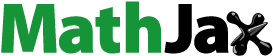
Abstract
The main objective of this research is to investigate the Predictability of Financial Statements Fraud-Risk Using Benford’s Law on the Tehran Stock Exchange. Therefore, based on financial fraud detection criteria, a sample of 50 companies was extracted that 25 companies had fraud-risk in financial statements (experimental group) and 25 did not have fraud-risk (control group). Next, the frequency distribution of the first left digit of the numbers in the financial statements as well as the financial ratios of both groups was extracted, and their conformity with Benford’s distribution was evaluated through the chi-square test to test the research hypotheses. The comparison between the mentioned frequency distribution and Benford’s distribution showed a significant difference. The result indicates that Benford’s law cannot predict the financial statements fraud-risk of companies, in other words, Benford’s law cannot separate companies with fraud-risk from those without fraud-risk in financial statements.
PUBLIC INTEREST STATEMENT
The study examines the effectiveness of applying Benford’s law in predicting fraud in the financial statements of companies listed on the Tehran Stock Exchange. According to the results of the investigation, Benford’s law cannot categorize fraudulent and non-fraudulent companies. Therefore, Benford’s law cannot predict financial statements fraud or, in other words, it has zero prediction accuracy. Auditors are advised not to rely too much on Benford’s law in their analysis of the possibility of fraud in financial statements. In addition to using this law, use other methods as well. It is recommended to investors, as previous studies in other countries have often shown that Benford’s law can predict financial statements fraud, possibly due to the special nature of corporate governance in Iran and the widespread presence of institutional and government owners in the capital market, companies are using more sophisticated methods of financial statement fraud that Benford’s law
1. Introduction
Capital markets are prone to fraud and make corruption due to the expansion of capital markets and the structured economic power beyond the geographical boundaries. Fraud means gaining something worthwhile or escaping from obligations that are done to deceive others, which harms the victims and brings benefits for those who commit it. Fraud is a big challenge that executives and auditors are facing, and today, it is a very significant issue and a global problem increasing day by day. Fraud in financial statements can have a detrimental impact on the market value of a business unit and its credibility and ability in achieving its strategic objectives to the extent that the nature of the company would be in jeopardy.
On the other hand, detection of fraud is complicated, and most of the fraud is not explicitly disclosed or, if disclosed, may not be reported. The increase in financial statements fraud has raised concerns about the quality of financial reporting. So, in recent years, the issue of fraud detection in financial statements has attracted special attention. Fraud detection has always been the center of attention of investors, legislators, managers, and auditors (Khani, Citation2007). Identifying the factors influencing this phenomenon as well as its proper prediction can be a great help to Decision-makers and investors (Farzad et al., Citation2018). Demand for greater transparency, procedural stability, and financial information have increased auditors’ responsibilities and insufficiency in capital markets has led to an increase in the cost of obtaining external resources on domestic resources and the creation of financial constraints for firms (Ahmadi and kordloie, Citation2018). Fraud is a major factor in recent financial crisis and the global economic downturn. Accordingly, a review of the previous studies on the performance of stock exchanges in Iran and the world shows that professional legislative assemblies of accounting and management pay particular attention to fraud and existing solutions to prevent fraudulent behavior in financial statements.
In Iran, like in other countries, there is a distortion of financial statements; however, less attention has been paid to this issue despite its economic consequences on companies, the economy of the country in the capital market, and the country’s research institutions and there is no direct institution for investigating and detecting possible cases of fraud. Also, institutions, such as the Tehran stock exchange (TSE), do not provide the public and analysts with possible information on fraudulent financial statements. Therefore, it is a fundamental challenge that TSE and no other institution in Iran investigate the possibility of fraud in the financial statements of companies listed on the Tehran Stock Exchange as an emerging economy. Accordingly, auditors, as an important part of the control and oversight systems in corporate governance in emerging economies, try to mitigate the effects of these problems. They in auditing financial statements try to use new analytical methods including Benford’s Law instead of using the traditional auditing and accounting process to investigate and detect fraud (Nia, Citation2015).
Benford’s law is regarded as one of the attractive rules of probability theory. In this regard, many studies have been conducted in various areas, including financial statements, using Benford’s law. This law is used for verifying information, detecting fraud, finding mistakes or violations in accounting data, and in various fields, such as optimizing computer applications. Also, auditors and tax authorities use this law to detect fraud as well as other manipulations in accounting and tax information (Durtschi et al., Citation2004). Therefore, the use of Benford’s law in auditors’ analytical reviews is of particular importance and is not given much attention in Iran. Due to the nature of Iran’s economy and its severe inflation and high tax evasion of companies, as well as the occurrence of many bankruptcies and manipulations of financial statements, auditors need to use this law. But studies in Iranian capital market research also show that researchers have not paid much attention to this issue and there is a deep research gap. There is also a need to examine the effectiveness of Benford’s law in emerging economies due to the specific nature of their capital markets. Because, this law may not be effective in the market of countries like Iran, which has not been studied yet. Therefore, due to the lack of studies on fraudulent financial statements and the importance of this issue, and the methods for preventing and detecting fraud, a study is needed to provide a proper tool for predicting financial statements fraud.
This study aimed to investigate the predictability of fraudulent financial statements in Iran using Benford’s Law as one of the most prominent tools for predicting financial statements fraud to fill this research gap. The main question of this research is whether Benford law can predict financial statements fraud or not. The contribution of this study is that first, it shows the effectiveness of applying Benford’s law in predicting fraud in the financial statements of companies listed on the Tehran Stock Exchange. Second, it provides auditors with suggestions for its use. Third, capital market researchers are drawn to the issue of financial statement fraud. The study is expected to help managers and auditors to detect fraud so that they can have a better understanding of the statistical methods for detecting financial statements fraud and find possible solutions to reduce fraudulent financial statements and provide regular financial reports to beneficiaries.
The continuation of this research consists of six sections. In the literature review section, Benford’s law and its application are discussed. The following is a background of theoretical foundations. Then, Hypotheses have been developed. In the methodology section, appropriate methods for testing hypotheses are provided. In the findings section, the research findings are analyzed. Finally, in the discussion and conclusion sections, the research findings are discussed and the general conclusions about the findings are discussed.
2. Literature
High-quality and reliable financial information is the lifeblood of the capital market (Davani and Rezaee & Davani, Citation2013); because error-free financial information, whether in the form of error or deliberate distortion and in general any misuse, has played a decisive role in the success of the capital market is performing the task of transmitting information (Pazarçeviren, Citation2005). According to Auditing Standard No. 24 of Iran, entitled “The Auditor’s Responsibility for Fraud and Mistake in the Audit of Financial Statements,” fraud can be defined as “any intentional or fraudulent act by one or more managers, employees or third parties to obtain an unlawful or unlawful advantage” (Auditing Standards Development Committee, 2012). According to the 2012 Association of Certified Fraud Investigators, the most common form of fraud in financial statements is in the form of deafening assets and revenues or reducing debts and expenses. All the above distortions indicate the manipulation of financial figures. Among these, the issue of fraud detection is of particular importance; due to the help of domestic researchers in this field to distinguish fraudulent companies from non-fraudulent companies, criteria such as adverse opinion or disclaimer of the opinion of the auditor, overstatement of assets, and material misstatement of generally accepted accounting principles are used. If the market is operating normally, the data in it have a logarithmic distribution called Benford’s law. This rule indicates the probability of the occurrence of different digits in a set of numbers. Relying on the specificity of the distribution of figures in a set called financial statements and using a theoretical distribution called Benford’s Law Distribution, a different method can be introduced as a tool to investigate and predict fraud (Amiram et al., Citation2015).
In 1881, Simon Newcomb, astronomer, and mathematician published the first paper on the use of more small figures, now known as Benford’s Law, in the American Mathematical Journal. By noticing a book, he concluded that the pages of books whose first pages had dealt with small digits were more worn than the pages that dealt with larger digits. He observed that his colleagues use the tables that begin with digit 1 more often than those that begin with digits 2, 3, and so on. The clear result was that more numbers are starting with digit 1 than larger digits. He inferred from this pattern that users are more often look up for digits that begin with low digits (1, 2, or 3), rather than multi-digit numbers beginning with digits 4 to 9. He concluded that the probability that a digit such as “d” appears as the first digit is as EquationEq (1)(1)
(1) .
Today, theoretical distribution about 1 is known by Benford’s law (Amiram et al., Citation2015). Almost fifty years later, independent of Newcomb’s original paper, Frank Benford, a physicist, came to the same conclusion Newcomb had arrived some years ago. He proved that the numbers with smaller leading digits are more than the numbers with larger leading digits. However, he tried to test his hypothesis by collecting and analyzing data. To this end, Benford collected more than 20,000 samples from diverse data sets, including a collection of river areas, atomic weights of elements, and numbers appearing in Reader’s Digest articles (Benford, Citation1938). Benford’s law is not limited to the first significant digit and can be extended to higher digits (Benford, Citation1938).
Diaconis and Freedman (1979) provided convincing evidence that Benford manipulated round-off errors to obtain a better fit to a logarithmic law. But even the non-manipulated data are a remarkably good fit. Benford found that numbers are continually fell into a pattern in which low digits occur more frequently in the first position than larger digits. In mathematics, the frequency of digits is known as Benford’s law (Durtschi et al., Citation2004).
In 1995, a mathematician named Hill provided proof for Benford’s law as well as demonstrating how it applied to stock market data, census statistics, and accounting data. He proved that Benford’s law is constant relative to the scale. He noted that Benford’s distribution, like the normal distribution, is an empirically observable phenomenon Hill’s proof relies on the fact that the numbers in sets that conform to the Benford distribution are combinations of other distributions. If distributions are randomly selected and random samples are taken from each of these distributions, then the digit frequencies of the combined samplings will converge to Benford’s distribution, even though the independent distributions do not precisely follow Benford’s law (Hill, Citation1995). The key is in the combining of numbers from different sources. Chow and Rice (Citation1982) showed that the data set follows Benford’s law when the components of this data result from random variables and various sources such as multiplying, dividing, or raising to integer powers. This explains why certain sets of accounting numbers often accurately follow Benford’s distribution. Accounting numbers are often the result of a mathematical procedure.
As said, today, the law is used in various sciences, including auditing as auditors can use this rule in their numerical analysis as an analytical method (Boyle, Citation1994). On the other hand, there are many incentives, such as revenues based on reported earnings (Boyle, Citation1994), maintaining or increasing stock market prices (Spathis, Citation2002), minimizing tax debt (Spathis, Citation2002), avoiding debt contracts (Spathis, Citation2002) for fraudulent financial reporting that manipulates financial statements. Therefore, fraud detection in financial statements is very important, but since there is not enough information about the features of fraud, fraud detection is not easy through routine business auditing. On the other hand, fraud methods are being updated.
Therefore, the methods for detection and prevention should also be updated. Benford’s Law is one of the updated analytical methods used to detect fraud. Given that fraud makes changes in the numbers of financial statements, therefore, it is expected that the data related to fraudulent companies will not comply with Benford’s law. However, it is expected that the data of the non-fraudulent companies follow Benford’s law, due to not manipulating the numbers of financial statements. Thus, the behavior of data in two groups of fraudulent and non-fraudulent companies should be investigated.
Hence, the present law is expected to provide a valuable framework for estimating the accuracy of data values and to identify anomalous patterns in the data set. Therefore, it is clear that the application of Benford’s law in auditors’ analysis is of particular importance and auditors should use it. Therefore, the research that is related to the subject of the present study and emphasizes the importance of the subject is mentioned below.
3. Research background
Among previous studies on fraud detection, Corazza et al. (Citation2010) examined the frequency distribution of the first digit of the price and daily returns of the index. The results of the Chi-square test at two significant levels of 1% and 5% indicated that the research data did not follow Benford’s law. The results also showed that returns are closer to Benford’s law than the price, and the analysis using Benford’s law can detect abnormal behavior in the capital market. In another study, Nigrini & Miller (Citation2009) used an analytical method of auditing based on Benford’s law. The results of this study showed that Benford’s law could be useful in discovering transactions, events, and unusual trends, and this law can identify signs that other analytical methods of auditing are incapable of identifying.
In the same year, Feltner and Christine Klein used the second form of Benford’s distribution in predicting the frequency of the second digit, along with other statistical methods in the study of psychological barriers. The results of this study showed that there were psychological barriers in research data, but their levels varied in different data. In another study, Papa Nikolaou & Grammatikos (Citation2013) noted that they used Benford’s law to detect fraudulent banking practices. He showed this law can help to find out to what extent there is a distortion in the financial information of banks’ financial statements in the year before the financial scandal. In the same year, in another study, Hanselman and colleagues claimed that the company’s annual report data follow Benford’s law, and this is a kind of tool for helping investors to evaluate risk in their decision-making process.
Lin (Citation2014) examined the relationship between conservatism, institutional investors, and earnings management using Benford’s law. The results of this study indicated that there is an opposite relationship between conservatism and earnings management, and when conservatism increases, profit management decreases. The results also showed that there is a negative relationship between earnings management and institutional investment. In another study, Amiram et al. (Citation2015) examined the deviation from Benford’s law on financial statements and its impact on the capital market, in which the results of this study showed that deviating from Benford’s law would reduce stock returns. Indrabudiman and Hidayat (Citation2016) also investigated the relationship between fraud and Benford law and introduced deviations from Benford’s law as a criterion for detecting fraud in the financial statements. Papanikolaou and Grammatikos (Citation2020) utilized Benford’s Law, which predicts the frequencies in different digits in data, to test if balance sheet and income statement data used to assess bank soundness were manipulated before, and also during, the global financial crisis. They found that banks adjust loan loss provisions to manipulate earnings and income upwards. Distressed institutions that have stronger incentives to conceal their financial difficulties also manipulate loan loss allowances and non-performing loans downwards. Moreover, manipulation is magnified during the crisis and expands to encompass regulatory capital.
Shams Al-dini et al. (Citation2018) stated the importance of using Benford’s law in continuous auditing. The results of this study indicated that the use of Benford Law is necessary for reducing the sampling risk, the cost of profit and its use, and the ability of this law to improve the quality of the information in the continuous auditing. The results of this study showed that the surveyed companies lacked cosmetic earnings management.
Hashemi and Hariri (Citation2017) Evaluated the quality of data in the Tehran Stock Exchange using Benford’s Law in a study entitled “Benford’s Law in Tehran Stock Exchange.” The results of this study indicated that the data of the study do not follow Benford’s law due to the behavioral abnormalities of the capital market. They Identified and predicted the detection of financial fraud using the degree of compatibility and deviation of financial statements from Benford’s law. In this regard, the companies listed in the Tehran Stock Exchange were investigated during 2014. To achieve the research objectives, three hypotheses were developed, and multiple linear and logistic regression was used. The results of the test of the first hypothesis indicated that the distribution of financial statements digits follows Benford’s law; in fact, it can be used to investigate misconduct in financial statements. Based on the findings of the second hypothesis test, the high deviation of financial statements from Benford’s law indicates financial fraud. The results of the third hypothesis show that in the year of fraud detection, the rate of deviation from Benford’s law decreases compared to years before fraud detection; in fact, the inability of managers in manipulating the digits in the year of fraud detection reduced the deviation of financial statements from Benford’s law compared to previous years.
4. Research hypothesis
The foundation theoretical for this study was the information manipulation theory (IMT) .Fraudulent financial reporting violates IMT conversational maxims of quality (untruthful financial reporting due to misrepresentation of material facts), quantity (uninformative financial reporting due to the omission of material facts), relation (irrelevant financial reporting due to unclear disclosure), and manner (ambiguous/unclear financial reporting due to improper accounting practices) (McCornack, Morrison, Paik, Wisner, & Zhu, 2014).
The current study proposed signal detection theory as a tool to test the efficiency and effectiveness (sensitivity) of auditors’ fraud risk assessment (judgment) as a way to minimize fraud misclassification type one and type two errors, and extended the application of information manipulation theory, arithmetic analysis (the Benford’s law) to fraud management. The goal was to provide fraud risk awareness and early monitoring tools to help protect investors and the public from potential fraud losses.
Based on the purpose and theoretical foundations of the study, the following hypotheses are developed:
H1: The frequency distribution of the numbers in financial statements of fraudulent companies is significantly different from Benford’s distribution compared to non-fraudulent companies.
H2: The frequency distribution of the numbers in financial ratios of fraudulent companies is significantly different from Benford’s distribution compared to non-fraudulent companies.
5. Research methodology
The present study is an applied study which is experimental-correlation research since it seeks to discover and determine the relationship between independent and dependent variables and it is also a post-event type. Because of the financial data of a company that is being prosecuted for fraud and its data is publicly available, so it is post-investigation research.
The research method includes data collection and descriptive and inferential statistics. The purpose of the study is to determine the extent and type of relationship between the variables.
The data used in this study are real data and can be generalized to the whole statistical population by inductive method. Considering that historical information has been used in this research and the data related to this research have been obtained through information about companies listed on the stock exchange whose financial statements contain risk of fraud, so this research is in the category the research is quasi-experimental. In this study, following the research of Spathis (Citation2002), Durtschi et al. (Citation2004), and Papa Nikolaou and Grammatikos (Citation2013), Benford’s Distribution was extracted for the numbers in financial statements and financial ratios between companies with a risk of fraud and no risk of fraud in financial statements in the year of fraud; then, their distribution was compared with Benford’s distribution through the Chi-square test. Also, Benford’s distribution of the numbers of financial statements was separately compared in fraudulent and non-fraudulent companies, to test the predictive power of Benford’s law in distinguishing companies with a risk of fraud from no risk of fraud in financial statements. Therefore, the statistical techniques (chi-square test) were used to analyze the probabilities of Benford’s law and the probability of the occurrence of certain digits in a set of numerical data that follow the logarithmic rules.
In this study, excel software was used to classify and calculate the research variables and SPSS software was used to calculate the descriptive statistics of the variables. The data required for the research were collected using databases and the existing software, and the data analysis was performed using excel and SPSS software.
5.1. Statistical society
The statistical population in this study was limited to the Iranian publicly traded firms whose financial statements were filed with the Iran SEC and publicly available at www.codal.ir and the research time frame is 20 March 2016. More noteworthy is the fact that the reason behind the 2016 selection was the greater number of samples in which fraud risk was detected.
5.2. Sampling
Samples were selected based on stratified sampling. In stratified sampling, the statistical society is divided into homogeneous groups; then, the number of samples is determined according to each group. Since the companies listed in the Stock Exchange were classified according to the industry, so the study tried to select at least one fraudulent sample (experimental) and one non-fraudulent sample (control) from each classification.
5.2.1. Sampling conditions
The end of the fiscal year must be the end of March 2015 to increase the comparability.
Sample companies must be not investment companies, holding companies, banks, and financial intermediaries since their activities are different from those of other industries.
The trading interruption of the sample companies must be maximized for three months.
Full details of sample companies in the year under investigation must be available.
During the investigation years, there must be no changes in activities or the fiscal year, and they must be active on the stock exchange during this period and must not be removed from the stock exchange.
They must be listed on the stock exchange before the year 2012, to homogenize the statistical sample in the investigation years.
5.3. Identification and classification of companies
The financial statement fraud can be viewed as a violation of quality, quantity, relation, and manner of the financial reporting due to an intentional misrepresentation or omission of material facts (amounts or disclosures). According to Iranian Auditing Standards No. 240 and 450, based on the amount and content of the data of the financial statements audited by the certified public accountants or the auditing organization, criteria can be provided to consider the distortion.
Considering the effect of tolerable distortion (level of importance) on the type of auditors’ comments and since according to the study (Shams Ahmadi, 2009) the level of importance in auditing the financial statements of companies listed on the Tehran Stock Exchange, auditing firms from different bases and percentages. In determining the level of importance, they have used that they do not have the same procedure in estimating the level of importance, so the acceptable statement alone cannot be considered as a sign of non-fraud of financial statements or minor fraud. Therefore, other criteria such as existence of fraudulent conditions, according to previous research, have been considered as follows:
1. Unacceptable audit comment
2- Existence of tax disputes with the tax field according to the income tax reserve note and the tax file and the condition clause of the audit report.
3. Existence of significant annual adjustments and revised financial statements.
The reasons for choosing the above criteria are as follows:
Table 1. Distribution of selected companies by industry
1- Unacceptable audit comment:
Significant fraud can lead to unacceptable comments. The existence of fraud schemes listed in in the condition of the audit report has been the basis for selecting companies with fraud risk.
Table 2. Distribution of fraud sample by type of fraud schemes
2- Existence of tax disputes with the tax field according to the income tax reserve note and the tax file and the condition clause of the audit report:
Tax disputes are mainly due to misinterpretation of tax laws and misapplication of the relevant provisions of the relevant laws, and in some cases, delays in tax identification and protection of liquidity, and other possible violations.
3- Existence of significant annual adjustments and revised financial statements:
Due to a number of erroneous cases and manipulation of financial statement items, especially income statement items in previous years, has led to the re-presentation of financial statements and a reason for the possibility of fraud in financial statements.
Finally, 50 companies were selected as the sample, including 25 companies with fraud-risk or fraud sample (FS) and 25 companies without fraud-risk or non-fraud sample (NFS) and non-fraudulent companies concerning the industry, the volume of activity, and the value of the fraudulent company (experimental group) in the same fiscal year of the fraud. shows the distribution of the companies as an industry breakdown.
provides a distribution of the fraud sample by fraud schemes. About 85% of the fraud-prosecuted firms misstated (misrepresented) their earnings/income by improper revenue recognition or overstating their sales/revenues. Whereas 20% of the fraud-prosecuted firms provided improper disclosures about their financial statements numbers, presentation, or footnotes. Improper disclosures occur when omitting relevant information or providing inaccurate information to the detriment of the users and violation of the GAAP.
About 16% of the fraud-prosecuted firms inconsistently applied the accounting standards (practices), as required by GAAP, whereas 14% of the fraud-prosecuted firms misstated their inventory balances by either overstating the quantity or the value of their inventory in order to boost their income. In contrast, 10% of the fraud-prosecuted firms understated their liabilities or debts.
About 9% of the fraud-prosecuted firms understated their expenses to boost their income figure and show better performance. Conversely, about 7% of the fraud-prosecuted firms overstated their assets and capitalized some of their costs to show better financial position.
5.4. 5.4 data collection
First, the financial statements of 50 companies (25 experimental groups and 25 control groups) including balance sheet, profit, and loss statement, and cash flow statement were extracted from the publishers informing system (Codal) in 2016. After dividing companies into companies with fraud-risk and no fraud-risk in financial statements based on the index presented in the company’s identification and classification section, the percentage of the frequency distribution for numbers 1 to 9 was separately calculated based on the balance in the financial statements of the companies with fraud-risk and no fraud-risk in financial statements. The percentage of the frequency distribution for numbers 1 to 9 in the first digit on the left side in financial ratios was also extracted from the financial statements of the selected companies.
5.5. Research variables
5.5.1. Dependent variable
The dependent variable of the research is the status of fraud in the financial statements (Fraud), which is qualitative and has a nominal scale. In measuring this variable, 1 is assigned to companies with fraud-risk or fraud sample (FS) based on Identification and classification of companies’ section (5.3) and 0 is assigned to companies without fraud-risk or non-fraud sample (NFS).
5.5.2. Independent variables
Independent variables of the study include the distribution of numbers in financial statements and ratios, and to evaluate Benford’s law more accurately, the distribution of the first left digit in financial ratios of the cost of sale goods, asset turnover, inventory turnover, and the period of payable accounts, extracted from the study of the previous literature, were studied.
(Gupta & Singh, Citation2012) (Santos & Ledur Brito, Citation2012) (Wuerges & Borba, Citation2014) (Kanapickienė & Grundienė, Citation2015) (Goel, Citation2014).
and compare the financial statements first- digit numbers of both fraud-risk companies (FS) and non-fraud-risk companies (NFS) to the Benford’s frequency distributions.
Table 3. First-digit numbers of both fraudulent companies and non-fraudulent companies
and show a comparison of the four financial ratios for both fraud and non-fraud samples to the Benford’s frequency distributions. The goal was to determine if the selected ratios for fraud and non-fraud samples conform to the Benford’s law frequency distributions, and any possible deviation (manipulation).
Table 4. Comparison of the four financial ratios for non-fraud samples to the Benford’s frequency distributions
Table 5. Comparison of four financial ratios for fraud samples to the Benford’s frequency distributions
6. Results
6.1. Test results of the first hypothesis
The first hypothesis of the study was developed as “the numbers of financial statements of fraudulent companies are different from the Benford’s distribution compared to non-fraudulent companies.” Which statistical hypothesis is as follows?
H0: Frequency distribution of the numbers in financial statements of fraudulent companies compared to non-fraudulent companies is not significantly different from Benford’s frequency distribution.
H1: Frequency distribution of the number in financial statements of fraudulent companies compared to non-fraudulent companies is significantly different from Benford’s frequency distribution.
6.1.1. The results of Chi-Square test for companies with no fraud-risk in financial statements
The results of Chi-Square test for 25 companies with no fraud-risk (NFS) in financial statements are presented in .
Table 6. The results of Chi-Square test for NFS
The test results of Chi-Square of financial statement numbers and Benford’s probability distribution for companies with non-fraud-risk in financial statements are shown in .
Table 7. The results of Chi-Square test for companies with no fraud-risk (NFS) in financial statements
The results of Chi-Square test for 25 companies with fraud-risk (FS) in financial statements are reflected in .
Table 8a. The results of chi-square test for FS
The test results of Chi-Square of financial statement numbers and Benford’s probability distribution for companies with fraud-risk in financial statements are shown in .
Table 8b. The results of Chi-Square test for companies with fraud-risk (FS) in financial statements
6.2. The results of the second hypothesis
The second hypothesis of the study developed as “the frequency distribution of the number of financial ratios in fraudulent companies compared to non-fraudulent companies is significantly different from Benford’s distribution.” So the statistical hypothesis will be as follows:
H0: The frequency distribution of the numbers in financial ratios of fraudulent companies compared to non-fraudulent companies is not significantly different from Benford’s distribution.
H1: The frequency distribution of the numbers in financial ratios of fraudulent companies compared to non-fraudulent companies is significantly different from Benford’s distribution.
Chi-square test of the numbers of financial ratios and Benford’s probability distribution for fraudulent companies are shown in .
The results of the Chi-square test of the numbers of financial ratios and Benford’s probability distribution for fraudulent companies are shown in .
Table 9. Chi-square test financial ratios and Benford’s probability distribution for fraudulent companies (FS)
Chi-square test of the numbers of financial ratios and Benford’s probability distribution for no fraudulent companies are shown in .
Table 10. The results of the Chi-square test for fraudulent companies
The results of the Chi-square test of the numbers of financial ratios and Benford’s probability distribution for no fraudulent companies are shown in .
Table 11. Chi-square test of the numbers of financial ratios and Benford’s probability distribution for no fraudulent companies (NFS)
Table 12. The results of the Chi-square test for non-fraudulent companies
6.3. Conclusions
Based on the results of the Chi-square test for the frequency distribution of the numbers in financial statements and ratios of the companies without fraud-risk in the financial statements compared to the Benford’s distribution, concerning the fact that the level of significance is greater than 0.05, no company was identified as fraudulent. Therefore, the frequency distribution of all companies was consistent with Benford’s frequency distribution (prediction accuracy of 100%). The results of the Chi-square test for the frequency distribution of the numbers in financial statements and ratios of the companies with fraud-risk in the financial statements compared to the Benford’s distribution, concerning the fact that the level of significance is greater than 0.05, no company of 25 companies with fraud-risk was identified as fraudulent and the frequency distribution of all numbers and ratios of 25 companies are following Benford’s distribution (Accuracy of 0% prediction). Therefore, Benford’s law cannot predict financial statements fraud or, in other words, it has zero prediction accuracy. Accordingly, Benford’s law cannot categorize fraudulent and non-fraudulent companies. Benford’s law cannot, therefore, complement the analysis of financial ratios and auditing processes as a means of predicting financial statements fraud.
The results of this study were analyzed along with those of (Gava & Vitiello, Citation2014), which studied the predictive power of Benford’s law by comparing the distribution of the occurrences of the first number of the profit and loss, cash flow statements, and balance sheet (observed distribution) with Benford’s law distribution (expected distribution) to determine the significant statistical deviations through the statistical tests Z and Chi-square and finally suggested that Benford’s law is not appropriate to help detect the deviations of financial statements.
According to the results of the investigation, auditors are advised not to rely too much on Benford’s law in their analysis of the possibility of fraud in financial statements. In addition to using this law, use other methods as well. It is recommended to investors, as previous studies in other countries have often shown that Benford’s law can predict financial statements fraud, possibly due to the special nature of corporate governance in Iran and the widespread presence of institutional and government owners in the capital market, companies are using more sophisticated methods of financial statement fraud that Benford’s law cannot predict. Therefore, try to pay attention to the reputation of independent, internal auditors and members of the audit committee in their investments. It is also offered to the Tehran Stock Exchange Organization to form a special working group to study other analytical methods in predicting fraud in financial statements and to prevent fraud from bypassing strict and punitive laws. It is recommended that the auditors of the auditing organization update their knowledge in investigating fraud and tax evasion.
Future researchers are advised to evaluate the accuracy of various statistical methods used to detect financial fraud based on various statistical methods such as system performance characteristic curves. Evaluate the ability to use Benford’s law in tracking down other corporate figures in financial statements such as earnings management. It is recommended that attention be paid to the ownership structure when examining Benford law in anticipating fraud. It is suggested that the present research on different industries be done separately for a specific industry.
Regarding research limitations, the effects due to the factors such as inflation, the difference in accounting methods in measuring and reporting financial events can affect research results; but the effect of these factors has not been considered in the research. In this research, the effects due to the type of industry have not been considered. Regarding the severity and weakness of relations in various industries, in interpreting the results the influence of various industries should be considered. Since this study was conducted in Iran, perhaps based on the inflationary nature of the Iranian economy and weak corporate governance, the results of this study cannot be generalized to other countries.
Additional information
Funding
Notes on contributors
Ali Amiri
Mehdi Rad is currently a PhD Accounting student at the Department of Accounting, Islamic Azad University Bandar Abbas Branch, Iran. His research interest focuses on a financial statement fraud risk and internal auditing.
Ali Amiri holds a PhD in Accounting Degree from the University of Kerala, India. He is Professor Assistance and Faculty member of Islamic Azad University Bandar Abbas Branch, Iran. His research interest includes Financial, Auditing, Managerial and Ethics.
Mohammad Hussein Ranjbar holds a PhD in finance from the university of Mysore, India. He is an assistant professor at the Islamic Azad University Bandar Abbas Branch, Iran. His research interests include finance, financial economics and statistics.
Hojjatollah Salari holds a PhD in accounting degree from the university of Kerala, India. He is Professor Assistance and Faculty member of Islamic Azad University Bandar Abbas Branch, Iran. His research interests include Financial, Auditing, Managerial and Ethics.
References
- Ahmadi, R., & Kordloei, H. (2018). The effect of financial distress on the investment behavior of companies listed on the Tehran stock exchange. Advances in Mathematical Finance and Applications, 3(4), 17–27. https://doi.org/10.22034/amfa.2019.565459.1108
- Amiram, D., Bozanic, Z., & Roen, E. (2015). Financial statement errors: evidence from the distributional properties of financial statement numbers. Review of Accounting Studies, 20(4), 1540–1593. http://dx.doi.org/10.1007/s11142-015-9340-0
- Benford, F. (1938). The Law of Anomalous Numbers. Proceedings of the American Philosophical Society, 78(4), 551-572. Retrieved from http://www.jstor.org/stable/984802
- Boyle, J. (1994). An application of Fourier series to the most significant digit problem. The American Mathematical Monthly, 101(9), 879–886. https://doi.org/10.1080/00029890.1994.11997041
- Chow, C. W., & Rice, L. S. (1982). Qualified audit opinions and share prices-An investigation, Auditing. A Journal of Practice and Theory, 1, 35–53. https://doi.org/10.1016/0165–4101(84)90018–1
- Corazza, M., Ellero, A., & Zorzi, A. (2010). Checking financial markets via Benford’s law: the S&P 500 case. In Mathematical and statistical methods for actuarial sciences and finance (pp. 93-102). Springer, Milano. https://doi.org/10.1007/978-88-470-1481-7_10
- Durtschi, C., Hillison, W., & Pacini, C. (2004). The effective use of Benford’s law to assist in detecting fraud in accounting data. Journal of forensic accounting, 5(1), 17-34.
- Farzad, S., Malekian, E., Fakhari, H., & Ghasemi, J. (2018). Predict the stock price crash risk by using a firefly algorithm and comparison with regression. Advances in Mathematical Finance and Applications, 3(2), 43–58. https://doi.org/10.22034/amfa.2018.540830
- Gava, A. M., & Vitiello, L. (2014). Inflation, quarterly balance sheets, and the possibility of fraud: Benford’s law and the Brazilian case. Journal of Accounting, Business & Management, 21(1), 43–52.
- Goel, S. (2014). The quality of reported numbers by the management. Journal of Financial Crime, 21(3), 355. https://doi.org/10.1108/JFC-02-2013-0011
- Gupta, R., & Singh, G. N. (2012). A data-mining framework for prevention and detection of financial statement fraud. International Journal of Computer Applications, 50(8), 7–14. https://doi.org/10.5120/7789-0889
- Hashemi, S., & Hariri, A. (2017). The analysis of Benford’s law ability to identify and predict financial fraud detection. Accounting and Auditing Review, 24 (2), 283–302. (In Persian)https://doi.org/10.22059/acctgrev.2017.220346.1007490.
- Hill, T. P. (1995). A statistical derivation of significant digit law. Statistical Science, 10(4), 354–363. https://doi.org/10.1214/ss/1177009869
- Indrabudiman, A., & Hidayat, T. (2016). Analysis Benford law for company evidence from Indonesia. International Journal of Engineering Sciences & Research Technology, 5(11), 1187–1207. https://doi.org/10.5281/zenodo.48844
- Kanapickienė, R., & Grundienė, Ž. (2015). The model of fraud detection in financial statements by means of financial ratios. Procedia-Social and Behavioral Sciences, 213, 321-327. https://doi.org/10.1016/j.sbspro.2015.11.545
- Khani, A. (2007). Official Fraudulent Activities and the Future of the Accounting Profession. Quarterly Journal of Auditor, 38, 44-48.
- Lin, L. (2016). Institutional ownership composition and accounting conservatism. Review of Quantitative Finance and accounting, 46(2), 359-385. https://doi.org/10.1007/s11156-014-0472-2
- Nia, S. H. (2015). Financial ratios between fraudulent and non-fraudulent firms: evidence from Tehran stock exchange. Journal of Accounting and Taxation, 7(3), 38–44. https://doi.org/10.5897/JAT2014.0166
- Nigrini, M. J., & Miller, S. J. (2009). Data diagnostics using second-order tests of Benford’s law. Auditing: A Journal of Practice and Theory, 28(2), 305–324. https://doi.org/10.2308/aud.2009.28.2.305
- Papa Nikolaou, N. I., & Grammatikos, T. (2013). Using Benford’s law to detect fraudulent practices in banking, working paper. Sussex University, Luxemburg University.
- Grammatikos, T., & Papanikolaou, N. I. (2020). Applying Benford’s Law to Detect Accounting Data Manipulation in the Banking Industry. Journal of Financial Services Research, 1-28. https://doi.org/10.1007/s10693-020-00334-9
- Pazarçeviren, S. Y. (2005). Adli muhasebecilik mesleği. Uluslararasi Yönetim Iktisat Ve Isletme Dergisi, 1(2), 1–19. https://dergipark.org.tr/en/pub/ijmeb/issue/54839/750849
- Rezaee, Z., & Davani, H. (2013). Does financial reporting fraud recognize borders? Evidence from bank fraud in Iran. Journal of Forensic & Investigative Accounting, 5(2). https://ssrn.com/abstract=2469020
- Santos, J. B., & Ledur Brito, L. A. (2012). Toward a subjective measurement model for firm performance. BAR - Brazilian Administration Review, 9(2), 95–117. http://dx.doi.org/10.1590/S1807-76922012000500007
- Shams Al-dini, K., Daneshi, V., & Mahallati Rayni, M., . A. (2018). Benford law’s ability to detect fraud in both profit or loss and balance sheet. Auditing Knowledge, 18 (73), 166–187. (In Persian)
- Spathis, C. (2002). Detecting false financial statements using published data: some evidence from Greece. Managerial Auditing Journal, 17(4), 179–191. https://doi.org/10.1108/02686900210424321
- Taherinia, M., & Rashidi Baghi, M. (2019). Prediction the return fluctuations with artificial neural networks’ approach’. Advances in Mathematical Finance and Applications, 4(2), 103–114. https://doi.org/10.22034/amfa.2019.580643.1149
- Wuerges, A. E., & Borba, J. A. (2014). Accounting Fraud: An estimation of detection probability. Revista Brasileira De Gestão De Negócios, 16(52), 466–483. https://doi.org/10.7819/rbgn.v16i52.1555