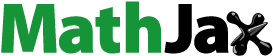
Abstract
This paper aims to understand the impact of demonetisation on the returns of listed firms in the NSE, as well as changes to their corresponding industry level systematic risk. Firstly, this paper examined a larger sample of Indian listed firms and a broader group of industries in its analysis compared to prior studies. Secondly, the changes to systematic risk due to demonetisation across industries with listed firms was measured. The study uses event study methodology over a thirteen-day event window (six days before and six days after the announcement) to test for abnormal returns across 1,054 listed firms. A regression analysis of 57 industry level returns is performed to test for changes in systematic risk. Significant negative abnormal returns are found for over 100 firms and 12 out of the 57 industry divisions in the sample. The same group of industries also show an increase in their systematic risk in the short run. This indicates that parts of the formal economy were also seriously affected by demonetisation. However, our paper does not find any long-term effect due to demonetisation.
PUBLIC INTEREST STATEMENT
The paper “Risk and returns of Indian listed firms after demonetisation” discusses how the stock returns and the systematic risk of firms listed in the National Stock Exchange were affected after demonetisation. Results showed that firms belonging to certain groups of industries, like real estate and construction, had negative abnormal returns. The risk levels for those industries also rose in the short run, suggesting that investors were wary of their future performance. A largely negative reaction to demonetisation, and an increase in risk that cannot be diversified (systematic risk) implies that investors expected firm profits to decline and its operations to be disrupted due to cash shortage. Results could help investors hedge their risk and become aware of industry level characteristics in the case of future shocks. Policymakers can better map out vulnerabilities by industries in the case of future policy initiatives, to help minimize disruptions to business operations and consumer activities.
1. Introduction
“To break the grip of corruption and black money, we have decided that the five hundred rupee and thousand-rupee currency notes presently in use will no longer be legal tender from midnight tonight, that is 8 November 2016”. The Ministry of Finance stated the following reasons for implementing demonetisation (Government of India, Ministry of Finance, Department of Economic Affairs, Citation2016):
Increasing volume of counterfeit notes
Hoarding of high denomination notes for illicit activities
Use of the above for terrorist activities
Rogoff (Citation2017), pointed out that while corrupt activities are largely funded through high denomination notes, a careful phasing out of those notes may be required to keep the economy stable throughout. Krishnan (Citation2019) suggested that demonetisation could be a corrective measure that opens up India to more digital payments and greater transparency in transactions. The sudden cash “crunch” had a wide-reaching effect on all parts of India’s economy, owing to the significant presence of high denomination notes in the money supply. The 500- and 1000-rupee notes accounted for approximately 86% of the total currency in circulation prior to 2016 demonetisation, with only 5% of those notes in government possession (Ministry of Finance, Citation2017).
Prior iterations of demonetisation exist in India’s history—one in 1946 and another in 1978. The 1946 demonetisation saw the 1,000; 5,000- and 10,000-rupee notes demonetised, while the same notes were demonetised again in 1978 under the High Denomination Bank Notes (Demonetisation) Act (Nag, Citation2016). The 2016 demonetisation was different from the previous two in terms of the proportion of high denomination notes in the economy and the sudden implementation. Strict rules and regulations were set in place to track and monitor all exchanges of notes. The Reserve Bank of India utilized a variety of its tools during the implementation process to better control liquidity levels in the economy (Reserve Bank of India, 2016 and Reserve Bank of India, 2017a).
Other nations have also implemented demonetisation. The European Union in 2002 unified nations under the Euro while demonetising national currency, and Zimbabwe in 2015 demonetised their notes to rein in hyperinflation. In the case of Nigeria and Ghana, however, citizens chose to engage in black market activities in order to handle the liquidity crunch they faced rather than wait for demonetisation to take full effect (Borkar, Citation2017).
High quantities of 500- and 1000-rupee notes in circulation meant that cash reliant sectors such as real estate, construction, and textiles would be among many that would be in disarray. Nag (Citation2016) predicted that old currency deposits could benefit banks in that they will obtain surplus liquidity. Ratings agencies reported similar predictions, stating that real estate, construction, jewellery, consumer durables and retail trade would be significantly affected (Sinha, Citation2016). Betala (Citation2017) and Joshi (Citation2017) also supported the predictions, arguing that performance of cement and steel sectors that were already under pressure from lower demand and high raw material prices could worsen.
This paper uses an event study to determine whether demonetisation had an impact on listed firms and their corresponding industries. We also test for changes in systematic risk levels for industries due to the extreme nature of the action and the surprise factor of the announcement (Ramiah et al., Citation2010). The main findings of our paper are as follows: firms in real estate, construction, cement, gems and jewellery, retail trade, consumer goods, agriculture and other manufacturing industries had significant negative abnormal returns after demonetisation. At the industry level, real estate and construction-related industries, some manufacturing industry groups, as well as recreational activity-based industries all showed persistent and negative returns in the days following demonetisation. Moreover, the above industries’ systematic risk increased significantly in the short run.
The desired contributions of our study are as follows. Academic literature on money supply and the resulting liquidity shocks are dominated by data from the US and European economies. Emerging markets like India provide the chance to understand how their stock markets respond to domestic policy shocks. We also hope to add to the empirical literature that examines the impact of shocks and surprises on emerging economies using event study methodology. As demonetisation was a corrective measure on the part of policymakers, we hope to contribute by providing insight on investor response to such actions. Findings relating to abnormal returns and varying levels of systematic risk can generate insight into vulnerabilities across industries and help investors and fund managers hedge their positions quickly. The next section examines relevant literature regarding money supply shocks and asset prices, and how it can apply to demonetisation.
2. Demonetisation
The sudden withdrawal of large denomination notes from circulation created a vacuum of liquidity until newer notes were introduced into the Indian economy. Early studies such as L. Christiano and Eichenbaum (Citation1992); L. J. Christiano & Eichenbaum (Citation1995) set the path for researchers to understand how changes to liquidity affect economic activity. The idea of various “channels” transmitting money supply changes is summarized by Mishkin (Citation1995), who explains that money supply shocks affect the investor allocation of resources, firm asset values and by extension their stock prices through the “asset price channel”. Cook and Hahn (Citation1989), Thorbecke (Citation1997), Booth and Booth (1997), Kurov (2010) and Claessens and Kose (Citation2017) are some empirical papers that found clear evidence of negative returns after money supply was restricted. They argued that investor movement to less risky assets and lower consumer spending can influence firm stock prices.
Strong empirical evidence of money supply changes affecting stock prices can be observed in advanced economies. Results are mixed in the case of emerging markets when it comes to macroeconomic indicators and stock markets, especially during changes in money supply levels. Ndlovu et al. (Citation2018) in their study of the Johannesburg stock market found a positive relationship money supply and stock returns, while Ullah et al. (Citation2017) found an inverse relationship between money supply levels and stock prices across a sample of select SAARC countries. A similar study by Alam (Citation2020) examining select South Asian economies found that inflation rates and GDP had a significant impact on stock returns. However, Nurasyikin et al. (Citation2017) found no link between money supply changes and stock index returns across three ASEAN countries. Chandrashekar (Citation2018) compared the response of Brazil’s and India’s stock prices to a host of macroeconomic variables including inflation and interest rates, and found that there was a significant and positive relationship between stock prices and macroeconomic variables for each of the countries. A recent paper by Thanh et al. (Citation2020) examined how unexpected money shocks affected stock prices in India over 24 years. Tightening policy during bull markets resulted in lower returns for the BSE Sensex.
The sudden announcement of demonetisation and a delay in the distribution of new notes created a shortage in money supply across certain sectors, which is quite similar to several of the conditions described in the above papers. Studies published immediately after demonetisation identified impacts at the economy and industry level. Sharma (Citation2017) found significant impact on aggregate output, with real estate, jewellery and FMCG sectors observing a drop in productivity and employment. Unorganized portions of manufacturing and service sectors were affected, leading to sharp declines in the GVAs of real estate, construction and consumer durables (Chopra, Citation2017) and even luxury housing segments saw downturns in rentals and sales (Verma & Verma, Citation2017). Chodorow-Reich et al. (Citation2018) found that areas that contained larger amounts of old notes suffered sharper declines in economic activity and bank credit, but were much more open towards using digital platforms over time. The assessment that demonetisation affected output at the economy and industry level was also supported by the findings of Yashoda (Citation2017), Kohli and Anand (Citation2017), Chandrasekhar and Ghosh (Citation2017), Bordoloi (Citation2018), Gautam and Jain (Citation2019).Bisen et al. (Citation2017) discovered that perishable agricultural goods faced an initial price hike followed by a drop in demand due to cash shortage. The lack of non-cash payment facilities in rural areas added to losses for farmers in terms of input, labour and consumption (Aggarwal & Narayanan, Citation2017), with supplies also being affected. Dixit et al. (Citation2019) also found evidence of the same in Uttar Pradesh, with transport of crops and seeds, as well as payments being considerably delayed after demonetisation. The adverse impact on the overall economy is well documented by research and government reports as well.
Demonetisation’s impact on the stock market is the focus of our paper. Event studies were used by several researchers in order to understand whether the announcement had an impact on stock markets. Significant impacts on sectoral indices such as real estate, automobiles, consumer goods, FMCG as well as overall market volatility were documented (Dev & Mohapatra, Citation2019; Dharmapala & Khanna, Citation2018; Kumar & Bhatia, Citation2018; Pervez & Khan, Citation2017; Sathyanarayana & Gargesha, Citation2017; Shanmugam & Irshad VK, Citation2017). Parab and Reddy (Citation2020) found that the Nifty 50 index was negatively affected by demonetisation, with Nifty Realty being most affected, while CIIs and FII were not significantly impacted. The announcement effect caused stock prices to decline for over 100 listed firms, especially as the weeks progressed and the disruption spread across the economy. Negative sectoral returns were observed in the case of IT, telecom, utilities and energy (Raza & Munir, Citation2020). However, some studies found evidence of no impact on stock indices due to demonetisation, like Sunil and Shenoy (Citation2017), Chauhan and Kaushik (Citation2017), and Upadhyay and Suvarna (Citation2018). The studies found sectoral impact to be concentrated in cash driven sectors such as real estate, FMCG, automobiles, but their samples used data from precalculated indices. In addition, studies that found no impact on stock indices due to demonetisation had only large capitalization stocks or major indices such as the Sensex or Nifty 50 as their focus. We addressed those issues by examining a larger and broader sample of non-financial firms in the NSE. We also examined not only the sectoral abnormal returns but also the change to the systematic risk levels of these sectors in the short and long run.
The rest of the paper is organized as follows: Section 3 explains the empirical framework used in our paper; Section 4 presents the results of our analysis, and Section 5 concludes the paper.
3. Empirical framework
3.1. Data
This study uses the daily returns of non-financial firm stocks listed in India’s National Stock Exchange, as well as the CNX 500 returns and the 91-day T bill returns. The data on the daily adjusted closing prices, index returns, and NIC codes for firms were obtained from the CMIE Prowess database. The 91-day RBI T bill rates were obtained from the RBI website. As this study uses daily prices of stocks and the stock index, we converted the fortnightly T Bill rates into daily rates.
The initial raw sample of NSE listed firms was 1,942. If missing observations for a firm exceeded 150 days, it was removed from the sample. Therefore, firms that had missing observations in the estimation and event windows were also removed from the sample. Firms that had news announcements in the days leading up to the event date were also removed from the sample, which left 1,054 firms in the final sample. Appendix A provides a count of the firms under each industry division in our final sample. The firm NIC codes were condensed to their two-digit NIC industry divisions. The majority of firms belong to the Manufacturing industry division, with chemical and chemical products (97 firms) and basic metals (67 firms). The next highest group is wholesale trade (except motor vehicles and motorcycles), with 63 firms. Industry divisions with less than 10 firms include human health activities (6 firms), specialized construction activities (4 firms), and several other divisions with only one firm in the sample. Appendix B provides the means, standard deviations, skewness, excess kurtosis and the range of the returns across the industry divisions during the estimation window period. The industry division with the highest average return was Division 77 (Rental and leasing activities) and the lowest was divisions 85 and 56 (education and food and beverage service activities).
3.2. Methodology
Event study methodology is an oft-used technique when testing market efficiency, especially the semi-strong form (Brown and Warner (Citation1980, Citation1985)). MacKinlay (Citation1997) provides an extensive guide to conducting analysis through the event study methodology. The event study methodology measures the impact of an event (announcement, policy measure, or even a natural disaster) on the returns of stocks. Event studies break up the timeline around the event date, and test whether the actual returns during the period of the event were “abnormal”, when compared to the predicted or expected returns.
Inoue et al. (Citation2017) and Hallman (Citation2017) found that rolling window estimations can help generate accurate predictions for time-series data, especially when large breaks occur. Demonetisation’s economy-wide impact and surprise announcement prompted us to employ a rolling window estimation for our event study. As demonetisation was a sudden announcement resulting in a liquidity vacuum in the economy, capturing the impact on stock prices is a challenge. Our analysis makes use of a rolling window to compute the parameters for the estimation window returns (Ramiah et al., Citation2010). Therefore, the data is broken up into three windows—the rolling window, which runs from March 2014 to October 2015 (264 days); the estimation window, which runs from November 2015 to October 2016 (244 days); and the event window, which runs from 1 November 2016 to 18 November 2016, for a total of thirteen trading days.
Several researchers have debated about the length of the window size used in event study analysis. Hillmer and Yu (Citation1979) compared intraday price changes in event and non-event periods to measure the time it took for prices to adjust efficiently. They argued that an event could generate an immediate response from prices, so the event window should capture changes related to only that event, and not any other news that arrives. Krivin et al. (Citation2003) compiled prior research on window sizes in event studies and found that researchers use a fixed window when the sample under observation is large with one or multiple events, as any errors in estimation of one firm’s reaction could be offset by another’s. However, they warned that smaller samples with single events may require a closer examination of the signal to accurately measure changes to stock prices. As demonetisation is an economy wide event, and as the scope to affect majority of the sectors in the Indian economy, we opt for a larger event window of six days before and after the event to set a boundary. However, we focus on the abnormal returns for one day before and after [−1, +1] demonetisation was announced. As the announcement occurred after market hours on 8 November 2016, 9 November 2016 is the first trading day after the announcement, and is therefore chosen as the event date for our analysis. Cumulative abnormal returns are measured over two days and five days after the announcement, in order to check for persistence in abnormal returns for the remaining days in our event window.
Ramiah et al. (Citation2010) used an event study to analyse the impact of various terrorist attacks on Australian industry returns and systematic risk. While demonetisation was certainly no terrorist attack, the element of shock and wide-reaching consequences could potentially affect systematic risk. In order to conduct an event study, the expected returns for the stocks were calculated used the following formula:
The abnormal returns for the event study were estimated according to Brown and Warner (Citation1985). The abnormal returns in the study are calculated as the difference between the actual and expected returns of firm “i” at time “t”. The event study is also a test of market efficiency (Fama, Citation1970), as it tests the ability of the stock prices to incorporate new information into their price levels without delay. The hypothesis tested by the event study is that the abnormal returns generated by new information (or an “event”) is not significantly different from zero.
H0: AR = 0
While Brown and Warner (Citation1985) use, among others, the market model as the base for calculating the estimation period returns, this study uses the excess return CAPM model:
where is the intercept and
is the coefficient, or the market risk premium. The abnormal return for firm i at time t is then calculated as
The returns at the firm level are further aggregated by their two-digit NIC divisions into industry/sector divisions as follows:
where I indicates the industry division and N is the number of firms in that industry division. The modified null hypothesis for firm and industry abnormal returns is
If the abnormal returns of a firm or an industry division post demonetisation is significantly different from zero, it means that the announcement had a significant impact on the firm or industry division. The tests used to measure the statistical significance are the standard t statistic for the abnormal and cumulative abnormal returns:
We also conduct non-parametric tests on the abnormal returns asa robustness check, as in Ramiah et al. (Citation2010). Literature on abnormal returns has indicated that parametric tests rejected the null hypothesis more frequently in the case of positive abnormal returns, and vice versa in the case of negative abnormal returns. Corrado and Truong (Citation2008) discovered that Asia Pacific stock return data requires additional robustness checks during event study analysis. Therefore, we use a non-parametric rank test to ensure robustness of our results (Corrado, Citation1989). First, the abnormal returns of each firm are converted into ranks
for the estimation and event window of 257 days (
):
The ranks of the firms are compared to the average rank under the null hypothesis (no abnormal returns), where the average rank is calculated as follows:
After calculating the ranks for each firm, the final industry level non-parametric statistic is as follows:
, the standard deviation of the average rank, can be calculated as follows:
The second part of our analysis focuses on the effect demonetisation had on the systematic risk of the industry divisions. We use regression analysis to measure the change in systematic risk of industries in the sample. Both the short- and long-term changes to systematic risk are measured. Following Ramiah et al. (Citation2010):
where
—returns of industry group I at time t
—risk free rate at time t
D—dummy variable that takes the value of 1 on the event date and 0 otherwise
The standard CAPM model is supplemented with a dummy variable, in order to test for a change in the short-term systematic risk of the industry group. shows the systematic risk prior to the announcement, and
shows the change in the systematic risk post the announcement. EquationEq. (12
(12)
(12) ), therefore, tests for short term changes to the systematic risk of the industry group. In addition, we also test for any effects on the CAPM intercept for each industry using the following model:
where determines whether or not a significant shift has occurred in the intercept of the industries due to demonetisation.
Next, a regression with a structural dummy variable is estimated to find out if there were any long-term changes to the systematic risk after demonetisation was announced. EquationEquation (14)(14)
(14) measures changes in the long-term systematic risk that resulted from demonetisation where SD is a dummy that takes the value of 1 after the event date and 0 before.
indicates the risk levels prior to demonetisation, and
indicates the change in the long-term systematic risk of the industry after demonetisation.
The next section provides the results of the event study analysis as well as systematic risk.
4. Findings
Our study analyzed the impact of demonetisation on firms listed in the NSE. The first portion of the analysis was an event study, with an event window of six days before and after the announcement. Firm and industry level abnormal returns were examined in the day following the announcement, as well as the subsequent days in the event window. Results showed persistent and negative abnormal returns in several sectors, most of whom were reliant on cash for operations or revenue. The second portion of the analysis examined whether the effect on abnormal returns extended to the systematic risk levels of industries. Regression models were used to examine the short-term and long-term impact on systematic risk due to demonetisation. Our analysis discovered statistically significant impacts on systematic risk of industries due to demonetisation, with several industries experiencing an increase in their risk levels in the short run.
4.1. Abnormal returns
On the day following the demonetisation announcement, our event date 9 November 2016, several firms experienced significant abnormal returns. Seventy-eight firms had negative abnormal returns while 20 firms showed positive abnormal returns (). Firms with significant negative abnormal returns were mainly from the construction and civil engineering divisions, followed by manufacturing and wholesale trade divisions. As for positive abnormal returns, most firms belonged to the manufacturing of pharmaceuticals and power supply divisions. Our findings supported the predictions made by the RBI regarding the specific effect demonetisation would bring to construction and its related sectors. The non-parametric tests support the results of the parametric analysis ().
Table 1 Firm-Level Significant Abnormal Returns on 9 November 2016 This table reports the results of the event study analysis the day after the announcement. The highest and lowest significant abnormal returns are provided below, along with the firm name, industry division (NIC), percentage abnormal returns (AR) and the corresponding t-statistics. Equation (3) is used to calculate abnormal returns of firms with
being the expected returns calculated using the CAPM model, over an estimation window of November 2015 to October 2016
Table 2 Event Study Results by NIC Divisions for 9 November 2016 This table reports the NIC industry division-wise abnormal returns for the day after the announcement (first trading day since the announcement). Each NIC division’s abnormal returns (AR) is calculated as such: , with the firm ARs aggregated by industry. Cumulative abnormal returns (CARs) are calculated by adding up the industry ARs over 2 days (CAR (2)) and 5 days (CAR (5))
Table 3 Non-parametric test results for the event study
An examination of the industry level abnormal returns showed 11 sectors that were adversely affected immediately after demonetisation (). Manufacturing industries relating to apparel and non-metallic products, construction and civil engineering industries, retail trade (except motor vehicles and motorcycles), telecommunications, sports and membership organizations were all adversely affected the day after the announcement. In order to examine whether the abnormal returns were persistent in the days following demonetisation, we examined the cumulative abnormal returns (CARs) for two and five days after demonetisation. We find that the construction of buildings division had abnormal returns of—5.12% (t = −4.97), which supports the results of Betala (Citation2017), Sharma (Citation2017) as well as Pervez and Khan (Citation2017). Three industry divisions that had significant and negative abnormal returns until two and five days after demonetisation were manufacturing of non-metallic and mineral products (Division 23), construction of buildings (Division 41), which confirms the findings of both Sinha (Citation2016) and Joshi (Citation2017) who both predicted an extreme response from the construction and real estate sectors, as well as sports activities and amusement and recreation activities (Division 93). The full results for industry level impacts of demonetisation are reported in .
4.2. Systematic risk
Our second set of analyses sought to understand whether demonetisation significantly altered the systematic risk of the industries in our sample. We found that the short-term systematic risk of industries was significantly affected by demonetisation in the short run. Of the 22 industry divisions that had their systematic risk affected due to demonetisation, 12 sectors showed an increase in their short-term systematic risk levels (, columns 3 and 4). For instance, Division 1’s systematic risk before demonetisation was 1.352 (t = 17.77), but it rose to 1.692 (t = 2.40) after demonetisation. In addition, six of the sectors that experienced an increase in systematic risk also had significant and negative abnormal returns immediately after demonetisation ().
Table 4. Regression analysis: Short-term systematic risk by NIC division: This table presents the estimation results of for the following model: Each industry level returns at a given time are examined against the market risk premium (
, as well as the premium interacted with a dummy variable that takes the value of 1 on the event date (9 November 2016) and 0 otherwise (
.
shows the systematic risk prior to the announcement, and shows the change in the systematic risk post the announcement. Robust t-statistics are provided in parentheses below the coefficients
Figure 2. This graph shows a comparison of pre and post demonetisation systematic risk levels in the short run. Notes: SysRisk_Before is the systematic risk of the given NIC industry division prior to demonetisation being announced, and SysRisk_After is the systematic risk of the given NIC industry division after demonetisation. Only NIC divisions that showed a statistically significant change in systematic risk are displayed here

When examining whether or not the shock from demonetisation affected the systematic risk in the long run, we first studied its impact on the intercept of the CAPM (). Several industries exhibited a downward shift in their intercept, suggesting that demonetisation announcement could have had a longer-term impact on stock returns of industries. Once again, we observed the same group of industries in amongst those with a lower intercept after demonetisation. (columns 3 and 4) provides the results of the long-term impact on systematic risk. The industries in our sample experienced a significant change in their levels of systematic risk in the long run. However, when compared to their pre-demonetisation risk levels, only one industry division (Division 16—Manufacture of wood and products of wood and cork, except furniture; manufacture of articles of straw and plaiting materials) experienced an increase in its long-term systematic risk.
Table 5 Regression analysis: CAPM Intercept: This table provides the results of the test of the CAPM intercept, estimated using the model:
determines whether a statistically significant shift in the industry’s intercept has occurred after demonetisation was announced. Robust t-statistics are provided in parentheses
Table 6 Regression analysis: Long-term systematic risk: This table provides the results from estimation of the model: . The long-term changes to systematic risk are measured using
which is interacted with SD, a structural dummy variable that takes the value of 1 after the event date, and 0 otherwise.
represents the systematic risk prior to demonetisation, and
represents the change in the systematic risk level after demonetisation. Robust t-statistics are provided in parentheses
Overall, our sample showed strongly negative reactions to demonetisation, through lower abnormal returns at firm and industry levels and significant alteration in systematic risk at the industry level. The adverse impact that demonetisation had on real estate and construction-related industries, as well as cash driven industries is consistent across our findings. Division 23, manufacturing of non-metallic and mineral products, contains firms that deal with the production of concrete, cement, plaster and lime, which are raw materials used for construction-related activities. Division 41, the construction of buildings, constituted firms that handled contractual or fee-based construction, maintenance and assembly of prefabricated constructions. This persistent and focused impact of our findings echoes the conclusions made by Dharmapala and Khanna (Citation2018) and Raza and Munir (Citation2020). However, our findings contradict those of Upadhyay and Suvarna (Citation2018), as well as Sunil and Shenoy (Citation2017), who found no stock market impact due to demonetisation. With a broader sample of firms, and industry grouping using a different set of criteria, our results showed significant impact on stock prices and systematic risk of listed firms.
Interestingly, Division 93, which contains sports clubs, amusement parks, sports events and related operations, also performed poorly after demonetisation. Other industries such as retail trade, telecommunications, textiles/wearing apparel are all known to involve significant amounts of labour, which could mean cash payments for employees and cash-based revenue. As a cash shortage gripped the economy following demonetisation, it could be possible that consumer spending on events and recreational activities declined or were postponed.
5. Conclusion
The surprise announcement demonetizing the 500- and 1000-rupee notes created a sudden shortage of cash across India. Our paper focused on testing for the presence of abnormal returns as well as any changes in the systematic risk of stocks since the demonetisation announcement. Our sample consisted of 1,054 firms across 57 NIC divisions. Event study results showed a strong negative response from construction and real estate industry divisions, as well as retail trade, jewellery and agriculture. Further analysis of systematic risk complemented the results of the event study, with the same industry divisions experiencing a short-run increase in their systematic risk. The above findings confirmed that of prior literature, as well as initial reports by RBI and the Economic Survey, that demonetisation had an adverse effect on major portions of the economy, including specific sectors and listed firms. Our study also contradicted the findings of some studies which do not find any significant stock market impact of demonetisation. To the best of our knowledge, an analysis using such a large sample of listed firms, as well as exploring their systematic risk has not yet been addressed by existing studies.
To sum up, we find that demonetisation had a significant and negative impact on the stock prices of several firms and sectors. The sectors in question experienced an increase in their systematic risk levels in the short run, signaling a structural break in their stock prices due to demonetisation and its associated money supply shortage. While a significant impact on short-run systematic risk was observed for several sectors with negative abnormal returns, long-run impact was not evidenced. However, as the deadline for notes exchange and declaration is 30 December 2016, it is very much possible that money supply levels continued to fluctuate for a period of time outside our analysis window. In addition, the delay in the distribution of new notes also increased the liquidity vacuum for consumers and businesses. It could be possible that stock returns of certain sectors may be affected after some time, especially around earnings period following demonetisation. Even though the immediate response of several of the cash driven sectors is negative, we expect that decline in spending by consumers could affect other industries as well, for the next half yearly period. For example, services sector, especially those that involve hospitality, leisure, etc. could experience a decline in the medium term.
With an evolving financial system, the relationship between monetary shocks and asset prices is of increasing importance. The strong response of the stocks in our sample to demonetisation indicates a significant presence of the asset price channel of transmission, helping add to the empirical literature which examines monetary policy transmission. Our results also contribute to empirical research that uses event studies to scrutinize the impact of shocks on financial markets, especially those in the Asia Pacific, by investigating a unique corrective action that will have long-term consequences to a major Asian economy. Also, we were able to identify industry divisions that were significantly impacted by this action, which could assist policymakers in keeping track of vulnerabilities in the case of future surprise actions. In addition, investors and fund managers can adjust their market positions and portfolios with this knowledge about industry characteristics and their response to policy shocks. Future research could examine each of the sectors more deeply to understand the sources and magnitude of heterogeneity among industries, especially in the case of policy surprises.
Acknowledgements
We are immensely grateful to Dr Sanjay Ramchander of McCoy College of Business Administration, Texas State University for his valuable support and suggestions while writing this manuscript. We would also like to thank the reviewers and journal editors for their supportive and critical suggestions for improving our manuscript.
Additional information
Funding
Notes on contributors

Varsha Sureshkumar
Varsha Sureshkumar is a PhD Scholar with Amrita School of Business, Amrita Vishwa Vidyapeetham, Coimbatore. Her broad research interests include financial economics, asset pricing, econometrics and market microstructure. Her current focus is on understanding how economic policies and financial systems interact when there are surprises or shocks. She is currently exploring the financial system impacts of demonetisation in India. She hopes to better understand how financial systems evolve and respond to surprises and crisis events.
Dr Balasubramanian P. is Professor at Amrita School of Business, Amrita Vishwa Vidyapeetham, Coimbatore. He completed his PhD dissertation from Jawaharlal Nehru University. He has fifteen years’ experience in teaching and research. He teaches topics in economics, financial derivatives, financial modelling and valuation, as well as behavioural economics for PhD students. His research focuses include event studies in financial markets and behavioural biases in finance.
References
- Aggarwal, N., & Narayanan, S. (2017), “Impact of India’s demonetization on domestic agricultural markets”, Working paper, SSRN, Available at: https://doi.org/10.2139/ssrn.3066042
- Alam, Q. N. (2020). Impacts of macroeconomic variables on the stock market returns of South Asian Region. Canadian Journal of Business and Information Studies, 2(2), 24–30. https://doi.org/10.34104/cjbis.020.24034
- Betala, V. (2017), “Market wire: Large corporates pull through from cash shortage, demonetisation impact credit neutral”, India Ratings & Research. available at: https://www.indiaratings.co.in/PressRelease?pressReleaseID=26320&title=Market-Wire%3A-Large-Corporates-Pull-Through-from-Cash-Shortage%2C-Demonetisation-Impact-Credit-Neutral-
- Bisen, J., Kumar, S., Venkatesh, P., & Aditya, K. S. (2017). Impact of demonetization on agriculture: A case study. Indian Journal of Economics and Development, 5(2),1-11. www.iseeadyar.org
- Booth, J. R., & Booth, L. C. (1997). Economic factors, monetary policy, and expected returns on stocks and bonds. FRBSF Economic Review, 2, 32–42. https://search.proquest.com/scholarly-journals/economic-factors-monetary-policy-expected-returns/docview/208781674/se-2?accountid=38661
- Bordoloi, J. (2018). Impact of Demonetisation: Net effect. International Journal of Research Culture Society, 2(1), 6–10. https://ijrcs.org/wp-content/uploads/201801002.pdf
- Borkar, N. (2017). Here’s a list of countries that have tried demonetisation before India. Indiatimes, 23, 6. https://www.indiatimes.com/news/world/here-s-a-list-of-countries-that-have-tried-demonetisation-before-india-265743.html
- Brown, S. J., & Warner, J. B. (1980). Measuring security price performance. Journal of Financial Economics, 8(3), 205–258. https://doi.org/10.1016/0304-405X(80)90002-1
- Brown, S. J., & Warner, J. B. (1985). Using daily stock returns. Journal of Financial Economics, 14(1), 3–31. https://doi.org/10.1016/0304-405X(85)90042-X
- Chandrasekhar, C. P., & Ghosh, J. (2017), “What is really happening in Indian manufacturing?”,The Hindu Business Line, available at: https://www.thehindubusinessline.com/opinion/columns/c-p-chandrasekhar/what-is-really-happening-in-indian-manufacturing/article9818148.ece
- Chandrashekar, R. (2018). Macroeconomic variables and stock prices in emerging economies: A panel analysis. Theoretical and Applied Economics, 26(3), 91–100.
- Chauhan, S., & Kaushik, N. (2017). Impact of Demonetization on stock market: Event study methodology. Indian Journal of Accounting, 49(1), 127–132. https://doi.org/10.26808/rs.rmf.v2i1.01
- Chodorow-Reich, G., Gopinath, G., Mishra, P., & Narayanan, A. (2018), “Cash and the economy: Evidence from India’s Demonetization”, NBER Working Paper Series No. 25370, National Bureau of Economic Research.
- Chopra, R. (2017). Impact of Demonetization on Indian economy. Global Journal of Enterprise Information System, 9(2), 100–103. https://doi.org/10.18311/gjeis/2017/15857
- Christiano, L., & Eichenbaum, M. (1992), “Liquidity effects and the monetary transmission mechanism”, Working Paper, No. 4129, National Bureau of Economic Research, Minneapolis.
- Christiano, L. J., & Eichenbaum, M. (1995). Liquidity effects, monetary policy, and the business cycle. Journal of Money, Credit and Banking, 27(4), 1113–1136. https://doi.org/10.2307/2077793
- Claessens, S., & Kose, M. A. (2017). Asset prices and macroeconomic outcomes: A survey Working No. 1718. Koç University-TÜSİAD Economic Research Forum (ERF).
- Cook, T., & Hahn, T. (1989). The effect of changes in the federal funds rate target on market interest rates in the 1970s. Journal of Monetary Economics, 24(3), 331–351. https://doi.org/10.1016/0304-3932(89)90025-1
- Corrado, C. J. (1989). A nonparametric test for abnormal security-price performance in event studies. Journal of Financial Economics, 23(2), 385–395. https://doi.org/10.1016/0304-405X(89)90064-0
- Corrado, C. J., & Truong, C. (2008). Conducting event studies with Asia-Pacific security market data. Pacific-Basin Finance Journal, 16(5), 493–521. https://doi.org/10.1016/j.pacfin.2007.10.005
- Dev, S., & Mohapatra, P. (2019). Impact of Demonetization on the Indian stock market. The IUP Journal of Applied Economics, 18(4), 72–88. https://doi.org/10.1177/0971890718788226
- Dharmapala, D., & Khanna, V. S., (2018), “Stock Market Reactions to India’s 2016 Demonetization”, U of Michigan Law & Econ SSRN Research Paper No. 17-010, Available at: https://ssrn.com/abstract=2983965
- Dixit, P., Al-kake, F., & Ahmed, R. R. (2019). A critical review on short term impact of demonetisation on inclusive growth of India. Russian Journal of Agricultural and Socio-Economic Sciences, 90(6), 161–167. https://doi.org/10.18551/rjoas.2019-06.22
- Fama, E. F. (1970). Efficient capital markets: a review of theory and empirical work. The Journal of Finance, 25(2), 383–417. https://doi.org/10.2307/2325486
- Gautam, I., & Jain, S. (2019). Demonetization in India and Its Aftermath. Pragyana - International Journal of Multidisciplinary Research, 1(1), 61–66. http://himadritrust.in/pragyana/wp-content/uploads/2018/12/Demonetization-in-India-and-its-Aftermath.pdf
- Government of India, Ministry of Finance, Department of Economic Affairs (2016). “Notification of Demonetisation” [Press Release].
- Hallman, L. (2017). The Rolling Window Method: Precisions of Financial Forecasting. [Degree Project in Mathematics, KTH Royal Institute of Technology School of Engineering].
- Hillmer, S. C., & Yu, P. L. (1979). The market speed of adjustment to new information. Journal of Financial Economics, 7(4), 321–345. https://doi.org/10.1016/0304-405X(79)90002-3
- Inoue, A., Jin, L., & Rossi, B. (2017). Rolling window selection for out-of-sample forecasting with time-varying parameters. Journal of Econometrics, 196(1), 55–67. https://doi.org/10.1016/j.jeconom.2016.03.006
- Joshi, A. (2017), “Market Wire: Demonetisation to Derail Cement Sector Growth”, India Ratings & Research, available at: https://www.indiaratings.co.in/PressRelease?pressReleaseID=26061&title=Market-Wire%3A-Demonetisation-to-Derail-Cement-Sector-Growth
- Kohli, B., & Anand, M. (2017). Assessment of the impact of demonetization on Indian economy. International Journal of Research in Commerce & Management, 8(5), 44–47. https://ijrcm.org.in/article_info.php?article_id=7599
- Krishnan, A. (2019). “Demonetization Anniversary: Decoding the Effects of Indian Currency Notes Ban”, The Economic Times, May 21, pp. 2. Available at: https://economictimes.indiatimes.com/tdmc/your-money/demonetization-anniversary-decoding-the-effects-of-indian-currency-notes-ban/articleshow/61579118.cms?from=mdr
- Krivin, D., Patton, R., Rose, E., & Tabak, D. (2003). Determination of the Appropriate Event Window Length in Individual Stock Event Studies. SSRN Electronic Journal, 25. https://doi.org/10.2139/ssrn.466161
- Kumar, V., & Bhatia, B. S. (2018). The effect of demonetisation in Indian stock market with special reference to Sectorial Indices: An Event Study. SSRN Electronic Journal, 1–18. http://doi.org/10.2139/ssrn.3245889
- Kurov, A. (2010). Investor sentiment and the stock market’s reaction to monetary policy. Journal of Banking & Finance, 34(1), 139–149. https://doi.org/10.1016/j.jbankfin.2009.07.010
- MacKinlay, A. C. (1997). Event studies in economics and finance. Journal of Economic Literature, 35(March), 13–39. http://www.jstor.org/stable/2729691
- Ministry of Finance (2017), “Demonetisation: To Deify or Demonize?” Economic Survey, 2016-17.
- Mishkin, F. S. (1995). Symposium on the monetary transmission mechanism. Journal of Economic Perspectives, 9(4), 3–10. https://doi.org/10.1257/jep.9.4.3
- Nag, A. (2016). Lost Due to Demonetisation. Economic & Political Weekly, 51(48), 18–21. https://www.epw.in/system/files/pdf/2016_51/48/Lost_Due_To_Demonetisation_0.pdf
- Ndlovu, B., Faisa, F., Resatoglu, G., & Tursoy, T. (2018). The impact of macroeconomic variables on stock returns: A case of the Johannesburg stock exchange. Romanian Statistical Review, 6(2), 87–104. https://www.researchgate.net/profile/Turgut-Tursoy/publication/325877382_The_Impact_Macroeconomic_Variables_on_Stock_Returns_A_Case_of_the_Johannesburg_Stock_Exchange/links/5b2a5feb4585150c63410677/The-Impact-Macroeconomic-Variables-on-Stock-Returns-A-Case-of-the-Johannesburg-Stock-Exchange.pdf
- Nurasyikin, J., Shahnaz, I., & Syamimi, A. M. (2017). Macroeconomic variables and stock market returns: Panel analysis from selected ASEAN countries. International Journal of Economics and Financial Issues, 7(1), 37–45. http:%0Awww.econjournals.com
- Parab, N., & Reddy, Y. V. (2020). A cause and effect relationship between FIIs, DIIs and stock market returns in India: Pre- and post-demonetization analysis. Future Business Journal, 6(1), 10. https://doi.org/10.1186/s43093-020-00029-6
- Pervez, A., & Khan, A. M. (2017). How the demonetization impacted stock indices in India. International Journal of Research in Commerce & Management, 8(10), 90–92. https://ijrcm.org.in/article_info.php?article_id=8075
- Ramiah, V., Cam, M.-A., Calabro, M., Maher, D., & Ghafouri, S. (2010). Changes in equity returns and volatility across different Australian industries following the recent terrorist attacks. Pacific-Basin Finance Journal, 18(1), 64–76. https://doi.org/10.1016/j.pacfin.2009.07.001
- Raza, S., & Munir, S. (2020). Did demonetization affects Indian stock market? A short-term analysis. SSRN Electronic Journal, 1–19. https://doi.org/10.2139/ssrn.3522052
- Reserve Bank of India. (2016), “Reserve Bank of India Annual Report 2015-16”.
- Reserve Bank of India (2017a), “Macroeconomic Impact of Demonetisation- A Preliminary Assessment”, Reserve Bank of India Occasional Papers, Available at: https://doi.org/10.2478/v10117-012-0018-4
- Rogoff, K. S. (2017). The Curse of Cash. Princeton University Press.
- Sathyanarayana, S., & Gargesha, S. (2017). “The Impact of Policy Announcement on Stock Market Volatility: Evidence from Currency Demonetisation in India”. IOSR Journal of Business and Management, 19(1), 47–63. https://doi.org/10.9790/487X-1901074763
- Shanmugam, V., & Irshad VK, M. (2017), “An Event Study on the Impact of RBI’s Monetary Policy Announcements and Demonetization on the NSE Bond Indices”, SSRN. Available at: https://doi.org/10.2139/ssrn.3059514
- Sharma, J. (2017). Demonetization in India - A study of intent, agenda, and impact on Indian economy. Pranjana:The Journal of Management Awareness, 20(1), 39–47. https://doi.org/10.5958/0974-0945.2017.00004.8
- Sinha, S. K. (2016), “Market Wire: Black Day for Black Money – Structural Benefits of Demonetisation Outweigh Short Term Agony”, India Ratings & Research. Available at: https://indiaratings.co.in/PressRelease?pressReleaseID=25042&title=Market-Wire%3A-Black-Day-for-Black-Money-%E2%80%93-Structural-Benefits-of-Demonetisation-Outweigh-Short-Term-Agony
- Sunil, T., & Shenoy, S. V. (2017). Impact of demonetization on stocks of selected sectors – an event study. International Journal of Research in Finance and Marketing (IJRFM), 7(5), 29–38.
- Thanh, S. D., Canh, N. P., & Maiti, M. (2020). Asymmetric effects of unanticipated monetary shocks on stock prices: Emerging market evidence. Economic Analysis and Policy, 65(November), 40–55. https://doi.org/10.1016/j.eap.2019.11.005
- Thorbecke, W. (1997). On stock market returns and monetary policy. The Journal of Finance, 52(2), 635–654. https://doi.org/10.1111/j.1540-6261.1997.tb04816.x
- Ullah, G. M. W., Islam, A., Alam, M. S., & Khan, M. K. (2017). Effect of macroeconomic variables on stock market performance of SAARC countries. Asian Economic and Financial Review, 7(8), 770–779. https://doi.org/10.18488/journal.aefr.2017.78.770.779
- Upadhyay, D., & Suvarna, S. W. (2018), “Impact of Demonetization on the Indian Stock Market”, Paradigm, Available at: https://doi.org/10.1177/0971890718788226
- Verma, N., & Verma, T. L. (2017), “Demonetization and Possibility to Transform Real Estate Business in India”, Working paper, SSRN, Available at: https://ssrn.com/abstract=3012407.
- Yashoda (2017). Demonetization and its impact. International Journal of Research in Commerce & Management, 8(8), 65–67. https://ijrcm.org.in/article_info.php?article_id=7898