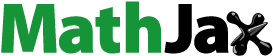
Abstract
This paper investigates whether the size of the shadow economy increases income inequality in Uganda. This p3aper applies the autoregressive distributed lag (ARDL) bounds testing approach to cointegration, to test the long- and short-run relationship between the shadow economy and income inequality. The results indicate a positive and statistically significant relationship between the size of the shadow economy and income inequality in both the long-run and short-run, all else equal. The results show that a large size of the shadow economy significantly increases income inequality, in both the long- and short-run. This suggests that people who fail to be absorbed into the formal economy face fewer livelihood opportunities, giving them the incentive to operate in the shadow economy as a means of survival, for them and their families since there are fewer chances of success in the formal economy. Our findings suggest that income inequality could be partially driven by increasing informality in the country. The practical implication of these results is that policies aimed at tackling income inequality should also be directed at addressing the underlying factors that drive the shadow economy.
PUBLIC INTEREST STATEMENT
Income inequality and shadow activities are two of the most challenging issues of our time. Both of them impose costs to the economy and society as a whole because of the distortion they cause to the realization of equitable income distribution and fiscal economic goals. Increasing income inequality undermines government effort to realize social equity, while a large size of the shadow economy leads to a fall in tax revenue because businesses operating underground evade taxes which results into tax revenue shortfall and worsening of poverty and income inequality. There is evidence that a large size of the shadow economy worsens income inequality both in the long-run and short-run.
1. Introduction
This paper investigates whether the size of the shadow economy increases income inequality in Uganda. Specifically, do changes in the shadow economy lead to changes in income inequality? This is a pertinent question that has many policy implications for our time since both of these variables affect economic growth (Dell’Anno, Citation2016a). For instance, where the shadow economy is disproportionately large, effective enforcement may curb its size but may also worsen income inequality and slow down growth given that most of those engaged in the shadow economy are poor and marginalized. As indicated by United Nations (Citation2020), widening income inequality is a global challenge of our time for both the developed and developing countries. Rising inequality is a matter of great concern to many policy makers because it signals a lack of opportunity and income mobility which reflects a persistent vulnerability of certain segments of the population (Hailemariam & Dzhumashev, Citation2020). It affects the momentum in which growth enhancing policies can influence poverty reduction as shown by Ravallion (Citation2001). This implies that in countries where income inequality is at its highest, implementation of any growth enhancing policies may have little or no effect on poverty reduction rendering a larger proportion of the population more vulnerable to poverty. The rising trend in income inequality across many countries in recent years generates renewed interest in understanding its drivers and consequences (Brueckner & Lederman, Citation2018). In a number of countries, the income gap between the poor and the rich has continued to widen over the last decades, which has become a cause of concern to policy makers (United Nations, Citation2020). Given this worrying trend in income inequality, investigating some of the underlying factors that drive the rising trend remains an important area of concern.
Moreover, widening income inequality has significant policy implication for macroeconomic stability and growth since it concentrates decision making and political power in the hands of the very few who have the resources to influence the political decisions in the country. This implies that decisions will be based on political loyalty and not on principles of equity and fairness. This leads to suboptimal use of economic and human resources which increases economic vulnerability in the country (Rajan, Citation2010). As shown by Stiglitz (Citation2012), high and sustained levels of income inequality may create large social costs and undermine government’s ability to advance citizens welfare. Furthermore, Berg and Ostry (Citation2011) show that high-income inequality negatively affects economic growth and perpetuates poverty among the poor members of society. With high-income inequality, citizens can lose confidence in their government for failure to ensure better living conditions for them. This provides an incentive for them to channel their efforts towards opportunities that secure their future, such as running businesses outside the “radar” of regulators. This leads to misallocation of economic resources, from the formal economy to the informal one, hence the rise of the activities in the informal sector. This way, income inequality and the shadow economy become interrelated. The increase in the size of the shadow economyFootnote1 is also an issue of concern because of its distortionary nature. First, shadow economy activities are difficult to measure and tax since they are concealed from the “eyes” of public officials and regulators.
Given the above discourse, it is apparent that pervasive rates of income inequality have become an issue of grave concern. Thus, the extent of income inequality and its drivers is one of the hotly debated issues by economist and policy makers, in the face of the rising gap between the poor and the rich, over the recent decades. This paper attempts to ask whether any changes in the size of the shadow economy lead to changes in income inequality. Put another way, does the size of the shadow economy increase income inequality in Uganda? Recently, estimates of the shadow economy have shown an upward trend implying that a substantial amount of goods and services are being produced in outside of the formal economy. For example, recent estimates by Medina and Schneider (Citation2019) indicate that across the 157 countries (both developed and developing) surveyed, the shadow economy accounts for an average of 31% of gross domestic product (GDP) worldwide. Of the 157 countries surveyed, Latin America topped the charts with Bolivia recording the highest; that is, 62.9% of GDP, followed by Europe, where Georgia recorded nearly 62%. Similarly, African countries showed rising trends as well, with Nigeria recording 56.8%, followed by Tanzania, with 56% (Medina & Schneider, Citation2019). This implies that the shadow economy is on the rise, contrary to the notion put forward by Harris and Todaro (Citation1970) that the informal sector is an unproductive and stagnant sector which is a source of livelihood to largely the urban unemployed and a “refuge” for the new arrivals (migrants) from rural areas who have no productive skills.
As evidenced by the rising trends of this sector, the informal sector has become central to the production of goods and services and creation of the much needed jobs (Esaku, Citation2019a, Citation2020; Fourie, Citation2018; Organization, Citation2002; Mugoda et al., Citation2020). There is a strong recognition that the informal sector is not a temporary phenomenon but rather a persistent sector that dominates the business landscape of many countries. Notwithstanding its challenges, this sector plays an important role in providing a source of livelihood for the poor and vulnerable members of society. Some of the reasons for its rise seem to point to failure by the formal economy to absorb the unemployed population, who seem to possess semi or no skills at all (Fourie, Citation2018) and high taxes and burdensome regulatory framework (Elgin & Erturk, Citation2019; Goel & Nelson, Citation2016).
The shadow economy and income inequality have become two intertwined social challenges in both developing and developed countries (Dell’Anno, Citation2016a). There is reason to suggest that the size of the shadow economy could be a significant driver of income inequality. For instance, Chong and Gradstein’s (Citation2007) model shows that a rise in income inequality leads to an increase in the size of the shadow economy as the poor seek alternative sources of survival. According to the above authors, this effect is compounded in the presence of weak institutions or institutional framework that does not provide effective tools for enforcement. Similarly, there is indication in the literature that the direct link between income inequality and informality is on its impact on human capital accumulation. Widening income inequality among the poor means that they cannot afford decent education and health care for their children, which in turn affects human capital formation among the poor (Dell’Anno, Citation2016a). Moreover, empirical evidence supports the view that poorer families produce more children than their counterparts that are rich, hence increasing income inequality among these poor households decreases human capital accumulation due to higher fertility rates. Consequently, a large proportion of children (would be workers) may still remain poor and unskilled which makes them unlikely to join the formal sector but rather operate informally in order to survive.
Although there is considerable work in the extant literature on income inequality, a few studies address the question of whether a larger size of the shadow economy increases income inequality. Previous works have examined the relationship between income inequality and the size of the shadow economy by focusing on whether income inequality increases the size of the shadow economy. Moreover, their analyses were largely based on panel data and cross-sectional regression approaches. Such studies rely on the assumption of homogeneity of coefficients, where they assume that economies are the same and findings can be generalized across all the countries (Keho, Citation2017). However, this may not always be the case as countries are heterogeneous with respect to quality of institutions, level of development, business environment, technology and other factors. This cross-sectional homogeneity assumption can be violated, leading to policy recommendations that do not reflect the local economic conditions of the countries involved. This paper attempts to fill the above gap by investigating the relationship between income inequality and the size of the shadow economy in a country-level analysis. Furthermore, this study explores whether changes in the size of the shadow economy cause changes in income inequality in a rarely studied context, Uganda. Issues of inequality have recently taken center stage in discussions concerning economic development in the country. As shown by Lwanga-Ntale (Citation2015), the degree of inequality in the country has been on the increased despite poverty reduction programs initiated by government over the past three decades. There is evidence that many households presumed to have “prospered” or improved their incomes over the past two decades, are still very vulnerable to poverty (Ssewanyana & Kasirye, Citation2012). As recently shown by the World Baank (Citation2019), poverty in the country has declined but at a slower rate between the period 2005 and 2012. However, inequality still remains an issue of grave concern. This could imply that higher economic growth alone might not be adequate for poverty reduction and equitable income distribution. As a result, further reductions in poverty and income inequality require a deliberate effort towards inclusive growth and income equalizing policy framework.
This paper makes two important contributions to the literature. First, this study investigates an important relationship between the shadow economy and income inequality, which has many implications for policy. For instance, how can policies be crafted that shape the size of informal sector activities in the presence of high-income inequality? Countries across the world are trying to mitigate the expansion of the informal sector activities but to no avail. This would require addressing the key drivers of the shadow economy and ensuring that effort is devoted to tackling underlying causes of income inequality. Second, this paper provides evidence of the relationship between income inequality and the shadow economy in the context of Uganda, and Africa in general. Uganda offers the best testing ground for our empirical ideas on account that it shares a number of characteristics with most countries in Africa.
The remainder of the paper is structured as follows. Section 2 reports the data and descriptive statistics. Section 3, presents the methodology, while section 4 reports the findings and discussion, and section 5 concludes the paper.
2. Data and descriptive statistics
This section presents the data and data sources. The data are annual time series drawn from a number of internationally recognized sources, and cover the period from 1991 to 2015. First, data for the outcome variable, income inequality (gini) is taken from The Standardized World Income Inequality Database (SWIID) developed by Solt (Citation2020) version 9.0. Gini gives an estimate of Gini index in equivalized household disposable income calculated following Luxembourg income data as the standard measure. Similarly, also used is Gini index equivalized in household market income (gim), to check the robustness of our findings. This variable is also from the same source. Second, our main explanatory variable, the size of the shadow economy (se15) is from Medina and Schneider (Citation2018), covering the period from 1991 to 2015. Included are a number of control variables: inflation (inf), economic growth (gw), government expenditure as a proportion of gross domestic product (GDP), denoted as (Gov/gdp), control of corruption (cor), institutionalized democracy (Demo) and financial development (Fd). Inflation is measured by consumer price index; economic growth is measured by growth of GDP; and financial development (Fd) is measured using money and quasi money as a share of GDP (M2q). These variables including Gov/gdp are from World Development Indicators (World Bank, Citation2014, Citation2020). Control of corruption index is our preferred measure of institutional quality, and is from International Country Risk Guide (International Country Risk Guide (ICRG) Researchers, Citation2017) published by Political Risk Services (PRS). Our measure of institutionalized democracy is drawn from the Center for Systemic Peace (Polity5 version). The summary and data sources of the main variables is provided in Appendix A, Table A.1.
, panels (a) and (b), presents summary statistics and correlation matrix, respectively. The average values of the key variables are shadow economy (Se15), 38.743, income inequality (gini), 43.854, gim, 46.308, inflation (inf), 8.146, growth (gw), 3.254, government expenditure (Gov/gdp), 11.607, control of corruption (cor), 2.160, institutionalized democracy (Demo), 0.462, and financial development (Fd), 15.550. Apart from summary statistics, the correlation matrix is presented in panel (b). In Panel (b) the variables of interest (gini and gim) have a negative correlation with the size of the shadow economy. This could imply that the shadow economy influences these variables in a negative manner. However, negative correlation does not necessarily imply that a large size of the shadow economy reduces income inequality. This calls for formal long-run and short-run tests to determine if this assumption holds.
Table 1. Summary statistics and correlation matrix
3. Methodology
This section presents model specification and econometric methodology used in the empirical exercises. In the model specification, the relationship between the size of the shadow economy and inequality is formally expressed in Equationequation (1)(1)
(1) . Then, econometric model used in the estimation is also expressed formally in Equationequation (2)
(2)
(2) .The above are described in the next sections in detail.
3.1. Model specification
This section details the empirical model for testing the long- and short-run relationship between the main variables. Accordingly, this paper posits that income inequality is influenced by variables that can be expressed as follows:
where Gini is the measure of income inequality in equivalized household disposable income, se15 is the size of the shadow economy, inf denotes inflation, gw is economic growth or simply, growth. Similarly, Gov/gdp is government expenditure as a share of GDP, cor is corruption control index, demo is institutionalized democracy and Fd which denotes financial development is measured using money and quasi money as a share of GDP (m2q). These explanatory variables are considered following our review of the literature and in line with previous studies. Recent studies show that the shadow economy can influence the size of income inequality, implying that the two variables could be correlated (Dell’Anno, Citation2016a). Inflation is added as a control variable, which has also been noted to be a significant determinant of income inequality (see Siami-Namini & Hudson, Citation2019). Additionally, governance is important for the successful allocation of productive resources and provision of public goods and services hence we include demo variable to proxy for governance in the country. Furthermore, institutional quality and/or control of corruption matters in shaping the structure of economic activities in the economy. Furthermore, the level of a country’s development is also important in the provision of public goods and services that have the potential to improve the quality of life of the citizenry (see, Goel & Nelson, Citation2016). This implies that improvement in economic growth could either increase or reduce income inequality (see Fawaz et al., Citation2014; Rajaram, Citation2012; Rubin & Segal, Citation2015). Hence, the level of economic development is added as a control variable, proxied by GDP growth per capita. Correspondingly, recent studies show that government spending is important for the provision of public goods and services which could also have a positive effect on the welfare of the poor citizens hence reducing the income gap between the rich and the poor (see Doumbia & Kinda, Citation2019; Lustig et al., Citation2013). Accordingly, this variable is included in the main estimation equation. Lastly, some studies have shown the importance of financial development in reducing income inequality (see Clarke et al. Citation2006), although these results are disputed by other studies (see Seven & Coskun, Citation2016). Thus, this variable is also included into the main estimation model. In what follows, this paper presents the econometric methodology in the next section.
3.2. Econometric methodology
This section details the econometric methodology that we follow in the estimation process. First, stationarity tests are carried out and then the ARDL bounds tests for the presence of long-run relationship between the variables are implemented in the second step. In the third step, empirical estimation of the ARDL model for testing the relationship among variables is carried out. Accordingly, this paper follows the ARDL bounds testing approach to cointegration introduced by Pesaran et al. (Citation2001), which has benefits over traditional cointegration techniques. Specifically, this approach is considered a robust econometric method for analyzing time-series data regardless of the sample size (Tang, 2010), and it can be employed regardless of the order of integration of the variables, whether the variables are integrated of order zero, I(0)s and/or one, I(1)s. This means that variables can either be I(0)s and/or I(1)s but not I(2)s (Pesaran & Shin, Citation1999). Thirdly, the ARDL method has the advantage that it can correct for any possible endogeneity among the independent variables (Wolde-Rufael, Citation2010).
The ARDL model for the empirical estimation of Equationequation (1)(1)
(1) is expressed as follows:
where denotes the constant term while
denote the short-run and long-run coefficients, respectively, and
denotes the error term.
To implement ARDL bounds testing procedure, this paper first tests for cointegration relationship among variables to ascertain the existence of a long-run relationship among the variables using the F-statistic. The null hypothesis of no cointegration, is then tested against the alternative hypothesis that there is cointegration among variables,
. The result of the F-statistic is then compared to the critical values specified in Pesaran et al. (Citation2001). The decision rule is as follows: if the calculated values of F-statistic are above the upper critical bound values, the
(the null hypothesis) is rejected and vice versa. But, if the F-statistic values fall within the bounds, then it means that the test result is inconclusive. Before conducting ARDL bounds testing procedure, the optimal lag length for the ARDL model is first ascertained. This is ascertained according to the appropriate lag length selection criteria based on the Schwartz–Bayesian criterion (SBC).
Based on results of the cointegration test on Equationequation (2)(2)
(2) , this paper proceeds to express the error correction model (ECM), if there is a long-run relationship, as follows:
where denotes the coefficient of the ECT (error correction term) capturing the long-run adjustment to the equilibrium after deviations, while
is the residual error term. The importance of the ECT coefficient lies in its size and sign, which denotes the speed of adjustment and validity of the results. Thus, the coefficient of the error correction term (
) should be negative, less than 1 and statistically significant as shown in Enders (Citation2004).
4. Results and discussion
4.1. Stationarity tests
To conduct the empirical tests, this paper first tested the variables to determine whether they are integrated of order zero, I(0), and/or order one, I(1) using two different stationarity tests. This paper used Augmented-Dickey–Fuller test (ADF) and Phillip–Perron (PP) tests, with intercept and with trend and intercept. The results of these tests are reported in . confirms that the main variables are either stationary in levels or after first differencing, and vary according to the type of stationarity test used. After conducting unit root tests, this paper then proceeded to implement the ARDL bounds testing approach. In panel (a), this paper formally expressed the equations to be tested, while panel (b) reports the results of the ARDL bounds test for cointegration. As can be seen from panel (b), the calculated F-statistic is higher than the asymptotic critical value bounds given in Pesaran et al. (Citation2001). Given the results of the F-statistic, this paper rejects the null hypothesis of no cointegration and concludes that the variables are cointegrated. Additionally, our diagnostic tests show that these results are reliable and not driven by any biases. After the tests, this study proceeded to estimate the long-run and short-run coefficients for the model by first determining the optimal lag length according to the Schwartz information criterion (SIC), which selected ARDL (2,1,0,0,0,1,0,0) model for Equationequation (1)(1)
(1) and ARDL (2,1,1,2,2,2,2,2) model for Equationequation (2)
(2)
(2) .
Table 2. Results of stationarity tests for all the variables
Table 3. Results of the ARDL bounds test
4.2 The long-run relationship between the income inequality and the shadow economy
In this section, the results of the long-run relationship between the size of the shadow economy and income inequality are presented. First, the ARDL results are reported in , columns 2 and 6. Then the fully modified ordinary least squares (FMOLS) technique is used as a robustness check on the results of ARDL model. The results of FMOLS are reported in columns 4 and 8. From , column 2, the results indicate that a large size of the shadow economy is directly correlated with an increase in income inequality in the long-run, all else equal. The coefficient on the size of the shadow economy (se15) is positive and statistically significant at 1% level. This suggests that a large size of the shadow economy has a positive impact on income inequality in the long-run. Specifically, these results confirm that an increase in the size of the shadow economy significantly increases income inequality by 0.016 units. This implies that addressing the factors that drive the expansion of shadow economy could also help mitigate the rise in income inequality in the long-run.
Table 4. Long-run relationship between income inequality and the size of the shadow economy
Furthermore, these findings seem to line up with the suggestion that increased level deprivation among citizens increases incentives for them to engage in shadow activities so as to survive. This suggestion seems to agree with the view that the shadow economy is a “refuge” for segments of the population who cannot find employment in the formal economy (Fourie, Citation2018; Organization, Citation2002). Indeed, this sector plays a key role in supplementing the role of the formal sector. However, the shadow economy has also its disadvantages given that informal sector workers miss out on social security contributions and health insurance that formal sector workers get. Moreover, workers in the informal sector earn wages since a number of them are non-salaried. Given that this sector pays fewer benefits to its workers, this decreases their incomes hence the rise in the income gap between the rich and the poor. Since the bulk of workers in the informal sector are poor and unskilled, a large size of the shadow economy should really increase income inequality.
The above positive long-run relationship between the size of the shadow economy and income inequality could have important implications. One main implication of this long-run relationship is that a large size of the shadow economy is detrimental to the fight against income inequality. In low-income countries like Uganda where poverty and political patronage exerts pressure on resource allocation, operating in the informal sector is a necessity (so that one can survive) rather than wanting to exploit a business opportunity (see Chong & Gradstein, Citation2007). Given this, the shadow economy reinforces income inequality instead of curbing it. This implies that citizens with poor livelihood opportunities will be more inclined to operate in the shadow economy since their chances of success in the formal economy are limited.
The rest of the results in are also discussed. From , it is shown that an increase in economic growth leads to a reduction in income inequality consistent with the findings of Rajaram (Citation2012). An increase in economic growth leads to 0.001 units decline in income inequality. A look at the coefficient on government expenditure (spending) shows that increased government spending leads to increased income inequality. The coefficient on government expenditure shows that an increase in government spending leads to 0.028 units increase in income inequality, statistically significant at 1% level. Similarly, an improvement in the control of corruption reduces income inequality by 0.041 units, statistically significant at 10% level. Improvement in the level of institutionalized democracy (demo) reduces income inequality by 0.157 units. In the same vein, an improvement in financial development increases income inequality by 0.069 units statistically significant at 1% level. This finding is in line with the empirical evidence in Seven and Coskun (Citation2016) which shows that financial development seems to hurt those with lower levels of income.
As a robustness check, Menegaki (Citation2019) suggests to use either dynamic ordinary least squares (DOLS) and/or fully modified ordinary least squares (FMOLS) to verify the robustness of the findings since these two econometric techniques can generate asymptotically efficient coefficients while addressing the issue of endogeneity and serial autocorrelation at the same time (Menegaki, Citation2019). These results are reported in columns 4 and 8. These results are qualitatively and quantitatively similar to the ARDL results. One can conclude that an increase in the size of the shadow economy significantly increases income inequality in the long-run, in the case of Uganda. Further, this paper conducts residual diagnostics to ensure that these results are not driven by any bias. Specifically, implemented are Breusch–Godfrey serial correlation LM, Heteroskedasticity- Breusch–Pagan–Godfrey (BPG), and normality tests. The results of these tests show no evidence of any bias in the empirical estimation of the ARDL models. In sum, it can be concluded that a large size of the shadow economy widens income inequality, all else equal.
4.3 The short-run relationship between income inequality the shadow economy
This section presents the short-run results of empirical analysis in . Column 2 reports results for Equationequation (1)(1)
(1) , while column 5 reports the results for Equationequation (2)
(2)
(2) . It can be observed that the coefficients on gini and gim are positive and statistically significant at 5% level. This would imply that, in the short-run a large size of the shadow economy increases income inequality, all else equal. This paper therefore establishes that the shadow economy and income inequality are correlated both in the long-run and short-run, a finding that is important for policy.
Table 5. Short-run relationship between income inequality and the size of the shadow economy
This paper argues that shadow activities do increase inequality and could be detrimental to economic growth on account that entrepreneurs who operate in this sector rarely pay tax, leading to tax evasion. Tax evasion hinders governments’ effort to collect tax revenues which could be used to provide public goods and services. These public goods and services such as infrastructure, hospitals, education, and security, among others are important in improving the welfare of the population. If there are inefficiencies that cause difficulty in the provision of these public goods and services, then governments will be unable to meet this obligation which increases impoverishment among the poor. Consequently, the income gap between the rich and the poor widens resulting into sections of the population engaging in shadow activities. Indeed, the shadow economy is considered a “refuge” for people who cannot find employment in the formal sector (Fourie, Citation2018; Organization, Citation2002).
Relatedly, this paper also discusses the remainder of the findings. In column 2, an increase in economic growth reduces income inequality by 0.002 units. This finding seems to agree with the suggestion of Rajaram (Citation2012) who shows that an increase in the level of economic growth reduces income inequality. Correspondingly, we find evidence that an increase in government spending increases income inequality, instead of reducing it. Some studies have shown that government spending is effective in reducing income inequality only if this spending is on social transfers (protection) and infrastructure (see Doumbia & Kinda, Citation2019; Lustig et al., Citation2013). Similarly, the coefficient on corruption seems to indicate that high corruption reinforces income inequality, statistically significant at 10% level. Correspondingly, these findings seem to suggest that an improvement in institutional democracy reduces income inequality by 0.149 units, statistically significant at 1% level. Additionally, the coefficient on financial development is positive and statistically significant at 1% level. This finding indicates an improvement in financial development increases income inequality since it is mostly the rich who benefit from it (Seven & Coskun, Citation2016). This is a plausible explanation because poor segments of the population find it difficult to access domestic credit since most often they lack collateral which is needed for securing credit (Seven & Coskun, Citation2016). Consequently, lenders of domestic credit view targeting poor segments of the population as a risky venture because they often default in their loan repayments. This implies that financial development will continue to serve the rich who can afford the terms of lending, which in turn widens the gap between the rich and the poor.
The evidence of both the long- and short-run relationship between the shadow economy and inequality agrees with previous studies that provide evidence of a positive relationship between these two variables. For example, Mishra and Ray (Citation2010) study the relationship between income inequality and the shadow economy, in a sample of 68 economies and find empirical evidence indicating that high level of inequality reinforces the size of the shadow economy. Similarly, Berdiev and Saunoris (Citation2019) use a panel of 144 economies to examine the relationship between income inequality and the size of the shadow economy and establish a bidirectional positive relationship between these variables. This paper provides additional evidence of the long- and short-run relationship between the shadow economy and income inequality using Uganda as a case.
Finally, analyzing how the economy adjusts to any deviations from long-run equilibrium, this finds that the lagged coefficient of the error correction term (ECT) is negative and statistically significant at 1% level. The ECM results imply that income inequality adjusts to any deviations from long-run equilibrium at a speed of adjustment that is shown by the coefficient of the lagged error correction term which is 93.6% and statistically significant at 1% level. In sum, these findings suggest that addressing the underlying factors that drive the shadow economy in Uganda could be partially an effective policy option for reducing income inequality in both the long-run and short-run. This seems to be a viable policy option since income inequality and the size of the shadow economy are positively correlated. The practical implication of these results is that policies aimed at tackling income inequality should also be directed at addressing the underlying factors that drive the shadow economy, such as poverty, high taxes, overregulation, and corruption among others. Also conducted is residual diagnostics to ascertain whether these findings are reliable. Both the results of Breusch–Godfrey serial correlation LM, Heteroskedasticity; Breusch–Pagan–Godfrey (BPG) and normality tests show no evidence of any biases in the empirical estimation of the ARDL models.
4.4. Diagnostic tests
After the estimation of the long-run and short-run estimates of the relationship between the size of the shadow economy and income inequality, this paper conducted stability diagnostics by analyzing recursive residuals. The plots of cumulative sum of recursive residuals (CUSUM) and the plots of cumulative sum of squared residuals (CUSUMQ) for both long-run and short-run coefficients are reported. report both the CUSUM and CUSUMQ plots and provide further evidence on the validity of the estimated ARDL models, for Equationequation (1)(1)
(1) and Equationequation (2)
(2)
(2) . All these plots present evidence showing that the ARDL models estimated are stable. As shown in these two figures, the results of the residual plots do not cross the boundaries at 5% level of significance which confirms that there is stability in the parameters of the ARDL models.
Figure 1. Plots for CUSUM and CUSUMQ for long-run and short-run coefficients Equationequation (1)(1)
(1)

Figure 2. Plots for CUSUM and CUSUMQ for long-run and short-run coefficients Equationequation (2)(2)
(2)

5. Conclusion
This paper examines whether a large shadow economy increases income inequality. This study is done at the country-level applying the ARDL modeling technique using annual time-series data drawn from a variety of data sources, covering the period from 1991 to 2015. This paper finds that a large shadow economy significantly increases income inequality, in both the long-run and short-run, all else equal. These results suggest that, for the case of Uganda, a large size of the shadow economy seems to reinforce income inequality instead of curbing it. This would seem to suggest that people who fail to be absorbed into the formal economy face fewer livelihood opportunities, giving them the incentive to operate in the shadow economy as a means of survival for them and their families since there are fewer chances of success in the formal economy. This results into an increasing income gap between the rich and the poor, inducing the poor to seek livelihood opportunities in the shadow economy. The practical implication of these results is that policies aimed at tackling income inequality should also be directed at addressing the underlying factors that drive the shadow economy, such as poverty, high taxes, overregulation, and corruption among others. Finally, one main limitation of this study is that the findings are drawn from rigorous empirical exercises; future studies could add theoretical models to further explain the relationship between income inequality and the shadow economy and channels through which this relationship thrives.
Acknowledgements
The author is thankful to the editor and anonymous reviewers of the journal for their helpful comments that improved the quality of this paper. The remaining errors and omissions are solely the responsibility of the author.
Additional information
Funding
Notes on contributors
Stephen Esaku
Stephen Esaku is a lecturer of Economics in the department of Business and management Cavendish University Uganda. He holds a PhD in Economics specializing in international economics and Development. His research interests are in the areas of international economics (firms in international trade, regional integration, foreign direct investment), and Development economics (focusing on economic growth and development, poverty dynamics, corruption and gender studies) and empirical industrial economics (learning, pricing and market strategies). Stephen has published papers on firm-level investment and Exporting; Export markets and productivity; employment dynamics in Sub-Saharan Africa; Job creation and destruction in an African economy, among others. His teaching experiences spans 8 years.
Notes
1. In this paper shadow economy, informal sector/economy, underground economy, unobserved economy/sector; mean the same thing, and we use these terms interchangeably.
References
- Berdiev, A. N., & Saunoris, J. W. (2019). On the relationship between income inequality and the shadow Economy. Eastern Economics Journal, 45(2), 224–20. https://doi.org/10.1057/s41302-018-0120-y
- Berg, A., & Ostry, J. D. (2011). Inequality and unsustainable growth: Two sides of the same coin? IMF staff discussion note 11/08. International Monetary Fund.
- Brueckner, M., & Lederman, D. (2018). Inequality and economic growth: The role of initial income. Journal of Economic Growth, 23(3), 341–366. https://doi.org/10.1007/s10887-018-9156-4
- Chong, A., & Gradstein, M. (2007). Inequality and Informality. Journal of Public Economics, 91(1–2), 159–179. https://doi.org/10.1016/j.jpubeco.2006.08.001
- Clarke, G. R., Xu, L. C., & Zou., H-F. (2006). Finance and Income Inequality: What Do the Data Tell Us? Southern Economic Journal, 72(3), 578–596. https//:doi.org/10.2307/20111834
- Dell’Anno, R. (2016a). Analysing the determinants of the shadow economy with a “Separate Approach”. An application of the relationship between inequality and the shadow economy. World Development, 84(2016), 342–356. https://doi.org/10.1016/j.worlddev.2015.08.026
- Dell’Anno, R. (2016b). Inequality and informality in transition and emerging countries. IZA World of Labor, 2016, 325. https://doi.org/10.15185/izawol.325
- Doumbia, D., & Kinda, T. (2019). Reallocating public spending to reduce income inequality: Can it work?IMF Working Paper No. 19/188
- Elgin, C., & Erturk, F. Informal economies around the world: Measures,determinants and consequences. (2019). Eurasian Economic Review, 9(2), 221–237. 2019. https://doi.org/10.1007/s40822-018-0105-5
- Enders, W. (2004). Applied Econometric time series (2nd ed.). John Wiley & Sons.
- Esaku, S. (2019). Trade liberalisation, firm dynamics and export market participation in Sub-Saharan Africa. Doctoral Thesis: North-WestUniversity Press. Avaliable at:.http://dspace.nwu.ac.za/handle/10394/33122.https://scholar.google.com/citations?user=gC6nudMAAAAJ&hl=en
- Esaku, S. (2020). Job creation, job destruction and reallocation in Sub-Saharan Africa: Firm-level evidence from Kenyan manufacturing sector. Cogent Economics & Finance, 8(1), 1-22. https://doi.org/10.1080/23322039.2020.1782113
- Fawaz, F., Rahnama, M., & Valcarcel, V. J. (2014). A refinement of the relationship between economic growth and income inequality. Journal of Applied Economics, 46(27), 3351–3361. https://doi.org/10.1080/00036846.2014.929624
- Fourie, F. (2018). Enabling the forgotten sector: Informal sector realities, policy approaches and formalization in South Africa. In C. V. Frederick & N. Fourie (Eds.), The South African informal sector: Creating jobs, reducing poverty (pp. 439-476). Human Sciences Research Council.
- Goel, R. K., & Nelson, M. A. (2016). Shining a light on the shadows: Identifying robust determinants of the shadow economy. Economic Modelling, 58, 351–364. https://doi.org/10.1016/j.econmod.2016.06.009
- Hailemariam, A., & Dzhumashev, R. (2020). Income inequality and economic growth: Heterogeneity and nonlinearity. Studies in Nonlinear Dynamics & Econometricss, 24(3), 2020. https://doi.org/10.1515/snde-2018-0084
- Harris, J. R., & Todaro, M. P. (1970). Migration, unemployment and development: A two-sector analysis. American Economic Review, 60(1), 126–142. https://www.aeaweb.org/aer/top20/60.1.126-142.pdf
- International Country Risk Guide (ICRG) Researchers (2017), ‘International Country Risk Guide (ICRG) researchers dataset’. https://doi.org/10.7910/DVN/4YHTPU, Havard Dataverse, V1. Political Risk Services Group.
- Keho, Y. (2017). The impact of trade openness on economic growth: The case of Cote d’Ivoire. Cogent Economics & Finance, 5(1), 1332820. https://doi.org/10.1080/23322039.2017.1332820
- Lustig, N., Lopez-Calva, L. F., & Ortiz-Juarez, E. (2013). Declining inequality in Latin America in the 2000s: The cases of Argentina, Brazil, and Mexico. World Development, 44, 129–141. https://doi.org/10.1016/j.worlddev.2012.09.013
- Lwanga-Ntale, C. (2015). Inequality in Uganda: Issues for discussion and further research. Development, 57(3–4), 601–617. https://doi.org/10.1057/dev.2015.44
- Medina, L., & Schneider, F. (2018). Shadow economies around the world: what did we learn over the last 20 years?IMF Working Paper No. 1817
- Medina, L., & Schneider, F. (2019). Shedding light on the shadow economy: A global database and the interaction with the official one. CESifo Working Paper No. 7981
- Menegaki, A. N. (2019). The ARDL method in the energy-growth nexus field; best implementation strategies. Economies, 7(4), 105. https://doi.org/10.3390/economies7040105
- Mishra, A., & Ray, R. (2010). Informality, corruption and inequality.Bath economics research paper no. 13/10. Department of Economics, University of Bath
- Mugoda, S., Esaku, S., Nakimu, R. K., & Bbaale, E. (2020). The portrait of Uganda’s informal sector: What main obstacles do the sector face? Cogent Economics & Finance, 8(1), 1843255. https://doi.org/10.1080/23322039.2020.1843255
- Organization, I. L. (2002). Decent work and the informal economy. International Labour Office.
- Pesaran, H., Shin, Y., & Smith, R. J. (2001). Bounds testing approaches to the analysis of level relationships. Journal of Applied Econometrics, 16, 289–326. https://doi.org/10.1002/()1099-1255
- Pesaran, M. H., & Shin, Y. (1999). An utoregressive distributed lag modelling approach to cointegration analysis. S. Strom Ed., Econometrics and economic theory in the 20th century. Chapter 11 (371–413). The Ragnar Frish Centennial Symposium, Cambridge University Press.
- Rajan, R. G. (2010). Fault lines: how hidden fractures still threaten the world economy. Princeton University Press.
- Rajaram, R. (2012). Poverty, income inequality and economic growth: A spatial analysis for the US counties. The Indian Economic Journal, 60(2), 73–88. https://doi.org/10.1177/0019466220120206
- Ravallion, M. (2001). Growth, inequality and poverty: Looking beyond averages. World Development, 29(11), 1803–1815. https://doi.org/10.1016/S0305-750X(01)00072-9
- Rubin, A., & Segal, D. (2015). The effects of economic growth on income inequality in the US. Journal of Macroeconomics, 45, 258–273. https://doi.org/10.1016/j.jmacro.2015.05.007
- Seven, U., & Coskun, Y. (2016). Does financial development reduce income inequality and poverty? Evidence from emerging countries. Emerging Markets Review, 26, 34–63. https://doi.org/10.1016/j.ememar.2016.02.002
- Siami-Namini, S., & Hudson, D. (2019). Inflation and income inequality in developed and developing countries. Journal of Economic Studies, 46(3), 611–632. https://doi.org/10.1108/JES-02-2018-0045
- Solt, F. (2020). Measuring income inequality across countries and over time: The standardized world income inequality database. Social Science Quarterly, SWIID Version 9.0. 101(3), 1183–1199. October 2020. https://doi.org/10.1111/ssqu.12795
- Ssewanyana, S., & Kasirye, I. (2012). Poverty and inequaity dynamics in Uganda: Insights from the Uganda national panel surveys 2005/06 and 2009/10.Research Series No. 94. Retrieve from: https://media.africaportal.org/documents/series94.pdf
- Stiglitz, J. (2012). The Price of Inequality. Pengiun UK.
- Tang, T. C. (2010). A reassessment of aggregate import demand function in the Asean-5: A cointegration analysis. The International Trade Journal, 18(3), 239–268. https://doi.org/10.1080/08853900490478131
- Teobaldelli, D., & Schneider, F. (2013). The influence of direct democracy on the shadow economy. Public Choice, 157(3–4), 543–567. https://doi.org/10.1007/s11127-013-0098-2
- United Nations (2020). World social report. https://www.un.org/development/desa/dspd/wp-content/uploads/sites/22/2020/01/World-Social-Report-2020-FullReport.pdf
- Wolde-Rufael, Y. (2010). Bounds test approach to cointegration and causality between nuclear energy consumption and economic growth in India. Energy Policy, 38(1), 52–58. https://doi.org/10.1016/j.enpol.2009.08.053
- World Baank. (2019). Impact of fiscal policy on poverty and inquality in Uganda. Fiscal Incidence Analysis Using the UNHS 2016/2017.Policy Research Working Paper 9051
- World Bank. (2014). World development indicators. World Bank. www.worldbank.org
- World Bank. (2020). World development indicators. World Bank. www.worldbank.org
Appendix A
Table 1. Summary of main variables and data sources