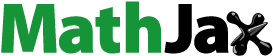
Abstract
This study investigates the patterns of global coffee trade flows and identifies the major determinants of global coffee trade by incorporating RTAs as important variable. Gravity modeling with OLS and PPML estimator was employed for the analysis using panel data on bilateral coffee trade flows of 18 major coffee exporters and 201 trading partners for the period 2001–2015. Both exporter GDP (and population) as well as importer GDP were found to be important determinants enhancing coffee trade. Of the bilateral distance variables, physical distance is found to impede coffee trade, while common border was found to enhance it. On the other hand, cultural (distance) variables like colonial link, common colonizer and common language were also found to enhance coffee trade. Other variables that were found to significantly enhance coffee trade include depreciation in exporting country’s exchange rate, the amount of arable land in exporting country, infrastructure and global financial crisis. On the other hand, importing country tariff was found to significantly reduce coffee trade as expected. Surprisingly, the RTA variable had no significant impact on coffee bilateral trade.
PUBLIC INTEREST STATEMENT
This study investigates the patterns of global coffee trade flows and identifies the major determinants of global coffee trade by incorporating RTAs as an important variable. Result highlights that both exporter GDP (and population) as well as importer GDP were found to be important determinants enhancing coffee trade. Physical distance is found to hamper coffee trade, while common border was found to enhance it. On the other hand, cultural (distance) variables like colonial link, common colonizer and language were also found to enhance coffee trade. Other variables that were found to significantly enhance coffee trade include depreciation in exporting country’s exchange rate, the amount of arable land in exporting country and infrastructure. On the other hand, importing country tariff was found to significantly reduce coffee trade as expected. The RTA variable had no significant impact on coffee bilateral trade.
1. Introduction
Coffee is produced in more than 50 developing countries, providing income for approximately 25 million farmers (Petit Citation2007).This makes coffee production one of the main cash crop sector and a significant source of livelihood, employment and foreign exchange in most of developing countries. There are countries known for coffee production on a global scale. In this respect, countries from Latin America, Asia, and Sub-Saharan Africa constitute the largest producers of green coffee in terms of value and quantity (FAO, Citation2016). Accordingly, Brazil is the main producer of green coffee beans in 2013, followed by Vietnam, Indonesia, Colombia, and India. Importantly, the coffee sector accounts for a significant proportion of foreign earnings in some of the countries listed. For instance, in countries such as Ethiopia, Uganda, and Burundi, the share of coffee exports relative to total export earnings exceeded 10% in 2013, although the importance of coffee for other countries is still lower (FAO, Citation2016; World Bank, Citation2015)
Moreover, coffee is the second most internationally traded commodity in terms of volume next to crude oil and one of the most valuable globally traded agricultural commodities in human history. Spreading out from Ethiopia in the Horn of Africa, it is now produced in over seventy countries worldwide. Over 2.25 billion cups of coffee are consumed in the world every day (Ponte, Citation2002). It is the world’s most widely traded tropical agricultural commodity with an estimated export of US$ 18.4 billion in 2014/15 (Fair Trade Foundation, Citation2016).
Over 90% of coffee production takes place in developing countries, while consumption happens mainly in the industrialized economies. Three countries alone have produced around 55% of the world’s coffee in recent years: Brazil (32%–34%), Vietnam (12%–13%) and Colombia (8%–9%). In 2010 total coffee sector employment was estimated to be about 26 million people in 52 producing countries (International Trade Centre, Citation2011; Maurice & Davis, Citation2011). In total, around 125 million people worldwide depend on coffee for their livelihoods (Fair Trade Foundation, Citation2012).
Coffee exports are not only an important contributor to foreign exchange earnings but also account for a sizable proportion of tax revenues and gross domestic product of many countries. For instance, during the period of 2005–2010, the average share of coffee in total export earnings for eight coffee-producing countries exceeded 10% (International Trade Centre, Citation2011). Given the importance of coffee in global trade and its contribution to national economies for many countries, it would be worthwhile to evaluate the evolution of the sector in global trade through analyzing the determinants of its bilateral trade flows.
Analysis of the determinants of bilateral trade has been the subject of much research in the past. Many studies that analyze determinants of trade flows commonly employ the gravity model. Since the seminal works by Tinbergen (Citation1962) and Pöyhonen (Citation1963), the gravity model has been a widely used approach to explain bilateral trade. The model postulates that trade is boosted by the economic size of the trading partners and negatively influenced by the geographical distance between them. Other observable characteristics of each pair of countries such as having a common language or sharing a common border that affect bilateral trade flows can be augmented in the gravity model (Agostinoet al., Citation2007; Disdier & Head, Citation2008; Leamer & Levinsohn, Citation1995). More importantly, gravity equations have been extensively employed in the analysis of the impact of trade policies, including evaluation of the impact of FTAs on trade (Abrams, Citation1980; Aitken, Citation1973; Baier & Bergstrand, Citation2007; Bergstrand, Citation1985; Brada & Mendez, Citation1985; Frankel, Citation1997; Frankel et al., Citation1995).
While much of the available literature focused on total or aggregate trade flows, another strand of research has attempted to explore trade at a disaggregated level. The latter mainly focused on analyzing trade flows at a more disaggregated level like manufacturing trade, trade in parts and components, and agricultural trade by applying a gravity model. In particular, there are quite a number of studies that have assessed agricultural trade. However, most of these studies also focused on the total agricultural trade (exports or imports). Only few studies have attempted to evaluate trade at product levels (Agostino et al., Citation2007; Aiello & Cardamone, Citation2010; Nguyen & Arcand, Citation2009). Often, research that uses the gravity model use aggregate trade data between countries. However, Anderson and Wincoop (Citation2004) and Nguyen (Citation2020) state that analyses with disaggregated trade data are also plausible and necessary, since there are great sector variations in terms of trade flows and costs.
The use of aggregate trade data masks several key issues. In particular, the responsiveness of aggregate exports to changes in explanatory variables may not explain the behavior of exports at a more disaggregated level for instance, coffee trade flow. Sectoral or more disaggregated trade in goods may matter for many reasons. First, it may matter due to growth if some sectors are growth drivers. Secondly, factors constraining growth may be more easily identified at the sectoral level. Third, many policies are framed for specific products that are not identified among the relatively aggregated sectors. Finally, most trade policy negotiations are conducted at highly disaggregated “tariff lines”.Footnote1 The existing literature mainly focusing on export trade pattern at aggregate level which provide us misleading conclusion for the countries specializing in commodity-specific exporting item, for instance, coffee sector. Such a research gap in the current literature should be filled in order to allow effective trade policy design and implementation in the coffee sector. Our study is based on a set of panel data and evidence is from 18 coffee exporting and 201 importing countries that are at different levels of development state and we believe our findings will have broad acceptability.
The purpose of this paper is, therefore, to examine the patterns of bilateral trade at product level for coffee. Accordingly, the paper assesses the determinants of global coffee trade flows among major coffee exporting countries and their coffee importing partners. The knowledge of the world coffee trade pattern allows both importing and exporting countries, to decide on trade and market strategies that may increase the gains from trade. In particular, the paper aims at exploring important factors that affect coffee exports. The paper tries to present new evidence regarding the evolution of global coffee trade between 2001 and 2015 for 18 major coffee exporting countries.
Moreover, the current paper seeks to contribute to the literature by providing new evidence on the trends and determinants of global coffee trade for major coffee exporting countries in the world. As such, the paper builds on the previous studies mentioned above so as to provide comprehensive evidence regarding the recent global coffee trade pattern. In particular, the paper extends the work of De Almeida et al. (Citation2012) by considering a more representative sample with a broader regional coverage which is absent so far. In doing so, the study makes use of bilateral coffee trade data for the period of 2001–2015 for 18 major coffee producing and exporting nations that represent over 90% of world coffee exports in recent years. Moreover, the paper also tries to address problems and biases arising from all sources of endogeneity (selection, simultaneity and measurement error) as well as those arising from aggregation of trade flows. Therefore, the key contribution of this study is twofold. First, the study provides valuable empirical contributions to the existing literature regarding disaggregated product level by analyzing what factors could improve and impede the coffee trade pattern in the world. Second, results from the study are expected to provide empirical support for policy design and implementation in the coffee sector that aims to increase trade performance through the creation of different trade integration.
The article proceeds as follows. The next section provides a brief review of existing literature. This is followed by an outline of empirical specification of the gravity model and estimation strategy. Issues pertaining to data are also discussed in this section. Next, the estimation results are discussed. The final section concludes the paper.
2. Literature review
There are a number of empirical studies that evaluate bilateral trade flows between countries. These studies indicate that empirical analysis of bilateral trade flows can be appropriately carried out using the gravity model.Footnote2 As Bayoumi and Eichengreen (Citation1997) noted, the gravity equation has long been a workhorse for empirical studies of the pattern of trade. In particular, the gravity equation has been widely employed in international trade to study the ex post effects of FTAs and customs unions on bilateral merchandise trade flows. The gravity equation is typically used to explain cross-sectional variation in country pairs’ trade flows in terms of the countries’ incomes, bilateral distance, and dummy variables for common languages, common borders, and the presence of an FTA (Baier & Bergstrand, Citation2007).
Tinbergen (Citation1962) was the first to use the gravity equation to evaluate the effect of FTA on bilateral trade flows. His results suggested an economically insignificant “average treatment effects” of FTAs on trade flows. Since then, various studies have come up with quite mixed results. Aitken (Citation1973), Abrams (Citation1980), and Brada and Mendez (Citation1985) found the European Community (EC) to have significant effect on trade flows among member countries, whereas Bergstrand (Citation1985) and Frankel et al. (Citation1995) found insignificant effects. Frankel (Citation1997) found positive significant effects from MERCOSUR, insignificant effects from the Andean Pact, and significant negative effects from membership in the EC. Frankel (Citation1997) and Oguledo and MacPhee (Citation1994) also provide an extensive summary of the coefficient estimates of FTA from various other studies.
A major concern that seems to be not adequately addressed by majority of the earlier studies relates to the issue of endogeneity, which mainly stems from the assumption that the FTA is exogenous. According to Baier and Bergstrand (Citation2007), the FTA dummies are not exogenous random variables. In fact countries are likely to self-select into FTAs due to unobservable reasons which are possibly correlated with the level of trade.
In addition to endogeneity problem, the issue of what is termed as “multilateral resistance terms” also warrants attention. According to Anderson and Wincoop (Citation2004), bilateral trade is determined by relative trade costs, not simply by absolute trade costs. They have rigorously shown that controlling for the relative trade costs is crucial for a well-specified gravity model. To explain this, consider the impact on trade between countries i and j of a change in trade costs between countries i and k, say, as in the case where countries i and k enter into a preferential trade agreement that lowers tariffs on their respective goods. Such a change may well impact the trade of country j, even though it is not a party to the agreement. Such heterogeneity is often unobservable and hence results in biased estimates for the RTA coefficient in the gravity equation.
Many papers have tried to address such biases in different ways. Some used instrumental variable (IV) techniques (Terfler, Citation1993; Ghosh & Yamarik, Citation2004; Lee & Swagel, Citation1997). However, Baier and Bergstrand (Citation2007) argue that most of the available instrumental variables used in empirical analysis of trade agreements are not that convincing. Using a wide array of feasible instrumental variables, they conclude that IV estimation is not a reliable method for addressing the endogeneity bias of the RTA binary variable in the gravity equation. They argue that the likely problem is that the vast numbers of variables that are correlated cross-sectionally with the probability of having an RTA are also correlated with trade flows, which prevents the elimination of endogeneity bias. Another widely used procedure to address such issues consists of using country fixed effects for importers and exporters (Baldwin & Taglioni, Citation2006; Rose & Van Wincoop, Citation2001).
Other studies have assessed the impact of non-reciprocal preferential trade policies (NRPTPs) on the export performance of developing countries (Oguledo & MacPhee, Citation1994; Goldstein et al., Citation2003; Nouve & Staatz, Citation2003; Lederman & Özden, Citation2004; Subramanian & Wei, Citation2005; Verdeja, 2006; Agostinoet al., Citation2007). Agostino et al. (Citation2007) note that the gravitational approach offers a framework for assessing whether NRPTPs affect bilateral trade flows between beneficiaries and donors. The gravity model posits that the normal level of trade is positively affected by the economic mass of the trading countries (richer and larger nations both export and import more) and negatively influenced by the geographical distance between them.
These authors, however, raise two major problems that are associated with the previous studies. The first relates to the focus on total trade (exports) from the beneficiary to the preference-giving countries. Secondly, the mentioned studies model NRPTPs by augmenting the gravity model with a preference dummy. Agostino et al. (Citation2007) argue that such methodological choices are misleading. The reason for this stems from the belief that the objective of NRPTRs is not to affect total trade of the beneficiaries, but rather to alter the incentives for developing countries to export more in specific sectors in which preferences are granted. Accordingly, they hypothesize that when overall exports are considered, the impact of NRPTPs might be underestimated, especially if the export shares of the sectors that account for a higher margin of preference are small.
Generally, empirical evidence pertaining to trade at sectoral level has been relatively thin as compared to that at aggregate level, though there has been a growing interest in the area in recent years. There are quite a few studies that have assessed trade in agricultural products. Some of these focused on total/aggregate agricultural trade flows (Hatab et al., Citation2010; Kim et al., Citation2004), while others dealt with a more disaggregated level (commodity/product-level) of analysis (Gebrelibanos, Citation2005; Getachew, Citation2009; Jayasinghe & Sarker, Citation2008; Jordaan & Eita, Citation2007; Nguyen & Arcand, Citation2009). However, coffee as an important agricultural commodity has not been extensively analyzed in the framework of gravity modeling. To the knowledge of the authors, only three studies (Agostino et al. Citation2007; Aiello & Cardamone, Citation2010; De Almeida et al., Citation2012) have attempted to analyze bilateral coffee trade flows using gravity equation.
In particular, De Almeida et al. (Citation2012) attempted to assess the impact of non-tariff measures (Technical Barriers to Trade—TBT and Sanitary and Phytosanitary—SPS notifications) on international coffee trade using data for the period of 1996–2010. The study is particularly very relevant as it is the only one that has attempted to directly address the research issue which the current study is seeking to address. However, there are a couple of limitations of the study. Firstly, with only five countries considered, the country coverage appears to be not sufficient to provide the complete picture of the global coffee trade. Moreover, the fact that three of the five sample countries considered were from Latin America may fall short of capturing the regional variability in coffee exports and hence render the sample to be non-representative. Furthermore, for none of these countries was coffee a major export earner for the period under the study.
The remaining two studies (Agostinoet al., Citation2007; Aiello & Cardamone, Citation2010) analyzed agricultural trade for different levels of aggregation with the aim of assessing the impact of preferential trading agreements on LDC exports. The papers have employed gravity model estimates for different product categories and different levels of aggregation including coffee. However, the main goal of both studies was not to analyze global coffee trade patterns. In particular, Agostino et al. (Citation2007) were mainly concerned with the assessment of the effect of non-reciprocal trade preferences with emphasis on finding out whether or not aggregation matters.Footnote3 On the other hand, Aiello and Cardamone (Citation2010) mainly focused on the assessment of the effectiveness of the Everything but Arms (EBA) initiative launched by the EU in 2001.
The study conducted by Nsabimana and TafesseTirkaso (Citation2019) examines the impact and implications of the East African Community and the Common Market for Eastern and Southern Africa preferential trade agreements on coffee export performance of eight East and Southern African countries by employing a static and dynamic gravity modeling framework for the period 1998–2013. They found that regional trade agreements play a vital role in increasing coffee trading in East and Southern African countries. Factors including geographical distances, income, and population size in importing and exporting countries are also statistically significant determinants of coffee exports. The study also found that the exporting countries are currently under-performing with respect to their maximum potential in the global market indicating room for improvement.
Ekanayake et al. (Citation2010) analyzed the trade creation and trade diversion effects of the regional trade agreements (RTAs) in Asia and their effects on intra-regional trade flows using annual trade data for the period 1980–2009 and find that the coefficients of real GDP, population, and distance had significantly affected export trade flow in Asian countries. More to product-level analysis, the work by Nsabimana and TafesseTirkaso (Citation2019) examines the impact and implications of the East African Community and the Common Market for Eastern and Southern Africa preferential trade agreements on coffee export performance of eight East and Southern African countries by employing a static and dynamic gravity modeling framework for the period 1998–2013 and they found that factors including geographical distances, income, and population size in importing and exporting countries are also statistically significant determinants of coffee exports. Further, they also found that the exporting countries are currently under-performing with respect to their maximum potential to the global market indicating groom for improvement.
3. Methodology
3.1. Empirical framework: the gravity model
As pointed out by Nsabimana and TafesseTirkaso (Citation2019), the traditional gravity modeling approach associates trade flow to different economic indicators and distances such as the national income of trading countries and the physical distances between the trading countries. Meanwhile, recent studies have rigorously expanded the model by incorporating other economically meaningful variables such as potential markets (proxy for population size), trading barriers, country integration, community membership and other sets of dummies representing shared cultural and historical characteristics (see, Melitz 2003; Martínez-Zarzoso 2013; Dal Bianco et al., Citation2015; Saggi et al., Citation2018).
This section provides the econometric specification of the gravity equation. A gravity model states that the trade flows between two countries (exports or imports) can be explained by three kinds of variables. The first group of variables describes the potential demand of the importing country (importer GDP, importer population), the second one considers the supply conditions in the exporting countries (exporter GDP, exporter population) and the third group consists of all the factors that may hinder or favor the bilateral trade flow (i.e., distance, common border, language, past colonial ties, religion, and other relevant variables).Footnote4 Accordingly, the model can be specified in its general form as follows (Baier & Bergstrand, Citation2007; Agostino et al., Citation2010; Hatab et al., Citation2010; Dal Bianco et al., Citation2015):
where the subscript i refers to the exporters, j to the importers and t to time. X is the export flow (coffee), Y is the gross domestic product, P is the population instrumented by potential markets and D is the distance between the capital cities. is error term. To control for observable country-pair specific factors affecting bilateral trade, the model includes some dummy variables. In particular, L and B are two binary variables set to unity if the trade partners share a common language or border, respectively. C is a binary variable which is unity if country i was a colony of country j. Finally, Z is a vector of variables that possibly affect bilateral coffee trade flows (like exchange rate and supply-side constraints like infrastructure, governance and other related variables).
For the purpose of estimation, the model in EquationEquation (1)(1)
(1) is expressed in log form as:
Most studies that focus on bilateral trade also seek to assess the impact of Regional Trade Agreements (Baier & Bergstrand, Citation2007; Kepaptsoglou et al., Citation2010; Nsabimana & TafesseTirkaso, Citation2019). This study also does the same by including RTA variable in the gravity specification as a dummy variable set to unity if i and j belong to the same RTA (such as EFTA, NAFTA, COMESA or a bilateral agreement between the trading countries), and zero otherwise. Following the line of argument advanced by Baier and Bergstrand (Citation2007), we examine the impact of RTA on coffee trade while taking care of the endogeneity problem discussed above. After including the RTA dummy variable, the resulting augmented gravity model, therefore, becomes:
However, estimating Equationequation (3)(3)
(3) in its current form would not account for the endogeneity problem of the RTA variable. As discussed in the literature review section, one way to account for endogeneity bias is to use fixed effects (bilateral fixed effects and country-and-time effects (Baier & Bergstrand, Citation2007). The fixed effect estimator is appropriate if the study centers on a particular set of N countries because FE is equally adopted to estimate the panel regression model by accounting for the non-randomness of the cross-section of 18 coffee exporting countries. This procedure will also take care of importers’ and exporters’ multilateral resistance terms (Anderson & Wincoop, Citation2004).
3.2. Estimation strategy
As a starting point, we first estimate Equationequation (3)(3)
(3) using OLS including various fixed effects. In these sets of estimation, we will basically include the standard gravity variables. Here the discrepancy between estimates with no fixed effects included and those with different fixed effects measures the relevance of the unobservable country heterogeneity. Next, we will run another set of OLS regressions by including additional explanatory variables (such as supply side variables, exchange rates, tariffs and non-tariff, etc.). As trade flows with zero values are dropped in OLS estimation, we apply Poisson Pseudo Maximum Likelihood (PPML) technique to estimate similar regressions. It is worthwhile to use the Poisson Pseudo Maximum Likelihood estimator so as to avoid potential bias due to zero trade.
As argued by Nguyen (Citation2020), using FE with the basic gravity model faces some problems. First, FE will lose the information on time-invariant variables like geography distance, common border and language or country colinizer. Besides, the model will delimit the zero trade, leading to selection bias in the case of using FE for export trade flow. Meanwhile, PPML can overcome selection bias created by zero trade and the presence of heteroskedasticity. This method also covers the impact of time-invariant variables that cannot be obtained by FE. Besides, the goodness of fit of PPML (through R2) is higher than FE, leading to higher fitness of the models. Therefore, PPML is more appropriate than FE. In such case, the parameters were obtained from the PPML panel data model, with fixed effects for time, exporters and importers.
3.3. Data and variables
This study covers the period of 2001–2015. The coffee trade statistics (exports) at HS-4Footnote5 digit level (i.e., 0901 for coffee) were extracted from the United Nations COMTRADE database. To collect data from the UN Comtrade database, we primarily use the four-digit harmonized system (HS) code. Specifically, the HS code for “roasted and not roasted” coffee is HS 0901. It should be emphasized the fact that the types of coffee particularly high-quality Arabica and low-quality commodity robusta produced and marketed by the countries that make up the model are different. However, due to the unavailability of specific data on international trade in each of these types of coffees, this paper is restricted to the analysis of whole coffee as a whole.
The set of exporting countries is comprised of 18 major coffee exporting countries from three regions of the world: six from Africa, five from Asia and seven from the Americas.Footnote6 These countries together contributed to nearly 92% of the total world coffee production on average for the period of 2007–2015 () and roughly about the same percentage of the total world coffee exports for the period of 2008/09-2014/15 (). On the other hand, the number of importing countries included is 201, that is, all the countries for which trade statistics are available (the complete list is provided in appendix A). The period under the study covers the years 2001–2015.Footnote7
Table 1. Coffee production by exporting countries, 2007–2015 (000 bags of 60 kg)
Table 2. Coffee exports by exporting countries, 2008/09-2014/15 (000 bags of 60 kg)
Data on GDPs are obtained from the United Nations Statistics Division. Complete information on distance and other time-invariant bilateral gravity variables such as contiguity, adjacency, and so on were obtained from CEPII’sFootnote8 database. The RTA dummy is constructed based on a list of FTAs provided on the WTO website. This dummy includes RTAs notified based on the GATT Article XXIV as well as the Enabling Clause. The list of RTAs considered is provided in appendix B. Other variables used in the study include real exchange rates, tariffs, Non-Tariff Measures (NTMs), and infrastructural indices for both exporting and importing countries. Appendix C provides the complete list of all the explanatory variables used in this study along with their descriptions and data source.
As COMTRADE only reports the positive trade flows declared by each country, the issue of the non-reported data needs to be handled with care. The non-reported data could either be missing (as in the case where countries do not report trade) or zero-trade values (as in the case where countries do not trade). A commonly used procedure to address this is to consider these values as missing. We follow the same.
4. Results and discussion
This section reports and discusses the major empirical results emerging from the analysis. shows the results from the OLS estimation of Equationequation (3)(3)
(3) . Column (1) reports results time or country fixed effects (without fixed effects). Column (2) reports results with time fixed effects meaning time dummies, which are included to account for changing nature of the relationship over time. Column (3) and (4) show results for time and bilateral pair (BP) fixed effects, and bilateral pair and country-by-time fixed effect resepectively (time-invariant importer and exporter fixed effects and for time-varying exporter and importer fixed effects, respectively).
Table 3. The description of the trade gravity model variables
Both exporter and importer GDPs have the expected positive sign and are significant in all specifications except for columns (4), where importer GDP loses significance. In line with the logic behind the gravity model for aggregate trade flows, the higher the level of income (GDP) of exporting countries, the greater the capacity to produce/supply and hence the higher the trade level or exports. This suggests that an average increase in income from trading partner countries could positively affect the volume of coffee export. However, this may not necessarily hold true for disaggregated trade like the coffee trade. De Almeida et al. (Citation2012) found a negative and insignificant estimate for exporter GDP and stated that such findings are possible for product-level (disaggregated) analysis. In contrast, our findings somehow conform to the widely accepted logic of gravity modeling. In this connection, the existing literature stresses the need to consider sector GDPs instead of the overall national GDPs for trade analysis at disaggregated level. In fact, given that coffee is a commodity traded between LDCs and MDCs in such a way that it is predominantly exported from low-income countries to high-income countries; it is even possible that the coefficient of exporter GDP is negative as was actually found in one of the regressions (column 2). One possible explanation as to why such could be a possibility is that as countries develop, they are more likely to expand in non-agricultural sectors and hence tend to import agricultural products like coffee than really producing and exporting them. This is particularly a possible scenario since coffee exports are predominantly in the form of non-processed coffee (green coffee). According to International Trade Centre (Citation2011), on average, soluble coffee exports constituted about 0.2% and roasted coffee about 7.0% of the total coffee exports during 2007/08-2010/11. It is, therefore, quite interesting to consider using sectoral GDP data instead of national GDP data and find out a more appropriate effect of the variables on coffee trade flows.
With regard to population, it was found that the coefficient for exporter population was positive as expected but insignificant in all equations except Equationequation (2)(2)
(2) , while that for importer was found negative and significant in all equations. This could be due to the fact that coffee importing countries are typically high-income low-population developed countries and hence possibly leading to negative relationship between coffee exports and importing country population. It could similarly be argued that the opposite could hold true with regard to exporting country population.
Distance is consistently negative in all equations as expected but only significant in Equationequations (3)(3)
(3) and (4). That is, the greater the distance between trading country pairs, the lower the coffee trade between them, thereby implying that the cost of transportation is an important factor in global coffee trade. This variable is a proxy for transportation costs and time, access to market information, access to markets, and other factors that make it difficult for nations to engage in bilateral coffee trade. Similarly, the variables “common border”, “common colonizer” and common language are found to be consistently positive in all equations in line with expectation and significant in most equations, meaning that these variables play an important for enhancing bilateral coffee trade. Exporter’s exchange rate is positive and significant (columns 1 and 2) as expected. This implies that depreciation in the exporter country’s exchange rate vis-à-vis US$ enhances the competitiveness of its exports and hence increases the exporter country’s exports.
The remaining coefficient RTA estimates were found to be statistically insignificant or defy expectations; namely, as for the RTA, the negative coefficients obtained in all equations are in contradiction with findings in many past studies. However, the fact that they are consistently insignificant in all equations is quite interesting in terms of solving this puzzle. A possible explanation could be found in the fact that the bulk of coffee trade flow is mainly from LDCs (which are mainly the coffee exporters) to MDCs (where coffee consumption mainly happens), while most of the RTAs considered were of an intra-regional nature. Consequently, this might lead to a possible negative relationship provided that the regional (intra-regional) trade creation impact of the relatively large number of intra-regional RTAs considered in the sample far exceeds the amount of coffee trade created by the relatively small number of inter-regional RTAs included in our sample. Previous studies have come up with diverse information on the effect of RTAs on trade expansion. As argued by Baier et al. (Citation2015) and Carrere (Citation2006) find that an ex post assessment of RTAs shows a significant increase in trade flows and the increase is greater for the deeper RTA agreements while Vicard (Citation2009) argues that RTAs are by nature a predetermined occurrence and the signature an agreement has no effect on the existing trend behavior. However, in case of disaggregated product-level, in our case, coffee trade further studies should be conducted for confirmation of the result.
Finally, since the results are not as consistent with theory for the common language variables, we tried to combine the two in one dummy variable (the official language and ethnic language). However, the results didn’t show much difference after running regressions using the new dummy variable.
It is worthy of note that results confirm that the initial impact of the global financial crisis which was originated in the US in 2008, on coffee exports found to be statistically significant and negative. It was argued that, due to crisis, price fluctuations have been aggravated and showed a low-income elasticity of demand for coffee exporting countries. Arguably, the crisis might therefore worsen the position of coffee producers in the global value chain, thus further reducing their coffee export.
Table 4. Estimates of gravity model with different fixed effects Dependent Variable is a log of coffee exports in levels and the estimation method is OLS
Table 5. Determinants of bilateral coffee exports Dependent Variable is a log of coffee exports in levels and the estimation method is OLS
reports estimation results where various supply-side variables are included. The variable “percentage of arable land” was found to be positive and significant as expected. On the other hand, the variable “percentage of paved roads”, which was included as proxy for infrastructure in the exporting country turned out to be significantly negative, unlike what is expected. This finding is perhaps suggestive of the need to consider a more appropriate measure of the quality of infrastructure. The coefficient of applied tariff on primary products imposed by importing countries, which was included to capture tariff measures on coffee trade flows turned out to be negative and significant in line with prior expectation. However, as a follow-up to this analysis, there is a need to use actual tariff data at the most detailed tariff line for coffee and also include data on non-tariff measures (NTMs) to find out the actual effects of these trade barriers imposed by importing countries on coffee trade globally. The results with regards to the remaining variables are quantitatively similar to those discussed earlier.
Finally, reports estimation results using the PPML. Again, while the results are broadly similar to those discussed in above qualitatively, the results were quantitatively quite different. In particular, the size of the coefficients of the PPML estimates for exporter and importer GDPs were far smaller. On the other hand, the PPML coefficient estimates for the distance variable are a bit bigger compared to those of the OLS estimates. Therefore, we omit the results of in the discussion, because the discussion based on the Estimates of determinants of bilateral coffee exports applying MPML are similar to those of the OLS estimations.
Table 6. Estimates of determinants of bilateral coffee exports Dependent Variable is a log of coffee exports in levels and the estimation method is PPMLFootnote9
Moreover, similar to previous discussion, the estimated coefficient of the dummy variable, RTAs, has a negative sign. This variable is expected to capture the degree of trade-diverting effects between members and non-members, compared to the “normal” bilateral coffee trade flows. This result confirms with the study of Ekanayake et al. (Citation2010). Another pessimist argument of RTA effect on developing countries might be justified by the similarity of resource endowment of the partner members and the frequent failure by these countries to implement fully the terms of their regional integration agreement makes it hard for them to increase intra-regional trade. In some cases there has been deliberate undermining of the integration agreements.
Due to unavailability of specific data on international trade in different types of coffees, the determinant of world coffee trade was analyzed based on coffee as a whole without considering trade in Arabic and robusta separately which might be a significant difference of pattern of trade in both coffee differentiation. This would be a shortcoming of the analysis and it calls for further research on issue related with coffee differentiation across the world.
5. Conclusion
This study was conducted with the aim of investigating the patterns of global coffee trade flows and identifying the major determinants of coffee exports. Gravity modeling was employed for the analysis using panel data on bilateral coffee trade flows of 18 major coffee exporters and their trading partners (201 countries) for the period of 2001–2015. Both OLS and PPML estimation techniques were employed.
Overall, the gravity model has explained coffee trade flows very well with R-squared values as high as 0.87 in some cases. The major findings are generally supportive of those found in most previous studies and can be summarized as follows.
The basic gravity model variables like exporter GDP (and population) and importer GDP were found to be important determinants enhancing coffee trade. Of the bilateral distance variables, physical distance is found to impede coffee trade, while common border was found to enhance it. On the other hand, cultural (distance) variables like colonial link, common colonizer and language were also found to enhance coffee trade. Other variables that were found to significantly enhance coffee trade include depreciation in exporting country exchange rate and the amount of arable land of exporting country. On the other hand, importing country tariff and global financial crisis were found to significantly reduce coffee trade as expected. The RTA variable was found to have no significant impact on coffee trade flows.
Therefore, policy makers should consider supply and demand side factors for the coffee sector as an essential path towards coffee sector development. Interestingly, the trade potentials from the study revealed that there are substantial opportunities for the coffee exporting countries to exploit the available markets as the countries are still under-exporting compared to the coffee market needs.
While our study examined additional variables beyond the basic gravity variables, it would be worthwhile to raise a number of issues that could help improve our understanding of the central issue we attempted to address. Some of these issues include: the use of data on sectoral GDP rather than overall national GDP; revision of the RTA variable considering only selected RTAs that are based on agreements on inter-regional provisions of preferential treatments or considering Preferential Trade Agreements (PTAs) from developed nations to developing countries like Everything But Arms (EBA), African Growth and Opportunity Act (AGOA), and so on. Moreover, the use of actual tariff data and also of tariff-equivalent of NTM imposed by importing countries specifically for coffee instead of applied tariff on primary agricultural products might have helped better understand the issue at hand. The analysis could have also benefitted from the use of the coffee trade data flows at HS six digit level including the various forms of coffee exported (like roasted, non-roasted, decaffeinated, etc.) so as to be able to analyze the margins of coffee trade by types of coffee.
Acknowledgements
We would like to thank the journal editor and four anonymous reviewers for their critical comments and suggestions during the review process.
Disclosure statement
No potential conflict of interest was reported by the authors.
Additional information
Funding
Notes on contributors

Jemal Abafita
Tekilu Tadesse is an Assistant Professor of Economics and researcher at Jimma University. His research interests include financial economics, efficiency analysis, impact evaluation; market analysis; macroeconomics, and microeconomics policies analysis. He has published nine papers in pre-reviewed international journals.
Jemal Abafita (Ph.D.) is an Associate Professor at the department of economics, Jimma University. His research interests include poverty, (rural) markets, rural development; natural and environmental economics; policy impact evaluation, and microeconometrics, and health economics. He has published 22 papers in peer-reviewed international journals.
Notes
1. Narayanan and Vashisht (Citation2008b) provides list of Free Trade Agreement (FTA) that has been negotiated between India and Thailand, which involves tariff cut proposals at HS-6 level.
2. Kepaptsoglou et al. (Citation2010), also provides a 10-year review of empirical studies on the recent use of the gravity specification for modeling international trade flows and FTA effects.
3. They make their assessment based on three levels of data aggregation (total exports, total agricultural exports and 2-digit industry code)
4. In general, GDP and population are the most common mass variables (with a few exceptions), while impedance is described by distance and a variety of factors enhancing or discouraging trade (Kepaptsoglou et al., Citation2010).
5. See appendix D for the detailed classification of coffee products traded internationally.
6. Cameroon, Cote d’Ivoire, Ethiopia, Kenya, Uganda, and United Rep. of Tanzania from Africa; India, Indonesia, Malaysia, Thailand, and Viet Nam from Asia and Brazil, Colombia, Costa Rica, Guatemala, Honduras, Mexico, and Peru from the Americas
7. For the analysis the estimation was limited to the period of 2001–2015 due to data limitation on such variables as infrastructure, arable land, and applied tariff rate by importing countries.
8. Centre d’Etudes Prospectivesetd’Informations Internationales (CEPII)
9. cccccc
10. This list may be modified depending on the final data compilation.
11. World Development Indicators
References
- Abrams, R. K. (1980). International trade flows under flexible exchange rates. Economic Review, 65(3), 3–22.
- Agostino, M. R., Aielo, F., & Cardamone, P. (2007), Analyzing the impact of trade preferences in gravity models. Does Aggregation Matter? TRADEAG Working Paper 07/4.
- Aiello, F., & Cardamone, P. (2010), Analyzing the effectiveness of the EBA initiative by using a gravity model. Pue&Piec Working Paper 10/7
- Aitken, N. D. (1973). The effect of the EEC and EFTA on European trade: A temporal cross-section analysis. TheAmerican Economic Review, 5, 881–892.
- Anderson, J. E., & Wincoop, E. (2004). Trade costs. Journal of Economic Literature, 42(3), 691–751. https://doi.org/10.1257/0022051042177649
- Baier, S. L., & Bergstrand, J. H. (2007). Do free trade agreements actually increase members international trade? Journal of International Economics, 71(1), 72–95.
- Baier, S. L., Bergstrand, J. H., & Clance, M. W. (2015). Tech. rept. Heterogeneous economic integration agreement effects. Journal of international Economics.
- Baldwin, R., & Taglioni, D. (2006). Gravity for dummies and dummies for gravity equations.CEPR. In Discussion papers (pp. 5850). National Bureau of Economic Research.
- Bayoumi, T., & Eichengreen, B. (1997). Is regionalism simply a diversion? evidence from the evolution of the EC and EFTA. In T. Ito & A. O. Krueger (Eds.), Regionalism vs. multilateral arrangements. 1-31. The University of Chicago Press.
- Bergstrand, J. H. (1985). The gravity equation in international trade: some microeconomic foundations and empirical evidence. Review of Economics and Statistics, 67(3), 474–481. https://doi.org/10.2307/1925976
- Brada, J. C., & Mendez, J. A. (1985). Economic integration among developed, developing and centrally planned economies: A comparative analysis. Review of Economics and Statistics, 67(4), 549–556. https://doi.org/10.2307/1924798
- Carrere, C. (2006). Revisiting the effects of regional trade agreements on trade flows with proper specification of the gravity model. European Economic Review, 50(2), 223–247. https://doi.org/10.1016/j.euroecorev.2004.06.001
- Dal Bianco, A., Boatto, V. L., Caracciolo, F., & Santeramo, F. G. (2015). Tariffs and non-tariff frictions in the world wine trade. European Review of Agricultural Economics, 43(1), 31–57. https://doi.org/10.1093/erae/jbv008
- de Almeida, F. M., Fernandes, M., Gomes, M., & da Silva, O. M. (2012), Non-tariff measures in international coffee trade. Poster prepared for presentation at the International Association of Agricultural Economists (IAAE) Triennial Conference, Foz do Iguaçu, Brazil, 18-24 August, 2012.
- Disdier, A. C., & Head, K. (2008). The puzzling persistence of the distance effect on bilateral trade. Review of Economics and Statistics, 90(1), 37–48. https://doi.org/10.1162/rest.90.1.37
- Ekanayake, E. M., Mukherjee, A., & Veeramacheneni, B. (2010). Trade blocks and the gravity model: A study of economic integration among Asian developing countries. Journal of Economic Integration, 25(4), 627–643. December 2010. https://doi.org/10.11130/jei.2010.25.4.627
- Fair Trade Foundation. (2016). Fair trade and coffee.
- FAO. 2016. FAOSTAT - Food and Agriculture Organization of the United Nations.http://www.fao.org/faostat/en/#home
- Foundation, F. T. (2012). Fair Trade and Coffee. Retrieved on 25 Aug 2014 from http://www.fairtrade.net/fileadmin/user_upload/content/2009/resources/2012_Fairtrade_and_coffee_Briefing.pdf
- Frankel, J. A. (1997). Regional Trading Blocs. Institute for International Economics.
- Frankel, J. A., Stein, E., & Wei, S. J. (1995). Trading blocs and the Americas: the natural, the unnatural, and the super-natural. Journal of Development Economics, 47(1), 61–95. https://doi.org/10.1016/0304-3878(95)00005-4
- Gebrelibanos, H. M. (2005), “The effect of exchange rate policy on the agricultural export commodities in Ethiopia: The case of oilseeds”, Unpublished Msc Thesis, Addis Ababa University.
- Getachew, Y. (2009), “Determinants of cut-flower export supply of Ethiopia: A panel data evidence”, Unpublished Msc Thesis, Addis Ababa University.
- Ghosh, S., & Yamarik, S. (2004). Are regional trading arrangements trade creating? an application of extreme bounds analysis. Journal of International Economics, 63(2), 369–395. https://doi.org/10.1016/S0022-1996(03)00058-8
- Goldstein, J., Rivers, D., & Tomz, M. (2003), How Does the Trade Regime Affect International Trade? Paper prepared for the annual meetings of the American Political Science Association, Philadelphia, PA, August 28-31, 2003.
- Hatab, A. A., Romstad, E., & Huo, X. (2010). Determinants of Egyptian agricultural exports: a gravity model approach. Modern Economy, 1, 134-143.
- International Trade Centre. (2011). The Coffee Exporter’s Guide (3rd Ed ed.).
- Jayasinghe, S., & Sarker, R. (2008). Effects of regional trade agreements on trade in agrifood products: evidence from gravity modeling using disaggregated data. Applied Economic Perspectives and Policy, 30(1), 61–81.
- Jordaan, A. C., & Eita, J. H. (2007), “Determinants of South Africa‟s exports of leather products”, University of Pretoria, Department of EconomicsWorking Paper No.21.
- Kepaptsoglou, K., Karlaftis, M. G., & Tsamboulas, D. (2010). The gravity model specification for modeling international trade flows and free trade agreement effects: a 10-year review of empirical studies. The Open Economics Journal, 3(1), 1–13. https://doi.org/10.2174/1874919401003010001
- Kim, M., Cho, G. D., & Koo, W. W. (2004). Does the exchange rate matter to agricultural bilateral trade between Canada and the U.S.? Canadian Journal of Agricultural Economics, 52(1), 127–145. https://doi.org/10.1111/j.1744-7976.2004.tb00098.x
- Leamer, E., & Levinsohn, J. (1995). International trade theory: the evidence. In G. Grossman & K. Rogoff (Eds.), Handbook of international economics. 1339-1394. Elsevier.
- Lederman, D., & Özden, Ç. (2004), U.S. trade preferences: all are not created equal. Central Bank of ChileWorking Paper No. 280.
- Lee, J. W., & Swagel, P. (1997). Trade barriers and trade flows across countries and industries. Review of Economics and Statistics, 79(3), 372–382. https://doi.org/10.1162/003465300556968
- Maurice, N. E., & Davis, J. (2011), Unraveling the underlying causes of price volatility in world coffee and cocoa commodity markets. UNCTAD Discussion paper. 1.
- Narayanan, B. G., & Vashisht, P. (2008b). Determinants of competitiveness of the Indian Auto Industry (No. 201). Indian Council for Research on International Economic Relations.
- Nguyen, D. D. (2020). Determinants of Vietnam’s rice and coffee exports: Using stochastic frontier gravity model. Journal of Asian Business and Economic Studies, (ahead–of–print). Vol. ahead-of-print No. ahead-of-print. 1-16. https://doi.org/10.1108/JABES-05-2020-0054
- Nguyen, T., & Arcand, J. L. (2009), Gravity equation for different product groups: A study at product level. Working Paper Series No. 18
- Nouve, K., & Staatz, J. (2003), Has AGOA Increased Agricultural Exports from SubSaharan Africa to the United States? Paper presented at the International Conference Agricultural policy reform and the WTO: where are we heading), Capri (Italy), June 23-26.
- Nsabimana, A., & TafesseTirkaso, W. (2019). Examining coffee export performance in Eastern and Southern African countries: Do bilateral trade relations matter? Agrekon. https://doi.org/10.1080/03031853.2019.1631864
- Oguledo, V. I., & MacPhee, C. R. (1994). Gravity Models: A Reformulation and an Application to Discriminatory Trade Arrangements. Applied Economics, 26(2), 107–120. https://doi.org/10.1080/00036849400000066
- Petit, N. (2007). Ethiopia Coffee sector: A Bitter or Better Future, Journal of Agrarian Change,7(2), 225–262.
- Ponte, S. (2002). 'Latte Revolution'? Regulation, Markets and Consumption in Global Coffee Chain. World Development, 38(7), 1099–1122.
- Pöyhonen, P. (1963). A tentative model for the volume of trade between countries. WeltwirtschaftlichesArchiv, 90(1), 93–99.
- Rose, A. K., & Van Wincoop, E. (2001). National money as a barrier to international trade: the real case for currency union. American economic review (pp. 386–390).
- Saggi, K., Stoyanov, A., & Yildiz, H. M. (2018). Do free trade agreements affect tariffs of nonmember countries? A theoretical and empirical investigation. American Economic Journal. Applied Economics, 10(3), 128–170.
- Subramanian, A., & Wei, S. J. (2005), The WTO promotes trade, strongly but unevenly. CEPR Discussion Paper No 5122.
- Terfler, D. (1993). Trade Liberalization and thee Theory of Endogenous Protection: An Econometric study of U.S Import Policy, Journal of Political Economy, 101(1), 138–60.
- Tinbergen, J. (1962). Shaping the world economy: suggestions for and international economic policy. the Twentieth Century Fund.
- Vicard, V. (2009). On trade creation and regional trade agreements: Does depth matter? Review of World Economics, (weltwirtschaftliches archiv). 145(2), 167–187.
- World Bank. 2015. World Development Indicators (WDI) - Data Catalog. https://datacatalog.worldbank.org/dataset/world-development-indicators
APPENDIX A.
List of exporting and importing countriesFootnote10
APPENDIX B.
List of RTAs considered
ASEAN Free Trade Area (AFTA), ASEAN-Australia-New Zealand, ASEAN-CHINA, ASEAN-India, ASEAN-Rep. of Korea, Asia Pacific Trade Agreement (APTA), Canada—Colombia, Canada—Costa Rica, Canada—Peru, Central American Common Market (CACM), Chile—Costa Rica (Chile—Central America), Chile—Guatemala (Chile—Central America), Chile—Honduras (Chile—Central America), Chile—India, Chile—Malaysia, China—Costa Rica, Colombia—Northern Triangle (El Salvador, Guatemala, Honduras), Common Market for Eastern and Southern Africa (COMESA), Costa Rica—Mexico, Costa Rica—Peru, Dominican Republic—Central America, Dominican Republic—Central America—United States Free Trade Agreement (CAFTA-DR), Economic and Monetary Community of Central Africa (CEMAC), Economic Community of West African States (ECOWAS), EFTA—Colombia, EFTA—Mexico, EFTA—Peru, EU—Cameroon, EU—Cote d’Ivoire, EU—Mexico,
India—Afghanistan, India—Japan, India—Malaysia, India—Singapore, Japan-Indonesia,
Japan—Malaysia, Japan—Mexico, Japan—Thailand, Japan—Viet Nam, Lao People’s Dem. Rep.—Thailand, Latin American Integration Association (LAIA), Malaysia—Pakistan, MERCOSUR—India, Mexico—El Salvador (Mexico—Northern Triangle), Mexico—Honduras
(Mexico—Northern Triangle), Mexico—Nicaragua, New Zealand—Malaysia, North American
Free Trade Agreement (NAFTA), Panama—Costa Rica (Panama—Central America), Panama—Guatemala (Panama—Central America), Panama—Honduras (Panama—Central America),
Peru—China, Peru—Singapore, Protocol on Trade Negotiations (PTN), Rep. of Korea—Peru, South Asian Preferential Trade Arrangement (SAPTA), Southern African Development Community (SADC), Thailand—Australia, Thailand—New Zealand, US—Peru, West African Economic and Monetary Union (WAEMU).