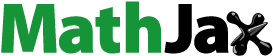
Abstract
Determinants of bid-ask spread have been explored significantly for low-frequency datasets in many developed markets. Researchers have identified share price, traded volume, market–capitalization, return volatility, and number of trades as the prime spread drivers. However, the validity of these determinants has not been explored in high-frequency trading. The present study attempts to articulate the validity of low-frequency determinants of the bid-ask spread in high-frequency trading. It used “bigglm” concept to estimate various determinants of spread, one of the study’s major contributions. The study found a positive relation between market–capitalization and spread, supporting the theory that a higher trading volume cannot decrease the bid-ask spread. Explanatory variables are all significant and show different impacts in different market conditions and sectors. For pooled data, share price, traded volume, quote return, trading frequency, and return volatility are in inverse relation with the spread. The study investigates sectoral determinants of the bid-ask spread to understand sector-specific influences. It also explores the influence of up and down market, settlement cycles, opening and closing intervals, and price and market-cap on determinants. The findings have significance regarding influence on the market microstructure for trading, designing of trading liquidity, and reduction of transaction cost.
PUBLIC INTEREST STATEMENT
Bid-ask spread provides information to traders on liquidity and profit margin in the stock market. The determinants of bid-ask spread have been explored in low-frequency data. However, it has not been examined in high-frequency data in the Indian stock market. The article examines various determinants of bid-ask spread using tick-by-tick data, with a representative sample of 60 stocks involving six dominating sectors over a period of 3 months. The study finds the narrowest bid-ask spread for the IT-Telecom sector compared to a wide bid-ask spread in the case of sectors, such as Services & Healthcare and Automobile & Industrial Manufacturing. Similar to Madhavan (Citation2000), the article finds that market–capitalization, stock price, return volatility, and trading volume are the major determinants of bid-ask spread along with stock quote return and number of trades which are the new determinants under high-frequency trading in the Indian context. The study will be helpful for traders while placing orders in an order-driven stock market.
1. Introduction
Advances in electronic trading together with the ability to process and store fast, large amounts of high-frequency data bring the concept of high-frequency trading (HFT) into the market. HFT is an investment strategy where stocks are bought and sold in a short time by a computer algorithm and are held for a very short time, normally milliseconds (or even microseconds) (O’Hara et al., Citation2019). This is done to profit from taking advantage of extremely short-term changes in the market. Transactions are so fast that many trading organizations place their servers close to the exchange computers to catch trading instructions at the speed of light. HFT possesses the following characteristics: a) it is program-driven trading by computers, b) it has an extremely high trading volume, and c) the rate of return is low, but on the whole, the return is stable. Stocks can be bought and sold multiple times within a trading day. However, understanding HFT is different for market participants, regulators, and academics. At the extremes, any intraday activity where the trading time is measured in microseconds is high-frequency. HFT improves market quality, reduces spreads, increases market depth, and enhances price discovery (O’Hara, Citation2015; Rojcek & Ziegler, Citation2016).
In HFT, traders’ decisions to buy and sell depend on different transaction costs, such as processing fees, exchange fees, and liquidity costs. Processing and exchange fees are regulated by stock exchanges. Bid-ask spread, price impact, and opportunity cost are influenced by traders’ expectations, market forces, and information asymmetry. High-speed and high-volume trading narrow the bid-ask spread in the market. Market microstructure literature (Madhavan, Citation2000) defines the bid-ask spread as the value paid for immediacy. In an order-driven market, traders execute trades by submitting market or limit orders. The bid price is the price an investor is willing to pay for an order, and the ask price is the price an investor is willing to receive from an order. The difference between the bid and the ask prices is known as the bid-ask spread, which is the compensation for immediacy. Spreads are pertinent to high-frequency traders, because a higher spread may generate higher profits. But spread may also occasionally imply greater risk (Ghasemiyeh et al., Citation2017), when traders cannot exit their positions at their desired price.
There are typically three types of bid-ask spread: the quoted spread, the effective spread, and the realized spread (Harris, Citation2003; Su & Tokmakcioglu, Citation2020). The quoted spread represents economic costs in terms of barriers to trade. It is the difference between the best ask price and the best bid price at a specific moment. An effective bid-ask spread is the difference between the actual price at which a dealer buys a security and that at which they subsequently sell it, or vice versa (Harris, Citation2003). An effective spread can measure marketable orders executed in relation to the market center’s quoted spread and considers hidden and midpoint liquidity to be available. In other words, it is the gross underwriting spread, adjusted for impact by a common stock offering’s announcement on a firm’s share price (NASDAQ). A realized spread can be described as the theoretical profits of a liquidity provider. For this study, an effective spread is considered the measured bid-ask spread.
The theoretical literature identifies three main factors determining spread: inventory-holding costs (Amihud & Mendelson, Citation1980; Ho & Stoll, Citation1983), adverse-selection costs (Easley & O’Hara, Citation1992; Glosten & Milgrom, Citation1985), and order processing costs (Brock & Kleidon, Citation1992). Jha et al. (Citation1998) suggest that order processing costs are incurred from orders of different sizes and frequencies for the Indian equity market. The adverse-selection cost increases in the presence of traders with rich information about a security’s value. But the inventory-holding cost is present whenever there is uncertainty about a security’s future payoffs in an equally information-distributed market. Each cost component depends on various factors that call for an analysis of the spread determinants.
Determinants of bid-ask spreads have been extensively studied using low-frequency day-end data. Determinants and their impact on spreads are important for traders, speculators, hedgers, and arbitrageurs, who utilize information from these variables to predict prices (Huang & Stoll, Citation2001). Trading activity, risk, information, and competition are the prime drivers of spreads (McInish & Wood, Citation1992; Schwartz, Citation1988). Researchers have empirically assessed the trading frequency (Copeland & Galai, Citation1983; Kim & Ogden, Citation1996), trading volume (Kim & Ogden, Citation1996; Madhavan, Citation2000; Narayan et al., Citation2014; Stoll, Citation1978), transaction volume (Benston & Hagerman, Citation1974), price (Madhavan, Citation2000; Narayan et al., Citation2014), the number of shares traded (McInish & Wood, Citation1992), and return volatility (Kim & Ogden, Citation1996; Madhavan, Citation2000) as possible determinants of bid-ask spread.
This study initiated an extensive literature review focusing on the determinants of the bid-ask spread in HFT in the Indian market. It focuses on methodologies to empirically examine the determinants of the bid-ask spread in HFT under different market conditions. This study is motivated by the recent movement of HFT in the Indian stock market as well as by previous studies by Stoll (Citation1978), Madhavan (Citation2000), Giouvris and Philippatos (Citation2008), Benston and Hagerman (Citation1974), and Kim and Ogden (Citation1996). The study examines the effects of the various determinants for each sector to understand the sectoral effects. Gkillas et al. (Citation2019) found a day-of-the-week effect in the adjustment speed of spreads. This study analyzes settlement day-wise as well as opening and closing interval-wise analysis to understand the effects of days and times on spread determinants.
The paper is organized as follows. Section 2 presents the literature review on the different aspects of the bid-ask spread and its determinants under low-frequency as well as HFT. Section 3 discusses the objectives and hypotheses of the study. Section 4 explains the methodology used to estimate the bid-ask spread and its determinants. Details on the data selected from the National Stock Exchange and the study time period are provided in section 5. The study findings are given in section 6. Section 7 summarizes the findings of this study and Section 8 presents managerial implications of the findings. Section 9 points out the limitations, and the last section concludes the paper.
2. Literature review
2.1. Liquidity and spread
The bid-ask spread comprises profit and transaction cost; it indirectly measures liquidity or immediacy (Demsetz, Citation1968). An investor may face difficulty in buying or selling a security in the absence of a significant number of trades. Fewer liquid assets, such as small-cap stocks, generally have a higher spread compared to large-cap index stocks. A quoted spread is the generic measure of the bid-ask spread. In both quote- and order-driven markets, quote prices are just the negotiation starting point. But trades may or may not happen at the quote price itself. Frequently, trades occur within or outside the quote prices. So in these scenarios, rather than considering the quoted spread as a measure of the execution cost, an effective spread is considered a useful measure of the execution cost. An effective spread is the difference between the trade and mid-quote price and is potentially a superior measure of the execution cost (Rath, Citation2004). The easy availability of order, quote, and transaction data from different stock markets in different countries has stimulated research on bid-ask spread determinants. There is a significant number of studies on developed countries’ stock exchanges, such as the New York Stock Exchange (NYSE), LSE, NASDAQ, FTSE, Paris Bourse, and Sydney Stock Exchange as well as on stock markets of countries like Saudi Arabia (SSM), Brazil, and India (NSE) (Al-Suhaibani & Kryzanowski, Citation2000; Chakrabarty & Jain, Citation2005; Minardi et al., Citation2006).
2.2. Spread and information
Glosten and Harris (Citation1988) present the asymmetric information model, where the bid-ask spread is broken into transitory and adverse-selection components. Results show the spread is determined by trade size and the adverse-selection component. Menyah and Paudyal (Citation2000) find that along with the order processing cost, the spread is influenced by the inventory cost in a quote-driven market. Giouvris and Philippatos (Citation2008) compare components of the bid-ask spread and their determinants for FTSE100 and FTSE250 stocks. Determinants such as the number of trades have an impact on the bid-ask spread as well as on asymmetric information and order processing cost components. Trading volume has a significant effect on spread and its components for small stocks rather than big stocks. Volatility also has positive effects on all the components. Plerou et al. (Citation2005) show that spread is influenced by order flow and volatility. McInish and Wood (Citation1992) consider trading activity, risk, information, and competition as determinants of spread in NYSE-listed stocks. They find that spread has an inverse relation with trading size and a positive relation with asymmetric information and risk. Kim and Ogden (Citation1996) find a positive relation of spread with negative information, volatility, and trading volume. To examine intertemporal variations in the spread, they perform time-series and cross-sectional tests, finding that the frequency of trades and order size are related to firm size. Kim and Ogden (Citation1996) results also show that although volatility has a positive relation to spread, it also has a relation to firm size, trade frequency, and order size. But past trade frequency has a negative relation to the bid-ask spread. Madhavan (Citation2000) finds that price inverse is an important determinant of the cross-sectional variation in stock spreads. The author’s empirical findings confirm that market–capitalization, price, stock volatility, and trading volume are the prime determinants of spread. The study also provides empirical evidence of the nonlinear effect of volume and price on spread. In the case of the Brazil market, Minardi et al. (Citation2006) find positive relationships between spread, stock price, and liquidity; spreads are negatively related to conventional liquidity measures, such as stock turnover, the volume traded, and the number of trades. Alzahrani (Citation2011) finds volume and volatility to be in positive relation with effective bid-ask spread, while trading frequency is found to be in inverse relation in a study of the Saudi Market, using high-frequency data. The study has achieved overall very low R-squared fitting of the models for different metrics of bid-ask spread. Huang (Citation2004) discusses the determinants of information asymmetry components and order processing costs in his comparative study of the Singapore and Taiwan stock exchanges and confirms a positive relation between the bid-ask spread and price level and return volatility. On the other hand, a rise in the number of transactions and the total volume of trading can lower order processing costs. Based on Demsetz’s (Citation1968) and Hasbrouck’s (Citation2006) studies, Huang (Citation2004) suggests a negative relation between order processing costs and trading activities. Riedl and Serafeim (Citation2011) find that a higher information risk will lead to higher levels of information asymmetry, leading to increasing bid-ask spreads across financial instruments. Fender and Lewrick (Citation2015) conclude that the increased presence of informed traders significantly influences the spread.
2.3. Determinants of spread
Empirical research by Demsetz (Citation1968) is the basis for research in investigating determinants of the bid-ask spread. Demsetz examined the impact of transaction costs on transaction rates (i.e., the number of transactions over a given time period) on NYSE stocks. Benston and Hagerman (Citation1974) estimate the bid-ask spread using trading volume, price volatility, the number of market makers, and the number of transactions as independent variables. They find that spread is positively related to price volatility and is negatively related to competition in market making. Benston and Hagerman (Citation1974) examined the nonlinear relationship between spreads with share price, number of stockholders, number of dealers, unsystematic risk or inventory holding risk, the number of transactions per security, and insider losses. Copeland and Galai (Citation1983) empirically observe relations between the bid-ask spread and variables like price volatility, asset price level, and volume. The volume’s negative effect on spreads is also argued by others. Easley and O’Hara (Citation1992) and Brock and Kleidon (Citation1992) find a positive relationship between spread and volume. Cohen and Maier (Citation1986) find a positive relationship between transaction price volatility and bid-ask spreads when price change is measured over short intervals, such as daily intervals. Similar results have also been reported by Tinic and West (Citation1972). However, Chordia et al. (Citation2001) find a negative effect of volatility on spreads. Wang et al. (Citation1994) find that bid-ask spread and price volatility are jointly determined and positively related. The results indicate that the major determinants of bid-ask spreads are price risk, volume per transaction, and competition in market making. Wang (Citation1999) examines components of the bid-ask spread in the Sydney Futures Exchange and mentions that the bid-ask spread has a higher adverse-information component and a lower order-processing component. Hussain (Citation2011) finds that a contemporaneous and lagged trading volume and the bid-ask spread have a statistically significant effect on return volatility. Amihud and Mendelson (Citation1987) demonstrates that the bid-ask spread has a positive effect on return variance. They also show (Amihud & Mendelson, Citation1986) that asset returns increase with bid-ask spreads. Chen and Kan (Citation1996) also adopt Amihud and Mendelson (Citation1986) model but cannot find any reliable relation between the CAPM risk-adjusted return and the relative bid-ask spread. Demsetz (Citation1968) finds a positive relationship between the share price spread, while McInish and Wood (Citation1992) find a negative relationship. Nath et al. (Citation2017) find a significant relationship between the bid-ask spread and its determinants, such as order imbalance, trade execution, volatility, and the trading volume in the case of the intraday trading of the government securities market. Rahman et al. (Citation2002) find a positive relation between the intraday variations of the bid-ask spread and intraday return volatility. Zhang et al. (Citation2008) find that the bid-ask spread increases with return volatility. Paital and Sharma (Citation2016) investigate the relationship between return volatility, trading volume, and the bid-ask spread in the high-frequency data of the Indian stock market. The authors find a weak relation of volatility with both volume and spread and conclude that the Indian market is informationally inefficient. Ripamonti (Citation2016), in studying the emerging market of Brazil, finds a relation between the asymmetric information measure of spread and variables such as the market-to-book ratio, debt on equity, size, and return. In the Chinese futures commodity market, Liu et al. (Citation2016) find a positive relationship between bid-ask spreads and volatility and a negative relation with trading volume. In the Indian market, Chakrabarty and Jain (Citation2005) examined variables affecting the bid-ask spread of NSE-listed stocks using only day-end data. The results confirmed a negative relationship between spread and trading volume, while volatility is positively related to spread. These authors’ findings could be different, given high-frequency intraday data.
3. Research objectives and hypotheses
The above discussed literature presents an in-depth review of the determinants of bid-ask spread. The literature outlines share price, market–capitalization, the number of trades, the square of return, and trading volume as the prime drivers of bid-ask spread. The majority of the studies were conducted in developed markets with a low-frequency dataset.
Analyzing the determinants of bid-ask spreads in the Indian market using high-frequency datasets is an evolving area of current research. This study will contribute to existing literature by studying determinants of the bid-ask spread under HFT in the Indian stock market for different sectors, market conditions, settlement periods, pools of stocks based on characteristics (volume, share price, and market–capitalization), and different timeframes. The study has framed the following objectives to examine various determinants of the bid-ask spread in HFT:
Using “pooled bigglm, (bounded memory linear and generalized linear models),” the study will examine various determinants of the bid-ask spread.
Sectoral analysis will be conducted to articulate sector-specific influence on bid-ask spread determinants.
The study will conduct settlement day-wise bid-ask spread analysis to understand the impact of settlement days on bid-ask spread determinants.
The first and last ticks of the day carry different information than the other ticks of the day. Hence, the study uses the first and last ticks of the day to analyze bid-ask spread determinants.
The study will separate stocks on the basis of low and high price and low and high market–capitalization.
This study also formulates the following hypotheses to be tested during the study:
HT 1: Stocks with large trading volumes will have narrower bid-ask spreads than those of low trading volume.
HT 2: The bid-ask spread is narrow when volatility is low and risk is at a minimum.
HT 3: For low-priced stocks, the bid-ask spread will tend to be larger.
Using “pooled bigglm,” the study will examine determinants of the bid-ask spread separately for each data set.
4. Methodology
4.1. Estimations of bid-ask spread
Quoted Spread
As per Bessembinder and Venkataraman (Citation2010), a quoted spread is computed using Equationequation 4.1(4.1)
(4.1) :
where is the quoted spread, ait is the best quoted ask price, and bit is the best quoted bid price, where t=1,…,n is the stock being observed, t=1,…,T is the time, Quotedspreadit is the quoted spread at time t, Askpriceit is the ask price at time t, and Bidpriceit is the bid price at time t.
The quoted percentage spread is the measurement of the execution cost, described on a percentage basis. Studies (Benzennou et al., Citation2020; Bessembinder & Venkataraman, Citation2010) propose computing the quoted percentage spread as follows (Equationequation 4.2(4.2)
(4.2) ):
where is the quoted percentage spread, ait is the best quoted ask price, and bit is the best quoted bid price, where i=1,…,n is the stock being observed, is the time, QuotedpercentageSpreadit is the quoted percentage spread at time t, Askpriceit is the ask price at time t, Bidpriceit is the bid price at time t, and Midquoteit is the mid-quote at time t, computed as
.
Effective Spread
The effective spread is twice the absolute value of the difference between the actual trade price and the midpoint of the market quote (i.e., between the quoted bid price and the quoted ask price), divided by the midpoint between these two prices. Using the trade indicator (buy or sell), in Roll (Citation1984) and Huang and Stoll (Citation1997), the effective spread is computed from the difference between the trade price and the quoted midpoint:
where t=1,…,n is the stock being observed, t=1,…,T is the time, Pit is the transaction price, is the midpoint of the posted bid and ask quotes, and Dit is the trade indicator, whose values are “+1” for a buyer-initiated order and “–1” for a seller-initiated order.
is the quote midpoint
, where
is the posted ask price and
is the posted bid price.
Classification of Buy and Sell Transactions
In the high-frequency quote and trade data of the NSE, taken from the Bloomberg server, there are no flags for classifying whether a trade was buyer-initiated or seller-initiated. In studying the market microstructure, this classification is important, as it is supported by different empirical studies. To calculate the effective spread, first, the computation of the trade indicator is needed, to understand the impact on the spread from the buyer/seller initiation of trade. This classifier is the trade indicator. There are two approaches to computing this indicator: 1) the tick test, comparing the trade price to adjacent trades and 2) comparing the trade price to the quote (bid and ask) prices of the prevailing quote. Lee and Ready (Citation1991) take the tick test approach, defining the trade indicator direction of the trade initiation (bid or ask). Value is computed as (+1) for buy-initiated orders and (–1) for sell-initiated. Lee and Ready (Citation1991) propose an algorithm for computing trade direction, based on the tick test. The computation of trade direction is based on several conditions comparing trade prices to preceding trades. Trades can be categorized into four categories: uptick, downtick, zero-uptick, and zero-downtick. If the current trade price is higher (lower) than the previous price, it is called uptick (downtick). If the current price is equivalent to the previous price, it is zero tick. In case of a zero tick in a previous trade, a check is needed for the last price change. If that price change was an uptick (downtick), the current trade is called a zero-uptick (zero-downtick). Lee and Ready (Citation1991) argue that a better result is obtained from a tick test than from other tests. Using a tick test of preceding trades, buy orders are identified:
where Pt is the trade price and Pm is the mid-quote price.
In high-frequency data, one major finding is that of duplicate timestamps in the data. To handle that, this study selected the best bid and ask from the quote data and the mean trade from the trade data for computation from duplicate timestamp ticks.
4.2. Determinants of bid-ask spread
For high-frequency determinants of bid-ask spreads, the study used a modified Madhavan (Citation2000) model to estimate the following regression equation for measuring the tick spread from the big data perspective:
where for security i at time t, is the stock spread and dependent variable.
Regarding the independent variables, Mitis market–capitalization, Pitis price, QRit is the quote return of the stock computed as, NTit is the number of trades, RVit is the volatility of return, and Vitis the trading volume. The quote return is estimated as the absolute value of the return at day t, calculated from the mid-points of the bid-ask quotes. Brown et al. (Citation2010) also tested a similar model, mentioned above, for the bid-ask spread.
Several factors influence the bid-ask spread, the most evident being trading volume. Stocks with large trading volumes will have narrower bid-ask spreads than those that are infrequently traded (Brock & Kleidon, Citation1992; Copeland & Galai, Citation1983; Easley & O’Hara, Citation1992; Kim & Ogden, Citation1996; McInish & Wood, Citation1992). Stock with a low trading volume is considered illiquid, demanding more compensation in the form of a larger spread. Copeland and Galai (Citation1983) and McInish and Wood (Citation1992) found a negative relation between spread and trading volume. Another important aspect affecting bid-ask spread is return volatility. This usually increases during periods of rapid market decline or advancement. During high-return volatility, the bid-ask spread is much wider, because liquidity providers want to book profit, and when volatility is low and uncertainty and risk are at a minimum, the bid-ask spread is narrow. Many empirical studies have documented a positive relation between bid-ask spread and volatility (Cohen & Maier, Citation1986; G. H. Wang et al., Citation1994; Kim & Ogden, Citation1996; Tinic & West, Citation1972; Zhang et al., Citation2008). But Chordia et al. (Citation2001) found a negative relation. While McInish and Wood (Citation1992) found a negative relationship between spread and price, Minardi et al. (Citation2006), in the Brazilian market, found a positive relation. With a higher trading frequency, the bid-ask spread tends to be narrow, as a higher trading frequency means a large trading volume for a stock. Minardi et al. (Citation2006) found a similar result in the Brazilian market. The empirical literature cannot establish conclusive evidence for the impact of market–capitalization, price, and volume on the bid-ask spread.
For analysis of large-scale data, the study has followed the Bigglm package in the regression and classification models. This package was specifically designed by Lumley (Citation2011) to build generalized linear models on big data. This package takes the following steps to reduce the load on memory and process large-scale datasets efficiently:
data is loaded into memory chunk by chunk;
after the last chunk of data is processed, the model statistics must be updated;
after disposition of the last chunk, the next chunk is loaded; and
these steps are repeated until the end of the file.
Any algorithm is classified based on the amount of time or space it requires (Narahari, Citation2002). The growth of time/space requirements can be specified as a function of the input size (p). There are two kinds of algorithm complexity: time complexity, the execution time of the program as a function of the size of the input; and space complexity, the amount of memory required during the execution as a function of input size. Big O notation (O) is a convenient way to describe the complexity of an algorithm or program. Time taken by linear regression is O(np2+p3), which is not so easily reducible. In R, the implantation for the same consumes memory O(np+p2), but building the model matrix using chunks (Miller, Citation1992) can reduce the memory (n is sample size and p is number of variables). Bigglm has generally been used in studies for the computation of big data (Muggeo & Adelfio, Citation2010; Myers et al., Citation2016; C. Wang et al., Citation2016).
5. Data and period of study
Tick-by-tick data for the selected stocks listed on the NSE CNX500 covering the period of June 2016 to August 2016 was collected from the Bloomberg servers and was processed as a large-scale database. The data covered major stocks from dominating sectors, such as Consumer Goods, Financial Services, IT & Telecom Services, Services & Healthcare, Pharmaceuticals, and Automobile & Industrial Manufacturing. Sixty companies were considered for the study, ten from each market sector. The period also consisted of the ups and downs of market conditions of Indian equity markets. The trade and quote data collected had more than 100 million ticks in total. After a cleaning process, 85 million data points remained for processing. This satisfied all seven Vs of big data: volume, velocity, variety, variability, veracity, visualization, and value. The HFT technological infrastructure enables it to compete on speed, time, and superiority, an advantage over low-frequency trading (Seddon & Currie, Citation2017).
Besides using the pooled dataset, this study also includes datasets for different time periods and market conditions for analysis. Sector-wise analysis was done to capture the behavior and information absorption capacity of different sectors. This study also brings settlement-day-wise study of bid-ask spread to capture different trading behaviors during different settlement periods of the month. To capture behavior of small market–capitalization stocks and low price stocks, separate spread determinant analyses were conducted on the subsample dataset.
6. Results and findings
6.1. Summary statistics: effective spread of tick-by-tick data
Effective spread was measured for 60 stocks over 64 trading days for 6 different sectors from 1 June 2016 to 31 August 2016. represents the sector-wise estimates of the mean and standard deviation of the effective spread for the time period. and represents summary statistics and correlation analysis of independent variables.
Table 1. Mean effective bid-ask spread: sector-wise analysis: June–August 2016 Table 1 represents the sector-wise estimates of the mean and standard deviation of the effective bid-ask spread for the time period of 3 months June, July and August 2016. IT—Telecom sector found to have narrowest average monthly effective spread for all the 3 months, where Automobile and Industrial Manufacturing has widest spread. Findings are also similar for standard deviation of spread in the three months
6.2. Determinants of bid-ask spread
Determinants of the bid-ask spread were discussed in the literature review. The study used a modified version of the Madhavan (Citation2000) model to study these determinants, where the effective spread was considered a dependent variable, and share price, traded volume, volatility, market–capitalization, number of trades, and squared return were independent variables. The study analyzed spread determinants across sectors, up and down markets, settlement periods, beginning and closing intervals of the day, high- and low-priced stocks, and market-cap wise stocks.
Explanatory variables of the bid-ask spread were found to be less volatile; there was no significant correlation among them.
6.2.1. Bid-ask spread determinants: pooled datasets
All explanatory variables of the pooled regression of the bid-ask spread (), along with their expected relations, were found to be significant, as per the literature (Benston & Hagerman, Citation1974; Giouvris & Philippatos, Citation2008; Kim & Ogden, Citation1996; Madhavan, Citation2000; Stoll, Citation1978). The pooled regression satisfied the basic requirements of a robustness test. For pooled datasets, we performed an ARCH effect to test heteroskedasticity and also performed an augmented Dicky–Fuller test to examine the stationary property. These tests were conducted for all pooled datasets.
Table 2. Summary statistics: independent variables Table 2 represents summary statistics of explanatory variables used throughout the study. Given the mix of different categories of stocks in the study sample, standard deviation is highest in case of traded volume and subsequently in market capitalization
Table 3. Correlation of independent variables Table 3 represents correlation analysis among explanatory variables. No significantly high correlation (>0.6) was observed. Quoted return generally have inverse correlation with traded volume. In the events of market becoming significantly liquid with larger volumes of buy and sell orders, the quoted return decreases significantly and trading takes place around mid-quote price
Table 4. Bid-Ask spread determinants: pooled data sets method: pooled bigglm; period: June—August 2016; dependent variable: effective spread Table 4 represents results from pooled regression on total sample data. Except Market Cap, all other explanatory variables (including trade price of stock) are in inverse relations with effective bid-ask spread
Table 5. Bid-Ask spread determinants: pooled data sets—sector-wise analysis method: pooled bigglm: sector-wise analysis; period: June—August 2016; dependent variable: effective spread represents Sector Wise determinants for Effective Bid-Ask spread. Our objective is to analyse impact of individual determinants on effective spread at different sector level. One major observation here is that Price and Volatility are in positive relation with effective spread in case of Automobile & Industrial Manufacturing sector which is opposite in case of other sectors. Overall, pharmaceuticals sector has highest explainability power by determinants of effective spread
Table 6. Bid-Ask spread determinants: pooled data sets—settlement days-wise analysis method: pooled bigglm: settlement days-wise analysis; Period: June—August, 2016; dependent variable: effective spread represents Settlement Day wise analysis of determinants of effective spread. We randomly chose three different settlement weeks from 3 months covered under this study to analyse the impact of determinants in various phases of settlement cycles. Variables like quote return and volatility tend to act differently in different settlement periods. This confirms the effect of settlement periods on spread management. For all three experiments, we have seen similar level of explained variation
Table 7. Bid-Ask spread determinants: pooled data sets—opening and closing interval-wise analysis method: pooled bigglm: opening and closing interval-wise analysis; period: June—August, 2016; dependent variable: effective spread Table 7 represents Settlement Day wise analysis of determinants of effective spread. We randomly chose 3 different settlement weeks from 3 months covered under this study to analyse the impact of determinants in various phases of settlement cycles. Variables like quote return and volatility tend to act differently in different settlement periods. This confirms the effect of settlement periods on spread management. For all three experiments, we have seen similar level of explained variation
Table 8. Pooled bigglm: price range-wise analysis period: June—August 2016; dependent variable: effective spread Table 8 represents different price—range (of stocks) wise spread analysis in terms of its determinants. The study found model explainability power to be highest for High Price range stocks and lowest for Low Price range stocks. For high price range stocks, except return volatility, all other variables have significant effect on effective spread
Table 9. Pooled bigglm: market cap-wise analysis period: June—August 2016; dependent variable: effective spread Table 9 represents different market capitalization (of stocks) wise spread analysis in terms of its determinants. The study found model explainability power to be highest for Small cap stocks and lowest for Mid cap stocks. Only price, traded volume and market cap have significant impact on spread in case of small cap stocks. Though in case of large and mid cap stocks, all the variables have significant impact on spread
The negative relation of spread to number of trades and volume implies a lower bid-ask spread for a higher number of trades and volume, consistent with the literature (Benston & Hagerman, Citation1974; Kim & Ogden, Citation1996, in the NYSE; Giouvris & Philippatos, Citation2008, in the LSE). This supports Hypothesis (HT) 1, stating that with large traded volumes, spread tends to be narrower. Similar to Stoll (Citation1978) for NASDAQ, Jegadeesh and Subrahmanyam (Citation1993) for the NYSE, and Heflin and Shaw (Citation2000), the study found an inverse relation between spread and stock price in the Indian market. A negative relation between spread and quote return is consistent with Narayan et al. (Citation2014); however, the negative relationship indicates that the bid-ask spread does not price the risk. This also supports Hypothesis (HT) 3, implying that for lower-price stocks, spread tends to be wider. Return volatility has an inverse relationship to spread, indicating that it reduces the spread. A positive relation between market–capitalization and spread indicates that despite a higher trading volume, spread does not decrease. This is consistent with Kim and Ogden (Citation1996) and Heflin and Shaw (Citation2000).
6.2.2. Bid-ask spread determinants: pooled datasets—sector-wise analysis
Analysis of determinants for bid-ask spread was conducted sector-wise to capture the impact of sector-specific information and behavior on spread. The study conducted pooled regression analysis for Consumer Goods (sector 1), Financial Services (sector 2), IT-Telecom (sector 3), Services & Healthcare (sector 4), Pharmaceuticals (sector 5), and Automobile & Industrial Manufacturing (sector 6), where each sector had 10 identified stocks having a larger market share.
In the sectoral effective spread graph, , differences in spread levels are clearly visible among different sectors.
In sector 2 (Financial Services) and sector 4 (Services & Healthcare), there were more ups and downs in spread during the study period. There was no common trend in spread among different sectors of the economy, and each sector had its own way of movement; these needed to be analyzed separately. This study tried to capture the impact of different sectoral behaviors on effective spread.
The sector-specific pooled regression revealed similar results in a larger perspective; however, price had a direct relation to spread in the Automobile & Industrial Manufacturing sector, unlike other sectors. Similarly, quote return was statistically insignificant in the Consumer Goods sector, and return volatility had a direct relation in the Automobile & Industrial Manufacturing sector, unlike in other sectors. Price as an explanatory variable comparatively had more explanatory power in the Pharmaceuticals sector than in others. Similarly, quote return as an explanatory variable comparatively had less explanatory power in the Services & Healthcare sector than in others. The pooled regression satisfied the basic requirements of a robustness test. Sector-wise analysis captured the behavior and information absorption capacity of different sectors.
6.2.3. Bid-ask spread determinants: pooled datasets—settlement days-wise analysis
Investors square off their positions, and delivery of stocks occurs on settlement days. This regulatory requirement might have some impact on spread. To observe this, the study conducted a settlement day-wise analysis of spread. For June 2016, the last week of settlement days was considered; for July 2016, the mid-month settlement week was considered; and for August 2016, the first week of the settlement period was considered. This covered the full cycle of the settlement period over 3 months to discern different behavioral changes in spread.
Unlike pooled regression, price had a positive relation to spread in the July/August settlement days. Quote return had a negative relation to spread in the beginning of the August settlement days, not found in the mid- and end-settlement days of June/July. Meanwhile, quote return had a higher explanatory power at the beginning of the August settlement days. Return volatility had a significant inverse relation in the beginning settlement days of August compared to other settlement days of June/July. Thus, the study concludes that the settlement period has some impact on the spread. The pooled regression satisfied the basic requirements of a robustness test.
6.2.4. Bid-ask spread determinants: pooled datasets—opening and closing interval-wise analysis
Investors’ buying and selling behaviors are pronounced during the day’s opening and closing. To factor this behavior into the spread, separate pooled regression was conducted for the opening and closing 30 minutes.
During the opening and closing 30-min intervals in different sectors, the market exhibits different trends, as per the graph. While the opening 30 minutes show a smooth trend, though differently for different sectors, the closing 30 minutes exhibit abnormal behavior. To capture the opening and closing interval behaviors of spread, the study separately analyzed them. represents the relationship between spread and various determinants .
Price had a direct relation to spread in the closing interval, opposite from the opening interval. Similarly, the square return had a direct relation to spread in the closing interval but an inverse relation in the opening interval. The pooled regression satisfied the basic requirements of a robustness test.
6.2.5. Price range-wise analysis
High-priced and lower-volume stocks generally have a higher spread, while low-priced and high-volume stocks have a low spread. Usually, low-priced and high-volume stocks are more prone to spread changes. To capture this effect of price on spread, the study conducted analysis of determinants for the bid-ask spread on different price division-wise stocks.
As per the pooled regression, the price range-wise determinant analysis revealed similar results in a larger perspective, except for a difference in the relation of quote return to spread. Quote return had a direct relation to spread for mid- and low-priced stocks but an inverse relation for high-priced stocks. Also, price, traded volume, return volatility, and market-cap as explanatory variables comparatively had more explanatory power for high-priced stocks than for other stock price ranges. The pooled regression satisfied the basic requirements of a robustness test .
6.2.6. Market cap-wise analysis
High market–capitalization can be from either a high volume or high price of a stock. Similarly, low market–capitalization can be from either a low volume or low price of a stock. The study conducted different market–capitalization analyses on spread to capture the behavior of small stocks on spread determinants.
Spread determinant regressions for high, mid and low market–capitalization stocks revealed similar results except in the relations of volatility and number of trades to spread. Though quote return and number of trades had an inverse relation to spread for mid- and small-market-cap stocks, both had a positive direct relation to spread for high-market-cap stocks. The study results for low-priced stocks also support Hypothesis (HT) 3, where for lower-priced stocks, the spread tends to be wider. The pooled regression satisfied the basic requirements of a robustness test.
7. Summary of findings
In this study, bid-ask spread and its determinants have been assessed under different market conditions as well as stock level properties. Past studies (Chakrabarty & Jain, Citation2005; Giouvris & Philippatos, Citation2008; Kim & Ogden, Citation1996; Madhavan, Citation2000) have found a strong relation between spread and determinants like volatility, trading volume, frequency of trades, order size, and stock price. The empirical findings by Madhavan (Citation2000) have confirmed that market–capitalization, price, stock volatility, and trading volume are the prime determinants of spread. This study finds return volatility, share price, trading volume, and number of trades to be significant determinants of spread, as per the prior studies (Benston & Hagerman, Citation1974; Giouvris & Philippatos, Citation2008; Kim & Ogden, Citation1996; Madhavan, Citation2000; Stoll, Citation1978). The negative relations of spread with the number of trades and trading volume imply that bid-ask spread will be lower for higher numbers of trades and volume, consistent with the findings from Benston and Hagerman (Citation1974), Kim and Ogden (Citation1996), and Giouvris and Philippatos (Citation2008). We found an inverse relation between spread and stock price. Similar relations were established by Stoll (Citation1978) in NASDAQ, Jegadeesh and Subrahmanyam (Citation1993) in the NYSE, and Heflin and Shaw (Citation2000). Higher volatility increases the risk, which indicates that bid-ask spread is not pricing the risk. Consistent with findings from Narayan et al. (Citation2014), we found return volatility to have an inverse relationship with spread, indicating thereby that return volatility reduces the spread. As spread has a positive relation to market–capitalization, it indicates that despite higher trading volume, spread does not decrease. This is consistent with findings by Kim and Ogden (Citation1996) and Heflin and Shaw (Citation2000).
The sector-specific analysis reveals similar results in a larger sense; however, price has a direct relation with spread in the Automobile & Industrial Manufacturing sector, unlike in other sectors. Return volatility has a positive relation with spread in the Automobile & Industrial Manufacturing sector, opposite to the other sectors. Price, as an explanatory variable, comparatively has more explanatory power in the Pharmaceuticals sector than in other sectors. Settlement days-wise analysis of spread indicates that the settlement periods have some impact on the spread in terms of different behaviors by determinants. In the price-range wise analysis, the study found that volatility has a positive relation with spread in the case of mid-priced and low-priced stocks, but an inverse relation in the case of high-priced stocks. Stock price, traded volume, quote return and market–capitalization have comparatively more explanatory power in the case of high-priced stocks than other price ranges. Similarly, market–capitalization specific analysis reveals that volatility and number of trades have an inverse relation with spread in the case of mid-cap stocks.
8. Managerial implications
Traders generally manage inventory of cash and stocks with continuous buying and selling of stocks to manage the inventory in a dynamic setup. Buy orders reduce the cash inventory, and sell orders increase the cash inventory. While placing the buy and sell order, traders generally influence their inventories with a motive of increasing the trading profit. In this context, our article provides information to traders on improvement of trading profit through spread management.
The findings reveal that exchanges can manage the bid-ask spread by improving trading volume and trading frequency. The study has found that higher priced stocks have low trading volume and high spread. In this context, exchanges should design a policy for the splitting of high-priced stocks to reduce their spread. The study has made a significant contribution in estimating and analyzing the high-frequency bid-ask spread of selected CNX 500 stocks, across the six sectors, listed on the National Stock Exchange of India (NSE). It discerns the behavior of spread and assesses the factors impacting such behavior on the basis of market-microstructure theories. The results are more pervasive than those of previous studies. The sectoral analysis of spread provides information to traders for the systematic management of buy and sell orders for the IT-Telecom sector where the spread is very narrow, while the Automobile & Industrial Manufacturing sector shows a widened spread. The sector having a widened spread might be at a high risk of illiquidity, and our article provides empirical evidence to traders to manage the trading profit of high-spread stocks. The traders should be very careful while churning the inventory of stocks. To maximize trading profit, the timing of buy and sell is not symmetrical in cases of large-cap stocks, due to a positive relation between quote return and spread. However, in cases of mid-cap stocks, the timing of buy and sell is very important, as quote return and spread are inversely related. Exchanges should also advise companies with high-priced stocks to adopt share splitting to improve trading and to reduce their spread. Exchanges should specifically design monitoring parameters for very low price and small market–capitalization stocks, which are more prone to high spreads and low market liquidity. Since the bid-ask spread indicates the trading and liquidity levels in the market, exchanges should monitor transaction costs and information asymmetry, which have significant impacts on the bid-ask spread.
9. Limitations and future directions
The study has a few limitations with respect to the sample data and modelling. We have not considered the non-linearity aspect of financial time series in the study. The non-linearity aspect of time series data can be factored to understand the evolvement of the bid-ask spread in the stock market. The study was confined to the cash market only and ignored the implications of options and other derivative segments on the bid-ask spread. But, the study can be extended to capture the intraday bid-ask spread and expected returns in the options, futures, and other derivatives segment of the Indian equity market. Apart from that the actual behavior of traders through primary data collection was not captured in this article. This could be another potential area of research in future. A deep dive analysis for selected sectors (Birjandi et al., Citation2019) can bring out more factors like information asymmetry, company conditions, opinions etc., which were beyond scope of this study.
10. Conclusion
The study examined the determinants of the bid-ask spread in HFT for selected sample stocks of the NSE, India. According to the authors’ knowledge, this is the first study that has considered such a deep analysis of the determinants of bid-ask spread in the Indian order-driven market under HFT. This study is unique in terms of analyzing the effects of various market conditions and stock properties on spread and its determinants. The literature has empirically established return volatility, share price, trading volume, number of trades, and quote return to be significant determinants of the bid-ask spread in the NYSE, NASDAQ, and many emerging stock exchanges. The empirical analysis in this study reveals that in the Indian equity market, return volatility, share price, trading volume, and number of trades are significant determinants of spread. This study used the “bigglm” method to model spread determinants, an approach that is unique and that has not been performed in earlier studies. This method takes care of the effects of big data when running regression models.
The findings indicate that the bid-ask spread is not pricing the risk in the Indian market. The positive relation between market–capitalization and spread reveals that higher trading volumes cannot decrease spread. The month-wise analysis on the pooled datasets revealed that explanatory variables have different impacts on spread in different trading time periods; the pricing of information on the spread is not uniform across time periods. The results of sector-wise pooled regression were not similar across sectors, indicating that sector-specific information is captured in the spread determinants. The impact of brisk trading during settlement days reveals that beginning and end settlement cycles have a larger impact on spread. The analysis found that opening and closing intervals both have an impact on spread. The study’s empirical analysis reveals that price and return volatility have direct and inverse relations, respectively, with spread, with respect to closing intervals, while the relations are the opposite for opening intervals. The spreads of mid-priced and low-priced stocks are more prone to return volatility. Price, traded volume, volatility, and market-cap as explanatory variables have comparatively more explanatory power for high-priced stocks than for other stock price ranges. The analysis of spread determinants could help stock exchanges design the market microstructure for trading. The findings on NSE-listed sample stocks reveal that exchanges need to enhance trading volumes, free float shares, and reduce return volatility to reduce spread.
Figure 1. Effective spread among different sectors in the time period of Jun–Aug, 2016

Figure 2. Effective spread among different sectors on 22 July 2016

Figure 3. Effective spread among different sectors on 22 July 2016

Additional information
Funding
Notes on contributors

Aritra Pan
r. Aritra Pan is Assistant Professor and Chairperson at IMT Ghaziabad in the area of Business and Analytics. Dr. Pan has done his Ph.D. from IIT Kharagpur in the area of Financial Markets Analytics. Dr. Pan has completed his M.Tech and B.E. from IIEST, Shibpur. Dr. Pan was previously associated with global corporate firms like PricewaterhouseCoopers (PwC) and RS Software. Dr. Pan has extensively worked in the areas of Data Science, Business Analytics and Business Intelligence.

Arun Kumar Misra
Dr. Arun Kumar Misra is an Associate Professor of Finance at Vinod Gupta School of Management, Indian Institute of Technology (IIT), Kharagpur. Before becoming an academician, he worked as a banker in a leading public sector bank. He has published research articles in the fields of banking, capital markets, and corporate finance.
References
- Al-Suhaibani, M., & Kryzanowski, L. (2000). An exploratory analysis of the order book, and order flow and execution on the Saudi stock market. Journal of Banking & Finance, 24(8), 1323–23. https://doi.org/10.1016/S0378-4266(99)00075-8
- Alzahrani, A. (2011). Liquidity cost determinants in the Saudi market. International Journal of Trade, Economics and Finance, 2(3), 185. https://doi.org/10.7763/IJTEF.2011.V2.101
- Amihud, Y., & Mendelson, H. (1980). Dealership market: Market-making with inventory. Journal of Financial Economics, 8(1), 31–53. https://doi.org/10.1016/0304-405X(80)90020-3
- Amihud, Y., & Mendelson, H. (1986). Asset pricing and the bid-ask spread. Journal of Financial Economics, 17(2), 223–249. https://doi.org/10.1016/0304-405X(86)90065-6
- Amihud, Y., & Mendelson, H. (1987). Trading mechanisms and stock returns: An empirical investigation. The Journal of Finance, 42(3), 533–553. https://doi.org/10.1111/j.1540-6261.1987.tb04567.x
- Benston, G. J., & Hagerman, R. L. (1974). Determinants of bid-asked spreads in the over-the-counter market. Journal of Financial Economics, 1(4), 353–364. https://doi.org/10.1016/0304-405X(74)90014-2
- Benzennou, B., Ap Gwilym, O., & Williams, G. (2020). Commonality in liquidity across options and stock futures markets. Finance Research Letters, 32, 101096. https://doi.org/10.1016/j.frl.2019.01.008
- Bessembinder, H., & Venkataraman, K. (2010). Bid-ask spreads: Measuring trade execution costs in financial markets. In Encyclopedia of Quantitative Finance (pp. 184–190).
- Birjandi, A. K., Akhyani, F., Sheikh, R., & Sana, S. S. (2019). Evaluation and selecting the contractor in bidding with incomplete information using MCGDM method. Soft Computing, 23(20), 10569–10585. https://doi.org/10.1007/s00500-019-04050-y
- Brock, W. A., & Kleidon, A. W. (1992). Periodic market closure and trading volume: A model of intraday bids and asks. Journal of Economic Dynamics & Control, 16(3), 451–489. https://doi.org/10.1016/0165-1889(92)90045-G
- Brown, C., Dark, J., & Davis, K. (2010). Exchange traded contracts for difference: Design, pricing, and effects. Journal of Futures Markets, 30(12), 1108–1149. https://doi.org/10.1002/fut.20475
- Chakrabarty, B. K., & Jain, P. (2005). “Understanding the microstructure of Indian markets”, National Stock Exchange of India, Research Paper Series, NSE, Final Paper No. 128.
- Chen, N. F., & Kan, R. (1996). Center for Research in Security Prices. In K. Saitou, and K. Kubota (Eds.), Expected Return and the Bid-Ask Spread. Graduate School of Business, University of Chicago.
- Chordia, T., Roll, R., & Subrahmanyam, A. (2001). Market liquidity and trading activity. The Journal of Finance, 56(2), 501–530. https://doi.org/10.1111/0022-1082.00335
- Cohen, K. J., & Maier, S. F. (1986). The Microstructure of Securities Markets. Prentice-Hall.
- Copeland, T. E., & Galai, D. (1983). Information effects on the bid-ask spread. The Journal of Finance, 38(5), 457–1469. https://doi.org/10.1111/j.1540-6261.1983.tb03834.x
- Demsetz, H. (1968). The cost of transacting. Quarterly Journal of Economics, 82(1), 33–53. https://doi.org/10.2307/1882244
- Easley, D., & O’Hara, M. (1992). Time and the process of security price adjustment. The Journal of Finance, 47(2), 577–605. https://doi.org/10.1111/j.1540-6261.1992.tb04402.x
- Fender, I., & Lewrick, U. (2015). “Shifting tides–market liquidity and market-making in fixed income instruments”, BIS Quarterly Review, March.
- Ghasemiyeh, R., Moghdani, R., & Sana, S. S. (2017). A hybrid artificial neural network with metaheuristic algorithms for predicting stock price. Cybernetics and Systems, 48(4), 365–392. https://doi.org/10.1080/01969722.2017.1285162
- Giouvris, E., & Philippatos, G. (2008). Determinants of the components of the bid-ask spreads on the London stock exchange: The case of changes in trading regimes. Journal of Money, Investment and Banking, 1(1), 49–61.
- Gkillas, K., Vortelinos, D. I., Babalos, V., & Wohar, M. E. (2019). “Day-of-the-week effect and spread determinants: Some international evidence from equity markets”, available at SSRN 3434805.
- Glosten, L. R., & Harris, L. E. (1988). Estimating the components of the bid/ask spread. Journal of Financial Economics, 21(1), 23–142. https://doi.org/10.1016/0304-405X(88)90034-7
- Glosten, L. R., & Milgrom, P. R. (1985). Bid, ask and transaction prices in a specialist market with heterogeneously informed traders. Journal of Financial Economics, 14(1), 71–100. https://doi.org/10.1016/0304-405X(85)90044-3
- Harris, L. (2003). Trading and exchanges: Market microstructure for practitioners. OUP USA.
- Hasbrouck, J. (2006). Empirical Market Microstructure: The Institutions, Economics, and Econometrics of Securities Trading. Oxford University Press.
- Heflin, F., & Shaw, K. W. (2000). Blockholder ownership and market liquidity. Journal of Financial and Quantitative Analysis, 35(4), 621–633. https://doi.org/10.2307/2676258
- Ho, T. S., & Stoll, H. R. (1983). The dynamics of dealer markets under competition. The Journal of Finance, 38(4), 1053–1074. https://doi.org/10.1111/j.1540-6261.1983.tb02282.x
- Huang, R. D., & Stoll, H. R. (1997). The components of the bid-ask spread: A general approach. The Review of Financial Studies, 10(4), 995–1034. https://doi.org/10.1093/rfs/10.4.995
- Huang, R. D., & Stoll, H. R. (2001). Tick size, bid-ask spreads, and market structure. Journal of Financial and Quantitative Analysis, 36(4), 503–522. https://doi.org/10.2307/2676222
- Huang, Y. C. (2004). The components of bid-ask spread and their determinants: TAIFEX versus SGX-DT. Journal of Futures Markets, 24(9), 835–860. https://doi.org/10.1002/fut.20113
- Hussain, S. M. (2011). The intraday behaviour of bid-ask spreads, trading volume and return volatility: Evidence from DAX30. International Journal of Economics and Finance, 3(1), 23. https://doi.org/10.5539/ijef.v3n1p23
- Jegadeesh, N., & Subrahmanyam, A. (1993). Liquidity effects of the introduction of the S&P 500 index futures contract on the underlying stocks. Journal of Business, 66(2), 171–187. https://doi.org/10.1086/296600
- Jha, R., Murthy, K. V., Nagarajan, H. K., & Seth, A. K. (1998). “Components of the wholesale bid-ask spread and the structure of grain markets: The case of rice in India”, IIM Bangalore Research Paper, (106).
- Kim, S.-H., & Ogden, J. P. (1996). Determinants of the components of bid-ask spreads on stocks. European Financial Management, 2(1), 127–145. https://doi.org/10.1111/j.1468-036X.1996.tb00032.x
- Lee, C. M., & Ready, M. J. (1991). Inferring trade direction from intraday data. The Journal of Finance, 46(2), 733–746. https://doi.org/10.1111/j.1540-6261.1991.tb02683.x
- Liu, Q., Hua, R., & An, Y. (2016). Determinants and information content of intraday bid-ask spreads: Evidence from Chinese commodity futures markets. Pacific-Basin Finance Journal, 38, 135–148. https://doi.org/10.1016/j.pacfin.2016.04.002
- Lumley, T. (2011). “Biglm: Bounded memory linear and generalized linear models.” R package version 0.8, available at: http://cran.r-project.org/web/packages/biglm.
- Madhavan, A. (2000). Market microstructure: A survey. Journal of Financial Markets, 3(3), 205–258. https://doi.org/10.1016/S1386-4181(00)00007-0
- McInish, T. H., & Wood, R. A. (1992). An analysis of intraday patterns in bid/ask spreads for NYSE stocks. The Journal of Finance, 47(2), 753–764. https://doi.org/10.1111/j.1540-6261.1992.tb04408.x
- Menyah, K., & Paudyal, K. (2000). The components of bid–ask spreads on the London Stock Exchange. Journal of Banking & Finance, 24(11), 1767–1785. https://doi.org/10.1016/S0378-4266(99)00102-8
- Miller, A. J. (1992). Algorithm AS 274: Least squares routines to supplement those of Gentleman. Journal of the Royal Statistical Society. Series C, Applied Statistics, 41(2), 458–478. http://www.jstor.org/stable/2347583
- Minardi, A. M. A. F., Sanvicente, A. Z., & Monteiro, R. (2006). “Bid-ask spread and liquidity premium in Brazil”, ( No. wpe_53). Insper Working Paper, Insper Instituto de Ensino e Pesquisa.
- Muggeo, V. M., & Adelfio, G. (2010). Efficient change point detection for genomic sequences of continuous measurements. Bioinformatics, 27(2), 161–166. https://doi.org/10.1093/bioinformatics/btq647
- Myers, K., Lawrence, E., Fugate, M., Bowen, C. M., Ticknor, L., Woodring, J., … Ahrens, J. (2016). Partitioning a large simulation as it runs. Technometrics, 58(3), 329–340. https://doi.org/10.1080/00401706.2016.1158740
- Narahari, Y. (2002). “Data structures and algorithms”, Lecture Notes, Department of Computer Science and Automation, Indian Institute of Science, Bangalore.
- Narayan, P. K., Mishra, S., & Narayan, S. (2014). Spread determinants and the day-of-the-week effect. The Quarterly Review of Economics and Finance, 54(1), 51–60. https://doi.org/10.1016/j.qref.2013.07.008
- Nath, G. C., Rajaram, S., Shiraly, P., & Dalvi, M. (2017). Determinants of bid-ask spread in the Indian government securities market. Money, Banking and Finance, 52(12), 25. https://www.epw.in/journal/2017/12/money-banking-and-finance/determinants-bid-ask-spread-indian-government-securities
- O’Hara, M. (2015). High frequency market microstructure. Journal of Financial Economics, 116(2), 257–270. https://doi.org/10.1016/j.jfineco.2015.01.003
- O’Hara, M., Saar, G., & Zhong, Z. (2019). Relative tick size and the trading environment. The Review of Asset Pricing Studies, 9(1), 47–90. https://doi.org/10.1093/rapstu/ray009
- Paital, R. R., & Sharma, N. K. (2016). Bid-ask spreads, trading volume and return volatility: Intraday evidence from Indian stock market. Eurasian Journal of Economics and Finance, 4(1), 24–40. https://doi.org/10.15604/ejef.2016.04.01.002
- Plerou, V., Gopikrishnan, P., & Stanley, H. E. (2005). Quantifying fluctuations in market liquidity: Analysis of the bid-ask spread. Physical Review E, 71(4), 046131. https://doi.org/10.1103/PhysRevE.71.046131
- Rahman, S., Lee, C. F., & Ang, K. P. (2002). Intraday return volatility process: Evidence from NASDAQ stocks. Review of Quantitative Finance and Accounting, 19(2), 155–180. https://doi.org/10.1023/A:1020683012149
- Rath, S. (2004). The hidden costs of trade execution. JASSA, 4, 35–37.
- Riedl, E. J., & Serafeim, G. (2011). Information risk and fair values: An examination of equity betas. Journal of Accounting Research, 49(4), 1083–1122. https://doi.org/10.1111/j.1475-679X.2011.00408.x
- Ripamonti, A. (2016). Corwin-Schultz bid-ask spread estimator in the Brazilian stock market. Brazilian Administration Review, 13(1), 76–97. https://doi.org/10.1590/1807-7692bar2016150036
- Rojcek, J., & Ziegler, A. (2016). “High-frequency trading in limit order markets: Equilibrium impact and regulation”, Swiss Finance Institute Research Paper, 15–23.
- Roll, R. (1984). A simple implicit measure of the effective bid‐ask spread in an efficient market. The Journal of Finance, 39(4), 1127–1139. https://doi.org/10.1111/j.1540-6261.1984.tb03897.x
- Schwartz, R. A. (1988). Equity Markets: Structure, Trading, and Performance. HarperCollins College Div.
- Seddon, J. J., & Currie, W. L. (2017). A model for unpacking big data analytics in high-frequency trading. Journal of Business Research, 70, 300–307. https://doi.org/10.1016/j.jbusres.2016.08.003
- Stoll, H. R. (1978). The supply of dealer services in securities markets. The Journal of Finance, 33(4), 1133–1151. https://doi.org/10.1111/j.1540-6261.1978.tb02053.x
- Su, E., & Tokmakcioglu, K. (2020). A comparison of bid-ask spread proxies and determinants of bond bid-ask spread. In Borsa Istanbul Review. https://www.sciencedirect.com/science/article/pii/S2214845020300661
- Tinic, S. M., & West, R. R. (1972). Competition and the pricing of dealer service in the over-the-counter stock market. Journal of Financial and Quantitative Analysis, 7(3), 1707–1727. https://doi.org/10.2307/2329797
- Wang, C., Chen, M. H., Schifano, E., Wu, J., & Yan, J. (2016). “Statistical methods and computing for big data”, arXiv preprint: https://arxiv.org/abs/1502.07989; https://dx.doi.org/10.4310/SII.2016.v9.n4.a1.
- Wang, G. H., Michalski, R. J., Jordan, J. V., & Moriarty, E. J. (1994). An intraday analysis of bid‐ask spreads and price volatility in the S&P 500 Index futures market. Journal of Futures Markets, 14(7), 837–859. https://doi.org/10.1002/fut.3990140706
- Wang, J. (1999). Asymmetric information and the bid-ask spread: An empirical comparison between automated order execution and open outcry auction. Journal of International Financial Markets, Institutions and Money, 9(2), 115–128. https://doi.org/10.1016/S1042-4431(99)00002-5
- Zhang, M. Y., Russell, J. R., & Tsay, R. S. (2008). Determinants of bid and ask quotes and implications for the cost of trading. Journal of Empirical Finance, 15(4), 656–678. https://doi.org/10.1016/j.jempfin.2007.12.003