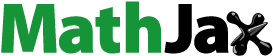
Abstract
Islamic financial instruments have been experiencing rapid growth in the last 50 years. Despite the unique motivation in formulating them, namely based on Syariah law, their movement might link to those of the conventional ones. This paper is devoted to investigating such interactions. It does so by applying two multivariate time series models to estimate various instruments, both Islamic and conventional ones. The models are the VAR (Vector Autoregression) and the VARMA-GARCH (Vector Autoregressive Moving Average-Generalized Autoregressive Heteroskedasticity). From the VAR model it finds evidence of bidirectional influences across both instruments. It also finds a conventional stock index that dominates the other series, namely the DJI (Dow Jones Index). From the VARMA-GARCH model, it finds influences from the conventional to Islamic index and vice versa, both in conditional mean and conditional variances. This paper suggests that the behavior of Islamic instruments are inseparable from the conventional ones. Future research might consider conditional correlations across these variables.
Subjects:
PUBLIC INTEREST STATEMENT
Islamic stock indices have some unique characteristics in terms of the companies included in the index, as well as the issues of Muslim loyalties who buy the stocks. These have raised questions of whether the stock indices have some correlation, comovement, or causality relationship with the conventional stock indices. This paper is meant to answer some parts of the questions. To be precise, this paper models the causality relationship across such indices. The results will be beneficial in some ways, such as the possibility of constructing a portfolio by combining both types of indices or predicting the behavior of Islamic stock indices based on the conventional ones, or vice versa. It finds evidence of bidirectional influences across both instruments. It also finds a conventional stock index that dominates the other series, namely the DJI (Dow Jones Index). This paper suggests that the behavior of Islamic instruments are inseparable from the conventional ones.
1. Introduction
Capital markets provide venues both for investment and speculation activities. The presence of Islamic instruments has provided more opportunities to do both. It also opens a new possibility in constructing an efficient portfolio that distributes the risk to minimize the individual risk. Investing in capital market would need to understand the behavior of instruments in such markets. This paper focuses on analyzing the interaction across Islamic and conventional instruments. Understanding such interaction would help to construct a portfolio that provides profit the most. Various articles have suggested such benefits (Kim & Sohn, Citation2016; Rahim & Masih, Citation2016).
The flow of information around the world provides the investors with various options of portfolio diversification to minimize risks based on real-time basis data tracking. Previous studies have provided a suggestion of strategies such as considering the interdependency and correlations among the stock markets (Rahim & Masih, Citation2016), combining Islamic stocks with the conventional ones (Kim & Sohn, Citation2016), and so on. Understanding the interaction of conventional and Islamic stocks provides a deeper analysis of why the investors should consider Islamic stocks on their portfolio and subsequently make it a more beneficial strategy to construct a portfolio that generates more benefits and minimizes the risks by combining and predicting the interbehaviour of both types of indices.
Despite the rapid growth of the Islamic economy, reaching 7% each year (Nurgalieva et al., Citation2018, p. 7), the portion of Islamic economy to conventional one is still shallow, namely 2.64%. Here is the calculation: the world GDP in 2020 is USD 91.98 trillion (World Population Review, Citation2020). In 2019, the world’s Islamic economy size was USD 2.2 trillion (Arabian Business, Citation2019). If the Islamic world economy grew by 7%, it could be expected that the statistic becomes USD 2.354. This means that in 2020, the Islamic economy is 2.6% of the world economy. This fact generates questions: despite their small number in economic magnitude, does the Islamic stock index influences the conventional ones. We would like to know as well whether the Islamic stock index is independent of the conventional counterpart.
There are a lot of papers that have investigated the nature of the relationship across both stock indices. Most of them include only stock indices, but some others consider economic variables such as Nazlioglu et al. (Citation2015) and Mensi et al. (Citation2017). Most papers use a multivariate model, while a small amount of them use a univariate model. Those who use the univariate model are Nazlioglu et al. (Citation2015), Rejeb (Citation2017), and Albaity and Ahmad (Citation2011). As predicted, multivariate models provide more straightforward steps in finding interdependencies across variables. However, univariate models are more flexible in including the independent variables, so they have a better chance to construct a theoretical model.
Various articles have estimated multivariate models to analyze both instruments, with or without the second-moment regressions. Those who do not consider the second-moment regressions are Ajmi et al. (Citation2014), who use Granger causality test, and Djedovic and Ergun (Citation2018), who use VAR and impulse response.
The articles that consider the second-moment regressions are Habibi and Pakizeh (Citation2017) who use VaR based on GED-GARCH (Generalized error distribution—GARCH); Mensi et al. (Citation2017) who use DECO-FIAPARCH (Dynamic Equicorrelation-Fractionally Integrated Asymmetric ARCH) by Engle and Kelly (Citation2012); Umar and Suleman (Citation2017) who use MVR-GARCH (Multi Variance-GARCH); and Toraman et al. (Citation2016) who uses multivariate GARCH. Some papers also accommodate dynamic conditional correlations such as Hashmi (Citation2018) and Majdoub and Mansour (Citation2014), who use DCC-GARCH (Dynamic Conditional Correlation-GARCH). Some articles find evidence of the asymmetric impact of a negative shock compared to positive counterparts such as Kareem (Citation2017) and Albaity and Ahmad (Citation2011).
This paper model the interactions across international stock indices, both Islamic and conventional ones. It uses a simple VAR model with the help of three techniques to interpret the results, namely the impulse response function, the forecast error variance decomposition, and the Granger causality test. It hopes that the results will reveal the true nature of the interactions across those instruments.
2. Methods
This paper uses time-series data of stock indices that represent both international conventional and Islamic stock indices. The variables are DJI (Dow Jones Industrial Average), DJII (Dow Jones Islamic Index), IMANX (Iman Fund Class K), NASD (Nasdaq Composite), and SP (S&P500). DJII and IMANX are selected to represent the international stock indices, and they are available in a reasonably long series. DJI, SP, and NASD are chosen to represent the global conventional stock indices. However, the model is more about simulation since there are no sound theories that link the index. For such purpose, this paper select a VAR (Vector Autoregressive) model of Sims (Citation1980) to estimate the empirical model. It is worth noted that VARs are not the ideal model for forecasting since it was not based on fully explicit economic theory (Sims, Citation1989). To better interpret the result, it considers Granger causality tests. The detail of the variables is in .
Table 1. Detail of variables in the model
This paper also considers estimating a VARMA-GARCH model of Ling and McAleer (Citation2003). This model accommodates the volatilities as well as the volatility spillovers across the indices.
VAR is a generalization of autoregressive models popularised by Sims (Citation1980). A VAR is a system regression model, namely. Let denote an (
) vector of time series variables. The basic p-lag vector autoregressive (VAR(p)) model is written as
where are
coefficient matrices and
is an
unobservable zero-mean white noise vector process (serially uncorrelated or independent) with a time-invariant covariance matrix. In this paper,
.
VAR modeling has some advantages. First, it does not need to specify which variables are endogenous or exogenous—all are endogenous. Second, it allows the value of a variable to depend on more than just its lags or combinations of white noise terms, so more general than ARMA modeling. Third, if there are no contemporaneous terms on the RHS of the equations, we can use OLS on each equation. Fourth, the forecasts that can be made from this model are often better than the traditional structural models.
VARMA-GARCAH model of Ling and McAleer (Citation2003) is a type of VAR model that accommodates the presence of volatility as well as volatility spillovers across variables in the model. A VARMA-GARCH model can be represented as follows:
where
,
and
are
matrices with typical elements
and
, respectively, for
is an
matrix,
and
are polynomials in
the lag operator,
is the past information available to time
is the
identity matrix, and
is an indicator function, given as:
In this model, .
Since the data are time series in nature, it is imperative that the paper test for the nature of stationarity of the variables. This paper will do so by conducting an ADF test for all the variables. However, since the variables are in terms of returns, they will likely be stationary in level. If that is the case, the paper will not need to construct a VECM (vector error correction model) to estimate the coefficients of the variables.
3. Results and discussion
The data of all variables, which are daily data with 5 days in a week, are presented in —the data spans from 5/11/2001 to 4/03/2020, which accounts for 7,754 observations. We can see from the graph that the data have positive trends, which is a sign that the series is nonstationary. Indeed, the Augmented Dickey–Fuller test results suggest that the series are nonstationary (see ).
Table 2. Unit root test, series in-level, ADF
This paper uses a series in terms of return to overcome a spurious estimation. For a series of Yt, the return (Rt) is calculated using the following formula:
where Yt and Yt−1 are Y at time t and Y at time t-1, respectively. The resulted series of returns can be seen in . The statistics of the return series are in . shows that the variables have constant means, which is a good sign of stationarity. We will use an Augmented Dickey–Fuller (ADF) test to confirm the stationary of the variables. The results are in . We can see that all variables are stationary in-level.
Table 3. Statistics of series in returns
Table 4. Unit root test, series in-returns, ADF
After testing the stationarity of the variables, this paper proceeds by estimating the VAR model. We let the data determine the lag length using various criteria such as AIC, SIC, and HQ. We start by estimating a VAR model using a maximum lag of 2. The result of the estimation is not presented in this paper to save space; the interested readers can get them from the author. To find whether the lag length is sufficient, the paper tests for the serial correlation. The result is in . Using a three lag of residuals, we can see that there is evidence of serial correlation, suggesting that the lag-length is not sufficient. The paper uses various criteria to find the appropriate lag length, as in .
Table 5. Serial correlation LM test for two lag length model
Table 6. VAR lag order selection criteria
shows that the HQ criterion suggests two lag-length, which we have seen to be not sufficient. SC criterion suggests even less lag length, namely one. AIC, as well as FPE, suggest seven lag-length, which this paper will use. The result of VAR estimation using lag of 7 is in . We have adjusted the table from the initial EViews output, wherein this table, each cell contains three numbers, from top to bottom one, namely the coefficient, t-statistic, and probability, respectively.
Table 7. VAR estimation, maximum of seven lags
Testing the autocorrelation of this model, we can see that only in lag four and eight that we cannot reject the null. It seems that overall, the model suffers from autocorrelation (see ). To overcome this problem, we will estimate a VARMA-GARCH model, assuming the autocorrelation is caused by the absence of volatility modeling in the system. This paper also tests for the system stationarity of the VAR model to avoid having spurious estimates (see ). From we can see that each modulus is less than 1, suggesting that the system is stationary, and the VAR satisfies the stability condition.
Table 8. Serial correlation LM test, maximum of eight lags
Table 9. System stationarity test
The estimation result of VAR in shows that DJI is influenced by all other variables; DJII is influenced by all variables except SP; IMANX is influenced by all variables, but it is not influenced by its own lag; NASD is influenced by all variables except IMANX, and SP is influenced by all variables. We can also say that DJI affects the other variables in three lags. DJII, IMANX, NASD, and SP affect the other variables only in lag 1. Therefore, we conclude that DJI is the dominant variable.
In addition to looking at the significance level in testing the significant influence of the independent variables on a particular dependent variable, we will confirm it using a Granger causality test.
4. Granger causality test
Causality in econometrics refers to the ability to predict. Econometricians refer to Granger causality in the sense that X is said to be a Granger cause of Y if current Y can be predicted with greater accuracy by using past values of X (see Thomas, Citation1997, p. 461). Granger causality is different from the causality measure given by an individual t-test in a way that the Granger causality test accommodates the influence of lags in the model. presents the test result. The table shows evidence of Granger causality from all variables to the others, except that SP does not Granger cause DJII, and that NASD does not Granger cause IMANX.
Table 10. Granger causality test (lags: 7)
The interaction between conventional to Islamic stock indices is supported by Abbes and Trichilli (Citation2015), although it tends to change over time. The support also comes from Ajmi et al. (Citation2014), who refute the longstanding decoupling hypothesis about Islamic financial markets. However, a different view is given by Rizvi et al. (Citation2015). They found that although the authors show that most of the economic downturns since 1996 can be attributed to excessive linkages from the US to the Asia Pacific, the real sector grounded Islamic markets exhibit a reduced exposure to crises. This indicates a weak interaction between conventional instruments and Sharia-based instruments. But the upside is that the Islamic stock market can be a buffer for economic crises, as well as a vehicle for spreading the risk.
5. VARMA-GARCH estimation
As previously mentioned, this paper estimates a VARMA-GARCH model of Ling and McAleer (Citation2003). This paper estimates three models, namely VARMA-GARCH with a lag of 1, 2, and 3, using EViews 9.0 software package. However, when estimating the model with a lag of 3, the software was not able to provide a result. This might be because the estimated coefficient was to many. This is known as the curse of dimensionality.
To choose which model is more appropriate, we use the Akaike Information Criterion, Schwartz Criterion, and Hannan–Quinn Criterion. The results are in . Based on the AIC, SC, and HQC, it seems that VARMA-GARCH with lag 2 is more appropriate than VARMA-GARCH with lag 1, except for IMANX, in which VARMA-GARCH with lag 1 is the more appropriate one, even though the difference between the criteria is very small. Therefore, we will use lag 2 as the basis for analysis, and in addition, we will compare the result of both lag for the IMANX estimation.
Table 11. Finding the most appropriate lag length in VARMA-GARCH models
The estimation output of the VARMA-GARCH with a lag of 2 is available in the Appendix. The estimation output of the VARMA-GARCH with a lag of 1 is available upon request to the authors.
The results of VARMA-GARCH lag two estimations are available in the Appendix (). The summary of the mean and volatility spillovers from the VARMA-GARCH lag two estimations is in .
Table 12. Summary of mean and volatility spillovers in a VARMA-GARCH lag 2 model
shows that, in terms of conditional mean, NASDAQ influences all variables except IMANX. Therefore, we can say that NASDAQ dominates the influences. We also find the influence of conventional indices to Islamic indices, namely from DJI to DJII and NASDAQ to DJII. We also find the influence of Islamic indices to conventional indices, namely from IMANX to both NASDAQ S&P500, and from DJII to S&P500. In addition, we find the influence across Islamic indices, namely from DJII to IMANX. We can conclude as well that the results of Granger that SP does not Granger cause DJII, and that NASD does not Granger cause IMANX, are supported by the result of VARMA-GARCH.
also shows that, in terms of conditional variances, IMANX influences all variables. Therefore, we can say that IMANX dominates the influences. We do not also find any influence from conventional indices to Islamic indices. We see the influence of Islamic indices to conventional indices, namely from IMANX to all other variables. In addition, we find the influence across Islamic indices, namely from IMANX to DJII.
The evidence of volatility spillovers across conventional and Islamic indices is supported by Muharam et al. (Citation2020), but only for cases in developed countries. Meanwhile, Majdoub and Mansour (Citation2014) do not provide strong evidence of US market spillovers into the Islamic emerging equity markets. In addition, there is evidence of weak correlation across the Indonesian market and the developed markets for both conventional and Islamic stock prices, suggesting the possibility of portfolio diversification. Another paper by Ben Nasr et al. (Citation2014) suggests the possibility of Islamic instruments as a venue to diversify risk. In addition, Saiti et al. (Citation2014) found that Islamic countries can be better diversification venues compared to Far East countries. However, Majdoub et al. (Citation2016) suggest a high connection between the developed markets for both conventional and Islamic indices. This can demotivate investors to use Islamic instruments as a potential venue to diversify financial risk.
6. Conclusion
This paper estimates a VAR model to analyze the mean interactions across international conventional and Islamic stock indices. It uses the Granger causality test to help confirm the result. This paper also estimates a VARMA-GARCH model of Ling and McAleer (Citation2003) to accommodate the presence of volatility and volatility spillovers across the indices,
The VAR estimation results suggest evidence of the influence across the indices, except for two cases, namely from the SP to DJII and from IMANX to NASDAQ. The estimation results of the Granger causality test also suggest similar results, namely that there is evidence of the influence of each index to the other index, except for two cases, namely from the DJI to SP to and from NASDAQ to IMANX.
The results of the VARMA-GARCH provide more limited evidence about the effect of one index to another. However, it can be said that there are influences of conventional indices to Islamic indices, namely from DJI to DJII and NASDAQ to DJII. We also find the influence of Islamic indices to conventional indices, namely from IMANX to both NASDAQ and SP, and from DJII to SP. In addition, we find the influence across Islamic indices, namely from DJII to IMANX. We can conclude as well that the results of Granger that the SP does not Granger cause DJII, and that NASD does not Granger cause IMANX, is supported by the result of VARMA-GARCH.
From the estimation result, this paper suggests that to construct an efficient portfolio, namely a portfolio which offers the highest expected return for a given level of risk or a portfolio with the lowest level of risk for a given expected return, one should consider including Islamic indices into it. However, one should not treat the Islamic indices with the same manner, because the behavior of an Islamic index might be different from the other, especially in its relation to the conventional ones.
Acknowledgements
The authors acknowledge the funds provided by the Economics Department, Faculty of Business and Economics, Universitas Islam Indonesia, 2019.
Additional information
Funding
Notes on contributors

Abdul Hakim
Abdul Hakim is an Associate Professor at the Department of Economics, UII. He has published papers in Applied Economics and Mathematics and Computers and Simulations.
Awan Setya Dewanta is a lecturer at the Department of Economics, UII. He has published his papers in the Asian Journal of Innovation and Entrepreneurship and Economic Journal of Emerging Markets.
Sahabudin Sidiq is a researcher and currently the chief of the Economics Department at UII. He has published papers in various international journals such as the Economic Journal of Emerging Markets and Asian Journal of Innovation and Entrepreneurship.
Riska Dwi Astuti is a lecturer at the Department of Economics, UII. She has published her papers in the Journal of Developing Economies and Annals of Nutrition and Metabolism.
References
- Abbes, M. B., & Trichilli, Y. (2015). Islamic stock markets and potential diversification benefits. Borsa Istanbul Review, 15(2), 93–23. https://doi.org/10.1016/j.bir.2015.03.001
- Ajmi, A. N., Hammoudeh, S., Nguyen, D. K., & Sarafrazi, S. (2014). How strong are the causal relationships between Islamic stock markets and conventional financial systems? Evidence from linear and nonlinear tests. Journal of International Financial Markets, Institutions & Money, 28(1), 213–227. https://doi.org/10.1016/j.intfin.2013.11.004
- Albaity, M., & Ahmad, R. (2011). Return performance, leverage effect, and volatility spillover in Islamic stock indices evidence from DJIMI, FTSEGII and KLSI. Investment Management and Financial Innovations, 8(3), 161–171. https://businessperspectives.org/author/mohamed-albaity
- Arabian Business. (2019). ITP Media Group, Dubai, Uni Arab Emirates. https://www.arabianbusiness.com/politics-economics/432979-22trn-the-size-of-the-worlds-islamic-economy
- Ben Nasr, A., Ajmi, A. N., & Gupta, R. (2014). Modeling the volatility of the Dow Jones Islamic market world index using a fractionally integrated time varying GARCH (FITVGARCH) model, 24(14), 993–1004. https://doi.org/10.1080/09603107.2014.920476
- Djedovic, I., & Ergun, U. (2018). Modeling volatility spillover between conventional and Islamic index in the United Kingdom. Journal of Management, Economics, and Industrial Organization, 2(3), 1–17. https://doi.org/10.31039/jomeino.2018.2.3.1
- Engle, R. F., & Kelly, R. (2012). Dynamic equicorrelation. Journal of Business Economics and Statistics, 30(2), 212–228. https://doi.org/10.1080/07350015.2011.652048
- Faal, E. (2007). Growth, investment and productivity in Papua New Guinea. Pacific Economic Bulletin, 22(1), 16–38. http://devpolicy.org/peb/2019/06/29/growth-investment-and-productivity-in-papua-new-guinea/
- Habibi, S., & Pakizeh, K. (2017). Risk spillover from U.S. and Japan to Iran’s Stock Market. European Journal of Economics, Finance and Administrative Sciences, 96, 38–48. http://www.europeanjournalofeconomicsfinanceandadministrativesciences.com/issues/PDF/EJEFAS_96_03.pdf
- Hashmi, O. (2018). Conventional vs. shariah stock indices: Volatility, financial contagion, interest rate risk and gold as safe haven [Master’s Thesis]. Charles University Faculty of Social Sciences Institute of Economic. https://www.semanticscholar.org/paper/Conventional-vs.-Shariah-stock-indices%3A-Volatility%2C-Hashmi/e2fb5eecf19d92e8e8ae45bff1a95aaa9844070e.
- Kareem, U. (2017). Volatility between conventional and Islamic stock market: Evidence from quantile regression analysis [Master’s thesis]. Capital University of Science & Technology. https://vdocuments.mx/volatility-between-conventional-and-islamic-stock-market-kareemmerciful.html
- Kim, H. B., & Sohn, T. (2016). Volatility transmission between conventional finance and Islamic finance in stock markets. Macrotheme Review, 5(4), 1–12. http://macrotheme.com/yahoo_site_admin/assets/docs/1MR54Ki.145120148.pdf
- Ling, S., & McAleer, M. (2003). Asymptotic theory for a vector ARMA-GARCH model. Econometric Theory, 29(2), 280–310. https://doi.org/10.1017/S0266466603192092
- Majdoub, J., & Mansour, W. (2014). Islamic equity market integration and volatility spillover between emerging and US stock markets. The North American Journal of Economics and Finance, 29, 452–470. https://doi.org/10.1016/j.najef.2014.06.011
- Majdoub, J., Mansour, W., & Jouini, J. (2016). Market integration between conventional and Islamic stock prices. The North American Journal of Economics and Finance, 37, 436–457. https://doi.org/10.1016/j.najef.2016.03.004
- Mensi, W., Hammoudeh, S., Al-Jarrah, I. M. W., Sensoy, A., & Kang, S. H. (2017). Dynamic risk spillovers between gold, oil prices and conventional sustainability and islamic equity aggregates and sectors with portfolio implications. Energy Economics, 67, 454–475. https://doi.org/10.1016/j.eneco.2017.08.031
- Muharam, H., Wahyudi, S., Pangeastuti, I. R. D., & Najmudin, N. (2020). Interaction of Islamic and conventional stock markets and the economic connectivity. Journal of Advanced Research in Law and Economics, 9(2), 591–602. https://journals.aserspublishing.eu/jarle/article/view/2486
- Nazlioglu, S., Hammoudeh, S., & Gupta, R. (2015). Volatility transmission between Islamic and conventional equity markets: Evidence from causality-in-variance test. Applied Economics, 47(46), 4996–5011. https://doi.org/10.1080/00036846.2015.1039705
- Nurgalieva, G., Kalinin, A., Nureev, B., Imanova, G. and Bapakhova, A.. (2018). The Islamic economy – The fastest growing large economy, Skolkovo Institute for Emerging Market Studies, Moskow. https://islamicbankers.files.wordpress.com/2019/02/ey-the-islamic-economy-in-eurasia-focus-2018.pdf.
- Rahim, A. M., & Masih, M. (2016). Portfolio diversification benefits of Islamic investors with their major trading partners: Evidence from Malaysia based on MGARCH-DCC and wavelet approaches. Economic Modeling, 54, 425–438. https://doi.org/10.1016/j.econmod.2015.12.033
- Rejeb, A. B. (2017). On the volatility spillover between Islamic and conventional stock markets: A quantile regression analysis. Research in International Business and Finance, 42, 794–815. https://doi.org/10.1016/j.ribaf.2017.07.017
- Rizvi, S. A. R., Arshad, S., & Alam, N. (2015). Crises and contagion in Asia Pacific – Islamic v/s conventional markets. Pacific-Basin Finance Journal, 34, 315–326. https://doi.org/10.1016/j.pacfin.2015.04.002
- Saiti, B., Bacha, O. I., & Masih, M. (2014). The diversification benefits from Islamic investment during the financial turmoil: The case for the US-based equity investors. Borsa Istanbul Review, 14(4), 196–211. https://doi.org/10.1016/j.bir.2014.08.002
- Sims, C. A. (1980). Macroeconomics and Reality. Econometrica, 48(1), 1–48. https://doi.org/10.2307/1912017
- Sims, C. A. (1989). Models and their uses. American Journal of Agricultural Economics, 71(2), 489–494. https://doi.org/10.2307/1241619
- Thomas, R. L. (1997). Modern econometrics. Addison-Wesley.
- Toraman, C., Igde, M., Bugan, M. F., & Kilic, Y. (2016). Volatility spillover effect from conventional stock markets to Islamic stock markets. International Journal of Academic Research in Economics and Management Sciences, 5(4), 264–285. https://doi.org/10.6007/IJAREMS/v5-i4/2521
- Umar, Z., & Suleman, T. (2017). Asymmetric return and volatility transmission in conventional and Islamic equities. Risks, 5(2), 1–18. https://doi.org/10.3390/risks5020022
- World Population Review. (2020). GDP ranked by Country, 2020. World Population Review. https://worldpopulationreview.com/countries/countries-by-gdp/.
Appendix.
The Result of a VARMA-GARCH model with lag of 2
Table A1. Mean estimation, dependent variable: DJI
Table A2. Variance estimation, dependent variable: DJI
Table A3. Mean estimation, dependent variable: DJII
Table A4. Variance estimation, dependent variable: DJII
Table A5. Mean Estimation, dependent variable: IMANX
Table A6. Variance estimation, dependent variable: IMANX
Table A7. Mean estimation, dependent variable: NASDAQ
Table A8. Variance estimation, dependent variable: NASDAQ
Table A9. Mean estimation, dependent variable: SP
Table A10. Variance estimation, dependent variable: SP