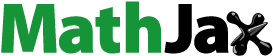
Abstract
The paper examines how loan portfolio diversification drives bank returns, mainly focusing on the conditioning roles of business models and market power in this nexus. We employ a sample of Vietnamese commercial banks from 2008 to 2019 to perform regressions in the dynamic panel models with the two-step system generalized method of moments (GMM) estimator. We find that increased sectoral loan portfolio diversification reduces bank returns, but not all banks are equally affected. Banks that adopted a business model towards non-interest activities are hurt less from loan portfolio diversification, and bank market power may mitigate the detrimental effects of loan portfolio diversification on bank returns. When such asymmetric effects are sizeable, neglecting them could miscalculate the choice of loan portfolio diversification. Our findings are robust to a rich set of bank return indicators and alternative loan portfolio diversification measures based on the Herfindahl-Hirschman (HHI)/Shannon Entropy (SE) indexes with different sectoral exposure profiles. Thus, both regulators and commercial banks should take the disadvantage of portfolio diversification into account when encouraging/pursuing a diversified strategy, which must be accompanied by the crucial caveat that the damage is most pronounced for banks with lower shares of non-interest income and less market power.
PUBLIC INTEREST STATEMENT
Should banks diversify their loan portfolios? Most existing studies have been only concerned about the average impact of loan portfolio diversification on bank performance, but not considering the heterogeneous effects across different bank groups. The paper examines how sectoral loan portfolio diversification drives bank returns, particularly focusing on the conditioning roles of business models and market power. Using a sample of Vietnamese commercial banks, we find that not all banks are equally affected by loan portfolio diversification: (i) less hampered banks are those that have moved away from traditional lending activities towards a business model that produces more non-interest income, and (ii) bank market power may mitigate this detrimental impact of loan portfolio diversification on bank returns. These findings point to the heterogeneity across banks in terms of information resources and competitiveness, which could speak to the causal effects of loan portfolio diversification.
1. Introduction
Should banks diversify loan portfolios or specialize in only some economic sectors? This topic has attracted much attention from academia and regulators over the years. Fundamentally, corporate finance theory indicates that banks should specialize in only a few economic sectors to seize the comparative advantage of managerial expertise and experience (Denis et al., Citation1997). By granting loans to several sectors, banks could better evaluate and monitor their customers and collateral values, which might reduce the adverse selection problem. Furthermore, portfolio diversification seems not to be an attractive strategy for any bank as it could enhance competition with other banks (Jensen, Citation1986). In sharp contrast, traditional banking theory calls for the pursuit of loan portfolio diversification. Accordingly, banks should diversify their credit portfolio to diminish asymmetric information and further reduce financial intermediation costs (Diamond, Citation1984). This theory also argues that less diversified banks are more vulnerable to the economic downturn because of their limited exposures to only a few economic sectors. In practice, during the last decades, many banking crises have also raised the concern that bank collapse is highly associated with concentration, so commercial banks should diversify their loan portfolios to avoid idiosyncratic shocks (Chen et al., Citation2014).
Though the impact of loan portfolio diversification on bank returns is a well-established concept in the banking literature, its empirical analysis has been limited in context and scope thus far. First, the cost-benefit implications of loan portfolio diversification have been mainly addressed for advanced economies. Concretely, exploring the banking sectors in Italy and Germany, many studies find portfolio concentration to be associated with more profit gains and less risk-taking, thus highlighting the diseconomies of diversification (Acharya et al., Citation2006; Behr et al., Citation2007; Hayden et al., Citation2007; Jahn et al., Citation2013). However, some competing effects dominated in other advanced markets. Rossi et al. (Citation2009) reveal that portfolio diversification reduces bank risk and increases profit efficiency for Austrian banks. Using a sample of US banks, Shim (Citation2019) shows that banks could promote financial stability when diversifying their loan portfolios across multiple economic sectors. Only a few papers investigate emerging markets and even exhibit mixed results. Analyzing the banking sector in Brazil, Tabak et al. (Citation2011) encourage loan portfolio concentration as this strategy completely improves bank performance (more returns and lower risks of default). Focusing on Chinese banks, Chen et al. (Citation2014) indicate a risk-return tradeoff of loan portfolio diversification in the way that it reduces bank profits and also mitigates bank risks simultaneously. Unlike banks in advanced economies, the available breakdown of loan portfolios is restricted at banks in emerging markets.
Second, almost all of the existing studies have been only concerned about the overall average impact of loan portfolio diversification on bank performance, but not considering the heterogeneous effects across different bank groups. Many prior authors have shown that the lack of expertise, information resources, and competitiveness capacity could explain the costs of loan portfolio diversification, but they do not provide additional evidence to shed further light on such reasons (e.g., Acharya et al., Citation2006; Behr et al., Citation2007; Hayden et al., Citation2007; Jahn et al., Citation2013; Tabak et al., Citation2011). If these aspects are at the center of the functioning of loan portfolio diversification, it should be expected that banks’ response to portfolio changes would differ according to heterogeneous bank characteristics.
Hence, more works should be conducted to fill the literature gaps. Primarily, we need to be interested in the heterogeneity across banks to shed further light on the impact of loan portfolio diversification on bank outcome. The critical idea is that some banks could be more affected by portfolio changes than others, so their different earning-making behavior in response to portfolio diversification could speak to the causal effects. In this regard, one moderating factor that should be examined is business models, in particular the shifts toward the non-interest banking activities. Theoretically, banks could reduce the asymmetric information problem by developing close relationships with their customers based on multiple interactions over time, regularly in the form of supplying numerous banking services (Boot, Citation2000). The useful information obtained through non-interest activities allows banks to assess their existing and potential customers more accurately, thereby improving loan quality and attracting trustworthy customers in the future (Baele et al., Citation2007). Concretely, Puri et al. (Citation2012) show that borrowers with prior non-traditional relationships tend to default less subsequently. Besides, the banking literature has also extensively indicated the benefits of non-traditional activities, such as profit improvement, credit risk reduction, financial stability gains, and lower probability of bank collapses (Abedifar et al., Citation2018; DeYoung & Torna, Citation2013; Köhler, Citation2014; Li & Zhang, Citation2013). Taken together, banks may benefit from a business model that relies more on non-interest income while expanding their loan portfolios into multiple economic sectors.
However, the literature also suggests various downsides of non-interest activities that could be detrimental to bank loan portfolios. Switching costs of most non-interest activities are lower than those of traditional lending activities (Deyoung & Roland, Citation2001). To build up longer-term relationships with customers based on available short-term connections, banks may relax their loan granting and monitoring standards. Lepetit et al. (Citation2008) document that banks tend to disregard credit risk when they desire to generate more non-interest income. This finding concurs with the argument of Abedifar et al. (Citation2018), who hypothesize that a business model more involved in non-interest income, alternatively speaking less lending exposure, could encourage banks to behave less conservatively with their loan portfolios. Besides, both diversification across banking activities and within lending segments could jointly lead to diseconomies of scope, which damages bank performance (Boot & Ratnovski, Citation2016).
Another aspect that could drive the differential effects of loan portfolio diversification on bank returns is bank market power. Even though there is no empirical work about the moderating role of market power in the relationship between loan portfolio diversification and bank performance, we are aware of some interesting theoretical literature that allows us to think of a potential mechanism. Under the “competition-fragility” hypothesis, market power could improve market discipline, thereby discouraging banks from excessive risk-taking (Hellmann et al., Citation2000; Keeley, Citation1990). Taking advantage of more abundant information resources, banks with greater market power may screen and monitor their borrowers more effectively (Allen & Gale, Citation2004). Dell’Ariccia et al. (Citation2012) argue that some banks could loosen their lending conditions when they are forced to compete with other dominant banks, who do not need to act similarly. Furthermore, during stressed times, more powerful banks possess enough resources to lower interest rates, thereby mitigating borrowers’ insolvency (Petersen & Rajan, Citation1995). Overall, these may result in excellent support for banks to better exploit economies of scope.
A competing view, according to the “competition-stability” hypothesis, suggests that market power may hurt bank activities in some routes. Greater market power could account for increased lending rates, which amplifies borrowers’ risk-shifting incentives (Boyd & De Nicoló, Citation2005). Lucchetta and De Nicoló (Citation2011) develop a model showing that a perfectly competitive market, i.e., there is no bank capable of dominating the market, is optimal in promoting the safety and soundness of the banking system. Thakor (Citation2005) claims that the lack of competition in the market also makes it more difficult for bank owners to evaluate the real efficiency of bank management. This situation could lead to inaccurate decisions, causing bank risks.
This paper empirically investigates whether and how loan portfolio diversification affects bank returns, particularly highlighting the conditioning roles of business models and market power in this nexus. We employ the banking data of Vietnamese commercial banks in the period of 2008–2019 to achieve the research objectives. To ensure the robustness of findings obtained from the dynamic panel models, we use multiple alternative variables of primary interest in the regression treatment. For loan portfolio diversification, we consider two traditional diversification measures, including the Herfindahl-Hirschman index (HHI) and the Shannon Entropy index (SE). Regarding bank return indicators, we analyze a rich set of accounting ratios, namely, return on assets (ROA), return on equity (ROE), and net interest margin (NIM). The non-interest income share and the Lerner index are the appropriate indicators that account for business models and bank-level market power in this study.
The banking sector in Vietnam offers a favorable laboratory to explore the current topic. The capital market in Vietnam is still underdeveloped, and the central engine to fuel economic growth dramatically depends on bank lending (Dang & Huynh, Citation2020). Therefore, it is of importance to study bank lending and, in particular, the structure of loan portfolios in this market. Additionally, the banking sector in Vietnam has experienced many significant reforms over the years, featured by the shifts from specialized to diversified business models, the promotion of non-interest activities alongside traditional lending, and the strengthening of competitiveness and market power in domestic banks amid the era of global financial integration. Given the significant variability in factors we are interested in, we could expect the elements of robustness and generality with our estimation results. Moreover, focusing on a single market might help us well explain the impacts of loan portfolio diversification within a uniform economic environment.
In these settings, our paper contributes to the existing banking literature in two important ways. First, we extend the portfolio diversification literature by focusing on a small emerging market, in the context that most prior works have been mainly interested in banking sectors in advanced economies. Banks in emerging markets have less mature levels and different regulatory backgrounds, so analyzing their performance’s response to loan portfolio diversification could elicit a better understanding of the current topic. Second, we offer more insight into the impact of loan portfolio diversification on bank returns by investigating its conditionality. Given that the existing empirical documents have disregarded the heterogeneity across banks while considering whether diversifying loan portfolios is an appealing strategy, this paper is the first to examine the conditioning role of business models and market power in the loan portfolio diversification-bank returns correlation. Importantly, when such asymmetric effects are sizeable, neglecting them miscalculates the choice of loan portfolio diversification.
We structure the remainder of this paper as follows. Section 2 presents the Vietnamese banking sector’s background, highlighting the development of lending activities over the years. Section 3 describes the methodology employed and data collected to perform the regression analysis. Section 4 reports and discusses the results obtained using the regression design proposed. Finally, section 5 concludes and displays insightful implications directly drawn from the main findings in the paper.
2. The evolution of the Vietnamese banking sector
Over the past 30 years, the Vietnamese banking sector has experienced various reforms, significantly transforming from policy-driven and specialized business to market-oriented and diversified business. This section summarizes and highlights the key reforms and the evolution of banking activities in Vietnam, which might contribute to our analysis of loan portfolios, bank business models, and market power in this study.
Prior to 1990, the State Bank of Vietnam (SBV) performed the functions of a central bank and a national commercial bank. A law enacted in 1990 separated these functions and required the new establishment of four wholly state-owned commercial banks to take over commercial banking operations.Footnote1 Accordingly, each bank specialized in a different economic sector, including the industry and trade, agriculture, international commerce, and investment and construction. The SBV’s operations shifted to a central bank’s real nature, covering monetary policy implementation, foreign exchange management, and supervision of credit institutions. Simultaneously, the government also allowed the foundation of private commercial banks and the limited presence of foreign participants in the form of joint venture banks and foreign bank branches. In general, commercial banks at this time in Vietnam operated as specialized institutions, as they focused their lending on only one or a few economic sectors.
Multiple subsequent banking reforms have been driven by Vietnam’s accession to international trade and investment agreements, most notably its entry to the World Trade Organization (WTO) in 2007. Deregulations in the banking sector gradually loosened market entry conditions for foreign participants. In 2008, the SBV first licensed wholly foreign-owned banks to enter the market. The growing presence of foreign participants has spurred reforms to enhance the competitive capacity and financial power of domestic banks, especially state-owned banks. Typical reforms included the partial privatization of state-owned commercial banks and the strengthening of bank capital. In 2011, the SBV continued its deregulation policy by allowing foreign bank branches to be treated equally as domestic banks (Ho & Baxter, Citation2011).
There were periods when banks allocated their loans to several sectors with high-profit expectations but containing many risks. For example, in 2008, the SBV warned 21 private commercial banks with an excessive proportion of outstanding loans in the real estate sector by the end of 2007. In response to the SBV’s tightening lending policies to potentially risky sectors, Vietnamese banks reported reduced real estate loans since 2009. Besides, the SBV also actively issued guidelines to encourage banks to increase lending in some priority areas, in line with the government’s economic development orientation.
Since 2012, the government enforced the “Decision No.254” project to comprehensively restructure the entire system of credit institutions in Vietnam. This project aims to upgrade the financial market and improve the efficiency of credit institutions, especially commercial banks, to serve economic development. In some detail, apart from solving the problems of bad debts and capitalization, the banking sector was encouraged to diversify its activities and increase non-lending income sources. These encouragements have fostered the extension of non-interest activities in recent years (Dang, Citation2020).
Overall, initially specialized banks have gradually modified their core operations to get involved in all economic sectors and offer a diverse range of financial services to the economy now. Despite multiple reforms and diversification of banking activities, the banking sector in Vietnam still highlights the dominant positions of the “big four” state-owned banks, while the market share of foreign participants remains considerably small.
3. Data and methodology
3.1. Sample data
The Vietnamese banking system consists of policy banks (non-profit banks) and commercial banks. The operation regime of non-profit policy banks differs significantly from that of commercial banks, so we do not pay attention to them in this study. In this regard, we manually collect data of Vietnamese commercial banks through financial statements published annually from 2008 to 2019. We utilize the financial statement footnotes to obtain the required information on sectoral loan portfolios. We eliminate banks that have been compulsorily acquired or under special control by the SBV due to the extreme difference in business orientations (four banks at the year-end of 2019). We also ignore joint-venture and foreign-owned banks because such banks are considerably small and account for a minor part of the Vietnamese banking system (two joint-venture banks and nine foreign-owned banks at the year-end of 2019). Most importantly, these excluded banks do not publish information on loan portfolios’ breakdown, a core item in this study.
Ultimately, we reach an unbalanced panel data consisting of 30 Vietnamese commercial banks, with the number of observations ranging from 259 to 356 depending on each available variable. Our sample creates an unbalanced panel, covering over 90% of the banking system’s total assets in Vietnam in any given year. To mitigate the impact of extreme outliers, we winsorize bank-specific variables at the 2.5% and 97.5% levels. Besides, to obtain macroeconomic data (including the annual inflation rate and the GDP growth rate of Vietnam), the study accesses the World Development Indicators’ data sources.
3.2. Variables
3.2.1. Bank returns
Consistent with most studies investigating the effectiveness of loan portfolio diversification, we first use two traditional accounting measures, including ROA and ROE, as bank return measures. Besides, supplementing previous studies, this paper employs net interest margin as an additional proxy for bank profits. Further importantly, the use of this measure is also to directly verifies the view that the high level of competition in some economic sectors may force banks to cut lending rates so that they could attract more customers when diversifying their loan portfolios (Jensen, Citation1986), which might hamper bank interest income. The consistency in regression results across three different return measures could produce great confidence in the main conclusions of this study.
3.2.2. Loan portfolio diversification
In line with almost any paper on the same topic, the study utilizes the HHI and SE indicators to measure banks’ loan portfolio diversification (e.g., Behr et al., Citation2007; Lydia et al., Citation2017; Tabak et al., Citation2011). Before illustrating how we precisely compute portfolio diversification measures, we determine the relative exposure of bank at time
to each economic sector
as follows:
Accordingly, the measure of loan portfolio diversification following the HHI index of bank at time
is defined:
where is the number of economic sectors in each loan portfolio. The greater value of the HHI measure implies that the bank has a higher level of loan portfolio diversification. In some detail, the value 0 of the HHI measure represents an extremely specialized bank, in which all loans are assigned to only one economic sector. In contrast, the value of (1–1/n) describes a bank with a perfectly diversified loan portfolio, suggesting all equal exposures to economic sectors.
Besides, the additional use of the SE index is to check whether our regression results are sensitive to the choice of different portfolio diversification measures. Hence, we build up the SE measure using the formula as:
The SE measure ranges from 0 to ln(n), in which a larger value indicates a more diversified loan portfolio. The lower bound indicates a perfect specialization scenario, while the upper bound denotes a perfect diversification case.
Due to the heterogeneous banking profiles in research markets, prior works indicate slightly different ways of calculating loan portfolio diversification measures. For instance, Acharya et al. (Citation2006) design a disaggregated sector decomposition based on each bank’s top five industrial exposures with a sixth exposure covering the sum of the remaining exposures (where some exposures could be to any of the 23 industries). Hayden et al. (Citation2007) use the German classification system with 23 economic sectors, noting that these sectors account for the largest outstanding loans at most banks surveyed. For the Brazilian market, thanks to the standardization of financial information published by all banks, Tabak et al. (Citation2011) efficiently compute consistent diversification measures with 21 classified economic sectors. Regarding the Chinese market, Chen et al. (Citation2014) start from original loan portfolios with 13 industries, then make some adjustments to reduce to 9 industries before calculating their portfolio diversification measures.
We have to pay careful attention when setting up the formats of calculating sectoral loan portfolio diversification measures in the Vietnamese banking context. Unlike banks in many developed markets using standard sector classification systems and publishing adequate financial information, Vietnamese banks show no consensus in dealing with their sectoral loan portfolio structures. More concretely, the number of economic sectors that banks are exposed to varies among banks. Given this practice, we thus follow the seminal empirical paper of Acharya et al. (Citation2006) to restructure Vietnamese banks’ sectoral loan portfolios. From an empirical standpoint, this study divides the loan portfolios into six sectoral exposures, including top five sectoral exposures and a sixth exposure containing the sum of all remaining exposures. Taking a step further than Acharya et al. (Citation2006), to ensure that the random structure in this manner does not affect the robustness of our study, we also apply alternative approaches with eight and ten sectoral exposures. In sum, we create a rich set of loan portfolio diversification variables: (i) three HHI measures with HHI10 (ten exposures), HHI8 (eight exposures), and HHI6 (six exposures); (ii) three similar SE measures with SE10 (ten exposures), SE8 (eight exposures), and SE6 (six exposures).
For the development of portfolio diversification variables, after collecting detailed data on the sectoral loan portfolio of each bank, we arrange outstanding loan items by sector from maximum to minimum. We then take ten, eight, and six sectoral exposures, respectively, as discussed previously (the “last sectoral exposure” is the sum of all remaining outstanding loan items in the portfolio). With this approach, the set of sectoral exposures may be theoretically not the same during years. However, based on Acharya et al. (Citation2006)’s arguments and the banking practice in Vietnam, we realize that the set of sectoral exposures (ten, eight, and six sectoral exposures) does not change significantly and mainly contains several key sectors.
The findings from both theoretical and experimental literature on the effects of loan portfolio diversification on bank returns have been ambiguous thus far. In the context of a nascent banking system like Vietnam, banks in general still have limited resources to exploit diversification advantages. Therefore, we expect the portfolio diversification variables to be negatively associated with bank returns.
3.2.3. Business models and market power
Business models
Following existing studies concentrating on non-interest banking activities (Demirgüç-Kunt & Huizinga, Citation2010; Hidayat et al., Citation2012; Kamani, Citation2019; Köhler, Citation2014), this paper estimates the shifts of banks’ business activities towards non-interest segments by the ratio of non-interest income to total operating income. In the Vietnamese banking context, non-interest income comes from service activities, foreign exchange trading, securities investments, and other non-interest business lines. Meanwhile, operating income consists of two main components, net interest income and non-interest income.Footnote2 Regarding the data collection, we filter and retain only banks with non-negative income components. This procedure ensures that the measure of non-interest activities or business models is restricted to a range of 0 to 1 (Meslier et al., Citation2014).
Banking theories propose that the expansion of revenue into non-interest segments may help banks reduce informational asymmetry, thus improving loan monitoring and increasing the chance of reaching potential borrowers (Boot, Citation2000). So, we expect that a business model that promotes non-interest income could mitigate the adverse effects of loan portfolio diversification on bank profits.
Market power
Assessing bank market power is a topic that has attracted much attention from academia so far. Concentration ratios (such as based on the HHI index) or the market share of leading banks are commonly used, but they are not accurate measures while attributing market share or concentration level to bank market power (Bikker et al., Citation2012). Furthermore, they are market-level measures. Given this context, the Lerner index shows the advantage of being a bank-level measure of market power, offering a bank-specific assessment and comparison between different banks in the system. Therefore, this study employs the Lerner index as a proxy for bank market power, in line with various previous studies (Berger et al., Citation2009; Fu et al., Citation2014; Maudos & De Guevara, Citation2007; Turk Ariss, Citation2010).
The Lerner index is defined as the ratio of the difference between the output price and the marginal cost to the output price. The output price is calculated by the ratio of total revenue to total assets of each bank. More complicatedly, the marginal cost is estimated using the translog cost function for the prices of one output (total assets) and three inputs (labor, physical capital, and finance). Concretely, we proceed to estimate the translog cost function for panel data with bank fixed effects as follows:
where represents the total costs of a bank and
is the total assets of a bank.
indicates the labor price (the ratio of personnel expenses to total assets),
indicates the physical capital price (the ratio of other non-interest expenses to fixed assets), and
indicates the financing price (the ratio of interest expenses to total deposits). All of these quantities are log-transformed before entering the cost function. We then use the estimated coefficients of the cost function to calculate the marginal cost as follows:
Once both the marginal cost and the output price are available, we employ the following equation to compute the Lerner index for each bank and thereby derives the direct value of bank market power:
The Lerner index ranges from 0 to 1, in which higher values indicate larger market power. The Lerner index displays the extent to which a bank gains the market power to set its price above the marginal cost.
Considering the two opposing theories of “competition-fragility” and “competition-stability”, we realize that market power could lead to heterogeneous behavior among banks. However, in the Vietnamese banking market, a bank with larger market power tends to gain the advantages in competitive capacity, business resources, expertise, and experience. Hence, assuming that these advantages might support banks with greater market power in loan portfolio diversification, we expect that the adverse impact of loan portfolio diversification on bank returns should be more pronounced at banks with less market power.
3.2.4. Control variables
In the model specification of bank returns, we control for bank capital (the ratio of bank equity to total assets), because more capitalized banks may grant loans cautiously and borrow less, thus decreasing their costs and increasing their profits (Tan, Citation2016). We also introduce the ratio of liquid assets to total assets to capture bank liquidity positions and the natural logarithm of total assets to measure bank size. We expect bank liquidity to be negatively correlated with bank returns, as more liquid assets regularly produce lower returns than less liquid assets (Dermine, Citation1986). As for bank size, larger banks may have a higher yield, since they could take greater advantage of economies of scale (Carter & McNulty, Citation2005; Hughes & Mester, Citation1998).
Apart from bank-level factors, we also allow for the macroeconomic variables in explaining bank returns. The business cycle, captured by the growth rate of gross domestic product (GDP) and the inflation, captured by the annual inflation rate, are considered as key determinants of bank performance. During the economic upturn, the demand for financial services tends to increase, thus improving bank returns (Athanasoglou et al., Citation2008; Dietrich & Wanzenried, Citation2014). Bank performance can also be affected by inflation under a mechanism in which inflation plays a critical role in shaping interest rates. For example, a higher inflation rate might lead to higher interest rates on loans, which potentially enhance bank profits (Pervana et al., Citation2015).
3.3. Model specification and econometric method
To empirically analyze the impact of loan portfolio diversification on bank return, we approach the dynamic panel model proposed by Acharya et al. (Citation2006) and Tabak et al. (Citation2011), in which we expand with interaction terms to explore the conditioning roles of business models and market power. Hence, the model is specified as follows:
where captures bank dimension and
denotes time dimension.
is the dependent variable, measuring bank returns.
indicates loan portfolio diversification variables, illustrated by either the HHI or the SE indicators.
is a vector of bank-level control factors,
comprises of macroeconomic control variables, and
is the error term.
represents bank business models and market power, separately. The regression coefficient on the
×
interaction term exhibits the differential impact of loan portfolio diversification on bank profitability across heterogeneous bank groups. Opposite signs between the regression coefficients of the standalone
variable and the interaction term imply a weakening role of business models or market power, while similar signs support the strengthening effect of these factors.
The presence of the lagged dependent variable is to adopt the dynamic nature or, in other words, to highlight the persistence in bank earning-making behavior. We take one-period lags of all independent variables with the purposes to (i) reduce the endogeneity problem caused by the causality between the explanatory variables and the dependent variables, and (ii) record that banks take time to absorb the changes in their balance sheet structure and the macroeconomic situation, before translating them into business outcomes.
The paper applies the generalized method of moments (GMM) estimator to estimate our dynamic panel model. This estimator is robust to possible multicollinearity, heteroskedasticity, and autocorrelation issues (Greene, Citation2012). Notably, the endogeneity problem (caused by the reciprocal relationship between explanatory and dependent variables or the omission of variables) could be well tackled by the GMM dynamic estimator. There are two different versions of the dynamic panel GMM estimator: (i) the difference GMM estimator performs regressions on the converted equation in the first-difference form, using variables in levels as instruments (Arellano & Bond, Citation1991), and (ii) the system GMM estimator conducts regressions on both primitive and transformed equations (level and first-difference equations), employing variables in levels and differences as instruments (Arellano & Bover, Citation1995; Blundell & Bond, Citation1998). This study prefers the system GMM estimator since it has been improved on the basis of the difference GMM version to yield better estimates (Roodman, Citation2009). The two-step estimator is also selected because it is more efficient than the one-step version, especially for the system GMM estimator (Windmeijer, Citation2005). To avoid the problem of “too many instruments” in the GMM estimator that could destroy the power of regressions, we limit the proliferation of instruments using the procedure proposed by Roodman (Citation2009), based on the rule that the number of created instruments should not exceed the number of banks. The usage of the dynamic system GMM estimator is justified by mandatory diagnostic tests, namely, the Hansen test and the Arellano-Bond tests. The null hypothesis of the Hansen test is that the instruments employed are not correlated with the residuals, meaning that the set of instruments is valid. The null hypothesis of the Arellano-Bond tests is that the errors in regressions display no first- and second-order autocorrelation.
4. Results and discussions
4.1. Descriptive statistics and correlations
exhibits summary statistics for all variables employed. The means of ROA, ROE, and NIM are 0.904%, 9.816%, and 3.866%, respectively. The statistical distribution of these variables indicates the considerable variations in banks’ performance (reflected in the large values of the standard deviations and the differences between extreme values).
Table 1. Descriptive statistics of variables
The scale of non-interest income of the Vietnamese banking system has continuously increased in recent years, while its share of the total operating income has shown various fluctuations. Looking at , we realize that this share continuously decreased, from 25.22% in 2008 to 14.66% in 2011, before increasing to 17.10% in 2012. After a period of fluctuations afterward, the share of non-interest income steadily increased since 2015, from 15.64% to 24.65% in 2019. The average share of non-interest income in the Vietnamese banking sector during the research period is 20.10%. This share is much lower than those in developed economies and other emerging markets (Doan et al., Citation2018), regardless of multiple efforts to improve this income source in the past years.
Figure 1. The evolution of the yearly average NIIshare measure and Lerner index during 2008–2019 in the Vietnamese banking system

The Lerner index assessing Vietnamese commercial banks’ market power has also shown many fluctuations during the research period (). The average annual Lerner index dropped significantly between 2009 and 2013, suggesting a depression in bank market power, or in other words, a higher level of competition in the banking system in this period. Our observation is relevant in the context that, after the reform process, the number of banks in Vietnam increased rapidly, making the level of competition in the market fierce and some banks no longer retained their dominant positions. However, after the adjustment process since 2013, Vietnamese banks’ market power tended to increase continuously and stably, potentially implying some banks established their positions and confirmed their strength in the marketplace. The Lerner index of our bank sample averages 0.185. If we compare the Vietnamese banks’ market power with the findings of the literature, we could realize that in general it is weaker than those of banks in countries of the same region, such as Malaysia (0.431), Indonesia (0.265), Philippines (0.317) and Thailand (0.362) (Fu et al., Citation2014).
displays the yearly average HHI and SE diversification measures (illustrated with HHI6 and SE6) during 2008–2019. The two portfolio diversification measures exhibit similar paths, which grew significantly from 2008 to 2011. This observation demonstrates that Vietnamese banks diversified their loan portfolios more in this period, in line with the practice that banking competition increased after market opening policies, and banks experienced more exposures to many economic sectors. Since 2011, however, banks’ loan portfolios decreased diversification levels, and both HHI and SE measures showed continuous ups and downs until 2019.
Figure 2. The evolution of the yearly average HHI6 and SE6 diversification measures during 2008–2019 in the Vietnamese banking system

presents the pairwise correlation coefficients between variables employed. The correlations between loan portfolio diversification variables are significantly high as all coefficients are greater than 0.90. Regarding bank return measures, there are also high correlations among ROA, ROE, and NIM (coefficients range from 0.44 to 0.79). These observations suggest that it is appropriate to use alternative variables to measure the same respect in the paper. Furthermore, we realize that our approach in establishing different portfolio diversification measures does not considerably alter the assessment of diversification levels. Besides, we see that the explanatory variables are not highly correlated with each other, except for the large correlation coefficient between bank size and capital (−0.730). However, this is not a concern when: (i) correlations between variables above 0.80 do indeed cause severe multicollinearity (Franke, Citation2010); (ii) further checks using the variance inflation factor (VIF) indicate that the VIF value is less than 10Footnote3; (iii) the GMM estimator is robust to the multicollinearity issue (Greene, Citation2012); and (iv) these two explanatory variables are not our focus in the study.
Table 2. Pairwise correlations
4.2. Baseline estimation results
Before entering the estimation results of main interest, we present our baseline regressions when not allowing interaction terms. The application of the dynamic GMM estimator is justified by a set of results as follows. First, the coefficients of the lagged dependent variable in all regressions are statistically significant, thereby confirming the choice of dynamic panel models. Second, all of the AR(1) and AR(2) tests denote that there exists first- but no second-order autocorrelation of the residuals. Third, all Hansen test results indicate that the set of instruments used is jointly valid. Fourth, the number of instruments used in all regressions does not exceed the total number of banks, thus avoiding the “too many instruments” problem.
As displayed in , all regression coefficients on loan portfolio diversification variables are negative and statistically significant.Footnote4 The estimation results also lead to economic plausibility. Taking the coefficient in column 1 as an example, we can infer that an increase of one standard deviation in the portfolio diversification variable HHI10 (0.081) may reduce ROA by 0.100 percentage points (–1.243 × 0.081).
Table 3. Baseline regressions results
Hence, our finding across alternative variables supports the notion that loan portfolio diversification is detrimental to bank returns in Vietnam, in line with previous patterns obtained in different markets (Acharya et al., Citation2006; Behr et al., Citation2007; Hayden et al., Citation2007; Lydia et al., Citation2017; Tabak et al., Citation2011). There are some explanations for this finding. According to Denis et al. (Citation1997), specialized banks could benefit from more effective credit granting and monitoring thanks to better experience, expertise, and information resources in some economic sectors. Besides, if banks expand lending to sectors already funded by other banks, they may encounter adverse selection that could potentially reduce loan quality (Jensen, Citation1986). The high level of market competition may stimulate banks to cut lending rates to attract customers, thereby adversely affecting bank interest income. This issue is directly verified via the NIM model specification. Taken together, the next subsections of this paper try to offer some insight into possible reasons for the adverse effects of loan portfolio diversification on bank returns.
4.3. The asymmetric effects by business models
present the estimation results for the moderating role of bank business models determined by the non-interest income share. Consistently, the statistically significant and negative coefficients on the standalone diversification measures once again suggest the adverse impact of loan portfolio diversification on bank returns. For the interaction term of interest, the coefficients are positive and statistically significant at least 5% level in some regressions with the dependent variables ROA and ROE (columns 3 and 6 in ), and at 1% level in all regressions in the function of NIM (). These results reveal that not all banks are equally hit by loan portfolio diversification. The less hampered banks are those that have moved away from traditional banking activities towards a business model that principally relies on non-interest income.
Table 4. Interaction effect of loan portfolio diversification and business models on ROA
Table 5. Interaction effect of loan portfolio diversification and business models on ROE
Table 6. Interaction effect of loan portfolio diversification and business models on NIM
Looking into the magnitude of coefficients, we realize that the conditioning effect of non-interest income is also reasonable in terms of economic significance. For example, considering the results at face value in column 6 of , an increase of one standard deviation in the SE6 diversification measure could lead ROE at banks with less non-interest income (e.g., 25th percentile) to decrease more than that at banks with more non-interest income (e.g., 75th percentile) by approximately 0.464 percentage points ([(27.960–12.335)×0.163 × 0.182]).
As suggested by the theories, the critical benefit of non-interest income expansion is that banks could reduce the problem of informational asymmetry when developing non-traditional relationships with their customers, thereby using the diverse information sources to support lending activities (Boot, Citation2000). This in turn contributes to explaining the mitigating role of business models when banks with more non-interest income diversify their loan portfolios.
4.4. The heterogeneous effects by market power
exhibit the interaction effects of loan portfolio diversification and market power captured by the Lerner index on bank returns. As expected, the regression coefficients of the standalone diversification variables confirm the harmful impact of loan portfolio diversification on bank profits. Turning to our main interest, the regression coefficients of the interaction term are significantly positive in most regressions with the dependent variables ROA, ROE, and NIM. Hence, these results imply that increased bank market power tends to mitigate the harmful effects of loan portfolio diversification on bank performance.
Table 7. Interaction effect of loan portfolio diversification and market power on ROA
Table 8. Interaction effect of loan portfolio diversification and market power on ROE
Table 9. Interaction effect of loan portfolio diversification and market power on NIM
Besides statistical significance, our estimation results also indicate that economic importance is substantial. Based on column 2 of , for example, we could speculate that a one standard deviation increase in the HHI8 diversification measure could cause banks with lower market power (e.g., 10th percentile) decrease their ROA by 0.203 percentage points ([(0.182–0.056)×20.655 × 0.078]) more than banks with greater market power (e.g., 50th percentile).
Some mechanisms could explain the weakening effect of market power. First, market power may go along with market discipline, which limits the excessive risk-taking strategies of banks as they expand their loan portfolios (Hellmann et al., Citation2000; Keeley, Citation1990). Second, banks with greater market power are not required to compete intensely with other banks in the system by lowering lending rates or loosening loan-granting standards (Dell’Ariccia et al., Citation2012), while banks having less market power have to act in contrary routes. Third, more powerful banks could secure sufficient resources to better exploit the economies of scope and operate more efficiently (Goldberg & Anoop, Citation1996; Maudos & De Guevara, Citation2007).
In addition to the main findings, the estimation results of some control variables are also consistent and significant. Bank capital induces a negative impact on bank profits. This result is in accordance with the previous finding of Altunbas et al. (Citation2007) that European banks were less efficient after holding more equity. More capitalized banks may take excessive risks to maximize the values for shareholders who invested more in their banks. Another finding in line with our expectation and other prior studies (Athanasoglou et al., Citation2008; Dietrich & Wanzenried, Citation2014) is that bank returns tend to improve during the economic upturn since the demand for financial services increases amid this period. Besides, our estimates indicate that inflation exerts a negative impact on bank returns, supporting the previous argument of Pervana et al. (Citation2015). An environment with high inflation forces banks to raise nominal interest rates to maintain real interests, which in turn increases borrowers’ funding cost and reduces their ability to repay loans to lending banks. Interestingly, the coefficients on the standalone variables of bank business models and market power are significantly negative in most of our regressions. These factors induce favorable indirect effects (through their interaction with loan portfolio diversification) but harmful direct effects on bank returns. So, the results support the view that Vietnamese banks gain no absolute benefits with a higher share of non-interest income and greater market power.
Non-interest income factors and market power, as standalone control variables, show adverse effects on bank profits. Thus, these factors have indirect effects (through diversification of loan portfolios) that potentially cultivate bank outcomes, while their direct effects harm bank profits. Overall, we argue that banks have no absolute benefits with a greater proportion of non-interest income or greater market power. There may be some dark sides that are detrimental to bank performance, such as high switching costs and diseconomies of scope regarding non-interest activities (Boot & Ratnovski, Citation2016; Deyoung & Roland, Citation2001), and the partial presence of the “competition-stability” hypothesis concerning market power (Boyd & De Nicoló, Citation2005; Lucchetta & De Nicoló, Citation2011).
5. Concluding remarks
This study examines how the effects of loan portfolio diversification on bank returns differ according to bank business models and market power. Using a sample of Vietnamese commercial banks in the 2008–2019 period and the two-step system GMM estimator in dynamic panel models, we exhibit evidence that sectoral loan portfolio diversification reduces bank returns. Going a further step than previous authors, we find that not all banks are equally affected by loan portfolio diversification. Concretely, the less hampered banks are those that have moved away from traditional lending activities towards a business model that produces more non-interest income. Similarly, market power may mitigate this detrimental impact of loan portfolio diversification on bank returns. These routes point to the heterogeneity across banks in terms of information resources and competitiveness, which could speak to the causal effects of loan portfolio diversification.
Our findings are robust to alternative measures. For the dependent variables, we employ a rich set of accounting indicators to capture bank returns (ROA, ROE, and NIM), which could provide a rigorous analysis as well. Regarding the explanatory variables of main interest, we design six loan portfolio diversification measures based on the HHI and SE indexes. The modification of sectoral exposure profiles while computing portfolio diversification measures is essential but overlooked in the related literature.
The paper suggests some insightful implications. Regulators should take the unfavorable effects of portfolio diversification into account when encouraging banks to pursue a diversified strategy, while banks themselves need to be extremely careful with the disadvantage of diversification across multiple economic sectors. Further importantly, these implications must be accompanied by the crucial caveat that banks with higher shares of non-interest income and greater market power could mitigate the cost of loan portfolio diversification. Regulators should establish a policy framework distinguishing regulations for different bank groups, and banks should pay close attention to improve their non-traditional activities and market power. From a research perspective, studies investigating the importance of loan portfolio diversification should allow for the heterogeneous effects across different banks to better reflect the economic significance of the portfolio diversification/bank returns nexus.
Acknowledgements
The paper is part of the doctoral thesis by Japan Huynh at the Banking University of Ho Chi Minh City, under the supervision of Associate Professor Van Dan Dang.
Additional information
Funding
Notes on contributors
Japan Huynh
Japan Huynh is a PhD student at the Banking University of Ho Chi Minh City, Vietnam. His research interests cover issues related to banking and finance.
Van Dan Dang is an Associate Professor at the Department of Finance, Banking University of Ho Chi Minh City, Vietnam. He received his PhD degree in banking and finance from Banking University of Ho Chi Minh City. His research interest could be found in subjects of banking, fiscal policy, and monetary policy.
Notes
1. These four state-owned banks are now known as the “big four” banks in Vietnam.
2. We also consider another proxy for business models, calculated by [1—(non-interest income share2 + net-interest income share2)] (Doan et al., Citation2018; Kamani, Citation2019; Shim, Citation2013). A greater value of this indicator implies that a bank is diversifying its revenue sources more. The empirical results obtained by this alternative variable also produce similar patterns, thus offering more reliable evidence to support our conclusions in the paper. We do not report these estimation results to conserve space.
3. We do not present the VIF results for the sake of brevity. We thank an anonymous reviewer for pointing this out.
4. This study performed regressions with three dependent variables (ROA, ROE, and NIM) and six alternative loan portfolio diversification measures (based on the HHI and SE indicators). All regressions yield consistent results. For the sake of brevity, we only report the results as given in .
References
- Abedifar, P., Molyneux, P., & Tarazi, A. (2018). Non-interest income and bank lending. Journal of Banking and Finance, 87, 411–24. https://doi.org/10.1016/j.jbankfin.2017.11.003
- Acharya, V. V., Hasan, I., & Saunders, A. (2006). Should banks be diversified? Evidence from individual bank loan portfolios. Journal of Business, 79(3), 1355–1412. https://doi.org/10.1086/500679
- Allen, F., & Gale, D. (2004). Competition and financial stability. Journal of Money, Credit, and Banking, 36(3b), 453–480. https://doi.org/10.1353/mcb.2004.0038
- Altunbas, Y., Carbo, S., Gardener, E. P. M., & Molyneux, P. (2007). Examining the relationships between capital, risk and efficiency in European banking. European Financial Management, 13(1), 49–70. https://doi.org/10.1111/j.1468-036X.2006.00285.x
- Arellano, M., & Bond, S. (1991). Some tests of specification for panel data: Monte Carlo evidence and an application to employment equations. The Review of Economic Studies, 58(2), 277–297. https://doi.org/10.2307/2297968
- Arellano, M., & Bover, O. (1995). Another look at the instrumental variable estimation of error-components models. Journal of Econometrics, 68(1), 29–51. https://doi.org/10.1016/0304-4076(94)01642-D
- Athanasoglou, P. P., Brissimis, S. N., & Delis, M. D. (2008). Bank-specific, industry-specific and macroeconomic determinants of bank profitability. Journal of International Financial Markets, Institutions and Money, 18(2), 121–136. https://doi.org/10.1016/j.intfin.2006.07.001
- Baele, L., De Jonghe, O., & Vander Vennet, R. (2007). Does the stock market value bank diversification? Journal of Banking and Finance, 31(7), 1999–2023. https://doi.org/10.1016/j.jbankfin.2006.08.003
- Behr, A., Kamp, A., Memmel, C., & Pfingsten, A. (2007). Diversification and the banks’ risk-return-characteristics – Evidence from loan portfolios of German banks. Bundesbank Series 2, Discussion Paper No. 2007(05), available at SSRN: https://ssrn.com/abstract=2793990
- Berger, A. N., Klapper, L. F., & Turk-Ariss, R. (2009). Bank competition and financial stability. Journal of Financial Services Research, 35(2), 99–118. https://doi.org/10.1007/s10693-008-0050-7
- Bikker, J. A., Shaffer, S., & Spierdijk, L. (2012). Assessing competition with the Panzar-Rosse model: The role of scale, costs, and equilibrium. Review of Economics and Statistics, 94(4), 1025–1044. https://doi.org/10.1162/REST_a_00210
- Blundell, R., & Bond, S. (1998). Initial conditions and moment restrictions in dynamic panel data models. Journal of Econometrics, 87(1), 115–143. https://doi.org/10.1016/S0304-4076(98)00009-8
- Boot, A. W. A. (2000). Relationship banking: What do we now? Journal of Financial Intermediation, 9(1), 7–25. https://doi.org/10.1006/jfin.2000.0282
- Boot, A. W. A., & Ratnovski, L. (2016). Banking and trading. Review of Finance, 20(6), 2219–2246. https://doi.org/10.1093/rof/rfv069
- Boyd, J. H., & De Nicoló, G. (2005). The theory of bank risk taking and competition revisited. The Journal of Finance, 60(3), 1329–1343. https://doi.org/10.1111/j.1540-6261.2005.00763.x
- Carter, D. A., & McNulty, J. E. (2005). Deregulation, technological change, and the business-lending performance of large and small banks. Journal of Banking and Finance, 29(5), 1113–1130. https://doi.org/10.1016/j.jbankfin.2004.05.033
- Chen, Y., Shi, Y., Wei, X., & Zhang, L. (2014). How does credit portfolio diversification affect banks’ return and risk? Evidence from Chinese listed commercial banks. Technological and Economic Development of Economy, 20(2), 332–352. https://doi.org/10.3846/20294913.2014.915246
- Dang, V. D. (2020). Do non-traditional banking activities reduce bank liquidity creation? Evidence from Vietnam. Research in International Business and Finance, 54, 101257. https://doi.org/10.1016/j.ribaf.2020.101257
- Dang, V. D., & Huynh, J. (2020). Holdings of sovereign bonds by commercial banks in Vietnam. Cogent Economics & Finance, 8(1), 1818409. https://doi.org/10.1080/23322039.2020.1818409
- Dell’Ariccia, G., Igan, D., & Laeven, L. (2012). Credit booms and lending standards: Evidence from the subprime mortgage market. Journal of Money, Credit and Banking, 44(2–4), 367–384. https://doi.org/10.1111/j.1538-4616.2011.00491.x
- Demirgüç-Kunt, A., & Huizinga, H. (2010). Bank activity and funding strategies: The impact on risk and returns. Journal of Financial Economics, 98(3), 626–650. https://doi.org/10.1016/j.jfineco.2010.06.004
- Denis, D. J., Denis, D. K., & Sarin, A. (1997). Agency problems, equity ownership, and corporate diversification. The Journal of Finance, 52(1), 135. https://doi.org/10.1111/j.1540-6261.1997.tb03811.x
- Dermine, J. (1986). Deposit rates, credit rates, and bank capital: The Klein-Monti model revisited. Journal of Banking and Finance, 10(1), 99–114. https://doi.org/10.1016/0378-4266(86)90022-1
- Deyoung, R., & Roland, K. P. (2001). Product mix and earnings volatility at commercial banks: Evidence from a degree of total leverage model. Journal of Financial Intermediation, 10(1), 54–84. https://doi.org/10.1006/jfin.2000.0305
- DeYoung, R., & Torna, G. (2013). Nontraditional banking activities and bank failures during the financial crisis. Journal of Financial Intermediation, 22(3), 397–421. https://doi.org/10.1016/j.jfi.2013.01.001
- Diamond, D. W. (1984). Financial intermediation and delegated monitoring. The Review of Economic Studies, 51(3), 393. https://doi.org/10.2307/2297430
- Dietrich, A., & Wanzenried, G. (2014). The determinants of commercial banking profitability in low-, middle-, and high-income countries. Quarterly Review of Economics and Finance, 54(3), 337–354. https://doi.org/10.1016/j.qref.2014.03.001
- Doan, A. T., Lin, K. L., & Doong, S. C. (2018). What drives bank efficiency? The interaction of bank income diversification and ownership. International Review of Economics and Finance, 55, 203–219. https://doi.org/10.1016/j.iref.2017.07.019
- Franke, G. R. (2010). Multicollinearity. Wiley International Encyclopedia of Marketing, John Wiley & Sons, Ltd. https://doi.org/10.1002/9781444316568.wiem02066
- Fu, X. M., Lin, Y. R., & Molyneux, P. (2014). Bank competition and financial stability in Asia Pacific. Journal of Banking and Finance, 38(1), 64–77. https://doi.org/10.1016/j.jbankfin.2013.09.012
- Goldberg, L. G., & Anoop, R. (1996). The structure-performance relationship for European banking. Journal of Banking and Finance, 20(4), 745–771. https://doi.org/10.1016/0378-4266(95)00021-6
- Greene, W. W. H. (2012). Econometric analysis 7th Ed. Prentice Hall.
- Hayden, E., Porath, D., & Westernhagen, N. V. (2007). Does diversification improve the performance of German banks? Evidence from individual bank loan portfolios. Journal of Financial Services Research, 32(3), 123–140. https://doi.org/10.1007/s10693-007-0017-0
- Hellmann, T. F., Murdock, K. C., & Stiglitz, J. E. (2000). Liberalization, moral hazard in banking, and prudential regulation: Are capital requirements enough? American Economic Review, 90(1), 147–165. https://doi.org/10.1257/aer.90.1.147
- Hidayat, W. Y., Kakinaka, M., & Miyamoto, H. (2012). Bank risk and non-interest income activities in the Indonesian banking industry. Journal of Asian Economics, 23(4), 335–343. https://doi.org/10.1016/j.asieco.2012.03.008
- Ho, A., & Baxter, R. A. (2011). Banking reform in Vietnam. Asia Focus.
- Hughes, J. P., & Mester, L. J. (1998). Bank capitalization and cost: Evidence of scale economies in risk management and signaling. Review of Economics and Statistics, 80(2), 314–325. https://doi.org/10.1162/003465398557401
- Jahn, N., Memmel, C., & Pfingsten, A. (2013). Banks’ concentration versus diversification in the loan portfolio: New evidence from Germany. Deutsche Bundesbank Discussion Papers.
- Jensen, M. (1986). Agency costs of free cash flow, corporate finance, and takeovers. American Economic Review, 76, 323–329. https://www.jstor.org/stable/1818789?seq=1
- Kamani, E. F. (2019). The effect of non-traditional banking activities on systemic risk: Does bank size matter? Finance Research Letters, 30, 297–305. https://doi.org/10.1016/j.frl.2018.10.013
- Keeley, M. C. (1990). Deposit insurance, risk, and market power in banking. American Economic Review, 80(5), 1183–1200. https://doi.org/10.2307/2006769
- Köhler, M. (2014). Does non-interest income make banks more risky? Retail- versus investment-oriented banks. Review of Financial Economics, 23(4), 182–193. https://doi.org/10.1016/j.rfe.2014.08.001
- Lepetit, L., Nys, E., Rous, P., & Tarazi, A. (2008). The expansion of services in European banking: Implications for loan pricing and interest margins. Journal of Banking and Finance, 32(11), 2325–2335. https://doi.org/10.1016/j.jbankfin.2007.09.025
- Li, L., & Zhang, Y. (2013). Are there diversification benefits of increasing noninterest income in the Chinese banking industry? Journal of Empirical Finance, 24, 151–165. https://doi.org/10.1016/j.jempfin.2013.10.004
- Lucchetta, M., & De Nicoló, G. (2011). Bank competition and financial stability: A general equilibrium exposition. IMF Working Papers, https://doi.org/10.5089/9781463927295.001
- Lydia, D. A., Agbloyor, E. K., & Aboagye, A. (2017). The effect of loan portfolio diversification on banks’ risks and return: Evidence from an emerging market. Managerial Finance, 43(11), 1274–1291. https://doi.org/10.1108/MF-10-2016-0292
- Maudos, J., & De Guevara, J. F. (2007). The cost of market power in banking: Social welfare loss vs. cost inefficiency. Journal of Banking and Finance, 31(7), 2103–2125. https://doi.org/10.1016/j.jbankfin.2006.10.028
- Meslier, C., Tacneng, R., & Tarazi, A. (2014). Is bank income diversification beneficial? Evidence from an emerging economy. Journal of International Financial Markets, Institutions and Money, 31(1), 97–126. https://doi.org/10.1016/j.intfin.2014.03.007
- Pervana, M., Pelivan, I., & Arnerić, J. (2015). Profit persistence and determinants of bank profitability in croatia. Economic Research-Ekonomska Istrazivanja, 28(1), 284–298. https://doi.org/10.1080/1331677X.2015.1041778
- Petersen, M. A., & Rajan, R. G. (1995). The effect of credit market competition on lending relationships. Quarterly Journal of Economics, 110(2), 407–443. https://doi.org/10.2307/2118445
- Puri, M., Rocholl, J., & Steffen, S. (2012). On the importance of prior relationships in bank loans to retail customers. SSRN Electronic Journal. https://doi.org/10.2139/ssrn.1572673
- Roodman, D. (2009). How to do xtabond2: An introduction to difference and system GMM in Stata. Stata Journal, 9(1), 86–136. https://doi.org/10.1177/1536867x0900900106
- Rossi, S. P. S., Schwaiger, M. S., & Winkler, G. (2009). How loan portfolio diversification affects risk, efficiency and capitalization: A managerial behavior model for Austrian banks. Journal of Banking and Finance, 33(12), 2218–2226. https://doi.org/10.1016/j.jbankfin.2009.05.022
- Shim, J. (2013). Bank capital buffer and portfolio risk: The influence of business cycle and revenue diversification. Journal of Banking and Finance, 37(3), 761–772. https://doi.org/10.1016/j.jbankfin.2012.10.002
- Shim, J. (2019). Loan portfolio diversification, market structure and bank stability. Journal of Banking and Finance, 104, 103–115. https://doi.org/10.1016/j.jbankfin.2019.04.006
- Tabak, B. M., Fazio, D. M., & Cajueiro, D. O. (2011). The effects of loan portfolio concentration on Brazilian banks’ return and risk. Journal of Banking and Finance, 35(11), 3065–3076. https://doi.org/10.1016/j.jbankfin.2011.04.006
- Tan, Y. (2016). The impacts of risk and competition on bank profitability in China. Journal of International Financial Markets, Institutions and Money, 40, 85–110. https://doi.org/10.1016/j.intfin.2015.09.003
- Thakor, A. V. (2005). Do loan commitments cause overlending? Journal of Money, Credit, and Banking, 37(6), 1067–1099. https://doi.org/10.1353/mcb.2006.0009
- Turk Ariss, R. (2010). On the implications of market power in banking: Evidence from developing countries. Journal of Banking and Finance, 34(4), 765–775. https://doi.org/10.1016/j.jbankfin.2009.09.004
- Windmeijer, F. (2005). A finite sample correction for the variance of linear efficient two-step GMM estimators. Journal of Econometrics, 126(1), 25–51. https://doi.org/10.1016/j.jeconom.2004.02.005