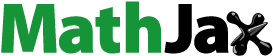
Abstract
The main challenge with respect to water in the rural setting, lies in access, control and management. Collective action has been taken up following the International Water Management (IWM) principles and institutionalized in the Kenyan legal framework through water resource users’ associations (WRUAs). We carried out this study to assess whether this collective action has any impact on household poverty using objective poverty measures (consumption and income), a subjective poverty measure and a water poverty measure. We used 2019 household survey data of 652 randomly selected rural households from the Upper Ewaso Ng’iro North Catchment Area. We employed the full information maximum likelihood endogenous probit regression model to obtain the impact of WRUA membership on household poverty status. We find that collective water management can have welfare improving impacts for rural households, especially where there low public investments in water provision, management and access. We recommend that WRUAs be empowered through financial, legal and capacity building interventions to enhance their community impacts.
PUBLIC INTEREST STATEMENT
“Water is life” is a well-known saying. The relationship between water access, poverty and development is quite intricate. For instance, according to the Food and Agriculture Organization (FAO), “3.2 billion people live in agricultural areas with high to very high water scarcity, of whom 1.2 billion people – roughly one sixth of the world’s population – live in severely water-constrained agricultural areas.” There is therefore an urgent need to manage the diminishing water resources sustainably to meet the needs of economic agents. Among the key water management strategies; Community-based natural resource management approaches have gained prominence, since the users are tasked with governing the natural resources at their disposal in a localized institutional framework. This study sought to evaluate the impact of this local water governance framework on household poverty. The findings show that localized water governance could offer pathways for rural households to escape poverty.
1. Introduction
Many rural poor households rely on the environment for key inputs in their production and consumption activities (Angelsen et al., Citation2014; Barbier, Citation2010). Water is perhaps the most important of these inputs, since all households require water for domestic use, irrigation and raising livestock (FAO, Citation2008; Seckler & Amarasinghe, Citation2000). However, the main challenge with respect to water in the rural setting, lies in access, control and management (FAO, Citation2008; Republic of Kenya, Citation2007). Whereby, most small-holder farmers lack sufficient power over water access, control and management. According to Republic of Kenya (Citation2007) the coverage of water in rural areas is insufficient and is estimated at around 40%, characterized by non-piped systems with multiple challenges of sustainability, reliability, water quality, and limited community ownership. In order to achieve water access, control and management, small-holder farmers join into community water development projects (CWDPs) and as a group obtains WRUA (Water Resource Users Association) membership. According to Suhardiman et al. (Citation2017) collective action is now recognized as central to addressing the water governance and management challenges. This is because collective action can strengthen the bargaining power of smallholders relative to other producers of environmental services and buyers of environmental services (Swallow et al., Citation2005).
The Kenyan water policy framework recognizes WRUAs as the legal, grassroots collective action organ entrusted with water resources management, allocation and control through the Water Act 2002 and Water Act 2016. Prior to the enactment of the Water Act 2002, local communities were managing water resources through informal groups. After the enactment of the legislation, the formation and registration of WRUAs have intensified. The key objectives of WRUAs include promotion of controlled and legal water use activities; good management practices that make efficient and sustainable use of water resources; the safeguarding of environmental flows for downstream ecological demands and basic human needs; the reduction of water use conflicts; and catchment conservation measures to improve water quantity and quality (Richards & Syallow, Citation2018; WRMA., WSTF, Citation2014).
Several studies have shown evidence where individual farmers prefer not to be WRUA members for different reasons, especially, where there is a negative relationship between elite capture and users’ satisfaction (Agrawal, Citation2002; Kadirbeyoğlu & Özertan, Citation2011; Richards & Syallow, Citation2018). Two key questions that arise are: did the Water Act create an avenue for the elites to capture smallholder households for their own predetermined ends or otherwise? And do smallholder rural households perceive WRUAs as hindrances to their escape from poverty and disempowerment? The only way to answer these questions, is through an empirical study at the household level, since economic theory shows that households as economic agents are rational and will engage/adopt/participate in policy interventions that maximize their utility and overall wellbeing. On the other hand, there is an inverse relationship between profit/welfare maximization and environmental conservation efforts as evidenced by different environmental development nexus models, key among them the Environmental Kuznets Hypothesis (Dinda, Citation2004; Horii & Ikefuji, Citation2014; Kijima et al., Citation2010).
Poverty takes several dimensions including, absolute, relative, situational, generational, rural, and urban poverty. The importance of rural poverty stems from the fact that poverty tends to be higher in rural areas and presents different characteristics (Cardoso & Teixeira, Citation2020; Lekobane & Seleka, Citation2017; Majeed & Malik, Citation2015) as compared to urban areas. For instance, the comprehensive poverty report for Kenya shows that a third of the country’s population are poor. Further, the poverty incidence in rural areas stands at 40% as compared to that of urban areas at 29% (KNBS, Citation2020). The duality presented by urban and rural poverty creates a problem for policy makers, since it limits the prospects for growth, by demanding two sets of interventions to tackle poverty at the urban and rural settings.
Previous studies show that some of the key causes of rural poverty include high dependence on agriculture, which has low productivity and low value added (Quang Dao, Citation2004); vulnerability of agriculture to weather and climate shocks (Börner et al., Citation2015); and the relative isolation of rural areas from important service delivery institutions, markets and infrastructure (Stifel & Minten, Citation2008). According to Bertolini (Citation2019), rural poverty is transmitted by the cumulative negative effects of the vicious circle of labour market, demography, education, and remoteness. However, it is important to note that the link between agricultural water management and poverty can be extremely variable and complex (Barbier, Citation2010; Castillo, Citation2007), depending on the context for two reasons as expounded by Johnson et al. (Citation2009). First, despite water being an important resource for household welfare, opportunities for reducing poverty by increasing the quantity or quality of water available to the poor may be limited. And secondly, while improved watershed management may have limited direct benefits in terms of poverty alleviation, there are important indirect linkages between watershed management and poverty, mainly through labour and service markets. We therefore based our context to focus on rural small-holder farmers, since majority of Kenya’s farmers are smallholder farmers.
Rural poverty has been studied in different aspects including; the determinants of rural poverty (Hassan & Babu, Citation1991; De Janvry & Sadoulet, Citation2000; Lekobane & Seleka, Citation2017; López & Valdés, Citation2000; Malik, Citation1996), effects of rural poverty (Bertolini, Citation2019; Cali & Menon, Citation2013; Chaudhry et al., Citation2006; Ravallion & Sen, Citation1994). Water and rural poverty (Garriga & Foguet, Citation2013; Hope, Citation2006; John & Firth, Citation2005; Madulu, Citation2003). Further, numerous studies have demonstrated that agricultural water management and irrigation, are key for poverty alleviation (Burney & Naylor, Citation2012; Huang et al., Citation2006; Hussain & Hanjra, Citation2004; Mwangi & Crewett, Citation2019; Van Koppen, Citation1998). Finally, several studies have looked into the link between collective action in the management of natural resources and poverty (Adhikari, Citation2005; Di Gregorio et al., Citation2008; Johnson et al., Citation2009).
The key issue that arises in most poverty studies is usually the question of poverty measures. Poverty can be measured objectively or subjectively. Subjective’ measures of poverty are where the research participants themselves are asked to rate their own position in the socio-economic hierarchy, in contrast to “objective” measures where the researcher determines the individual or household’s poverty based on data (Carletto & Zezza, Citation20066; Kullenberg & Nelhans, Citation2020; Ravallion, Citation2012; Ravallion et al., Citation2013; Yang, Citation2018). To allow for comparison, we utilized four rural poverty dimensions including; objective measures (consumption poverty and income poverty); a subjective measure (Economic Ladder Question); and a water poverty measure (derived from a household water security index), so as to further the knowledge on the relationship between collective agricultural water management and rural poverty using the case of smallholder farmers in rural Kenya.
1.1. Literature review
While few studies have assessed the impacts of water-related collective action on household welfare, the findings are contrasting. Mwaura et al. (Citation2020) analyzed the impact of WRUA membership on household welfare, using consumption per adult equivalent and income per adult equivalent. The findings showed that non-members could have significant increases in household welfare if they considered WRUA membership. McCarthy and Essam (Citation2009) analyzed the impact of water user associations (WUAs) on agricultural productivity in Chile, using combined household and community level data collected from the Maule Region to evaluate the factors affecting the decision to participate in yearly irrigation maintenance activities, and the influence of current behavior on farm revenues. Empirical results indicate that water user association characteristics explain much of the variation in participation decisions, contribution amounts, variable input purchases, and subsequent farm revenues. Shiferaw et al. (Citation2008) assessed the state of water-related collective action and its effects on natural resources and rural livelihoods in India. Factor analysis was used to develop aggregate indices of collective action and its effectiveness. Regression methods were then employed to test the effects of certain policy relevant variables and to determine the potential effects of collective action in achieving desired poverty reduction and resource improvement outcomes. Results showed a positive and highly significant effect of collective action on natural resource investments, but no evidence of its effects on household assets and poverty reduction outcomes.
Sinyolo and Mudhara (Citation2018) undertook a study on collective action and rural poverty reduction in Kwa-Zulu Natal, South Africa. The study investigated whether collective action through farmer groups improved incomes among rural households. The study used the propensity score matching (PSM) method and the treatment approach to analyze data collected from a sample of 984 households, where the total household income per adult equivalent was used as the welfare indicator. The PSM results indicated that participation in farmer groups significantly and positively influenced household incomes. The results also indicated that groups benefit more those who are educated and are males, suggesting a bias against the females and those less educated. From the empirical review, there is a limited scope of studies detailing the impacts of water-related collective action to household welfare. The findings of this study will help close this gap in the literature and also inform water policy.
This study will add to this rich body of literature by treating rural poverty as a set of different dimensions of poverty that include objective poverty (consumption and income poverty), subjective poverty and water poverty. The findings of this study will be useful for policy makers and development partners at the local, national, regional and international levels to relook at rural poverty with a different lens when designing strategies to tackle rural poverty, especially at the river basin level. We seek to identify whether collective management of water translates into less rural poverty or otherwise. We therefore aim to test the following hypothesis;
There is a positive relationship between household consumption poverty and WRUA membership.
There is a positive relationship between household income poverty and WRUA membership.
There is a positive relationship between household subjective poverty and WRUA membership.
There is a positive relationship between household water poverty and WRUA membership.
2. Materials and methods
2.1. Survey data
The study was undertaken in the Upper Ewaso Ng’iro North Catchment Area (ENNCA), which is the catchment area for the Ewaso N’giro River basin. The Ewaso N’giro River basin is the largest basin in Kenya (Ewaso Ng’iro North River Basin Development Authority (ENNDA, Citation2019)). According to Mungai et al. (Citation2004) the Upper Ewaso Ng’iro North Basin is located to the north and west of Mount Kenya, extending to the Aberdare Ranges between longitudes 36°30´E and 37°45´E and latitudes 0°15´N and 1°00´N. The upper catchment area is highly utilized for agricultural production due to favorable weather conditions, fertile soils and irrigation water availability through river abstractions. The main economic activity in Upper Ewaso Ng’iro North Catchment, is small-scale farming (rain-fed and irrigation), small-scale fishery and pastoralism. The area ranges from high potential high altitude to low potential arid and semi-arid zones. Due to the arid nature of most parts of the basin, the atmospheric demand for water is very high (Ericksen et al., Citation2012; Mutiga et al., Citation2010).
Data were collected in the period between September 2019 and December 2019 from a sample of 652 households. Multistage sampling technique was employed in the study. In the first stage, eight sub-catchments were sampled randomly out of the twenty-one sub-catchments of the Upper ENNCA; as a result the following sub-catchments were sampled; Ewaso Narok, Pesi, Rongai, Naromoru, Likii, Timau, Sirimon and Ngare Ndare. It is important to note that the eight sampled sub-catchments are also the WRUAs, since WRUAs are named as per sub-catchment. In the second stage, stratified sampling was done disproportionately to population size of these eight sub-catchments, since the number of households in each sub-catchment was unknown. Finally, simple random sampling was undertaken using a list from the WRUAs.
We utilized both primary and secondary data sources. Primary data were collected from households, WRUAs and key informants. Secondary data were collected from sources such as books, journals and reports. Data collected for the study included household data, group data, farm produce data and income data. A semi-structured questionnaire was administered to the small-scale farmers by trained enumerators, using the World Bank’s Computer Aided Personal Interview (CAPI) Program, through face-to-face interviews.
2.2 The methods used to obtain multi-dimensional poverty status; application of multi-criteria poverty cut-off lines
In order to categorize households as poor or non-poor, the cut-off poverty line is what matters. For objective poverty measures, i.e. consumption and income poverty, the study used the national rural poverty line of KES. 3,252 per adult equivalent per month (translating to KES 39,024 per adult equivalent annually) (KNBS, Citation2020; World Bank, Citation2020), to generate the income poverty dummy and consumption poverty dummy variables. If a household consumption per adult equivalent or income per adult equivalent, fell below KES 39,024, the household was categorized as poor, while households with consumption per adult equivalent or income per adult equivalent above KES 39,024 were categorized as non-poor.
On the other hand, to allocate subjective poverty status, we used the Economic Ladder Question with six steps. While using the ELQ self-assessment method, one can model subjective poverty using an ordered probit model (see, e.g., Ravallion & Lokshin, Citation2002), where the rungs of the ladder represent ordered outcomes. However, this study followed the approach by Mussa (Citation2014), Shams (Citation2014), Benfield (Citation2008), Devereux et al. (Citation2006)), and Kalugina and Najman (Citation2003), in defining a subjective poverty dummy as follows: households are subjectively poor if they fall on the bottom two rungs of the ladder and non-poor if they fall on rungs three to six.
Finally, to determine water poverty, we generated the household Water Poverty Index, using the water poverty index (WPI) methodology put forth by Sullivan et al. (Citation2003). Sullivan et al. (Citation2003) identify, via a community participatory approach, the following five components as key to a holistic WPI ( in the Appendix shows the specific variables used to construct each component of the WPI):
Resources: this captures physical availability of both surface and groundwater,
Access: this considers access to water for human use (drinking and nondrinking),
Capacity: this relates to the ability of people to manage water,
Use: this considers the multiple uses of water, and
Environment: this seeks to factor in environmental integrity related to water resources.
These five components are used to construct a WPI. Sullivan et al. (Citation2003) argue that the construction of the WPI should follow a structure similar to that of the Human Development Index (HDI). Specifically, each component is constructed via the following general formula:
where for each household, WPI and ɷI refers to the Water Poverty index; Ci refers to component I of the household’s WPI, with I = Access, Capacity, Use, and Environment, while wi is the weight applied to that particular component.
Principal component analysis (PCA) was then applied on the different components to generate the household water security index. According to Achia et al. (Citation2010) PCA is a multivariate statistical technique used to reduce the number of variables without losing too much information in the process. However, use of the conventional PCA would be erroneous since our data wewre not continuous but dummy. Therefore, the PCA results would not be reliable, to remedy this problem we used the polychoric PCA (Sinyolo et al., Citation2014). PCA and Factor Analysis have been used to create multi-criteria water security index for households (Matshe et al., Citation2013; Nadeem et al., Citation2018; Senna et al., Citation2019; Sinyolo et al., Citation2014). The first principal component with the largest variation was then used as the water security index as shown in .
Table 1. Water security principle components
Using this water security index, we categorized households into the water insecure (poor) and the water secure (non-poor). According to Sinyolo et al. (Citation2014), the challenge in categorizing households into groups of the water insecure (water poor) and water secure (water non-poor) is determining the cut-off line. Many studies assessing the socioeconomic status of households through categorization have used the 40th percentile as the poverty line for different aspects including poverty, food security and water poverty (Achia et al., Citation2010; Kabubo-Mariara & Kabara, Citation2015; Sinyolo et al., Citation2014). We therefore used the 40th percentile of the household water poverty index as the water poverty cut-off line to generate a water poverty dummy where Water poor = 1, and Water non-poor = 0.
2.3. The Empirical model estimating the impacts of WRUA membership on household poverty
We are interested in estimating the impact of WRUA membership on various binary poverty outcomes. According to Tesfaye and Tirivayi (Citation2018), unlike for continuous variables, accounting for sample selection and endogenous switching for binary outcomes where the data is fit using nonlinear models is challenging (Heckman, Citation1978). Estimations using a two-stage procedure would also lead to wrong conclusions and produce inconsistent results. We therefore utilized the endogenous switching probit (ESP) which is similar to the endogenous switching regression used for continuous outcomes (Lokshin & Glinskaya, Citation2009; Lokshin & Sajaia, Citation2011). The ESP model has been used in previous studies to measure impacts of different interventions on household welfare (Tesfaye & Tirivayi, Citation2018; Ayuya et al., Citation2015). The ESP model simultaneously models the membership to WRUA and the outcome equations in two stages. The first stage estimates the households’ decision to join WRUA membership using a probit model. In the second stage, the relationship between the binary outcomes and WRUA membership along with a set of explanatory variables is determined using a probit model with selectivity correction (Tesfaye & Tirivayi, Citation2018). We used the full information maximum likelihood (FIML) endogenous switching probit model to estimate parameters of interest as specified by Aakvik et al. (Citation2000), Lokshin and Glinskaya (Citation2009), and Lokshin and Sajaia (Citation2011).
Following Lokshin and Sajaia (Citation2011), we consider a household with two binary outcome equations (poor or non-poor) and the criterion function Wi (binary variable of household membership in WRUA) that determines the regime faced by the household. The potential values are presented as;
Where, ,
are latent variables (household poverty status) that define the observed poverty status p1 and p0 (whether household is poor or non-poor, respectively). Z is a vector of exogenous variables determining WRUA membership. Xi is a vector of exogenous variables determining poverty status, α and β are the vectors of parameters to be estimated while
,
and
are the disturbance terms.
The observed poverty status pi is defined as if
and
if
. With the assumption of joint normal distribution of
,
and
with a mean of zero, the correlation matrix is written as;
where is the correlation between
and µ and
is the correlation between
and µ, while
is the correlation between
and
. Consequently, the log-likelihood function for the model is given by;
where, is an optional weight for the ith household and
is the cumulative function of bivariate normal distribution (Lokshin & Sajaia, Citation2011). The advantage of the ESP model defined in Equationequation 6
(6)
(6) , is the possibility of deriving probabilities in counterfactual cases for the household’s poverty status on WRUA membership. Following Aakvik et al. (Citation2000) the effect of the treatment on the treated (TT) and the effect of treatment on the untreated (ATU) can be defined as shown by Equationequation 7
(7)
(7) and Equation8
(8)
(8) respectively;
where F is the cumulative function of the univariate normal distribution. Computing the average TT(x) on households participating in WRUA, results in the average treatment effect on the treated (ATT). Finally, computing the average TU(x) on households not participating in WRUA, results in the average treatment effect on the untreated (ATU).
2.4. Exclusion restrictions
For the model to be identified it is important to use exclusion restrictions as selection instruments, not only those automatically generated by the nonlinearity of the selection model of WRUA membership but also other variables that directly affect the selection variable but not the outcome variable (Di Falco et al., Citation2011; Mwaura et al., Citation2020; Tesfaye & Tirivayi, Citation2018). In our case, we used instrumental variables related to the household main water sources (piped water-tap in the compound, borehole water and river water) and the occurrence of water-related conflicts (water conflicts), following Mwaura et al. (Citation2020). We established the admissibility of these instruments by performing a simple falsification test: if a variable is a valid selection instrument, it will affect the membership decision but it will not affect the household consumption poverty, income poverty, subjective poverty and water among farm households that did not participate. of the appendix shows that the main water sources (MWS) and water conflicts can be considered as valid selection instruments. However, MWS-Piped water was dropped since it was not admissible since it had a significant effect on all household poverty measures except subjective poverty. In order to test for robustness of the ESP model, we also assessed the impact of WRUA membership on household poverty using the propensity score matching (PSM) using the nearest neighbor matching and the inverse probability weighting treatment effect.
3. Results and discussions
3.1. Descriptive statistics
The descriptive statistics are shown in . The results show that members and non-members are different with respect to the number of children, gender of the head, formal education, land size, the floor of main dwelling being predominantly sand and soil, the type of sanitation facility, the main source of lighting fuel, walls of the main dwelling, tropical livestock units, exposure to waterborne diseases, ratio of food expenditure to total expenditure, and adoption of improved crops. With regards to water, members have better access to both domestic and irrigation water, whereby, more members have piped water supply in their compound. On the contrary, non-members use more time and cover more distance to access domestic water, are more exposed to waterborne diseases. In order to cope with water shortage, non-members rely on rain fed cropping and rainwater harvesting and storage. Finally, despite members having significantly more consumption per adult equivalent and income per adult equivalent, they are more prone to being affected by water-related resource conflicts.
Table 2. Household and farm characteristics
3.1.1. Results of the Economic ladder question (ELQ)
In order to assess subjective welfare, the household head was given a hypothetical scenario with the following question; imagine six steps, where on the bottom, the first step, stand the poorest people, and on the highest step, the sixth, stand the rich. On which step are you today? The results show that cumulatively more than 80% of members and non-members subjectively place themselves on the second, third and fourth rungs of the ladder as shown in .
3.1.2. Objective, subjective and water poverty by WRUA membership
The results of the different poverty categories are illustrated in . The results show that more non-members are poorer across all the poverty categories as compared to members as shown by the highly significant t-test comparison of means. The results also reveal that rural income poverty, for the pooled sample stands at about 61% in the study area way above the national rural poverty rate of 40% (KNBS, Citation2020). On the other hand, the pooled rural consumption, subjective and water poverty in the study area stood at about 29%, 36% and 38%, respectively.
Table 3. Objective and subjective poverty by WRUA membership
To further assess if there is any correlation between the three types of poverty, the Spearman’s rank correlation was carried out between consumption poverty, income poverty, subjective poverty and water poverty, the results are quite interesting since, there is statistically no correlation between the four types of poverty as shown in . This is evidence to show that poverty in the Upper ENNCA is multifaceted and the determinants need to be explored.
Table 4. Correlation matrix of income poor, consumption poor and subjective poor
3.2. Determinants of WRUA membership
The factors that influence WRUA membership are presented in , i.e. the first-stage equation of the endogenous switching probit model. Based on the results, the significant determinants of WRUA membership included age, age squared, experience farming, gender, land size, title deed, growing improved crops, electricity, and the instrumental variables water conflict, MWS—river and the MWS—borehole.
Table 5. Determinants of WRUA membership (participation equation)
Age has a positive influence on WRUA membership, while age squared has a negative and significant influence, this suggests that the age of the household head has a diminishing effect on WRUA membership. Experience farming was found to influence WRUA membership positively. Previous studies have demonstrated a positive relationship between age, experience farming and collective action (Gyau et al., Citation2016; Mwaura et al., Citation2020). Male headed households had a higher probability of engaging in water-related collective action as compared to female-headed households, this could be due to community power relations where women are more marginalized or due to their low resource endowments. Land size had a positive influence on WRUA membership, implying that households with more land had a higher probability of joining WRUA membership, this was expected since an increase in land size increases per-acre water demand especially in irrigated production systems like in the study area; therefore, co-operation over water resource allocation increases utility while reducing conflicts. Holding a title deed had a positive influence on WRUA membership. This finding shows that WRUA membership is an important long-term investment pegged on tenure security and holding a title secures the property rights of land holders. Previous studies have shown the importance of property rights as a driver to collective action especially in natural resource management and long-term farm investments like water (Meinzen-Dick et al., Citation2002; Mwaura et al., Citation2020). Finally, previous studies have demonstrated the positive relationship between WRUA membership and water conflicts (Baldwin et al., Citation2018; Mwaura et al., Citation2020).
3.3 Determinants of WRUA members and non-members poverty status in terms of consumption, income, subjective and water poverty.
presents the estimates of the endogenous switching probit, i.e. the second-stage equation for consumption, income, subjective and water poverty, respectively. The likelihood-ratio tests for the joint independence of the selection and outcome equations were significant for consumption poverty (and water poverty (
, implying that the null hypothesis
can be rejected, suggesting that the error term in the selection equation is correlated with the error term in the outcome equation. However, likelihood-ratio test for the joint independence of the selection and outcome equations, was not significant for income poverty (
and subjective poverty (
, implying that the null hypothesis
cannot be rejected suggesting that the error terms in the selection and outcome equations are not correlated following Lokshin and Sajaia (Citation2011).
Table 6. Endogenous switching probit estimates of consumption, income, subjective and water poverty (outcome equation)
Further, are positive and significant for consumption and water poverty. This implies that there is a positive correlation between the unobserved characteristics which predict selection into WRUA membership and the poverty outcome of households justifying the use of the endogenous switching probit model. In other words, non-poor households in terms of consumption and water were more likely to self-select into WRUA membership. Further, for income poverty,
and
are not significant, however,
has a positive sign while
has a negative sign implying that non-poor household with respect to income were most likely to self-select into WRUA membership, while poor households were most likely to self-select into non-membership. Finally, for subjective poverty,
and
are not significant, however, both
and
have a positive sign implying that non-poor household with respect to subjective poverty were most likely to self-select into WRUA membership.
The findings show that years of experience farming had a positive influence on the probability of being income and water poor for members. This implies that members with more experience were, more likely to be income and water poor. This finding was unexpected, however, it could be explained since experience comes with age, and loss of physical strength compounded with health complications attributable to ageing. On the contrary, subjectively, household heads with more years of experience were less likely to feel poor at a subjective level for both members and non-members. These findings are consistent with the findings by Benfield (Citation2008) that though the decision of households to subjectively classify themselves as poor is heterogeneous, this decision is mostly based on the households’ perceptions of their vulnerability and adaptive expectations.
Household composition has an influence on household poverty. The findings show that non-member households with more male adults had a higher probability of being income poor. This implies that despite having more male adults who could work to earn a living, non-member households with more male adults were likely to be income poor, implying that the male adults are either unemployed or spend more time in non-income earning activities like idling or consuming alcohol. Further, member and non-member households with more female adults were less likely to be consumption poor, this finding reinforces the role of female adults on household food production, preparation and consumption. Previous studies have reported on the significant role played by female adults on household food security and nutrition (Amugsi et al., Citation2016; Lutomia et al., Citation2019). On the contrary, households with more female adults were more likely to be income poor for both member and non-members. Non-member households with more children were less likely to be consumption poor, this shows the role played by children in household chores like fetching water, firewood and farming activities, which though undocumented, have significant implications for the household poverty situation. On the contrary, member households with more children were more likely to be income poor and water poor. While there might not be a plausible explanation for this situation, previous studies have shown a negative relationship between household dependency burden, household size and welfare (Majeed & Malik, Citation2015). These findings reveal that breaking down the household composition rather than taking the household size could offer better policy insights for better approaches to rural poverty alleviation.
While gender of the household head has a negative influence across poverty types, it is a significant determinant of consumption poverty for non-members and income poverty for members. These findings imply that male-headed-households (MMHs) have a less probability of being poor across all categories except for water poverty. Previous studies have shown that FHHs are likely to be poor as compared to MHHs. Further, while land size too has a negative influence on the probability of being poor across the board, it is only significant for the probability of being subjectively poor for members. Further, non-member households holding a title for their land were also likely to be subjectively non-poor. These findings imply that households perceive land as an asset and a measure of wealth and are therefore, households holding more land with a title, were less likely to feel poor, these findings are similar to previous studies (Benfield, Citation2008). Similarly, households holding a title were less likely to be income poor, buttressing the importance of land property rights to household farm short-term and long-term investments as escape routes out of poverty. Previous studies have documented the impacts of land titling programs on household welfare (Deininger et al., Citation2011; Holden & Ghebru, Citation2013; Payne et al., Citation2009).
Household livestock ownership and the number of tropical livestock units (TLUs) show interesting results. Livestock ownership for members reduces the probability of being consumption poor for members and the probability of being subjective poor for non-members. On the contrary, livestock ownership has a positive influence on the probability of being consumption poor for non-members. Further, the number of TLUs had a positive influence on the probability of being consumption poor for members, while it has a negative influence on the probability of being consumption and income poor for non-members. This implies that member households are better off with few productive livestock, since they have other welfare improving activities like irrigation farming, while non-member households off-set the negative welfare from livestock ownership, by increasing the TLUs. Households whose primary occupation was farming had a higher probability of being income poor compared to households dependent on off-farm income, this finding is consistent with economic theory and previous studies.
Access to credit can offer an effective pathway out of poverty, and the results show that access to credit has a negative influence on the probability of being poor across the board. However, it is significant for consumption poverty status of members and income poverty status of non-members. Previous studies have shown the impacts of credit access on household welfare (Mwaura et al., Citation2020; Teka & Lee, Citation2020). Access to extension services has a negative influence on the probability of being income poor for members. Previous studies have reported on the impacts of access to extension on household welfare (Mwaura et al., Citation2020; Teka & Lee, Citation2020). Distance to the nearest tarmac road in kilometers influenced the probability of being consumption poor for members and non-members and income poor for non-members positively. This implies that households without access to improved access to improved transport infrastructure were likely to be poor. Previous studies have demonstrated the link between infrastructure and rural welfare (Bertolini, Citation2019; Songco, Citation2002). One interesting finding is that of improved crops whereby, it has a positive and highly significant influence on the probability of being subjective poor. This finding implies that despite households growing improved crops, they feel poor, while we did not investigate, the reasons as to this situation, this could be due to many factors including situations where farmers get low prices for their produce at the farm gate. Household whose main source of cooking fuel is firewood have a higher possibility of being poor across all categories. Previous studies have demonstrated that it is mostly the poor who are dependent on un-improved energy sources for their energy needs (Morrissey, Citation2017; Mwaura et al., Citation2020). Finally, access to electricity has a negative influence on the probability of households being subjectively poor. This intuitively implies that households connected to electricity feel less poor than those not connected to electricity.
3.3. Mean treatment effects of WRUA membership on household poverty
The results of the mean treatments effects of WRUA membership on different poverty categories are shown in . The results show that WRUA membership has a negative and significant influence on the probability of being poor across all poverty categories. The results from the different models are quite distinct. The findings from the endogenous switching probit model show that membership in WRUAs reduced the probability of being consumption poor by about 8%, income poor by about 47%, subjective poor by about 20% and water poor by about 14%. The IPW results show that membership in WRUAs reduced the probability of being consumption poor by about 5%, income poor by about 10%, subjective poor by about 9% and water poor by about 30%. Finally, the PSM results using the nearest neighbor matching of four neighbors show that membership in WRUAs reduced the probability of being consumption poor by about 7%, income poor by about 8%, subjective poor by about 10% and water poor by about 32%. We therefore reject all four hypotheses that there is no relationship between household consumption poverty, income poverty, subjective poverty, water poverty and WRUA membership.
Table 7. Mean treatment effects of WRUA membership on household poverty
The ATU results imply that non-members would have significant reductions in household poverty if they considered membership into Community-Based Water Development Projects in this case WRUA, whereby, they would have reduced consumption poverty by about 6%, reduced income poverty by about 25%, reduced subjective poverty by about 12% and reduced water poverty by about 11%. These findings are similar to the findings of Speranza et al. (Citation2016) who found that community water projects in the Ewaso Ng’iro North Catchment area, largely met the goals of water provision, in addition to improving local livelihoods through improved crop and livestock production not to mention improved health and sanitation. Further, Mwaura et al. (Citation2020) found that WRUA membership had a positive impact on household welfare in the study area and that non-members would have improved welfare of they considered becoming members. Finally, the findings are similar to the findings by McCarthy and Essam (Citation2009) who found that WRUA membership has a significant influence on agricultural productivity and incomes.
4. Conclusions and recommendations
We have demonstrated that rural poverty is complex and takes many dimensions including objective, subjective and water poverty. Further, the results have illustrated that households are heterogeneous and so is rural poverty. While evidence has shown that it is the poor who are largely dependent on natural resource for their livelihoods, collective water management can have welfare improving impacts for rural households, especially where there low public investments in water provision, management and access. Household characteristics, farm characteristics, dwelling characteristics, institutional and infrastructural factors all have an influence on rural poverty. These results imply two things, first is that non-members would be able to escape poverty if they considered to be members and secondly, there is need for a multipronged approach to tackle rural poverty. We therefore recommend that WRUAs and CWDPs be further empowered through financial, legal and capacity building interventions to enhance their community impacts, while minimizing conflicts.
The findings of the study reveal that breaking down the household composition rather than taking the household size could offer better policy insights for better approaches to rural poverty alleviation. Rural poverty is likely to affect the old significantly and is highly gendered; therefore, pro-poor policies and social-safety net programs targeting the old and FHH need to be up-scaled, since these groups are likely to be trapped into poverty. Improved access to improved energy sources for lighting and cooking such as electricity, solar and liquefied petroleum gas (LPG) should be prioritized through tax waivers or scrapping off of electricity connection fees, since the ripple effects in the long-run outweigh the immediate costs. We recommend the spurring of the rural economy through the creation of alternative off-farm sources of employment to avoid over-reliance on farming and also increase the number of employment opportunities. To achieve this goal, access to credit is key as demonstrated by the results. The National Treasury and the Central Bank of Kenya should consider amending the interest capping law to enable financial institutions off-load the much-needed credit to the market, with less hindrances citing credit risk, since rural farming households are considered high risk by formal financial institutions. Another way to spruce the rural economy is providing constant and timely extension services so as to improve and transform the rural cropping and livestock systems into sustainable agribusiness enterprises using a holistic value-chain approach. Infrastructure too is critical so as to achieve the necessary rural transformation, improved transport and communication network should be prioritized through the necessary national government and county government development funds. The results have also demonstrated the strong link between land property rights, collective action and rural poverty, the government should fast-track existing, and roll-out new titling programs so as to hand property rights to owners and secure their land tenure rights, so as to give them incentives to make long-term land-based investments, the titles could also be used as collateral to secure credit. Finally, it is important to note that, while this study was carried before the COVID-19 pandemic struck, the situation might be worse, since several development organizations have projected the expected impacts of the COVID-19 pandemic in the deepening of rural poverty in the short and medium term (see, FAO, Citation2020). Future studies should empirically determine the impacts of the COVID-19 pandemic on rural poverty at a wider scale. Finally, further studies are also needed to quantify and show the relationship between water quality and rural poverty in Kenya.
Conflicts of Interests
The authors declare no conflict of interest
Additional information
Funding
Notes on contributors

Simon Ng’ang’a Mwaura
Simon Mwaura is a lecturer in the Department of Economics in the School of Business and Economics, Maasai Mara University, Kenya. He is a PhD candidate in Agricultural Economics at Egerton University, Kenya, specializing in natural resource economics. He graduated with M.Sc. Agricultural and Applied Economics from Egerton University in 2012. He is an innovator and founder of Trendy Animal Feeds a social enterprise aimed at exploring the commercial viability of alternative animal feed protein sources, using the insects-for-feed approach. His research areas encompass, collective action, gender, natural resource management, impact evaluation, water governance, land governance, welfare, smallholder irrigation, and agricultural marketing.
References
- Aakvik, A., Heckman, J., & Vytlacil, E., 2000. Treatment effect for discrete outcomes when responses to treatment vary among observationally identical persons: An application to Norwegian vocational rehabilitation programs. Technical Paper 262. Cambridge, Mass: National Bureau of Economic Research.
- Achia, T. N. O., Wangombe, A., & Khadioli, N. (2010). A logistic regression model to identify key determinants of poverty using demographic and health survey data. European Journal of Social Sciences, 13(1), 38–22.
- Adhikari, B. (2005). Poverty, property rights and collective action: Understanding the distributive aspects of common property resource management. Environment and Development Economics, 10(1), 7–31. https://doi.org/10.1017/S1355770X04001755
- Agrawal, A. (2002). Common resources and institutional sustainability. In E. Ostrom, T. Dietz, N. Dolsak, P. C. Stern, S. Stonich & E. U. Weber (Eds.), The Drama of the Commons (pp. 41–85). National Academy Press.
- Amugsi, D. A., Lartey, A., Kimani-Murage, E., & Mberu, B. U. Women’s participation in household decision-making and higher dietary diversity: Findings from nationally representative data from Ghana. (2016). Journal of Health, Population and Nutrition, 35(1), 16. 2016. https://doi.org/10.1186/s41043-016-0053-1
- Angelsen, A., Jagger, P., Babigumira, R., Belcher, B., Hogarth, N. J., Bauch, S., Börner, J., Smith-Hall, C., & Wunder, S. (2014). Environmental income and rural livelihoods: A global-comparative analysis. World Development, 64(Suppl 1), S12–S28. https://doi.org/10.1016/j.worlddev.2014.03.006
- Ayuya, O. I., Gido, E. O., Bett, H. K., Lagat, J. K., Kahi, A. K., & Bauer, S. (2015). Effect of certified organic production systems on poverty among smallholder farmers: Empirical evidence from Kenya. World Development, 67, 27–37. http://dx.doi.org/10.1016/j.worlddev.2014.10.005
- Baldwin, E., McCord, P., Dell’Angelo, J., & Evans, T. (2018). Collective action in a polycentric water governance system. Environmental Policy and Governance, 28(4), 212–222. https://doi.org/10.1002/eet.1810
- Barbier, E. B. (2010). Poverty, development, and environment. Environment and Development Economics, 15(6), 635–660. https://doi.org/10.1017/S1355770X1000032X
- Benfield, W. (2008). Determinants of poverty and subjective well-being. Social and Economic Studies, 57(3/4), 1–51.
- Bertolini, P., 2019. Overview of income and non-income rural poverty in developed countries.In Proceedings of the Expert Group Meeting on Eradicating Rural Poverty to Implement the 2030 Agenda for Sustainable Development, United Nations Economic Commission for Africa, Addis Ababa, Ethiopia, 27 February–1, March
- Börner, J., Shively, G., Wunder, S., & Wyman, M. (2015). How do rural households cope with economic shocks? Insights from global data using hierarchical analysis. Journal of Agricultural Economics, 66(2), 392–414. https://doi.org/10.1111/1477-9552.12097
- Burney, J. A., & Naylor, R. L. (2012). Smallholder irrigation as a poverty alleviation tool in sub-Saharan Africa. World Development, 40(1), 110–123. https://doi.org/10.1016/j.worlddev.2011.05.007
- Cali, M., & Menon, C. (2013). Does urbanization affect rural poverty? Evidence from Indian Districts. The World Bank Economic Review, 27(2), 171–201. https://doi.org/10.1093/wber/lhs019
- Cardoso, S. M., & Teixeira, A. A. C. (2020). The focus on poverty in the most influential journals in economics: A bibliometric analysis of the “Blue Ribbon” journals. A Bibliometric Analysis of the “Blue Ribbon” Journals. Poverty & Public Policy, 12(1), 10–42. https://doi.org/10.1002/pop4.269
- Carletto, G., & Zezza, A. (2006). Being poor. Feeling Poorer: Combining Objective and Subjective Measures of Welfare in Albania. The Journal of Development Studies, 42(5), 739–760.
- Castillo, G. E. (2007). Reversing the flow: Agricultural water management pathways for poverty reduction. In D. Molden, et al., (Ed.), Water for Food, Water for Life (pp. 149–191). IWMI and Earthscan.
- Chaudhry, I., Malik, S., & Ashraf, M. (2006). Rural poverty in Pakistan: Some related concepts, issues and empirical analysis. Pakistan Economic and Social Review, 44(2), 259–276.
- De Janvry, A., & Sadoulet, E. (2000). Rural poverty in Latin America: Determinants and exit paths. Food Policy, 25(4), 389–409. https://doi.org/10.1016/S0306-9192(00)00023-3
- Deininger, K., Ali, D., & Alemu, T. (2011). Impacts of land certification on tenure security, investment, and land market participation: Evidence from Ethiopia. Land Economics Retrieved. 87(2), 312–334. January 22, 2021 https://doi.org/10.3368/le.87.2.312
- Devereux, S., Baulch, B., MacAuslan, I., Phiri, A., & Sabates-Wheeler, R. (2006).Vulnerability and social protection in Malawi, DFID Malawi Report. DFID https://www.ids.ac.uk/download.php?file=files/MalawiVulnerabilityReport_Final.pdf ( Accessed May 19, 2020).
- Di Falco, S., Veronesi, M., & Yesuf, M. (2011). Does adaptation to climate change provide food security? A Micro-Perspective from Ethiopia. American Journal of Agricultural Economics, 93(3), 829–846. https://doi.org/10.1093/ajae/aar006
- Di Gregorio, M., Hagedorn, K., Kirk, M., Korf, B., McCarthy, N., Meinzen-Dick, R., & Swallow, B., 2008. Property rights, collective action, and poverty: The role of institutions for poverty reduction. CAPRi working paper 81. Washington, DC: International Food Policy Research Institute
- Dinda, S. (2004). Environmental Kuznets curve hypothesis: A survey. Ecological Economics, 49(4), 431–455. https://doi.org/10.1016/j.ecolecon.2004.02.011
- Ericksen, P., Leeuw, J., Said, M., Silvestri, S., & Zaibet, L. (2012). Mapping ecosystem services in the Ewaso Ng’iro catchment. International Journal of Biodiversity Science Ecosystem Services & Management, 8(1–2), 122–134.
- Ewaso Ng’iro North River Basin Development Authority (ENNDA), 2019. Areas of coverage. http://www.ennda.go.ke/area-of-coverage/( Accessed March 17 2020).
- FAO, 2008. Water and the rural poor: Interventions for improving livelihoods in Sub-Saharan Africa. Food and Agriculture Organization. http://www.fao.org/3/i0132e/i0132e00.htm ( accessed 3 August 2020)
- FAO, 2020. COVID-19 and rural poverty: Supporting and protecting the rural poor in times of pandemic. https://digitallibrary.un.org/record/3859437 (accessed 4 August 2020).
- Garriga, R. G., & Foguet, A. P. (2013). Water, sanitation, hygiene and rural poverty: Issues of sector monitoring and the role of aggregated indicators. Water Policy, 15(6), 1018–1045. https://doi.org/10.2166/wp.2013.037
- Gyau, A., Mbugua, M., & Oduol, J. (2016). Determinants of participation and intensity of participation in collective action: Evidence from smallholder avocado farmers in Kenya. Journal on Chain and Network Science, 16(2), 147–156. https://doi.org/10.3920/JCNS2015.0011
- Hassan, R. M., & Babu, S. C. (1991). Measurement and determinants of rural poverty: Household consumption patterns and food poverty in rural Sudan. Food Policy, 16(6), 451–460. https://doi.org/10.1016/0306-9192(91)90045-L
- Heckman, J. J. (1978). Dummy endogenous variables in a simultaneous equation system. Econometrica, 46(4), 931–959. https://doi.org/10.2307/1909757
- Holden, S. T., & Ghebru, H. (2013). Welfare impacts of land certification in Tigray, Ethiopia. In S. T. Holden, K. Otsuka, & K. Deininger (Eds.), Land Tenure Reform in Asia and Africa. Palgrave Macmillan. https://doi.org/10.1057/9781137343819_6
- Hope, R. A. (2006). Water, workfare and poverty: The impact of the working for water programme on rural poverty reduction. Environment, Development and Sustainability, 8(1), 139–156. https://doi.org/10.1007/s10668-005-1780-4
- Horii, R., & Ikefuji, M. (2014). Environment and growth. In S. Managi (Ed.), Handbook of Environmental Economics in Asia. Routledge.
- Huang, Q., Rozelle, S., Lohmar, B., Huang, J., & Wang, J. (2006). Irrigation, agricultural performance and poverty reduction in China. Food Policy, 31(1), 30–52. https://doi.org/10.1016/j.foodpol.2005.06.004
- Hussain, I., & Hanjra, M. A. (2004). Irrigation and poverty alleviation: Review of the empirical evidence. Irrigation and Drainage, 53(1), 1–15. https://doi.org/10.1002/ird.114
- John, L., & Firth, D. (2005). Water, watersheds, forests and poverty reduction: A Carribbean perspective. The International Forestry Review, 7(4), 311–319. https://doi.org/10.1505/ifor.2005.7.4.311
- Johnson, N., Garcia, J., Rubiano, J. E., Quintero, M., Estrada, R. D., Mwangi, E., Morena, A., Peralta, A., & Granados, S. (2009). Water and poverty in two Colombian watersheds. Water Alternatives, 2(1), 34–52.
- Kabubo-Mariara, J., & Kabara, M., 2015. Climate change and food security in Kenya: Environment for development. www.efdinitiative.org/sites/default/files/publications/efd-dp-15-05.pdf (Accessed 4 August 2020)
- Kadirbeyoğlu, Z., & Özertan, G., 2011. Users’ perceptions of water user associations: Evidence from three cases in Turkey. Working Paper 2011–1. Istanbul: Boğaziçi University Department of Economics.
- Kalugina, E., & Najman, B. (2003). Labour and poverty in Russia self-rated perceptions and monetary evaluations. Экономический журнал Высшей школы экономики (Translation. Economic Journal of the Higher School of Economics, 7(1), 20–49.
- Kijima, M., Nishide, K., & Ohyama, A. (2010). Economic models for the environmental Kuznets curve: A survey. Journal of Economic Dynamics & Control, 34(7), 1187–1201. https://doi.org/10.1016/j.jedc.2010.03.010
- KNBS, 2020. The comprehensive poverty report: Children, youth, women, men and the elderly, from national to county level. Kenya National Bureau of Statistics.
- Kullenberg, C., & Nelhans, G. (2020). Measuring welfare beyond GDP- ‘objective’ and ‘subjective’ indicators in Sweden, 1968–2015. Valuation Studies, 5(1), 7–38. https://doi.org/10.3384/VS.2001-5992.17517
- Lekobane, K. R., & Seleka, T. B. (2017). Determinants of household welfare and poverty in Botswana, 2002/2003 and 2009/2010. Journal of Poverty, 21(1), 42–60. https://doi.org/10.1080/10875549.2016.1141381
- Lokshin, M., & Glinskaya, E. (2009). The effect of male migration on employment patterns of women in Nepal. The World Bank Economic Review, 23(3), 481–507. https://doi.org/10.1093/wber/lhp011
- Lokshin, M., & Sajaia, Z. (2011). Impact of interventions on discrete outcomes: Maximum likelihood estimation of the binary choice models with binary endogenous regressors. The Stata Journal: Promoting Communications on Statistics and Stata, 11(3), 368–385. https://doi.org/10.1177/1536867X1101100303
- López, R., & Valdés, A. (2000). Determinants of rural poverty in Colombia. In R. López & A. Valdés (Eds.), Rural Poverty in Latin America. Palgrave Macmillan. https://doi.org/10.1057/9780333977798_10
- Lutomia, C. K., Obare, G. A., Kariuki, I. M., Muricho, G. S., & Yildiz, F. (2019). Determinants of gender differences in household food security perceptions in the western and eastern regions of Kenya. Cogent Food and Agriculture, 5(1), 1694755. https://doi.org/10.1080/23311932.2019.1694755
- Madulu, N. F. (2003). Linking poverty levels to water resource use and conflicts in rural Tanzania. Physics and Chemistry of the Earth, 28(20–27), 911–917. https://doi.org/10.1016/j.pce.2003.08.024
- Majeed, M., & Malik, M. (2015). Determinants of household poverty: Empirical evidence from Pakistan. The Pakistan Development Review, 54(4I–II), 701–717. https://doi.org/10.30541/v54i4I-IIpp.701-718
- Malik, S. (1996). Determinants of rural poverty in Pakistan: A micro study. The Pakistan Development Review, 35(2), 171–187. https://doi.org/10.30541/v35i2pp.171-187
- Matshe, I., Moya-Maposa, S., & Zikhali, P. (2013). Water poverty and rural development: Evidence from South Africa. African Journal of Agricultural and Resource Economics, 8(2), 136–156.
- McCarthy, N., & Essam, T., 2009. Impact of water user associations on agricultural productivity in Chile (Discussion Paper No. 892). IFPRI.
- Meinzen-Dick, R., Knox, A., Place, F., & Swallow, B. (2002). Innovation in natural resource management: The role of property rights and collective action in developing countries. International Food Policy Research Institute. The Johns Hopkins University Press.
- Morrissey, J., 2017. The energy challenge in sub-Saharan Africa: A guide for advocates and policy makers: Part 2: Addressing energy poverty. Oxfam Research Backgrounder Series.
- Mungai, N., Ong, C. K., Kiteme, B., Elkaduwa, W., & Sakthivadivel, R. (2004). Lessons from two long-term hydrological studies in Kenya and Sri Lanka. Agriculture, Ecosystems & Environment, 104(1), 135–143. https://doi.org/10.1016/j.agee.2004.01.011
- Mussa, R. (2014). Impact of fertility on objective and subjective poverty in Malawi. Development Studies Research., 1(1), 202–222. https://doi.org/10.1080/21665095.2014.948898
- Mutiga, J. K., Su, Z., & Woldai, T. (2010). Using satellite remote sensing to assess evapotranspiration: Case study of the Upper Ewaso Ng’iro North Basin, Kenya. International Journal of Applied Earth Observation and Geoinformation, 12(1), 100–S108. https://doi.org/10.1016/j.jag.2009.09.012
- Mwangi, J. K., & Crewett, W. (2019). The impact of irrigation on small-scale African indigenous vegetable growers’ market access in peri-urban Kenya. Agricultural Water Management, 212(212), 295–305. https://doi.org/10.1016/j.agwat.2018.06.036
- Mwaura, S. N., Kariuki, I. M., Kiprop, S., Muluvi, A. S., Kiteme, B., Mshenga, P. M., & Tejada Moral, M. (2020). Impact of water-related collective action on rural household welfare in the Upper Ewaso Ng’iro North Catchment Area: The application of the endogenous switching regression. Cogent Food and Agriculture, 6(1), 1, 1834667. https://doi.org/10.1080/23311932.2020.1834667
- Nadeem, A. M., Cheo, R., & Shaoan, H. (2018). Multidimensional analysis of water poverty and subjective well-being: A case study on local household variation in Faisalabad, Pakistan. Social Indicators Research, 138(1), 207–224. https://doi.org/10.1007/s11205-017-1652-y
- Payne, G., Durand-Lasserve, A., & Rakodi, C. (2009). Social and economic impacts of land titling programs in urban and periurban areas: A short review of the literature. In S. V. Lall, M. Freire, B. Yuen, R. Rajack, & J. J. Helluin (Eds.), Urban Land Markets. Springer. https://doi.org/10.1007/978-1-4020-8862-9_6
- Quang Dao, M. (2004). Rural poverty in developing countries: An empirical analysis. Journal of Economic Studies, 31(6), 500–508. https://doi.org/10.1108/01443580410569244
- Ravallion, M. (2012). Poor or just feeling poor? On using subjective date in measuring poverty. Development Research Group, World Bank.
- Ravallion, M., & Bidani, B. (1994). How robust is a poverty profile? The World Bank Economic Review, 8(1), 75–102. https://doi.org/10.1093/wber/8.1.75
- Ravallion, M., Himelein, K., & Beegle, K., 2013. Can subjective questions on economic welfare be trusted? Development research group, World Bank: Poverty and inequality team. Policy Research Working paper 6726.
- Ravallion, M., & Lokshin, M. (2002). Self-rated economic welfare in Russia. European Economic Review, 46(8), 1453–1473. https://doi.org/10.1016/S0014-2921(01)00151-9
- Ravallion, M., & Sen, B. (1994). Impacts on rural poverty of land-based targeting: Further results for Bangladesh. World Development, 22(6), 823–838. https://doi.org/10.1016/0305-750X(94)90056-6
- Republic of Kenya, 2007. The National Water Services Strategy (NWSS) 2007–2015. Ministry of Water and Irrigation.
- Richards, N., & Syallow, D. (2018). Water resources users associations in the Mara Basin, Kenya: Pitfalls and opportunities for community based natural resources management. Frontiers in Environmental Science, 6, 138. https://doi.org/10.3389/fenvs.2018.00138
- Seckler, D., & Amarasinghe, U., 2000. Water supply and demand, 1995 to 2025. IWMI annual report 1999–2000. International Water Management Institute.
- Senna, L. D., Maia, A. G., & Medeiros, J. D. F. (2019). The use of principal component analysis for the construction of the Water Poverty Index. Rev. Bras. Recur. Hídr, 24, 1–14.
- Shams, K. (2014). Determinants of subjective well-being and poverty in rural Pakistan: A micro-level study. Social Indicators Research, 119(3), 1755–1773. https://doi.org/10.1007/s11205-013-0571-9
- Shiferaw, B., Kebede, T., & Reddy, V. R. 2008. Community watershed management in semi-arid India. The state of collective action and its effects on natural resources and livelihoods. CAPRi Working Paper No. 85, June, 2008.
- Sinyolo, S., & Mudhara, M. (2018). Collective action and rural poverty reduction: Empirical evidence from kwazulu-natal, South Africa. Agrekon, 57(1), 78–90. https://doi.org/10.1080/03031853.2018.1451349
- Sinyolo, S., Mudhara, M., & Wale, E. (2014). Water security and rural household food security: Empirical evidence from the Mzinyathi district in South Africa. Food Security, 6(4), 483–499. https://doi.org/10.1007/s12571-014-0358-0
- Songco, J. A., 2002. Do rural infrastructure investments benefit the poor? Evaluating linkages–A global view, A Focus on Vietnam. Policy Research Working Paper;No. 2796. World Bank. © World Bank. https://openknowledge.worldbank.org/handle/10986/15607
- Speranza, C. I., Kiteme, B., Wiesmann, U., & Jörin, J. (2016). Community-based water development projects, their effectiveness, and options for improvement: Lessons from Laikipia, Kenya. African Geographical Review. https://doi.org/10.1080/19376812.2016.1253485
- Stifel, D., & Minten, B. (2008). Isolation and agricultural productivity. Agricultural Economics, 39(1), 1–15. https://doi.org/10.1111/j.1574-0862.2008.00310.x
- Suhardiman, D., Nicol, A., & Mapedza, E. (2017). Water governance and collective action. Routledge. https://doi.org/10.4324/9781315174938
- Sullivan, C. A., Meigh, J. R., & Giacomello, A. M. (2003). The water poverty index: Development and application at the community scale. Natural Resources Forum, 27(3), 189–199. https://doi.org/10.1111/1477-8947.00054
- Swallow, B., Meinzen-Dick, R., & van Noordwijk, M., 2005. Localizing demand and supply of environmental services: Interactions with property rights, collective action and the welfare of the poor International Food Policy Research Institute CAPRI Working Paper 42
- Teka, A., & Lee, S. (2020). Do agricultural package programs improve the welfare of rural people? Evidence from smallholder farmers in Ethiopia. Agriculture, 10(5), 190. https://doi.org/10.3390/agriculture10050190
- Tesfaye, W., & Tirivayi, N. (2018). The impacts of postharvest storage innovations on food security and welfare in Ethiopia. Food Policy, 75(2018), 52–67. https://doi.org/10.1016/j.foodpol.2018.01.004
- Van Koppen, B. (1998). Water rights, gender, and poverty alleviation. Inclusion and exclusion of women and men smallholders in public irrigation infrastructure development. Agriculture and Human Values, 15(4), 361–374. https://doi.org/10.1023/A:1007537119163
- World Bank, 2020. Poverty and equity brief, Sub-Saharan Africa: Kenya. World Bank Group.
- WRMA., WSTF, 2014. Water Resource Users Association Development Cycle (WDC)
- Yang, L. (2018). Measuring well-being: A multidimensional index integrating subjective well-being and preferences. Journal of Human Development and Capabilities, 19(4), 456–476. https://doi.org/10.1080/19452829.2018.1474859
Appendix
Table A1. Falsification tests on the validity of the selection instruments
Table A2. Variables used for each component of the WPI