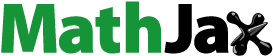
Abstract
The study examines the efficiency differences across the ownership structure of Indian microfinance institutions (MFIs) operating during the year 2005/06 to 2017/18 in response to regulatory reforms initiated by the Reserve Bank of India (RBI) in the year 2011. We remove the outliers from the dataset first. Thereafter, we employ the bootstrap data envelopment analysis (DEA) to assess the bias-corrected efficiency scores. To identify the performance determinants, we use bootstrap truncated regression. The empirical results suggest that the performance difference between NBFCs and Non-NBFC MFIs is not statistically significant in the sample period. Further, the study finds that the size and ownership structure of MFI has a positive and statistically significant impact on the efficiency level. Although the coefficient of PAR30 (Portfolio at risk, 30 days) is statistically insignificant, however, the results conclude that the deteriorating credit quality has hindered the efficiency level. The Indian MFI industry needs to focus on the adoption of more innovative technology and partnership with FinTech (financial technology) firms to reduce the transaction costs and service time. The RBI essentially endorses the regulatory-sandbox practices to offer micro-financial services to the poor.
PUBLIC INTEREST STATEMENT
The microfinance institutions (MFIs) play significant roles in empowering the poor and specifically women. The MFIs offer small credits, micro-insurance, remittance services, skill development programs to uplift the poor and make them capable to fight against poverty. In addition, in many developing countries like India, the MFIs are playing an incredible role in improving the quality of lives in the rural as well as urban slums. Therefore, the assessment of MFIs performance and identifying the factor determining their efficiency level is required. The existing study will provide inputs to MFIs management and policymakers in order to re-design the policy and strategy to further improve the efficiency level which will benefit all the stakeholder of microfinance.
1. Introduction
During the recent decades, Indian microfinance has gained international recognition with the claims that it is one of the significant devices for poverty reduction, women empowerment, household security and microenterprise development (Kaur, Citation2016; N. Kumar & Sensarma, Citation2017; Ambarkhane et al., Citation2019). Till the year 2005, the rapid growth of the MFI Industry in India was dependent on borrowed funds and government aids, etc. In addition, the ownership structure as adopted by most of MFIs were NGOs, Cooperatives, Societies and Trust which were not legally permitted to collect deposits from the clients (MFIN, Citation2018). Therefore, they were depending on outside funds, e.g., commercial banks, domestic financial institutions and foreign donors. Additionally, during the year 2005 to 2010, the MFIs transformed from NGOs to shareholder-owned MFIs and pursue growth driven by the increasing assets size and loan portfolio size (Sa-Dhan, Citation2016). In October 2010, the microfinance crisis erupted in Andhra Pradesh, which is known as the biggest crisis in microfinance history (Mader, Citation2013). This was mainly due to the inadequate regulation, pressure on MFIs to earn profits, over-indebtedness of borrowers and the coercive collection methods of leads to a high number of registered suicides by MFIs clients (Mader, Citation2013; Quidt et al., Citation2012; Yadav & Wongsurawat, Citation2018). This unfortunate event triggered the need to incorporate the regulatory framework immediately, to govern the Indian microfinance sector. Therefore, based on the recommendation of Malegam committee report 2011, the Reserve Bank of India (RBI) came with the new regulatory norms in the industry. The industry shifted to a new horizon and introduced a separate category for NBFC-MFIs which fully transformed the ownership structure of the Indian MFI industry (PwC, Citation2019). In the bank efficiency literature, plenty of studies have established the relationship between regulation, ownership structure and efficiency of Banks (See, for instance, Barth et al., Citation1997; Ahmad and Hassan, Citation2007; Shen & Chang, Citation2006; Pasiouras et al., Citation2009; Lin & Zhang, Citation2009; Kumar and Gulati; 2014; Boateng et al., Citation2015; Psillaki & Mamatzakis, Citation2017;). However, MFIs literature reports mixed results about the link between regulation, ownership structure and performance of MFIs (Ghose et al., Citation2018; Hartarska & Nadolnyak, Citation2007; Pati, Citation2012; Servin et al., Citation2012). Moreover, no study exists which established the association between ownership structure and efficiency of Indian MFIs in the light of regulatory reform initiated by the RBI in the Indian MFI industry after the year 2011. In addition, the MFIs need to be financially sustainable to serve the poor clients in the long run (N. Kumar & Sensarma, Citation2017). Therefore, assessing the efficiency of Indian MFIs across the distinct ownership structure will be useful to the practitioner of microfinance. The developed and efficient MFI industry will serve a large number of the poor section of society and empower the women by providing microcredit which ultimately assists the government financial inclusion and social development.
The existing study focuses on testing the following hypotheses:
1. Whether Indian MFIs show a rising trend in the efficiency level.
2. Whether ownership structure/Legal status affects the MFIs performance
3. Whether the regulatory framework designed by the RBI influence the MFIs performance
Against this backdrop, primarily, the present study aims to examine the trend in the efficiency of the Indian MFIs industry for the period 2005/06 to 2017/18. In particular, we employ the bootstrap DEA model to assess the bias-corrected efficiency estimates of individual MFIs. Besides, we investigate the performance difference of different ownership types of MFIs during the entire period and sub-periods as well. After the regulatory changes, the microfinance sector is dominated by NBFC-MFIs. The MFIs earlier registered in other legal form have also transformed into NBFC-MFIs to access the capital market and to gain the confidence of other stakeholders. Therefore, we divide all sample MFIs into two categories NBFC and Non-MBFC-MFIs to perform the analysis. Further, the study is extended to explore the efficiency determinants; we employ the bootstrap truncated regression to identify the factors which determine the performance of Indian MFIs.
The present study contributes to enriching the literature on MFI efficiency. Our study is dissimilar from the existing empirical literature of MFI efficiency in several parts. First, to the best of the author’s knowledge, almost all the studies have examined the efficiency by employing the traditional DEA model (except Wijesiri, 215; Bibi et al., Citation2018; Khan & Gulati, Citation2019; Khan & Shireen, Citation2020) which do not account the biasness in the efficiency estimates (Simar & Wilson, Citation2000). We assess the bias-corrected efficiency scores of individual MFIs for all the years by deploying the bootstrap DEA model which offers authentic estimates. Second, we explore the performance differences of the MFIs during the regulatory changes. Third, we explore the performance difference of MFIs with different ownership types and their separate strategy response to the regulatory change in the sub-periods. Fourth, to identify the factors determining the efficiency, we employ the bootstrap truncated regression.
The rest of this paper unfolds as follows. Section 2 briefly discusses relevant literature review. Section 3 describes the methodological framework and briefs about data and inputs-outputs used. Empirical results are discussed in section 4. Section 5 concludes the study with policy implications and direction for future research.
2. Relevant literature review
The objective of this section is to explore the empirical studies which seek to establish the linkages between, ownership structure, size, legal status and efficiency of the Indian MFI industry. The majority of studies in the literature have assessed the efficiency and productivity of MFIs using frontier methods (see, Fall et al., Citation2018, Gutiérrez-Nieto & Serrano-Cinca, Citation2019; Li et al., Citation2019). Hermes and Hudon (Citation2018) review 170 studies which identifies the determinants of financial and social efficiency of MFIs and found the MFI characteristics such as size, age and type of organization, source of funding, governance, macro-economic, institutional and political conditions may affect the MFIs financial and social performance.
We find the majority of researchers analyse the regulatory development in the microfinance sector in the different countries (see, for instance, Cull et al., Citation2011; Haq et al., Citation2008; Hartarska & Nadolnyak, Citation2007; Hoxhaj, Citation2010; Jackson & Islam, Citation2005; McGuire, Citation1999; Ndambu, Citation2011; Pati, Citation2012; Purkayastha et al., Citation2014; Ranjani, Citation2012; Trujillo et al., Citation2014; Trujillo-Tejada et al., Citation2015; Yadav & Wongsurawat, Citation2018). Liñares-Zegarra and Wilson (Citation2018) examine the association between size and growth of the MFIs across the different ownership structure and confirm that growth rate varies across the different ownership form of MFIs. Abdelkader and Mansouri (Citation2019) observe that the efficiency level of MFIs varies across the different regulatory environment in the Middle East and North Africa (MENA) region. Recently, Khan and Shireen (Citation2020) examine the drivers of financial and operational efficiency of MFIs operating in the Eastern Europe and Central Asia (ECA) region by using a bootstrap DEA approach.
In the Indian context, most of the existing studies have only theoretically examined the role of the MFIs and their challenges, operating models, and regulatory issues of the microfinance industry (See, for instance, Basu & Srivastava, Citation2005; Dasgupta, Citation2005; Field et al., Citation2013; Khandelwal, Citation2007; Nair, Citation2001; Ray & Mahapatra, Citation2016; Yadav & Wongsurawat, Citation2018). A few of the researchers have tried to investigate the root causes of the crisis in Indian microfinance and government actions (Bayar, Citation2013; Constantinou & Ashta, Citation2011; Dowling, Citation2011; Ghate, Citation2007; Hudon & Sandberg, Citation2013; Mader, Citation2013; Nadiya et al., Citation2012; Palmer, Citation2013; Priyadarshee & Ghalib, Citation2011; Quidt et al., Citation2012; Wichterich, Citation2012).
A very few authors (See, for instance, Masood & Ahmad, Citation2012; Muneer & Kulshreshtha, Citation2014; Muneer, Citation2016; Kaur, Citation2016; N. Kumar & Sensarma, Citation2017; Khan & Gulati, Citation2019; Ambarkahe et al., Citation2019; Singh and Pandey, Citation2019) assess the empirical performance of MFIs operating in India. However, all of them have employed the conventional frontier methods to examine efficiency and Productivity (except a few, i.e., Khan & Gulati, Citation2019; Singh and Pandey, Citation2019).
Only a few studies have examined the association between regulation, competition and performance of Indian MFIs. In particular, Pati (Citation2012) scrutinizes the impact of regulation on the performances of MFIs operating in India. The author has taken operational self–sustainability (OSS) and return on assets (ROA) as a proxy to measure the sustainability and profitability of MFIs. The author found that regulation is not statistically significant to affect the sustainability and profitability of MFIs. However, regulated MFIs have access to capital and have well-established system and process. They further conclude that regulation in Indian MFIs is getting stronger day-by-day. Ranjani (Citation2012) has analysed the regulatory framework for Indian MFIs with reference to international experiences. The author claims that the absence of regulation is one of the factors responsible for the recent microfinance crisis of Andhra Pradesh in the year 2010. Recently, Purkayastha et al. (Citation2014) examine the impact of competition and regulation on the performance of Indian MFIs operating during the year 2008/09 to 2012/13 and conclude that the level of competition positively associated with the MFIs outreach and operating efficiency however deteriorates the credit quality and profitability.
3. Methodological framework
3.1. Output-oriented CCR-based DEA model
The study deploys an output-oriented constant-returns-to-scale DEA model as suggested by Charnes et al. (Citation1978) to calculate the original efficiency scores of individual MFI. In the next step, the study uses the bootstrap procedure in the DEA framework to obtain the bias-rectified efficiency scores. Let us say, we have and each MFIs yield s outputs (
) by deploying m inputs (
). By solving the following linear programming problem, we can measure the efficiency estimate of particular MFI “o”:
Here stands for original efficiency scores of the MFI “o” and
represents the proportion by which MFI “o” can augment its outputs at the provided level of input resources, in order to become efficient.
represents the share of MFI j in defining an efficient target for the MFI “o”. If
= unity, the MFI is said to be fully efficient, if
, the MFI is relatively inefficient.
The classical DEA models do not account for the bias; therefore, one may obtain efficiency scores propelled by the bias and mislead the researcher (Khan & Gulati, Citation2019; Khan & Shireen, Citation2020; Wijesiri et al., Citation2015). Therefore, in order to obtain a reliable and true efficiency frontier, the study integrates the bootstrap technique in the DEA structure with N = 2000 bootstrap replication as instructed by Simar and Wilson (Citation2007). This is how we yield the bias-adjusted efficiency scores of individual MFIs by separating the bias from original efficiency estimates. Interested researchers may visit Simar and Wilson (Citation2007) Bogetoft and Otto (211) for a detailed explanation about bootstrap procedure and algorithms.
3.2. Bootstrap truncated regression (Simar & Wilson, Citation2007)
The efficiency estimates which ranges from zero to unity are serially correlated, subsequently, may mislead the researchers in the post-DEA analysis while identifying the factors responsible to determine them. Besides, the widely preferred Tobit, ordinary least-square (OLS) regression models were also condemned by Simar and Wilson (Citation2007). The authors argue that efficiency scores obtained from the conventional DEA model may offer an inconsistent and biased estimate in the second-stage of analysis. In order to overcome such limitations, Simar and Wilson (Citation2007) recommend the bootstrapped truncated regression which provides valid and reliable results so that a true inference may be drawn for policy formulation and articulating the optimum business strategies. Therefore, by following Wijesiri et al. (Citation2015), the study deploys the bootstrap truncated regression model as suggested by Simar and Wilson (Citation2007) to detect the factors influencing the efficiency level of Indian MFIs. Following is the regression model used in the study with the selected set of environmental variables;
where stands for efficiency of ith MFI at time t,
is the intercept and
are the parameters to be estimated and
is the error term. The log of total assets i.e., SIZE is taken as a proxy to measure the impact of scale of operation on the MFIs efficiency level. Additionally, the most favoured proxy portfolio at risk greater than 30 days (PAR30) has been incorporated in the model as a proxy to assess the impact of credit quality on MFIs’ efficiency level. Besides, the equity to total assets (EQTA) is deployed as a proxy to evaluate the degree of capitalization of MFIs. The EQTA specifies the stability of the MFIs, if the EQTA is higher, the MFI is said to be stable or vice-versa. Moreover, the study uses three dummy variables: REFORMS, OWNERSHIP, and AGE, in order to capture the effect of new regulatory reforms, ownership and experience of MFI, respectively. describes the definition and expected signs of discussed environmental variables used in the study. It is worth mentioning here that the study uses 2000 bootstrap iterations to estimate the optimum parameters in line with Simar and Wilson (Citation2000). The authors who are keen to know more details about bootstrap truncated regression procedure are referred to visit Simar and Wilson (Citation2007).
Table 1. Description of environmental variables
3.3. Input-output specifications and database
Broadly, the efficiency literature uses two approaches; first, the production approach (Benston, Citation1965) and second, the intermediation approach (Sealey & Lindley, Citation1997) to select an input-output combination to incorporate in the DEA framework. Moreover, MFIs have multi-objectives and usually, MFIs do not accept deposits, particularly in India. Therefore, none of the two approaches is suitable in the case of MFIs (N. Kumar & Sensarma, Citation2017). It is worth mentioning here that the study keeps in view the dual objectives of MFIs (i.e., financial and social) while selecting the output combination in line with Wijesiri et al. (Citation2015). Since the MFIs have dual goals to achieve, therefore, performance of them must be assessed on basis of dual perspectives; financial and social (Piot-Lepetit & Nzongang, Citation2014; Wijesiri et al., Citation2015; Jaiyeoba et al., Citation2018). Therefore, the study combines financial and social proxies to account for financial sustainability (i.e., financial revenue, gross loan portfolio) and social outreach (i.e., number of borrowers). The description of inputs—outputs used in the analysis and represented in .
Table 2. Description of input-output used in DEA framework
The data of selected inputs and outputs variables for and environmental variables are extracted from the Mix Market dataset of the World Bank (https://databank.worldbank.org/source/mix-market) for the Indian MFIs operating during the period 2005/06 to 2017/08. Additionally, the data extracted for the financial variable are taken in local currency (INR), and data for employees and borrowers are taken in actual numbers. To estimate the true production frontier, we first detected the outliers by using the procedure suggested by Banker and Gifford (Citation1988). We define an arbitrary decision rule and set the screen level at 1.6 by following Banker and Chang (Citation2006) [5]. If the for any MFI, we declare that MFI as an outlier and removed from the sample. The data with and without outliers are visualized in the Figures A1 and A2, respectively in the Appendices. After cleaning the dataset, we were left with 1102 observations. To avoid any possible inflation effect, we deflate the financial variables by gross domestic product (GDP) deflator, and after that the data are mean normalised following the Sarkis (Citation2007) [3]. The descriptive statistics of input-outputs used in the DEA model are reported in .
Table 3. Descriptive statistics of the inputs and outputs accommodated in DEA model
4. Empirical results and discussion
4.1. Estimation of efficiency estimates
We rely on the overall efficiency which represents the dual goal performance of financial and social aspects of microfinance. The study has combined three outputs namely; gross loans portfolio, financial revenue (i.e., to measure financial aspect) and number of borrowers (i.e., to measure the social aspects) and estimate the overall efficiency scores of individual MFI. We construct the separate efficiency frontier for each year despite a single multi-year frontier. The descriptive statistics of conventional DEA scores () and bias-corrected efficiency scores (
) are reported in and visualized in . The comparison of original and bias-corrected estimates is also shown in the frequency distribution and boxplot (see ). From the empirical results, we note that the original efficiency estimates are overestimated and may mislead the author. We observe that the average bias-corrected efficiency estimates vary from a minimum of 0.619 in the year 2009/10 to a maximum of 0.833 in the year 2017/18 (). Further, we note that the grand mean of original efficiency and bias-corrected efficiency are 0.806 and 0.735, respectively. The results obtained from the bootstrap DEA model are more reliable and useful for drawing the inferences or to use in the second stage of analysis (Khan & Gulati, Citation2019; Wijesiri et al., Citation2015). Therefore, our entire study is based on the bias-corrected efficiency estimates which are more reliable and reflect the real picture.
Table 4. Descriptive statistics of efficiency scores ownership wise
4.2. Trends in the efficiency of the Indian microfinance industry
We observe from the empirical results that the average bias-corrected efficiency estimates vary from a minimum of 0.619 in the year 2009/10 to a maximum of 0.833 in the year 2017/18. Further, during the entire study period, the average bias-corrected efficiency score is 0.735. This is evident that the MFIs industry can further increase the output by 26.5 percent without additional use of any input resources. Furthermore, the empirics of the study found that on average the efficiency of the Indian MFI industry declines sharply during the year 2009/10 (see ). It is worth noting here that this was an adverse impact of the Andhra Pradesh event on the performance of the entire MFI industry. However, the industry had recovered soon with the help of the revised regulatory framework, immediately initiated by the Government and the Reserve Bank of India (RBI) which redefined the operating model of the Indian MFI industry. Though the MFI industry has not grown significantly in terms of financial performance, the operating practices have completely transformed since the beginning. Further, the empirical results suggest that efficiency has declined in the year 2014/15 and 2016/2017. The strict regulatory actions and modifications in the existing policy hinder the performance of MFIs. In addition, the RBI has called application to issue a licence for Small Finance Banks (SFBs) and Payment Banks, this diverts the MFIs efforts from lending to acquiring the license. Further, in 2016/17 the demonetization announcement of the Prime Minister of India has crushed the liquidity of MFIs and decreased the quality of credit portfolio due to lower collection rate (MFIN, Citation2018). However, the MFI industry has recovered soon and the repayment rate has increased in the next financial year 2017/18 which ultimately enhances the efficiency level of MFIs. Furthermore, we found from the results that the industry has accelerated in terms of performance, but the rate of acceleration is not satisfactory to get operated on the best-practice frontier. During the first sub-periods the averages of efficiency estimates were relatively lower, but, after the revised regulatory norms, the performance sharply improves during the second sub-period. This is evident with the higher averages of both original and bias-corrected efficiency estimates during the second sub-period.
Table 5. Annual averages of original and bias-corrected efficiency score for the Indian MFI
During the year 2009/10, immediately before the regulatory changes, the industry was not making equal revenue, few of them were growing at a faster pace and charging higher interest rates, earning big profits out of the poor. This is evident from our empirical results that in the financial year 2006/07 and 2007/08, we observed more variations in the distribution of efficiency estimates. In addition, we note from that efficiency estimates were relatively higher in these years (i.e., before regulatory changes, i.e., first sub-period). We observe the notable efficiency improvement after the inception of new regulatory norms. It is worth noting here that the recommendation of the Malegam committee report has boosted the growth of NBFC-MFIs in the industry; therefore, the MFI industry becomes more professional, organized, regulated and efficient. This is evident from the empirical result of the study, we find a significant change in performances in the second sub-period (see , Panel C).
4.3. Efficiency across distinct ownership structure
To examine the difference across the distinct ownership structure, we divide the entire sample MFIs into two groups namely; NBFC-MFIs (i.e., a new category of MFIs created by the RBI based on the Malegam committee report), and Non-NBFC-MFIs (i.e., includes NGOs, Trust, Societies, and Cooperatives and Section 8 Companies). This section describes the performance differences between NBFC-MFIs and Non-NBFC-MFIs. The annual averages of bias-corrected efficiency estimates are reported in and visualized in . The empirical results reveal that during the first sub-period the performance of Non-NBFC-MFIs is higher than their NBFC counterparts. In contrast, during the second sub-period, the performance of NBFC MFIs is higher. This is evident from the results, during the first sub-period the annual averages of efficiency are 0.713 and 0.721, for NBFC and Non-NBFC MFIs, respectively. Besides, during the second sub-period, the average efficiency estimates are 0.759 and 0.738 for NBFC and Non-NBFC MFIs, respectively. This indicates that new regulatory reforms are favourable to the NBFC MFIs. However, the Non-NBFC-MFIs are equally efficient in the MFI industry during the entire period of study in comparison with their counterparts, i.e. NBFC-MFIs. This is evident from the empirical results; Li test indicates the differences in efficiency are not statistically significant during the entire study period and first sub-period. However, during the second sub-period, the differences in performance between NBFC and Non-NBFC were statistically significant.
Table 6. Averages bias-corrected efficiency score of MFIs across ownership group
We perform three non-parametric tests namely, (i) Adapted Li test (Li, Citation1996) following the guidelines of Simar and Zelenyuk (Citation2006), (ii) Kruskal-Wallis test, and (iii) Jonckheere-Terpstra test. In particular, all the above-mentioned tests are robust and have excellent statistical power to draw the valid inference. The Li test (Li, Citation1996) is adapted to the DEA context by following the guidelines of Simar and Zelenyuk (Citation2006). This test is more powerful and suitable to examine the density of two functions of DEA based efficiency estimates since it accounts for boundedness and continuity condition. One of the advantages of this test is it does not require the number of observation to be equal in each group. The empirical results of the tests are reported in Panel C of . We observe that the performance of Non-NBFC MFIs remained more or less equal with their NBFC counterparts during the entire study periods. Our observations are corroborated by the empirical results; the coefficient of the Li test is not statistically significant. Our findings are consistent with Abdelkader and Mansouri (Citation2019) who finds no statistically significant difference in performance across the legal status. Therefore, we accept the null hypotheses which claim that NBFC and Non-NBFC MFIs are equally efficient (see , Panel C).
4.4. Results of bootstrap truncated regression
We perform bootstrap truncated regression analysis as suggested by Simar and Wilson (Citation2007) to examine the factors explaining the efficiency of Indian MFIs. We regress the bias-corrected efficiency scores on selected environmental variables (using equation 2). The results of the second-stage bootstrap truncated regression are reported in .
Table 7. Results of bootstrap truncated regression
The predictor legal status seems to have a positive association with the financial performance of MFIs. Thus, the results reveal that the NBFC-MFIs are more financially efficient compared to their counterpart Non-NBFC MFIs. These MFIs are professionally managed and equipped with state-of-art technology; therefore, the cost of per rupee lend is low in the case of NBFCs (Servin et al., Citation2012). However, the results are contradictory to the findings of Gebremichael and Gessesse (Citation2016). The MFIs industry always remains in debate for the degree of regulation. The absence of regulation may lead to the industry in crisis, which is evident from the Indian microfinance sector (Quidt et al., Citation2012). Besides, Bassem (Citation2009) found that regulation and financial performance of MFIs have a positive association. The empirical results of our study show that new reforms have improved the financial efficiency performance of the Indian MFIs industry. The positive and statistically significant coefficient of reforms confirms our findings. The results are in contrast with Hartarska and Nadolnyak (Citation2007) who confirmed that regulation does not guarantee financial sustainability.
Further, the maturity (age) level of MFIs is expected to have a bidirectional association with the efficiency level (Hermes & Hudon, Citation2018). The older MFIs are likely to be financially efficient by reaping the advantage of accumulated experience. However, the new MFIs may achieve a higher efficiency level by using start-of-art technology and grow faster than their young and mature counterparts (Liñares-Zegarra & Wilson, Citation2018). Our results suggest that maturity has a positive impact on the financial performance of MFIs. However, the coefficient is not statistically significant. The empirical estimates of the study are in line with Wijesiri et al. (Citation2015).
Furthermore, like Daher and Le Saout (Citation2015), and Ghose et al. (Citation2018) our study found that MFIs enjoy economies of scale, as the size of scale grows the efficiency improves. This is evident from the results that when the assets base increases the MFI tends to become more financially efficient. The coefficient of size is statistically significant at one per cent level of significance. Consistent with Daher and Le Saout (Citation2015), we draw the inference from the empirical results that as the credit quality decreases, the efficiency is likely to declines. However, the coefficient is not statistically significant in our data sample. The results reveal that Equity has a negative and statistically significant impact on efficiency. Our findings are consistent with the conclusion given by Wijesiri et al. (Citation2015). However, Daher and Le Saout (Citation2015) assume that well-capitalised firms are better protected against unexpected operational expenses, and these MFI have a lower cost of funding.
5. Conclusions, policy implications direction for future research
The study examines the trend of efficiency of the Indian MFIs industry operating during the year 2005/06 to 2017/18 in response to the regulatory changes in the Indian microfinance industry. In addition, we investigate the performance difference across the ownership types of MFIs. Therefore, we divide all sample MFIs into two categories NBFC and Non-MBFC-MFIs to perform the analysis. We extend the analysis to explore the efficiency determinants by employing the bootstrap truncated regression.
The empirical results show that the performance of the MFI industry has significantly improved during the second sub-period after the new regulatory reforms. However, there is still scope for further improvements, and on average the MFI industry can further increase the output by 26.5 percent without any additional input resources. In particular, we note from the empirical results that the average efficiency estimates vary from a minimum of 0.619 in the year 2009/10 to a maximum of 0.833 in the year 2017/18. Further, we observe the efficiency levels are 0.735, 0.718 and 0.750 during the entire study period, first and second sub-period, respectively. Although, during the first sub-periods, the average efficiency estimates were relatively lower; however, after the revised regulatory reform the performance sharply improve during the second sub-period. To ensure sustainability, the Indian microfinance industry has seen significant regulatory and structural changes during the second sub-period. In response to the Andhra Pradesh crisis, the Reserve Bank of India (RBI) came with the new regulatory norms in the MFI industry with the purpose to inculcate confidence in the stakeholders. Moreover, we find that the performance of NBFC-MFIs has significantly improved after the regulatory reforms. However, we did not find any performance differences between the first and second sub-periods in the case of Non-NBFC-MFIs. In addition, we observe more growth in the efficiency level of NBFC-MFIs compared to their counterparts, i.e., Non-NBFC MFIs during the study period.
Additionally, the results of bootstrap truncated regression reveal the performance NBFC-MFIs is relatively better than their counterparts. Furthermore, we note that the excess of regulation might hinder the performance of MFIs. Therefore, policymakers and regulators need to tighten the knot when it is needed only; otherwise, this could hamper the performance of Indian MFIs. We note from the results that the deteriorating credit quality of the loan portfolio may hamper the financial performance of MFIs. Therefore, the practitioners of microfinance need to design the optimum framework to assess the risk involved in credit while taking the lending decision. We observe that the use of equity capital may have been much better. The results confirmed that overcapitalize MFIs are efficient, this may be due to the rise of agency problem in the case of the NBFC category of MFIs. The direction of equity capital flow is not towards the small MFIs but rather the large ones. We may conclude that small MFIs must be funded with equity capital by the investors having patience so that the small ones can grow and compete with the large MFIs which have easy access to the capital market.
The study suggests future policy initiatives need to focus on the adoption of more innovative technology in India by outsourcing services to FinTech (financial technology) firms. The financial technology will assist in reducing transaction costs and service time. Moreover, the policymakers and regulators of the Indian MFI industry must understand the basic characteristics of MFIs and formulate the most suitable policy. The regulators must try regulatory-sandbox practices and allow various new start-ups in the controlled environment to offer micro-financial services to the poor. The development of the MFI industry by setting up the proper regulatory framework and convenient operating space for every ownership type of MFIs will benefit in poverty alleviation and enhance the economic prosperity of the poor and women in India. The interested researchers may incorporate the other proxies such number of women borrowers or rural borrowers to measure the social aspects of MFIs. In addition, one may also enquire about the impact of the COVID-19 Pandemic on the performance level of MFIs
Additional information
Funding
Notes on contributors
Asif Khan
Dr. Asif Khan is the Assistant Professor in the Department of Economics and International Business, School of Business, University of Petroleum and Energy Studies (UPES), Dehradun, Uttrakhand, India. His research interest includes efficiency & productivity analysis, microfinance institutions. Asif Khan is the corresponding author and can be contacted at: [email protected]; [email protected]
Dr. Alam Ahmad is currently serving College of administrative and financial sciences, Saudi Electronic University (SEU), Saudi Arab, as an Assistant Professor. His research interest lies in the various fields of International Trade & Finance, Business Economics and Entrepreneurship. Dr. Alam Ahmad can be contacted at: [email protected], Phone: 00966 563,421,917
Saba Shireen is the associate member of the Institute of Company Secretaries of India (ICSI), New Delhi, India. She has vast experience in the industry as a corporate lawyer and company secretary. She has also handled various projects as a liaison officer for international affairs, Indian Institute of Technology (IIT) Roorkee, India.
References
- Abdelkader, I. B., & Mansouri, F. (2019). Performance of microfinance institutions in the MENA region: A comparative analysis. International Journal of Social Economics, 46(1), 47–20. https://doi.org/10.1108/IJSE-06-2017-0242
- Ahmad, A. U. F., & Hassan, M. K. (2007). Regulation and performance of Islamic banking in Bangladesh. Thunderbird International Business Review, 49(2), 251–277. https://doi.org/10.1002/tie.20142
- Athanassopoulos, A. D. (1997). Service quality and operating efficiency synergies for management control in the provision of financial services: Evidence from Greek bank branches. European Journal of Operation Research, 98(2), 300–313. https://doi.org/10.1016/S0377-2217(96)00349-9
- Banker, R. D., & Chang, H. (2006). The super-efficiency procedure for outlier identification, not for ranking efficient units. European Journal of Operational Research, 175(2), 1311–1320. https://doi.org/10.1016/j.ejor.2005.06.028
- Banker, R. D., & Gifford, J. L. (1988). A relative efficiency model for the evaluation of public health nurse productivity, pp. 1–15. In Carnegie Mellon University. Pittsburgh
- Barth, J. R., Nolle, D. E., & Rice, T. N. (1997). Commercial banking structure, regulation, and performance: An international comparison. Managerial Finance, 23(11), 1–39. https://doi.org/10.1108/eb018653
- Bassem, B. S., (2008). Efficiency of microfinance institutions in the Mediterranean: an application of DEA. Transition Studies Review,15(2), 343–354
- Bassem, B.S., (2009). Governance and performance of microfinance institutions in Mediterranean countries, Journal of Business Economics and Management,10(1), 31–43.
- Basu, P., & Srivastava, P. (2005). Exploring possibilities: Microfinance and rural credit access for the poor in India. Economic and Political Weekly, 40(17), 1147–1756. https://www.jstor.org/stable/4416534
- Bayar, Y. (2013). Future of microfinance in the light of recent crises in major microfinance markets. Social Business, 3(3), 243–249. https://doi.org/10.1362/204440813X13778729134363
- Benston, G. J. (1965). Branch banking and economies of scale. The Journal of Finance, 20(2), 312–331. https://doi.org/10.1111/j.1540-6261.1965.tb00212.x
- Bibi, U., Balli, H. O., Matthews, C. D., & Tripe, D. W. (2018). Impact of gender and governance on microfinance efficiency. Journal of International Financial Markets, Institutions and Money, 53(C), 307–319. https://doi.org/10.1016/j.intfin.2017.12.008
- Boateng, A., Huang, W., & Kufuor, N. K. (2015). Commercial bank ownership and performance in China. Applied Economics, 47(49), 5320–5336. https://doi.org/10.1080/00036846.2015.1047089
- Charnes, A., Cooper, W. W., & Rhodes, E., (1978). Measuring the efficiency of decision making units. European Journal of Operational Research,2(6), 429–444.
- Constantinou, D., & Ashta, A. (2011). Financial crisis: Lessons from microfinance. Strategic Change, 20(5‐6), 187–203. https://doi.org/10.1002/jsc.895
- Cull, R., Demirgüç-Kunt, A., & Morduch, J. (2011). Does regulatory supervision curtail microfinance profitability and outreach? World Development, 39(6), 949–965. https://doi.org/10.1016/j.worlddev.2009.10.016
- Daher, L., & Le Saout, E. (2015). The determinants of the financial performance of microfinance institutions: Impact of the global financial crisis. Strategic Change, 24(2), 131–148. https://doi.org/10.1002/jsc.2002
- Dasgupta, R. (2005). Microfinance in India: Empirical evidence, alternative models and policy imperatives. Economic and Political Weekly, 40(12), 1229–1237. https://www.jstor.org/stable/4416372
- Dowling, L. (2011). The Indian Microfinance Institutions (Development and Regulation) Bill of 2011: Microfinance beginnings and crisis and how the Indian government is trying to protect its people. The International Lawyer, 45(4), 1083–1091. https://www.jstor.org/stable/23827264
- Fall, F., Akim, A. M., & Wassongma, H., (2018). DEA and SFA research on the efficiency of microfinance institutions: A meta-analysis. World Development, 107(C): 176–188
- Field, E., Pande, R., Papp, J., & Rigol, N. (2013). Does the classic microfinance model discourage entrepreneurship among the poor? Experimental evidence from India. The American Economic Review, 103(6), 2196–2226. https://doi.org/10.1257/aer.103.6.2196
- Gebremichael, B. Z., & Gessesse, H. T. (2016). Technical efficiency of Microfinance Institutions (MFIs) Does ownership matter? Evidence from African MFIs. International Journal of Development Issues, 15(3), 224–239. https://doi.org/10.1108/IJDI-04-2016-0026
- Ghate, P. (2007). Consumer protection in Indian microfinance: Lessons from Andhra Pradesh and the microfinance bill. Economic and Political Weekly, 43(13), 1176–1184. https://www.jstor.org/stable/4419417
- Ghose, B., Paliar, S. J., & Mena, L. (2018). Does legal status affect performance of microfinance institutions?: Empirical evidence from India. Vision: The Journal of Business Perspective, 22(3), 316–328. https://doi.org/10.1177/0972262918786104
- Gutiérrez-Nieto, B., & Serrano-Cinca, C. (2019). 20 years of research in microfinance: An information management approach. International Journal of Information Management, 47(C), 183–197. https://doi.org/10.1016/j.ijinfomgt.2019.01.001
- Gutiérrez-Nieto, B., Serrano-Cinca, C., & Molinero, C. M. (2009). Social efficiency in microfinance institutions. Journal of the Operational Research Society, 60(1), 104–119. https://doi.org/10.1057/palgrave.jors.2602527
- Haq, M., Hoque, M., & Pathan, S. (2008). Regulation of microfinance institutions in Asia: A comparative analysis. International Review of Business Research Papers, 4(4), 421–450. http://www.bizresearchpapers.com/33%5B1%5D.Mamiza.pdf
- Haq, M., Skully, M., & Pathan, S. (2010). Efficiency of microfinance institutions: A data envelopment analysis. Asia-Pacific Financial Markets, 17(1), 63–97. https://doi.org/10.1007/s10690-009-9103-7
- Hartarska, V., & Nadolnyak, D. (2007). Do regulated microfinance institutions achieve better sustainability and outreach? Cross-country evidence. Applied Economics, 39(10), 1207–1222. https://doi.org/10.1080/00036840500461840
- Hermes, N., & Hudon, M. (2018). Determinants of the performance of microfinance institutions: A systematic review. Journal of Economic Surveys, 32(5), 1483–1513. https://doi.org/10.1111/joes.12290
- Hoxhaj, R. (2010). Regulation and supervision of microfinance in Albania. Business and Economic Horizons, 2(2), 75–81. https://doi.org/10.15208/beh.2010.19
- Hudon, M., & Sandberg, J. (2013). The ethical crisis in microfinance: Issues, findings, and implications. Business Ethics Quarterly, 23(4), 561–589. https://doi.org/10.5840/beq201323440
- Jackson, K. E., & Islam, T. (2005). Regulation of microfinance NGOs: General reflections and the case of Bangladesh. International Journal of Rural Management, 1(1), 45–57. https://doi.org/10.1177/097306800400100103
- Jaiyeoba, H. B., Adewale, A. A., & Ibrahim, K., (2018). Measuring efficiencies of Bangladeshi and Indonesian microfinance institutions: A data envelopment analysis and latent growth curve modelling approach. International Journal of Bank Marketing, 36(2), 305–321
- Kaur, P. (2016). Efficiency of microfinance institutions in India: Are they reaching the poorest of the poor? Vision: The Journal of Business Perspective, 20(1), 54–65. https://doi.org/10.1177/0972262916628988
- Khan, A., & Gulati, R. (2019). Assessment of efficiency and ranking of microfinance institutions in India: A two-stage bootstrap DEA analysis. International Journal of Business Forecasting and Marketing Intelligence, 5(1), 23–55. https://doi.org/10.1504/IJBFMI.2019.099008
- Khan, A., & Shireen, S. (2020). Drivers of financial and operational efficiency of MFIs: Empirical evidences from Eastern Europe and Central Asia. Benchmarking: An International Journal, 27(9), 2679–2697. https://doi.org/10.1108/BIJ-11-2019-0515
- Khandelwal, A. K. (2007). Microfinance development strategy for India. Economic and Political Weekly, 42(13), 1127–1135. https://www.jstor.org/stable/4419411
- Kumar, N., & Sensarma, R. (2017). Efficiency of micro finance institutions in India: A stochastic distance function approach. Journal of Emerging Market Finance, 16(2), 151–168. https://doi.org/10.1177/0972652717712372
- Lebovics, M., Hermes, N., & Hudon, M. (2016). Are financial and social efficiency mutually exclusive? A case study of Vietnamese microfinance institutions. Annals of Public and Cooperative Economics, 87(1), 55–77. https://doi.org/10.1111/apce.12085
- Li, L. Y., Hermes, N., & Meesters, A. (2019). Convergence of the performance of microfinance institutions: A decomposition analysis. Economic Modelling, 81(C), 308–324. https://doi.org/10.1016/j.econmod.2019.05.014
- Li, Q. (1996). Nonparametric testing of closeness between two unknown distribution functions. Econometric Reviews, 15(C), 261–274. https://doi.org/10.1080/07474939608800355
- Lin, X., & Zhang, Y. (2009). Bank ownership reform and bank performance in China. Journal of Banking and Finance, 33(1), 20–29. https://doi.org/10.1016/j.jbankfin.2006.11.022
- Liñares-Zegarra, J., & Wilson, J. O. (2018). The size and growth of microfinance institutions. The British Accounting Review, 50(2), 199–213. https://doi.org/10.1016/j.bar.2017.11.006
- Mader, P. (2013). Rise and fall of microfinance in India: The Andhra Pradesh crisis in perspective. Strategic Change, 22(1–2), 47–66. https://doi.org/10.1002/jsc.1921
- Malegam, Y. H., (2011). Report of the sub-committee of the central board of directors of Reserve Bank of India to study issues and concerns in the MFI sector. Reserve Bank of India, India
- Masood, T., & Ahmad, M. I. (2012). Efficiency of microfinance institutions in India for poverty alleviation”. American Journal of Entrepreneurship, 5(2), 64–78. http://search.ebscohost.com/login.aspx?direct=true&db=ent&AN=89460886&site=ehost-live
- McGuire, P. B. (1999). Policy and regulation for sustainable microfinance: Country experiences in Asia. Journal of International Development: The Journal of the Development Studies Association, 11(5), 717–729. https://doi.org/10.1002/(SICI)1099-1328(199907/08)11:5<717::AID-JID587>3.0.CO;2-H
- MFIN, (2018). Annual report 2018, Microfinance Institutions Network, New Delhi
- MIX Market, (2012). Global outreach & financial performance benchmark report-2012. Available at: http://www.themix.org.
- MIX Market, (2018). Global outreach & financial performance benchmark report-2017-2018.USA.
- Muneer, B. M. (2016). Macroeconomic determinants of technological change in Indian microfinance institutions. Global Business Review, 17(5), 1179–1195. https://doi.org/10.1177/0972150916656685
- Muneer, B. M., & Kulshreshtha, P. (2014). Productivity change and technical efficiency in Indian microfinance institutions. Studies in Microeconomics, 2(2), 165–200. https://doi.org/10.1177/2321022214545207
- Nadiya, M., Olivares-Polanco, F., & Ramanan, T. R. (2012). Dangers in mismanaging the factors affecting the operational self-sustainability (OSS) of Indian microfinance institutions (MFIs)-an exploration into Indian microfinance crisis. Asian Economic and Financial Review, 2(3), 448–462. http://www.aessweb.com/download.php?id=1403
- Nair, T. S. (2001). Institutionalising microfinance in India: An overview of strategic issues. Economic and Political Weekly, 36(4), 399–404. https://www.jstor.org/stable/4410238
- Ndambu, J. (2011). Does regulation increase microfinance performance in Sub-Saharan Africa. Frankfurt School of Finance and Management, Technical Note, 3/2011, 1–11. https://www.frankfurt-school.de/dam/jcr:48819922-873c-437b-b222-0a5f23fd08a0/Regulation%20and%20MF%20performance%20in%20SSA.pdf
- Palmer, E. (2013). The Andhra Pradesh microfinance crisis and American payday lending: Two studies in vulnerability. Éthique et économique/Ethics and Economics, 101, 44–57. https://philpapers.org/rec/PALTAP.
- Pasiouras, F., Tanna, S., & Zopounidis, C. (2009). The impact of banking regulations on banks’ cost and profit efficiency: Cross-country evidence. International Review of Financial Analysis, 18(5), 294–302. https://doi.org/10.1016/j.irfa.2009.07.003
- Pati, A. P. (2012). Regulation versus outreach and sustainability: A study of the performance of microfinance institutions in India. IUP Journal of Bank Management, 11(4), 41–56. https://ssrn.com/abstract=2186399.
- Piot-Lepetit, I., & Nzongang, J. (2014). Financial sustainability and poverty outreach within a network of village banks in Cameroon: A multi-DEA approach. European Journal of Operational Research, 234(1), 319–330. https://doi.org/10.1016/j.ejor.2013.10.004
- Priyadarshee, A., & Ghalib, A. K. (2011). The Andhra Pradesh microfinance crisis in India: Manifestation, causal analysis, and regulatory response. Brooks World Poverty Institute Working Paper No. 157. Brooks World Poverty Institute, [Online] https://ssrn.com/abstract=1983206 (Accessed 15 January 2018)
- Psillaki, M., & Mamatzakis, E. (2017). What drives bank performance in transitions economies? The impact of reforms and regulations. Research in International Business and Finance, 39(C), 578–594. https://doi.org/10.1016/j.ribaf.2016.09.010
- Purkayastha, D., Tripathy, T., & Das, B. (2014). The impact of regulations on microfinance industry: A strategic perspective. IUP Journal of Business Strategy, 11(3), 24–40. https://ssrn.com/abstract=2633408
- PwC, (2019). Vision of microfinance in India. PWC and SIDBI, New Delhi, India
- Quidt, J., Fetzer, T., & Ghatak, M. (2012). Microfinance, market structure, and borrower welfare: Regulatory lessons from the Indian crisis. LSE Paper, London School of Economics (LSE), London, UK. [Online] http://econ.lse.ac.uk/staff/mghatak/MFIreviewWB.pdf (Accessed 5 September 2018).
- Ranjani, K. S. (2012). Regulating microfinance institutions in India: A conceptual framework. Synergy, 10(1), 51–62. https://www.jstor.org/stable/23391209
- Ray, S., & Mahapatra, S. K. (2016). Penetration of MFIs among Indian states: An understanding through macro variables. International Journal of Development Issues, 15(3), 294–305. https://doi.org/10.1108/IJDI-05-2016-0030
- Reichert, P. (2018). A meta-analysis examining the nature of trade-offs in microfinance. Oxford Development Studies, 46(3), 430–452. https://doi.org/10.1080/13600818.2018.1427223
- Sa-Dhan, (2016). The Baharat microfinance report 2016, Sa-Dhan, New Dlhi, India
- Sa-Dhan, (2018). The Baharat microfinance report 2018, Sa-Dhan, New Delhi, India
- Sarkis, J. (2007). Preparing your data for DEA’. In J. Zhu & W. D. Cook (Eds.), Modeling data irregularities and structural complexities in data envelopment analysis. (pp. 305–320). Springer.
- Sealey, C. W., & Lindley, J. T. (1997). Inputs, outputs, and a theory of production and cost at depository financial institutions. The Journal of Finance, 32(4), 1251–1266. https://doi.org/10.1111/j.1540-6261.1977.tb03324.x
- Servin, R., Lensink, R., & Van Den Berg, M. (2012). Ownership and technical efficiency of microfinance institutions: Empirical evidence from Latin America. Journal of Banking and Finance, 36(7), 2136–2144. https://doi.org/10.1016/j.jbankfin.2012.03.018
- Shen, C. H., & Chang, Y. H. (2006). Do regulations affect banking performance? Government governance may matter. Contemporary Economic Policy, 24(1), 92–105. https://doi.org/10.1093/cep/byj013
- Simar, L., & Wilson, P. W. (2000). A general methodology for bootstrapping in non-parametric Frontier models. Journal of Applied Statistics, 27(6), 779–802. https://doi.org/10.1080/02664760050081951
- Simar, L., & Wilson, P. W. (2007). Estimation and inference in two-stage, semi-parametric models of production processes. Journal of Economics, 136(1), 31–64. https://doi.org/10.1016/j.jeconom.2005.07.009
- Simar, L., & Zelenyuk, V. (2006). On testing equality of distributions of technical efficiency scores. Econometric Reviews, 25(4), 497–522. https://doi.org/10.1080/07474930600972582
- Sinha, R. P., and Pandey, P., (2019). Efficiency of Microfinance Institutions in India: A Two-Stage DEA Approach. International Journal of Rural Management,15(1). 49–77.
- Trujillo, V., Rodriguez-Lopez, F., & Muriel-Patino, V. (2014). Microfinance regulation and market development in Latin America. The B.E. Journal of Economic Analysis & Policy, 14(4), 1615–1644. https://doi.org/10.1515/bejeap-2013-0145
- Trujillo-Tejada, V., Muriel-Patino, V., & Rodríguez-López, F. (2015). How is microfinance being regulated in Latin America? Enterprise Development and Microfinance, 26(4), 343–357. https://doi.org/10.3362/1755-1986.2015.028
- Wichterich, C. (2012). The other financial crisis: Growth and crash of the microfinance sector in India. Development, 55(3), 406–412. https://doi.org/10.1057/dev.2012.58
- Widiarto, I., & Emrouznejad, A. (2015). Social and financial efficiency of Islamic microfinance institutions: A data envelopment analysis application. Socio-Economic Planning Sciences, 50(C), 1–17. https://doi.org/10.1016/j.seps.2014.12.001
- Wijesiri, M., Viganò, L., & Meoli, M. (2015). Efficiency of microfinance institutions in Sri Lanka: A two-stage double bootstrap DEA approach. Economic Modelling, 47(C), 74–83. https://doi.org/10.1016/j.econmod.2015.02.016
- Yadav, R., & Wongsurawat, W. (2018). How regulation revived micro-lending after the Andhra Pradesh crisis. International Journal of Public Policy, 14(5–6), 360–373. https://doi.org/10.1504/IJPP.2018.096644