and resilience in the logistics services industry in a sustainable manner. The problems faced today are in line with the increasing frequency of using logistics services with the growth of E-commerce so the competition for logistics services companies, especially in urban areas, is getting tighter. This study used Mix Methods Research (MMR) with an explanatory design strategy and Structural Equation Model-Partial Least Square (PLS) analysis. In this study, a measurement model simulation used a system dynamic approach. Previous studies used the variant-based structural equation method but were limited to 1st-order models. This study aimed to formulate a more comprehensive sustainable logistics management model using the 2nd-order model and continued with a measurement model simulation up to 2040. The population in the study were employees of urban logistics services companies at the supervisor level with more than 5 years of service. Data were collected through questionnaires, observation, Focus Group Discussion (FGD), and literature study from January to August 2023. Based on the results, economic factors, environmental factors, social factors, and customer factors had a positive influence of 13.8 % (0.138 × 100%), 12.9 % (0.129 × 100%), 14.2 % (0.142 × 100%) and 12.9% (0.129 × 100%), respectively, meaning mutual support to the sustainable logistics model. Based on dynamic system analysis, the sustainable logistics model will have an increasing valuation every year. Thus, the relationship between variables in the sustainable logistics model was good in maintaining the company sustainability. This study is expected to give an exact recommendation for logistics companies to maintain their sustainability by always improving the four main factors with the positive influence namely environmental, customer, social, and economic factors.
PUBLIC INTEREST STATEMENT
Logistics companies are in need to maintain their sustainability. This study examined the four main factors of sustainable logistics companies in Indonesia namely economic, environmental, social, and customer factors using Structural Equation Modelling Partial Least Square (SEMPLS) as well as the simulation up to 2040 using system dynamics. Based on the results, all factors had positive influence to maintain the sustainability of logistics companies. The results are also in line with the simulated results using system dynamics, which consequently making the companies to always improving the four main factors mentioned above.
1. Introduction
Competition between logistics service companies is getting tighter, due to the emergence of companies selling goods via the Internet (E-commerce) (Alghababsheh & Gallear, Citation2021). A survey by MarkPlus, Inc in October 2020 showed that 39% of respondents had a significant increase in the frequency of using logistics services in Indonesia compared to before the COVID-19 pandemic (MarkPlus, Citation2020). This also has an impact on the increasing use of E-commerce as a medium for conducting online, remote and instant transactions (Mollenkopf et al., Citation2020). E-commerce covers all development, marketing, sales, delivery, service, and payment processes with the support of a wider network of business partners (Schöder et al., Citation2016).
Today’s companies are not only required to make profits but also to be able to show benefits to society, the environment, and nature. Wise development for society is sustainable development (Z. Wang et al., Citation2018). Logistics services activities have a significant impact on a country’s economy. These logistics services activities in aggregate contribute to the gross domestic product (GDP) ranging from 8.3% to 27%. For example, the United States at 8.5%, Brazil at 12%, South Africa at 12.8%, India at 13.0%, China at 18.0%, Vietnam at 25.0%, and Indonesia at 27.0% (Murphy & Knemeyer, Citation2018). The goal of sustainable development is to raise the well-being of people all over the world whether now or later but with the limit so as not to endanger the environmental condition (Lemaire & Limbourg, Citation2019). Since 2012, the United Nations (UN) had an agreement to make a better living world namely Sustainable Development Goals (SDGs). The agenda resulted in 17 Sustainable Development Goals (SDGs) or global goals, which will become a framework for achieving sustainable development in 2030 (UNDP, Citation2015).
The growth of e-market logistics services in a country is strongly influenced by the country’s economic growth in particular and global economic growth in general. During 2012, Indonesia’s economic growth was around 6.3% and global economic growth was around 3.5%. In general, a country’s logistics costs are around 20% of the country’s GDP (gross domestic product), so if Indonesia’s GDP in 2011 was 7,427 trillion, logistics costs were around 1,485 trillion. This figure reflects the size of the logistics services market in Indonesia, even based on predictions, the percentage of logistics costs from GDP in Indonesia is likely to be greater, namely 25% to 30%. With an average increase per year of 12.1%, the valuation in 2020 was USD 2.76 billion. In addition, in 2030, the logistics will be 2042 trillion rupiahs with 9.6% CAGR (Singh & Darroch, Citation2012).
Logistics services activities require transportation and warehousing operations which certainly consume resources, such as fuel and electricity, and emit emissions that can damage environmental quality. The aggregate costs of the logistics sector in GDP are mainly transportation and warehousing costs. Even a small percentage reduction in logistics costs will have an impact on environmental quality due to reduced fuel consumption, water, waste, and emissions. According to Martins et al. (Citation2019), logistics services activities are very closely related to the achievement of the Sustainable Development Goals (SDGs). Out of 6 from 17 are related to logistics, namely partnership, environment protection, gender equality, economic growth, high quality education, and sustainable city (Martins et al., Citation2019). However, until now, a sustainable logistics model has not been found in logistics services companies covering the six SDG points (Martins et al., Citation2019; Vivaldini, Citation2016). In addition, there has not been a comprehensive study on the effect of each factor on sustainability (Wong et al., Citation2020). In especially in the logistics freight transport segment, the literature on sustainability metrics incorporating social, environmental, and economic components is scarce (Kumar, Citation2022) This study analyzed sustainable factors in the logistics services industry, to serve as a reference for the concept of sustainable development and support development of logistics services activities, especially in urban areas.
Therefore, this study will be a novelty because there has not been a logistics performance measurement model integrating sustainable elements such as economic, environmental, social, and uncertainty risk elements that can influence sustainable logistics performance, namely consumer elements in logistics service companies in Indonesia, and no studies can provide comprehensive and specific analysis of the sustainable model through the development of sub-dimensions to explain the character of a general latent variable by considering several indicators influencing each sustainable factor as well as being equipped with real evidence with dynamic system simulations.
The problems in the logistics industry are quite varied. The problem is not far from the internal and external problems of the industry itself, both in terms of management and administration as well as human resources. On the other hand, another problem is the demand pattern from the market in each period, where the demand pattern is not constant. In addition, the company also faces competition from companies engaged in similar fields in order to meet market demand. This problem occurs when companies feel they are not effective and efficient in distributing goods (Sutoni & Agustian, Citation2017). The poor level of government oversight of urban spatial planning, for example, land that has been designated as a green belt is actually being built for buildings. In addition, poor land transportation management can cause traffic jams and centralization of economic activities in urban areas (Aksa et al., Citation2020). These problems can lead to delays and constraints owned by the distribution center in supplying logistics to the regions, where a portion of the total number of regions that must be supported have frequent problems related to transportation and location. The factors of these problems are dynamic so to minimize these problems flexibility is needed from the logistics distribution for effective and efficient handling in dealing with any changes.
This study is expected to increase insight and knowledge regarding the factors that influence the sustainability of logistics service companies in Indonesia and is also expected to be a means of developing knowledge that is theoretically studied in college. It is hoped that this study can serve as study material for logistics service companies in implementing and making the right decisions in an effort to implement more comprehensive practices/policies to increase organizational competitiveness and resilience.
2. Literature review
2.1. Pillars of sustainability in the logistics service industry
Sustainable development is a concept aimed at providing long-term global life through the wiser a more prudent use and administration of economic and environmental resources, as well as respect for human. This concept also explains the ability of human civilization to create a world for humans and living things in an environmental, social, and economic manner in order to be able to provide for the needs of the population without destroying the ability of the next generation (Somsuk & Laosirihongthong, Citation2017). According to (Khan et al., Citation2023), recently, many business including logistics services companies are keen to improve their opportunities by maintaining sustainability through the practices of industry 4.0 which commonly referred as sustainable supply chain practices (SSCPs).
Then, according to (Kelly et al., Citation2018), there are 4 aspects of sustainability in the service industry, namely economic sustainability, social sustainability, customer sustainability, and environmental sustainability. These four aspects are then called the four pillars of sustainability. The four pillars are interrelated and are the driving pillars for sustainable development. Ideally, these four things can run together and there is a link between the 4 pillars of sustainability or often expressed by a balance of formative indicators, namely economic, environmental, social, and customer management as a driving focus in sustainable development. It can be shown in Figure .
Figure shows the integration of sustainability into logistics services companies. Well-designed logistics services companies may give businesses a unique opportunity to offer dependable, long-term advantages to all value chain participants. The supply chain must engage customers to attract new customers, while, at the same time, seizing opportunities to meet, or even exceed, existing customer expectations (Hosseini-Motlagh et al., Citation2019).
Figure 2. Interaction of sustainability in logistics services.

In terms of environmental factors, the use of renewable energy can be an alternative leading to economic growth in logistics companies, making the world a better place in terms of carbon neutral (Khan et al., Citation2022). This also can be seen from the fact that a higher purchasing abilities from customers which ultimately leading to an economic growth, is influenced by the green capabilities of the company (Khan et al., Citation2022).
The presence of technology in logistics services companies does not only assist and provide ease for customers, but also can lead to improvement in other factors, such as economic factor due to an increase in sales, environmental factor due to the use of Internet of Things (IoT) making lower and effective energy usage, and social factor due to the efficient and effective work management (Khan et al., Citation2023).
3. Research method
Mixed methods, which include quantitative and qualitative research, were employed in this study. In order to produce more thorough, valid, dependable, and objective data for a study activity, mixed method research combines quantitative and qualitative methodologies (Johl et al., Citation2012).
3.1. Object
The objects of this study were logistics services companies in Indonesia. This study aims to explore the development of a sustainable logistics model for the logistics services industry in Indonesia. In this study, the independent variables were X1 or Economic Factor with several dimensions namely Capital and Cost Factors, Uncertainty and risk Management, Transportation Management, X2 or Customer Management with several dimensions namely Service Management, Information Technology Involvement, Customer Segmentation, X3 or Environmental Factor with several dimensions namely Internal Green Management, Resource Efficiency, Waste Reduction Commitment, X4 or Social Factor with several dimensions namely dimensi Commitment for employees, Commitment for Customers and the dependent variable (Y) was Sustainable Logistics.
3.2. Method
This study used a mixed method approach mixed method with four types namely embedded, explanatory, exploratory, triangulation (Özceylan & Paksoy, Citation2013). This study used explanatory type. Explanatory is a mixed-method study design that begins with conducting a quantitative study as the first stage and then verification is carried out in the second stage using sequential qualitative studies (Zhu et al., Citation2019). The explanatory study design can be seen in the following Figure .
4. Sample size
When it comes to the minimum sample size, there are several issues to consider. Simulation studies led to the suggestion of a minimum sample size of 100 (Anderson & Gerbing, Citation1984) showing the fail to converge with low sample size namely 50. There are two main things to consider namely based on chi-square test and fit indices (Hu & Bentler, Citation1999). The sample-to-independent-parameter ratio for SEM can be written as N:q to find out the optimal sample size (Jackson, Citation2001). However (Wolf et al., Citation2013), demonstrated that having more indicators per component results in reduced necessary sample sizes rather than bigger required sample sizes in general. Jackson’s findings reveal a very complicated set of interactions between magnitudes and N:q ratios, demonstrating that no simple principles exist. Therefore, it could be that the minimum sample size is 1:1 from N:q. Based on the analysis of the literature, the minimum number of samples used in this study was in accordance with a minimum number of indicators, namely 60 respondents, but the authors, to add to the richness of the data, taking as many as respondents up to 151 respondents with a minimum position of 5 years in the managerial field of a logistics company.
5. Data collection technique
Data were collected using questionnaire from January to August 2023. This study used Structural Equation Model (SEM) PLS, to be precise, its second-order as a form of measurement model in SEM with 2 levels demonstrating the correlation between first and second level. The general (structural) mathematical notation of the Hybrid Model is as follows:
Measurement model is as follows:
According to Bollen (Bollen, Citation1989), the relationship between first, second and higher order factors can be shown through EquationEquation (1)(1)
(1) . The Г£ component in EquationEquation (1)
(1)
(1) is not needed if higher order factors are part of η with their respective coefficients in B. As an alternative, Bη component in EquationEquation (1)
(1)
(1) is deleted if only second order factors are needed and there are no first-order factors that have a direct effect on each other (x = 0). First order loading factors from η on y is Λy. To obtain the entirety of multivariate model, whether its relationship or loading, Structural Equation Modeling (SEM) was used with the following steps:
5.1. Develop theoritical base model
The first stage in developing this model is to look for a model with a good rationale of the most important things. The SEM has no function to make a quality relationship but to justify theoretical causality through empirical test data (Ghayebloo et al., Citation2015). Changes in one variable are supposed to result in changes in other variables in structural equation models, which are based on causation. The theoretical rationale for the study, rather than the analytical method used, determines the strength of the causal link between the two variables postulated by the researchers. As a result, the link between variables in the model is a theoretical deduction. SEM cannot be utilized without a solid theoretical foundation.
5.2. Develop path diagram to describe causal relationships and translate into structural and measurement equations (structural and measurement equation)
After the theoretical relationship is formed, another method that can describe this relationship is through a path diagram. In creating a path diagram, arrows illustrate the connections between constructs. Straight arrows imply a direct causal link between two constructs. Only two-way arrows connecting constructions demonstrate a correlation between them. The construct in the path diagram is divided into two, namely Endogenous and Exogenous. Exogenous (X) is also known as the independent variable, while Endogenous (Y) is the dependent variable. After the theoretical model and path diagram are formed, the next step is to specify the model to a more formal view through equations. The aim is to link the operational definition of the construct to the theory for proper empirical testing.
5.3. Choose type of input matrix and proposed model estimation
This model differs from other methods for analysis in multivariables. However, variance or covariance matrices or correlation measurements are used as input data in SEM. Data for observation can be included in Smart PLS with a sample size of 85. Before calculating the covariance or correlation matrix, the outline data must be analyzed. There are two ways of analysis: estimating measurement model (between exogenous and endogenous) and estimating structural model (between exogenous) (Khan & Yu, Citation2021).
5.4. Structural model
Translating path diagrams to structural equations is a simple procedure. First, each endogenous construct is a separate dependent variable in the equation. Then, the predictor variables or (X) are all constructs in the straight arrow tails that point to the endogenous variables. The framework for the relationship between variables is as follows in Figure .
Figure 4. Conceptual framework for the relationship between latent variables.

Dependendent Variable
Sustainable Logistic (SL) (Y)Equation:
The number of variables in the sustainable logistics model (SL) consists of 4 Latent variables, 12 dimensions, and 60 indicators, as shown in Table (see Appendix).
5.5. Hypothesis
To test the hypothesis above, the following hypothesis testing is used:
Ho : γ2i = 0 : Insignificant influence of Xi on Y
H1 : γ2> 0 : Significant influence of Xi on Y
The statistical test used is as follows:
Reject Ho if t calculated > t table at α 5% significance level
Simultaneous hypothesis testing in SEM was carried out using Goodness of Fit (GOF), and not using F-Test. SEM simultaneous hypothesis testing with GOF is a substitute for path and path regression analysis. If the results of GOF testing in SEM are fit, then automatically the simultaneous model is fit. For partial testing, the t-test is still used. The t-calculated in SEM is the Critical Ratio (CR), Ho is rejected if CR > 1.96 at level 5% significance level (Kramer et al., Citation1988) as shown in Table .
Table 1. Hypothesis testing
6. Measurement model assessment
The reflecting measurement model assessment’s second phase examines internal consistency reliability between indicators. When evaluating the measurement model, the researcher must differentiate reflective or formative models; in this study, all variables are represented as reflective. The reliability, convergent, and discriminant validity of reflective latent variables are evaluated (Hair et al., Citation2019).
6.1. Reliability
Reliability is the assessment of the constructs’ internal consistency. A measurement is deemed to have high dependability if it continually produces the same results under the same conditions. SMART-PLS dependability is assessed using Composite dependability and Cronbach’s Alpha (Stefanic & Randles, Citation2015). The first criterion should be a Cronbach’s alpha analysis of the measurement model’s internal consistency reliability (Cronbach, Citation1951). J. F. Hair et al (Hair et al., Citation2019). argue that Cronbach’s alpha is a comparable internal consistency reliability test that yields less trustworthy findings than composite reliability. Cronbach’s alpha is a less accurate dependability measure since the components are not weighted. In contrast to Cronbach’s alpha, composite reliability loads the items based on the several build indicators, and the dependability is higher. The composite dependability rhoc, developed by Jöreskog in 1971, is one of the key metrics used in PLS-SEM. Higher degrees of dependability are associated with higher values. In exploratory research, for example, reliability levels between 0.60 and 0.70 are classified as “acceptable,” whereas reliability values between 0.70 and 0.90 are classified as “satisfactory to good.” Values more than 0.90 (and surely greater than 0.95) are problematic due to having redundancy (Hair et al., Citation2021). Because of the limits of Cronbach’s alpha, it is technically preferable to use an alternative measure of internal consistency reliability known as composite reliability (rhoC). However, both Cronbach’s alpha and rhoC have a cut-off value to assess reliability, which is above 0.7 (Hair et al., Citation2021; Henseler et al., Citation2015).
6.2. Convergent validity
Convergent validity aims to measure how far construct converges to account for the variation of its constituents. The statistic used to test a concept’s convergent validity is the average variance extracted (AVE) for all items on each construct (Hair et al., Citation2021). The average variance extracted (AVE) values serves the base for establishing convergent validity (Hair et al., Citation2019). To determine the mean value, each indicator loading on a construct must be squared to then be used in AVE. Furthermore, for the AVE to be regarded acceptable, AVE should be above 0.50 (Hair et al., Citation2021).
This criteria reveals how much an indicator is positively associated with other indicators under one construct. Indicators of reflective constructs are handled as distinct techniques to assessing the same construct using a domain sampling model. As a result, items used as indicators of an reflective construct must have a significant amount of variation. The researchers employ average variance extracted (AVE) with a cut-off value of 0.5 to assess the convergent validity of the reflective architecture, so that if AVE > 0.5, the model is declared valid (Sarstedt et al., Citation2021).
6.3. Discriminant validity
The discriminant validity of a construct measures its empirical difference from other things in the whole model (Hair et al., Citation2019). It experimentally analyzes how how distinct it is from other constructs (Gim & Cheah, Citation2020). Three techniques of evaluation have been offered (Hair et al., Citation2019).
Cross Loading
Discriminant validity reveals how empirically a construct varies from other constructs. Thus, proving discriminant validity indicates that a concept is distinct and captures things that other constructs in the model do not. Researchers have traditionally relied on two metrics of discriminant validity. Cross-loadings are commonly used to examine the discriminant validity of indicators. The projected cross-loading value should exceed 0.7 (Ghozali & Latan, Citation2015).
Fornell-Lacker
A second method for determining discriminant validity is the Fornell-Larcker criteria. This criteria compares the square root of the AVE value to the latent variable correlation. The square root of each construct’s AVE, in particular, must be bigger than its greatest correlation with the other constructs. An alternate method for analyzing the Fornell-Larcker criterion results is to see if the AVE is larger than the squared correlation with the other constructs. The theory of the Fornell-Larcker technique is based on the assumption that a construct’s variance with its related indicators is greater than that of any other construct. The connection with other constructs is larger than the predicted Fornell Lacker value.
Heterotrait-Monotrait Ratio Of Correlations (HTMT)
Cross-loading and the Fornell-Larcker Criterion, according to some experts, are less sensitive in determining discriminant validity. The recommended alternate approach for measuring discriminant validity is HTMT. The basis for measurement in this technique is a multitrait-multimethod matrix. To establish discriminant validity between the two reflective conceptions, the HTMT value must be smaller than 0.9 (Henseler et al., Citation2015).
7. Principal Component Analysis (PCA)
This statistical analysis can be called the Karhunen-Loeve or Hotelling transformation which is useful in simplifying components through linear transformations in order to get the best significant variance values, so as to be able to obtain reduced data with dense information or be able to describe the data as a whole (Lee et al., Citation1996).
The principal component analysis uses eigenvectors and values to reduce dimensions by eliminating correlations between independent variables. The process of eliminating correlation through eigenvectors and values is often called principal component transformation.
The Principal Component is obtained through several steps:
Subtract values with the average value in the form of a matrix
Calculate the covariance matrix
Get the eigenvector and value from the covariance matrix
The eigenvector with the highest eigenvalue is considered as the principal component (feature vector).
The feature vector matrix is transposed and then multiplied with the transposed data matrix
Mathematically it can be written as follows:
If the data matrix is considered Z and the metric matrix is N, then the transition matrix is as follows PCA consists of studying a data matrix Z, endowen with a metric Ip defined in Rp, and another metric N defined in Rn (generally N = (1/n) In. The matrix Z comes defined the following way:
The fit in Rn has to do with : N1/2ZZTN1/2v = λv, with vTv = 1
The transition relations can be written as:
Then to get the coordinates of each eigenvector element
ψα=Zuα
where
The coordinates of the active variables are obtained by the orthogonal projection of the columns of Z onto the directions defined by vα with the metric N. The coordinate values for obtaining the principal component are as follows
with the j-th element:
8. Linear Discriminant Analysis (LDA)
Linear Discriminant Analysis (LDA) aims to project the original data matrix into a lower dimension (Tharwat et al., Citation2017). To achieve this goal, several steps need to be taken, namely calculating the distance between the averages of the different classifications, which is called the inter-classification variance or the inter-classification matrix, and then formulating data with lower dimensions. Simply, the LDA process is carried out with the following formula (Izenman, Citation2008):
where
is called the between-class scatter matrix. This implies that
v1 a S−w1(m1 − m2)
and it can be computed from S−w1(m1 − m2) through rescaling
9. System dynamics
The system shows the unity of interaction between elements of an object within a certain scope working together to achieve goals. The intended interaction binds or connects elements so as to provide structure to the object, is able to differentiate from other objects, and influences the state of the object. The system dynamics is described by a flowchart with various functions with the aims and objectives which can be seen in the following table. In system dynamics, there is a boundary or scope separating the system and the external environment. This can be divided into two, namely a closed-loop system as a system free from the influence of the external environment and an open-loop system analyzing the influence of the external environment (Tian et al., Citation2014). Table (see Appendix) shows the flow diagram symbol and description.
Mathematically, system dynamics can be written as follows
10. Results and discussion
10.1. Formulating measurement model
In structural equation modeling, the measurement model measures the relationship between the observations obtained during the study (indicators) and the constructs or factors underlying the theory. The researcher assessed 60 indicators in the sustainability logistics model. The hypothesized measurement model determines the relationship between the 60 observed indicators and 12 latent variables supporting or influencing them. In this study, the measurement model used is as follows:
Economic Sustainability consists of several variables namely:
▪ Traffic Congestion Reduction (TRAFFIC) with 6 indicators
▪ Profitability Goals (PROFIT) with 7 indicators
▪ Financial Performance (FINANCE) with 5 indicators
Environmental Sustainability consists of several variables namely:
▪ Reducing Environmental Problems (ENVIRO) with 5 indicators
▪ Reducing Emissions (EMISSION) with 6 indicators
▪ Infrastructure Protection (INFRASTRUCTURE) with 5 indicators
Social Sustainability consists of several variables namely:
▪ Health Purposes (HEALTH) with 4 indicators
▪ Safety Purposes (SAFETY) with 5 indicators
▪ Employment Practices (EMPLOY) with 4 indicators
Customer Management Sustainability consists of several variables namely:
▪ Service Management (SERVICE) with 6 indicators
▪ Use of Information Technology (IT) with 4 indicators
▪ Customer Segmentation (SEGMENT) with 3 indicators
11. Model evaluation
The results of the reliability assessment with Cronbach’s alpha and rhoC and convergent validity with AVE can be seen as follows in Table .
Table 2. Reliability assessment
Based on the Table above, with Cronbach’s alpha and rhoC cutoff values > 0.7, all variables meet these criteria except Employment practices (Cronbach’s alpha < 0.7). Furthermore, AVE has a cutoff value > 0.5, so it is certain that several variables are not statistically valid, but the values are not too far away, such as Infrastructure Protection (0.455) and Safety Purposes (0.496). The expected cross-loading value is greater than 0.7, so if the cross-loading value is lower, it can be said that it does not meet discriminant validity. Another way besides cross-loading in determining discriminant validity is the Fornell-Lacker criteria as shown in Table .
Table 3. Fornell-Lacker test
Cross-loading and the Fornell-Larcker Criterion, according to some experts, are less sensitive in determining discriminant validity. The recommended alternate approach for measuring discriminant validity is HTMT. The HTMT value must be lower than 0.9 to be said to have discriminant validity as follows in Table .
Table 4. Heterotrait-monotrait Ratio assessment
In addition, it is also necessary to look at the relationship between variables with correlation analysis as follows in Table .
Table 5. Correlation assessment
The model results can be seen as follows in Table
Table 6. Model results
Validity testing on the latent variables of the sustainability logistics model is obtained for each latent variable, namely Customer, Environment, Economic, and Social. The standard loading factor value shows that these factors have a positive CFA value and the value is significant. Confidence interval (in this case 2.5% CI and 97.5% CI), shows how far and how big the influence of an indicator/variable is on other variables. So if there is a value of 0 between the two confidence interval values (between 2.5% CI and 97.5% CI), then it is considered to have no significant influence (Tan & Tan, Citation2010).The SEM matrix for the structural model is as follows.
In structural equation modeling (SEM), the measurement model measures the relationship between the observations obtained during the study (indicators) and the constructs or factors underlying the theory. Researchers assess the hypothesized measurement model determines the relationship between the observed indicators and latent variables. The structural model is predicting the relationship between latent variables, is able to test the overall model as well as individual paths, and is able to model error. Therefore, SEM matrix for the measurement model is as follows in Figure .
From the above model in Figure , it can be concluded that latent variables such as environmental, economic, social, and customer factors had a positive influence on sustainability logistics. Based on Table , the relationship between variables partially explained by the path coefficient value can be described as follows:
Hypothesis 1: The original sample, t-statistic, and p-value respectively were 0.23 (23%), 2.26 > 1.96, and 0,0 < 0.05. This shows positive as well as signfinicant influence between economic factors and social factors or it is accepted.
Hypothesis 2: The original sample, t-statistic, and p-value respectively were 0.76 (76%), 8,48 > 1.96, and 0,0 < 0.05. This shows positive as well as signfinicant influence between customer factors and social factors or it is accepted.
Hypothesis 3: The original sample, t-statistic, and p-value respectively were 0.29 (29%), 2.63 > 1.96, and 0.10 > 0.05. This shows positive but insignfinicant influence between customer factors and or it is accepted.
Hypothesis 4: The original sample, t-statistic, and p-value respectively were 0.56 (56%), 2.67 > 1.96 and 0.00 < 0.05. This shows positive as well as signfinicant influence between environmental factors and economics factors or it is accepted.
Hypothesis 5: The original sample, t-statistic, and p-value respectively were 0.01 (1%), 2.00 > 1.96, and 1.00. This shows positive but insignfinicant influence between economic factors and sustainable logistics or it is accepted.
Hypothesis 6: The original sample, t-statistic, and p-value respectively were −0,31 (31%), |-2.58| > 1.96, and 0.2. This shows negative and insignfinicant influence between customers factors and sustainable logistics or it is accepted.
Hypothesis 7: The original sample, t-statistic, and p-value respectively were 0.27 (27%), 2,7 > 1.96, and 0.3. This shows positive but insignfinicant influence between environmental factors and sustainable logistics or it is accepted.
Hypothesis 8:The original sample, t-statistic, and p-value respectively were 0.05 (5%), 2.5 > 1.96, and 0.3. This shows positive but insignfinicant influence between social factors and sustainable logistics or it is accepted.
12. Goodness of fit (GOF) test
Hair et al (Hair et al., Citation2019) and Schepsmeier (Schepsmeier, Citation2015) show that the final stage of the SEM analysis is the goodness of fit test. The goodness of fit can be tested with the absolute fit measurement, namely Chi Square and Standardized Root Mean Square Residual (SRMR). Chi-Square will be better if it has a lower value. The Chi-Square value is considered acceptable if the value is less than 2 times the degree of freedom. The degree of freedom value can be obtained from the number of variables minus one. Chi-Square can be calculated using the following formula:
Based on the calculation, the chi-square value obtained is 7.742486719, or less than 2 times the degree of freedom (N-1 or 61–1 = 60), so the model can be accepted.Standardized Root Mean Square Residual is the result of calculating the mean residual value as a result of matching the variance and covariance matrices. Hu and Bentler (Citation1999) showed that SRMR is a measure of absolute fit and is defined as the difference in observed and predicted correlation values with a limit of < 0.08. The formula is as follows:
p is the number of variables, Sij is the covariance, Sii and Sjj are the standard deviation
The calculation with RStudio can be performed with the following syntax.
Based on the calculations, the SRMR value obtained is 0.0871 or more than 0.08, although statistically the value is greater than 0.08, but with the distance/difference not being too large, the results can still be considered.
13. Discussion
13.1. The influence of economic factors on sustainability logistics model
The path coefficient hypothesis testing findings revealed that the economic variable has a positive influence with an estimated value of 0.128 (giving an influence of 13.8%) and is significant on sustainable logistics because it has a confidence interval value of 0.028 and 0.350. The results showed that economic factor had a positive and insignificant influence. The findings and conclusions in this study are in line with previous studies with the conclusion that although the transportation industry has historically been regarded as crucial for business activity, it now has a significant detrimental influence on the environment. As a result, complex optimization techniques are required to develop viable routes with minimal logistical costs (Reyes-Rubiano et al., Citation2021). The most important conclusion is that the studies highlighted are primarily focused on responsible consumption and production, industry, innovation, and infrastructure, as well as cheap and clean energy, with a secondary focus on sustainable cities and communities (Lazar et al., Citation2021). City logistics support urban economics and sustainable development, however, when demand develops, the negative externalities worsen (Škultéty et al., Citation2021).
13.2. The influence of customer factors on sustainability logistics model
The path coefficient hypothesis testing findings revealed that The customer variable has a positive influence with an estimated value of 0.129 (giving an influence of 12.9%) and is significant on sustainable logistics because it has a confidence interval value of 0.028 and 0.310. The results showed that economic factor had a positive and insignificant influence. The findings and conclusions of this study are in line with other studies which conclude that positive influences may represent positive influences on other dimensions of sustainability. Therefore, the challenge hinges on striving for positive influence (Lazar et al., Citation2021). As competition shifts from one company to the entire supply chain, the focus of the competitive market does not only stay on internal factor practices but also begins to focus on external factor practices (Wang et al., Citation2018). There are three dimensions to this factor, the first focuses on service sources, the second dimension produces services, and the third dimension focuses on product delivery, or between the first and second dimension.
13.3. The influence of environmental factors on sustainability logistics model
The path coefficient hypothesis testing findings revealed that environmental variables have a positive influence with an estimated value of 0.129 (giving an influence of 12.9%) and are significant on sustainable logistics because they have confidence interval values of 0.030 and 0.342. The results showed that environmental factors had a positive and insignificant influence. From an efficiency standpoint, the interaction between transportation and the environment has become one main part of sustainability (Lazar et al., Citation2021). Urban logistics is defined as the use of shipping management strategies that have been shown to affect traffic in urban settings. Therefore, the uniqueness of specific challenges as well as the urban setting must be considered (Škultéty et al., Citation2021). Logistics companies are encouraged to integrate sustainability concepts into their logistics activities due to environmental regulations and consumer pressures faced by logistics companies (Kumar, Citation2022).
13.4. The influence of social factors on sustainability logistics model
The path coefficient hypothesis testing findings revealed that The social variable has a positive influence with an estimated value of 0.142 (giving an influence of 14.2%) and is significant on sustainable logistics because it has a confidence interval value of 0.035 and 0.316. The results showed that social factors had a positive and insignificant influence. All smart city logistics systems attempt to use optimization techniques, technology improvements, and information and communication networks to make delivery system a flexible system capable of satisfying societal and industrial demands (Reyes-Rubiano et al., Citation2021). Social and industrial requirements are concerned not only with the economic impact of choices, but also with their social and environmental consequences, such as the impacts of pollution on the environment. The notion of selecting a development that fulfills present industrial and social demands while conserving resources for future generations gives rise to sustainability indicators. Synergies between social and environmental consequences are included in economic impacts (Alghababsheh & Gallear, Citation2021).
14. Principal Component Analysis (PCA)
After understanding the mathematical calculations, the PCA technique can be performed using the RStudio program, which results in values as shown collected in the form of variables in the accompanying tables collected.
Based on the Table above it can be concluded that:
The highest PCA value is found in the IT variable or “Use of Information Technology (Nagariya et al., Citation2022), (Kumar, Citation2022),(Nouri et al., Citation2019), with a value of 0.1514. This shows that the IT variable has the highest value in the variance/proportion determining the influences between variables.
The lowest PCA value is found in the INFRASTRUCTURE variable or “Infrastructure protection (Reyes-Rubiano et al., Citation2021), (Škultéty et al., Citation2021), with a value of 0.1110. This shows that the INFRASTRUCTURE variable has the lowest value on the variance/proportion determining the influences between variables.
Table 7. PCA value
Based on the Figure above, it is known that in the Sustainability Logistics Model questionnaire, of the 5 scales used, only 3 scales were the answers, namely scale 2 (not sustainable), scale 3 (fairly sustainable), and scale 4 (sustainable). The graph above shows that all factors prove to be supporting the Sustainability Logistics Model because almost all indicators in the plot are clustered and concentrated, with only a few outliers.
15. Linear Discriminant Analysis (LDA)
The actions that follow are utilized for performing out the LDA procedure using the RStudio software.
The graph above shows several indicators that are flanked by the two types of sustainability, including non-sustainable and fairly sustainable, non-sustainable and sustainable, and fairly sustainable and sustainable. There are even several indicators that are flanked by the three types of sustainability.Based on in Figure , in group 2 (Not Sustainable), the factor with the highest value or considered to have the most influence is “attention/consideration of the welfare of customers and society (Salamzadeh et al., Citation2022). In group 3 (Fairly Sustainable), the factor with the highest value or considered to have the most influence is “KIR test for vehicles (FGD Finding). In group 4 (Sustainable), the factors with the highest value or considered to have the most influence are “reducing emissions and waste (Reyes-Rubiano et al., Citation2021) and “regulations/commitment to road safety (Sodhi & Tang, Citation2018).
16. Simulation of sustainable logistics model
This study used the Sustainable Logistics model with four influencing sub-systems, namely: Economic Sustainability, Customer Sustainability, Environmental Sustainability, and Social Sustainability. Each sub-system has its own function which affects its condition with details as follows:
- Economic Sustainability can be explained by the Profit, Traffic, and Financial functions
- Customer Sustainability can be explained by the Service, IT, and Segmentation functions
- Environmental Sustainability can be explained by the functions of Environmental Problems, Emissions, and Infrastructure Protection
- Social Sustainability can be explained by the Health, Safety, and Employment functions
Causal Loop Diagram or often abbreviated as CLD refers to the characteristics of the system as mentioned in the previous section, namely the existence of supporting elements, internal and external feedback, and balance. CLD is a pre-condition before proceeding with the flow diagram process in the System Dynamics software, namely Powersim Studio 10.
A collection of cause-and-effect relationships forms a loop to provide feedback. The loop has two forms, namely Reinforcing (marked with the letter “R”) and Balancing (marked with the letter “B”). A reinforcing loop means mutually reinforcing relationships so that the interconnected variables will continue to increase or continue to decrease in value without a system balance. While the balancing loop means that the relationship provides a balance where the interrelated variables continue to increase and some continue to decrease so that it will provide system balance.Mathematically, by combining the coefficients in the SEM analysis, the system can be written as follows.
The relationship between variables with the flow diagram is as follows in Figure .
The results obtained using the Sustainable Logistics model simulation found that the MODEL VALUATION is as follows from 2020 to 2040 as shown in Figure .
Based on the graph and table above, the sustainable logistics model will have an increasing valuation every year. Thus, the relationship between variables in the sustainable logistics model is good in maintaining the company sustainability. It should be noted that the value of the rupiah unit (M) is not intended as a routine expenditure each year, but is used as an abstraction in the valuation of the sustainable logistics model, where if there is a continuous increase it is expected that in factual circumstances, the supporting factors will continue to have an increasing positive value so that it will maintain the company sustainability.
17. Conclusion
This study will be a novelty because there has not been a logistics performance measurement model integrating sustainable elements such as economic, environmental, social, and uncertainty risk elements that can influence sustainable logistics performance, namely consumer elements in logistics service companies in Indonesia, and no studies can provide comprehensive and specific analysis of the sustainable model through the development of sub-dimensions to explain the character of a general latent variable by considering several indicators influencing each sustainable factor as well as being equipped with real evidence with dynamic system simulations. Latent variables that make up the sustainability logistics model with positive and insignificant influences, namely economic factors, social factors, and environmental factors. Meanwhile, the customer factor had a negative or inverse effect and is not significant on the sustainability logistics model. However, all the indicators that make up the factors have a positive and significant influence. Based on the calculation, the chi-square value obtained is 7.742486719, or less than 2 times the degree of freedom (N-1 or 61–1 = 60), so the model can be accepted. Meanwhile, the SRMR value obtained is 0.0871 or more than 0.08, although statistically the value is greater than 0.08, but with the distance/difference not being too large, the results can still be considered. Based on SEM values, environmental variables have a positive and significant influence on Sustainable logistic, or it can be said that if environmental sustainability is improved, then sustainable logistic management will be better. Based on the SEM value, the customer variable has a positive and significant effect on Sustainable logistic, or it can be said that if customer sustainability is improved, then sustainable supply chain management will increase, which may be due to costs/costs used to improve facilities for customers that are getting bigger, so it is needed maximum limit/cost ceiling so that sustainability is maintained, economic variables have a positive and significant effect on Sustainable logistic, or it can be said that if economic sustainability is improved, then sustainable supply chain management will be better, which may be caused by wasted costs due to congestion, logistics costs, and accounting processes is getting bigger, so a maximum limit/cost ceiling is needed so that sustainability is maintained, social variables have a positive and significant influence on Sustainable logistic, or it can be said that if social sustainability is improved, then sustainable logistic will be better. The results of PCA showed that all factors prove to be supporting the Sustainability Logistics Model because almost all indicators in the plot are clustered and concentrated, with only a few outliers. The results of LDA showed that in group 2 (Not Sustainable), the factor with the highest value or considered to have the most influence is “attention/consideration of the welfare of customers and society, In Sustainable logistic group 2 (Fairly Sustainable), in group 3 (Fairly Sustainable), the factor with the highest value or considered to have the most influence is “KIR test for vehicles (FGD Finding)”, and in group 4 (Sustainable), the factors with the highest value or considered to have the most influence are “reducing emissions and waste” and “regulations/commitment to road safety”. In this study, Economic, Environmental, and Social Sustainability had a positive relationship because these three variables increased the company sustainability. On the contrary, Customer Sustainability had a negative relationship, if this variable continues to be increased without limitation it will reduce the company sustainability, for example, if the company continues to issue a budget for promotions without considering income, it will endanger the company’s financial condition. Based on the system dynamics analysis, the sustainable logistics model will have a valuation that continues to increase every year. Thus, the relationship between variables in the sustainable logistics model is good in maintaining the company sustainability. Therefore, companies should maintain their sustainability by improving factors with positive influence and paying attention to factor with negative influence. Further research can be carried out by conducting trials of model calculations based on sustainable logistics variables on the stability of a sustainable logistics business in logistics companies, especially in Indonesia.
Data availability statement
All data generated or analysed during this study are included in this published article (and its additional files). The ChIP-seq data have been deposited in the repository Politeknik APP database [eprint/3607] (https://repository.poltekapp.ac.id/id/eprint/3607). Requests for material should be made to the corresponding authors.
Disclosure statement
No potential conflict of interest was reported by the author(s).
Additional information
Funding
Notes on contributors

Teuku Yuri M. Zagloel
M. Tirtana Siregar is a doctoral student at the Faculty of Engineering at University of Indonesia (UI). Professor Dr. Ir. Teuku Yuri M. Zagloel, M.Eng.Sc. earned a doctorate degree at the University of Indonesia. He is currently working as a professor at the Doctoral of Industrial Engineering UI. His research interests are Total Quality Management and Manufacturing System. Prof. Dr. Akhmad Hidayatno ST. MBT. earned his doctoral degree at the University of Indonesia. He is currently working as a Professor at the Doctoral of Industrial Engineering UI. His research interests are System Modelling, Quality System, and Industrial Simulation. Dr. Andri Dwi Setiawan, ST. MSc. He received a doctorate degree from Eindhoven Univeristy of Technology, Netherlands. He is currently working as a management lecturer at UI. His research interests are Energy Management and Renewable Sustainable Energy Systems.
References
- Aksa, S. K., Adisasmita, S. A., Ramli, M. I., & Aly, S. H. (2020). Distribution intensity model of Retail logistics Outlets in metropolitan scale cities Based on spatial structures. Journal of Engineering and Applied Sciences, 15(1), 283–34. https://doi.org/10.36478/jeasci.2020.283.290
- Alghababsheh, M., & Gallear, D. (2021). Socially sustainable supply chain management and suppliers’ social performance: The role of social capital. Journal of Business Ethics, 173(4), 855–875. https://doi.org/10.1007/s10551-020-04525-1
- Anderson, J. C., & Gerbing, D. W. (1984). The effect of sampling error on convergence, improper solutions, and goodness-of-fit indices for maximum likelihood confirmatory factor analysis. Psychometrika, 49(2), 155–173. https://doi.org/10.1007/BF02294170
- Bollen, K. A. (1989). Structural equations with latent variables (Vol. 210). John Wiley & Sons.
- Cronbach, L. J. (1951). Coefficient alpha and the internal structure of tests. Psychometrika, 16(3), 297–334. https://doi.org/10.1007/BF02310555
- Dolinayova, A., & Cerna, L. (2019). The possibilities of increasing the economic efficiency of Regional Rail Passenger transport—A case study. Sustainable Rail Transport, 97.
- Ghayebloo, S., Tarokh, M. J., & Venkatadri, U. (2015). Developing a bi-objective model of the closed-loop supply chain network with green supplier selection and disassembly of products: The impact of parts reliability and …. Journal of Manufacturing Systems, 36, 76–86. https://doi.org/10.1016/j.jmsy.2015.02.011
- Ghozali, I., & Latan, H. (2015). Partial Least Squares Concepts, Techniques and Applications using the SmartPLS 3.0 Program (pp. 76–77). Diponegoro University Publishing Agency.
- Gim, G. C., & Cheah, W.-S. (2020). Pay satisfaction and organizational trust: An importance performance map analysis. Journal of Applied Structural Equation Modeling, 4(1), 1–16. https://doi.org/10.47263/JASEM.4(1)01
- Hair, J. F., Jr., Hult, G. T. M., Ringle, C. M., Sarstedt, M., Danks, N. P., & Ray, S. (2021). Partial least squares structural equation modeling (PLS-SEM) using R: A workbook. Springer Nature.
- Hair, J. F., Risher, J. J., Sarstedt, M., & Ringle, C. M. (2019). When to use and how to report the results of PLS-SEM. European Business Review, 31(1), 2–24. https://doi.org/10.1108/EBR-11-2018-0203
- Henseler, J., Ringle, C. M., & Sarstedt, M. (2015). A new criterion for assessing discriminant validity in variance-based structural equation modeling. Journal of the Academy of Marketing Science, 43(1), 115–135. https://doi.org/10.1007/s11747-014-0403-8
- Hosseini-Motlagh, S.-M., Nouri-Harzvili, M., Choi, T.-M., & Ebrahimi, S. (2019). Reverse supply chain systems optimization with dual channel and demand disruptions: Sustainability, CSR investment and pricing coordination. Information Sciences, 503, 606–634. https://doi.org/10.1016/j.ins.2019.07.021
- Hu, L. T., & Bentler, P. M. (1999). Cutoff criteria for fit indexes in covariance structure analysis: Conventional criteria versus new alternatives. Structural Equation Modeling: A Multidisciplinary Journal, 6(1), 1–55. https://doi.org/10.1080/10705519909540118
- Izenman, A. J. (2008). Modern multivariate statistical techniques (Vol. 1). Springer.
- Jackson, D. L. (2001). Sample size and number of parameter estimates in maximum likelihood confirmatory factor analysis: A Monte Carlo investigation. Structural Equation Modeling, 8(2), 205–223. https://doi.org/10.1207/S15328007SEM0802_3
- Johl, S. K., Bruce, A., & Binks, M. (2012). A study on the use of mixed method approach via sequential procedure to investigate corporate governance in corporate entrepreneurship among the 100 UK financial times stock exchange (FTSE) companies. African Journal of Business Management, 6(21), 6369. https://doi.org/10.5897/AJBM11.1771
- Kelly, E., Latruffe, L., Desjeux, Y., Ryan, M., Uthes, S., Diazabakana, A., Dillon, E., & Finn, J. (2018). Sustainability indicators for improved assessment of the effects of agricultural policy across the EU: Is FADN the answer? Ecological Indicators, 89, 903–911. https://doi.org/10.1016/j.ecolind.2017.12.053
- Khan, S. A. R., Tabish, M., & Yu, Z. (2023). Embracement of industry 4.0 and sustainable supply chain practices under the shadow of practice-based view theory: Ensuring environmental sustainability in corporate sector. Journal of Cleaner Production, 398, 136609. https://doi.org/10.1016/j.jclepro.2023.136609
- Khan, S. A. R., & Yu, Z. (2021). Assessing the eco-environmental performance: An PLS-SEM approach with practice-based view. International Journal of Logistics. https://doi.org/10.1080/13675567.2020.1754773
- Khan, S. A. R., Yu, Z., Umar, M., Zia-Ul-Haq, H. M., Tanveer, M., & Janjua, L. R. (2022). Renewable energy and advanced logistical infrastructure: Carbon-free economic development. Sustainable Development, 30(4), 693–702. https://doi.org/10.1002/sd.2266
- Kramer, M. S., McLean, F. H., Boyd, M. E., & Usher, R. H. (1988). The validity of gestational age estimation by menstrual dating in term, preterm, and postterm gestations. JAMA, 260(22), 3306–3308.
- Kumar, S. (2022). A quest for sustainium (sustainability premium): Review of sustainable bonds. Academy of Accounting & Financial Studies Journal, 26(2), 1–18.
- Lazar, S., Klimecka-Tatar, D., & Obrecht, M. (2021). Sustainability orientation and focus in logistics and supply chains. Sustainability, 13(6), 3280. https://doi.org/10.3390/su13063280
- Lee, H. W., Kim, J. I., Ban, J. S., & Min, B. W. (1996). Comparison morphine with meperidine in PCA intravenously for post-operative pain control after cesarean Section. Korean Journal of Anesthesiology, 31(1), 91–95. https://doi.org/10.4097/kjae.1996.31.1.91
- Lemaire, A., & Limbourg, S. (2019). How can food loss and waste management achieve sustainable development goals? Journal of Cleaner Production, 234, 1221–1234. https://doi.org/10.1016/j.jclepro.2019.06.226
- León, R., & Juan, A. A. (2014). Promoting corporate social responsibility in logistics throughout horizontal cooperation. Managing Global Transitions, 12(1), 79–93.
- MarkPlus, I. (2020). Frekuensi Penggunaan Jasa Kurir selama Pandemi Covid-19. Retrieved Desember 11, 2021 https://databoks.katadata.co.id
- Martins, V. W., Anholon, R., Quelhas, O. L., & Leal Filho, W. (2019). Sustainable practices in logistics systems: An overview of companies in Brazil. Sustainability, 11(15), 4140. https://doi.org/10.3390/su11154140
- Mollenkopf, D. A., Ozanne, L. K., & Stolze, H. J. (2020). A transformative supply chain response to COVID-19. Journal of Service Management, 32(2), 190–202. https://doi.org/10.1108/JOSM-05-2020-0143
- Murphy, P. R., & Knemeyer, A. M. (2018). Contemporary logistics. Pearson.
- Nagariya, R., Kumar, D., & Kumar, I. (2020). Service supply chain: From bibliometric analysis to content analysis, current research trends and future research directions. Benchmarking: An International Journal, 28(1), 333–369.
- Nagariya, R., Kumar, D., & Kumar, I. (2022). Sustainable service supply chain management: From a systematic literature review to a conceptual framework for performance evaluation of service only supply chain. Benchmarking: An International Journal, 29(4), 1332–1361. https://doi.org/10.1108/BIJ-01-2021-0040
- Nouri, F. A., Nikabadi, M. S., & Olfat, L. (2019). The role of supply chain features in the effectiveness of sustainability practices in the service supply chain: Application of fuzzy rule-based system. International Journal of Information Technology & Decision Making, 18(3), 867–899. https://doi.org/10.1142/S0219622019500111
- Özceylan, E., & Paksoy, T. (2013). A mixed integer programming model for a closed-loop supply-chain network. International Journal of Production Research, 51(3), 718–734. https://doi.org/10.1080/00207543.2012.661090
- Rahiminezhad Galankashi, M., & Mokhatab Rafiei, F. (2022). Financial performance measurement of supply chains: A review. International Journal of Productivity and Performance Management, 71(5), 1674–1707. https://doi.org/10.1108/IJPPM-11-2019-0533
- Reyes-Rubiano, L., Serrano-Hernandez, A., Montoya-Torres, J. R., & Faulin, J. (2021). The sustainability dimensions in intelligent urban transportation: A paradigm for smart cities. Sustainability, 13(19), 10653. https://doi.org/10.3390/su131910653
- Salamzadeh, A., Hadizadeh, M., Rastgoo, N., Rahman, M. M., & Radfard, S. (2022). Sustainability-oriented innovation foresight in international new technology based firms. Sustainability, 14(20), 13501. https://doi.org/10.3390/su142013501
- Sarstedt, M., Ringle, C. M., & Hair, J. F. (2021). Partial least squares structural equation modeling. In Handbook of market research (pp. 587–632). Springer.
- Schaltegger, S., & Burritt, R. (2014). Measuring and managing sustainability performance of supply chains: Review and sustainability supply chain management framework. Supply Chain Management: An International Journal, 19(3). https://doi.org/10.1108/SCM-02-2014-0083
- Schepsmeier, U. (2015). Efficient information based goodness-of-fit tests for vine copula models with fixed margins: A comprehensive review. Journal of Multivariate Analysis, 138, 34–52. https://doi.org/10.1016/j.jmva.2015.01.001
- Schöder, D., Ding, F., & Campos, J. K. (2016). The impact of e-commerce development on urban logistics sustainability. Open Journal of Social Sciences, 04(3), 1–6. https://doi.org/10.4236/jss.2016.43001
- Shi, M., & Yu, W. (2013). Supply chain management and financial performance: Literature review and future directions. International Journal of Operations & Production Management, 33(10), 1283–1317. https://doi.org/10.1108/IJOPM-03-2012-0112
- Singh, S., & Darroch, J. E. (2012). Adding it up: Costs and benefits of contraceptive services—estimates for 2012.
- Škultéty, F., Beňová, D., & Gnap, J. (2021). City logistics as an imperative smart city mechanism: Scrutiny of clustered eu27 capitals. Sustainability, 13(7), 3641. https://doi.org/10.3390/su13073641
- Sodhi, M. S., & Tang, C. S. (2018). Corporate social sustainability in supply chains: A thematic analysis of the literature. International Journal of Production Research, 56(1–2), 882–901. https://doi.org/10.1080/00207543.2017.1388934
- Somsuk, N., & Laosirihongthong, T. (2017). Prioritization of applicable drivers for green supply chain management implementation toward sustainability in Thailand. International Journal of Sustainable Development & World Ecology, 24(2), 175–191. https://doi.org/10.1080/13504509.2016.1187210
- Sroufe, R., & Gopalakrishna-Remani, V. (2019). Management, social sustainability, reputation, and financial performance relationships: An empirical examination of US firms. Organization & Environment, 32(3), 331–362. https://doi.org/10.1177/1086026618756611
- Stefanic, N., & Randles, C. (2015). Examining the reliability of scores from the consensual assessment technique in the measurement of individual and small group creativity. Music Education Research, 17(3), 278–295. https://doi.org/10.1080/14613808.2014.909398
- Sutoni, A., & Agustian, D. (2017). Penjadwalan Pengiriman Produk Kaos Oleh CV Chronicle Mart Kepada Sub Distributor Cianjur Dengan Mengunakan Metoda Drp (Distribution Requirement Planning). JURNAL MANAJEMEN INDUSTRI DAN LOGISTIK, 1(2), 137. https://doi.org/10.30988/jmil.v1i2.24
- Tan, S. H., & Tan, S. B. (2010). The correct interpretation of confidence intervals. Proceedings of Singapore Healthcare, 19(3), 276–278. https://doi.org/10.1177/201010581001900316
- Tharwat, A., Gaber, T., Ibrahim, A., & Hassanien, A. E. (2017). Linear discriminant analysis: A detailed tutorial. AI Communications, 30(2), 169–190. https://doi.org/10.3233/AIC-170729
- Tian, Y., Govindan, K., & Zhu, Q. (2014). A system dynamics model based on evolutionary game theory for green supply chain management diffusion among Chinese manufacturers. Journal of Cleaner Production, 80, 96–105. https://doi.org/10.1016/j.jclepro.2014.05.076
- Ubeda, S., Arcelus, F. J., & Faulin, J. (2011). Green logistics at Eroski: A case study. International Journal of Production Economics, 131(1), 44–51. https://doi.org/10.1016/j.ijpe.2010.04.041
- UNDP. (2015). Sustainable Development Goals (SDGs). Retrieved February 14, 2022 https://www.undp.org/sustainable-development-goals
- Vivaldini, M. (2016). Sustainable Logistical Operations: The Case of McDonald’s Biodiesel in Brazil [125-145]. https://api.elsevier.com/content/abstract/scopus_id/84948807869
- Wang, S., Tao, F., & Shi, Y. (2018). Optimization of location–routing problem for cold chain logistics considering carbon footprint. International Journal of Environmental Research and Public Health, 15(1), 86. https://doi.org/10.3390/ijerph15010086
- Wang, X., Yuen, K. F., Wong, Y. D., & Teo, C.-C. (2020). E-consumer adoption of innovative last-mile logistics services: A comparison of behavioural models. Total Quality Management & Business Excellence, 31(11–12), 1381–1407. https://doi.org/10.1080/14783363.2018.1485484
- Wolf, E. J., Harrington, K. M., Clark, S. L., & Miller, M. W. (2013). Sample size requirements for structural equation models: An evaluation of power, bias, and solution propriety. Educational and Psychological Measurement, 73(6), 913–934. https://doi.org/10.1177/0013164413495237
- Wong, L. W., Leong, L. Y., Hew, J. J., & Tan, G. W. H. (2020). Time to seize the digital evolution: Adoption of blockchain in operations and supply chain management among Malaysian SMEs. International Journal of Information Management, 52, 101997. https://doi.org/10.1016/j.ijinfomgt.2019.08.005
- Zhu, Y., Zhou, L., Xie, C., Wang, G., & Nguyen, T. (2019). Forecasting SMEs’ credit risk in supply chain finance with an enhanced hybrid ensemble machine learning approach. International Journal of Production Economics, 211, 22–33. https://doi.org/10.1016/j.ijpe.2019.01.032
Appendix
Table A1. Variables and indicators
Table A2. Flow diagram symbol and Description