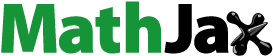
Abstract
The purpose of the study was to examine the effect of monetary policy on key macroeconomic indicators in Ghana. The study used annual time series data from 2002 to 2017, which was sourced from the World Development Indicator (WDI) and the Bank of Ghana (BOG). The data were converted to Quarterly data between 2002Q1 and 2017Q4, which covers a sample period of sixteen years. The study employed the Autoregressive Distributed Lagged Model (ARDL) for analyzing the data. Unit root test was conducted using the Augmented Dickey-Fuller (ADF) tests, and the results of the analysis exhibited a cointegration realtionship among the variables of order one (1). Monetary policy changes affected lending rates by (0.32%) compared to the other variables. Overall, the results suggests that monetary policy affects macro economic indicators performance in Ghana. Based on the foregoing, the paper recommends that, in efforts to enhance the effectiveness of monetary policy, the need for policy intervention in determining the stance of fiscal policies, develop financial markets, and liberalize controlled interest rates.
PUBLIC INTEREST STATEMENT
These days, a major concern which has interested the thoughts of public is that of the relative efficiency of monetary policy in manipulating macro economic variables. This is the result of existing evidence that effective control of monetary policy is vital to the macro economic objective. There is a general agreement among economists and policymakers that monetary policy stabilizes the economy through key macro economic indicators like interest rate and lending rate. As a result, several steps have been taken by governments to provide short-term solutions to the problem including a monetary management system, both globally and on sectorial basis. It is from this background that researchers tried to investigate the effect of monetary policy on key macro economic indicators in Ghana. The findings showed that monetary policy changes affects lending rates by (0.32%) compared to the other macro economic variables. Overall, monetary policy affects macro economic indicators performance in Ghana. In the end, appropriate recommendations have been forwarded in this paper.
1. Introduction
The early 1980s saw Ghana experience severe economic difficulty (Aryeetey & Baah-Boateng, Citation2015). As a result, several steps were taken by government to provide short-term solutions to the problem including a monetary management system of direct control involving the imposition of price ceilings, both globally and on sectorial basis (Aryeetey & Baah-Boateng, Citation2015). Individual commercial banks’ lending activities had to be consistent with national macroeconomic targets like growth, inflation, and external balance. However, these arrangements were largely regarded to be ineffective, only further augmenting the inefficiencies in the various sectors of the economy.
In response to the economic crisis in the early 1980s, the Structural Adjustment Program formulated by the World Bank and the IMF (Jeong, Citation1995) was rolled out. The program emphasized the elimination of financial repression elements within the economy like price and credit controls, and the removal of subsidies, while promoting economic liberalization policies like the promotion of free trade, among others. There is a general agreement among economists and policymakers that monetary policy works mainly through interest rates (Amidu, Citation2006). Monetary policy as one of the many factors that affected lending rate (Castro & Santos, Citation2010).
A major concern, which has interested the thoughts of governments for decades, is that of the relative efficiency of monetary policy in manipulating economic variables. Monetary policy refers to the attempt made in trying to realize the national economic objectives of full employment without inflation, rapid economic growth as well as balance of payments equilibrium through the management of the economy’s supply of money and credit” (Iyoha & Oriakhi, Citation2002). Monetary policy involves the use of different measures with the aim of regulating the value, supply and cost of money in consonance with the expected level of economic activity (Quartey & Afful-Mensah, Citation2014). The common objectives of any monetary policy may include price stability, maintenance of balance of payments equilibrium, creation of employment, output growth, and sustainable development (Quartey & Afful-Mensah, Citation2014).
The understanding of the transmission mechanism by most central banks has largely led to their success at controlling inflation and stabilising output within their domestic economies (Abradu-Otoo, Amoah & Bawumia, Citation2003). This is because many factors have been enumerated as causing changes in the lending rate of banks. Monetary policy can influence real sector activity and output through several channels.
The theory of transmission mechanism classified the channels as interest rate channel, asset price channel, and credit channels. However, the monetary policy has different transmission mechanisms to ensure its effectiveness. As Coll et al. (Citation2005) noted, the bank lending channel can only be possible under two conditions namely; the dependence of borrowers on the banks for debt financing, and the significant effect of monetary policy on the supply of loans of these banks. Monitary policy cannot be overlooked as banks are the principal providers of funds to governments, corporate bodies, and individuals as reported by Ladime, Kumankoma-Sarpong, and Osei (2013). According to Mishkin (Citation1995), there is the need for interpreting the effect of policy actions in an economy when conducting a monetary policy.
In this regard, it becomes necessary to identify some of the efficient monetary policy variables that hinders decisions to bring about more economic performance in Ghana. However, an appropriate measurement of the effects of monetary policy on policy targets of macroeconomic indicators is limited in Ghana. Based on these observations, there is a need for continuous inquiry on the effect of monetary policy on the macroeconomic variables. This is because the neo-classical economist believe that time preference plays an important role in determining interest rate as Adoah (Citation2015) noted.
Despite, quite a number of studies exists on the efectiveness of monetary policy on macroeconomic variables in Ghana (Kovanen, Citation2011; Havi & Enu, Citation2014; Akosah, Citation2015; Commodore, 2017). They were more focused on bank-specific factors, industry-specific factors and other macroeconomic variable like inflation. This study, however, focuses more on the macro-economic determinants of Ghana’s lending rates. Secondly, it is more appropriate to use quarterly data in monetary policy since quarterly data seem to be better for econometric model, especially in monetary policy, yet most of the existing studies that used econometric models for Ghana have been estimated with annual data.
The researchers adopted a Quadratic-Match Average Method of Low to High frequency conversion of annual data to quarterly data. Other alternative methods use by previous researchers to convert annual data to quarterly data are are the Denton (Citation1971) approach, the Chow-Lin (Citation1971) procedure, and the cubic spline interpolation process. The first two methods use related series as indicators to convert low-frequency data into high-frequency data, whereas the latter one was based on univariate framework. The rationale for this choice of quadratic-Match Average has the ability to fit local quadratice polynomial by using groups of three adjacent points from the low frequency annual data and fitting a quadratic so that the mean of the maximum frequency points corresponds to the minimum frequency data actually observed. Thirdly, the significant contribution to knowledge in this study is that it utilizes longer period of estimation. Further, we apply unit root tests to examine the time-series properties of derived quarterly series. The results from cointergation tests provide significant evidence of the existence of the long-run equilibrium relationship between the underlying series of interest and the indicator variable. It would also help improve the efficiency of forecasting of macroeconomic indicators. The derived higher frequency series would also be worthwhile in financial modelling. On the contrary, our study does not provide a guarantee that the sum of the quarterly totals will equal the total of the observed but Friedman argued that they need not be equal to provide reliable results. It is worth noting that we are not the first to convert annual data to quarterly data, several studies such as Kemal and Arby (Citation2004), Mehmood &Arby (2005), Hanif et al. (Citation2013), Abdul et al. (2013) have been converting annual data to quarterly data. This study used time series data, which cover a period of sixteen years, using quarterly data from 2002Q1–2017Q4 to estimate the results on the impact of monetary policy in Ghana. Finally, we examine the time-series properties of the obtained quarterly series. In sum, we deem that the results on monetary policy and the macroeconomic indicators that we present in this paper are robust, consistent, reliable, and efficient and also in line with other econometric approaches of univariate interpolation or processes of disaggregation.
2. Methodology
2.1. Data type and source
Secondary data were used for the study. Specifically, data from World Development Indicator (WDI) and the Bank of Ghana were employed. These also included annual banking reports, summary of economic and financial data and monetary policy committee report of the Bank of Ghana. However, the annual data were converted to quarter;y data. Quarterly time series data from 2002 to 2017 of all the variables, with a total of 63 quarterly observations was used. The data commenced from 2002 because the monetary policy rate (MPR) was first introduced and enacted during this period. It also used the arithmetic average of lending rates of all banks in Ghana at each particular point to estimate the lending rate within the period. Quarterly data were preferred since it showed a true reflection and more precise effect of the policy rate on the lending rate.
2.2. Model specification
Following Lartey (Citation2018) in determining the link between monetary policy and lending rate, this section specifies an appropriate model for the analysis. The monetary policy rate represented monetary policy in the model because MPR is the instrument that mostly influences commercial banks’ lending rates directly among all the other instruments of monetary policy.
The functional relationship of the empirical model is stated thus:
Where:
ALR = Average Lending rate (%)
MPR = Monetary Policy Rate (%)
TBILL = 91 days Treasury Bill Rate (%)
INF = Inflation
The data were transformed to minimize the possibility of spurious result amongst others. Hence, the log function is taken of all the variables. Log transformation is necessary since it can reduce the problems associated with heteroscedasticity and non-normality of the variables. The study used a log-linear modelling specification. The choice of this model is as a result of its superiority to other approaches based on both theory and empirical basis (Kalim & Shahbaz, Citation2009).
2.3. Variable description
2.3.1. Lending rate
It is described as the rate at which commercial banks lend to individuals. It is also the bank rate that usually finances the needs of the private sector. Every bank computes base-rate by factoring idiosyncratic variables as general industrial variables. However, the computation of the base rate was modified from April 2018 by the new Ghana Reference Rate (GRR), deemed as more uniform compared to the former formula. The risk premium of each individual is then added to arrive at the lending rate. The arithmetic average lending rates of all universal banks in Ghana in the respective years was used as a proxy for the market lending rate.
2.3.2. Monetary policy rate
In the conduct of monetary policy, the Central Bank use diverse tools. These tools are employed to stabilize prices in the economy and ensure economic growth. One may say the quest to prevent the occurrence of banking crises makes the central bank more robust in the operations. Therefore, the policy rate among other tools serves as a signalling rate for all other rates in the economy. Other countries in different jurisdiction however use different tools in their main monetary operations. Notable examples include: discount rates, repurchase rate and interbank rate etc.
2.3.3. Money supply (M2+)
The stock of money available in the country at a particular time period determines the level of growth and health status of the economy. It was one of the proximate targets of the monetary policy. Before the year 2002, this method was predominantly used to control the economy. The monetary aggregates were used to stabilize prices and economic growth. According to Mishkin (Citation2007) through the liquidity effect, an expansionary monetary policy will cause real interest rate to fall. Cost of capital reduces leading to an increase in investment spending by firms and households. It is quoted as Broad Money (m2+) as a percentage of GDP. A negative relationship is expected between lending rate and money supply in this study.
2.3.4. 91- day treasury bill rate
Interest rate on securities used to finance short-term financial requirements of the Government. The risk attitude and appetite for long-term credit of most Ghanaians are often low due to the instability of macro-economic indicators. This may be attributed to different reasons. Nonetheless the 91-day investment is predominantly bought into compared to the other securities. Banks prefer to lend to the government through treasury bill because the rates are often times above inflation rate and earn banks positive returns and are also relatively safe compared to other investment. However, it must be noted that Treasury bill rate is an indicator of interest rate policy undertaken by the government and acts as a benchmark for rates charged by commercial banks, Garr (Citation2013). Therefore, changes in the MPR affect money market rate, which later affect lending rate (Matemilola et al., Citation2015).
2.3.5. Inflation
Consumer Price Index (CPI) is used as a proxy for Inflation in the study. Changes in the average general prices in the economy determines the health of the economy. The main target or goal for the central bank thus to ensure consistency in the equation it was included. Theories categorize inflation to be either demand pull or cost push. Whichever the reason is, the study will only seek to identify the relationship with lending rates.
2.3.6. Expected Signs of Variables
2.4. Estimation method
The relationship between lending rates and monetary policy rate was analyzed using quantitative data. The statistical package used for computing and analyzing the data was Econometric Views (Eviews 9). Price series data are mostly nonstationary because there is no fixed level for the price. Therefore, a unit root test was conducted using the Augmented Dickey-Fuller (ADF) tests to test for the stationarity of the variables.
2.4.1. Unit root test
It is necessary to first evaluate the stationary state of the data to prevent misleading outcomes. In this regard, the unit root test was conducted to test for stationarity of the variables. The unit root test is a test carried out to assess if the variables under consideration are stationary. The bounds test is based on the assumption that the variables are either I (0) or I (1) series. Therefore, in order to avoid spurious results due to the presence of an I (2) series a unit root test was conducted. The Augmented Dickey-Fuller (ADF) test and the Phillips-Perron (PP) test were employed to test for stationarity.
The ADF test is used in determining the order of integration of each series in the model because it assumes the errors are statistically independent and have a constant variance. In addition to this method, a more robust approach was the PP test, which highly tested for heteroscedasticity and serial correlation in the error term. In analysis, if the paper fails to reject the null hypothesis of a unit root at level, first difference of the series is taken to ensure stationarity. Statistically, if the t-ratio is less than the critical value the null hypothesis of a unit root for each variable (i.e. the series is non-stationary) is accepted. The variables are therefore nonstationary in levels. This conclusion does not rule out the fact that some series will have an Integral order of zero I (0). For the series that are non-stationary, the first difference is evaluated. The null hypothesis is then rejected if the series is considered stationary. This implies that the series is integrated of order one I (1).
2.4.2. Co-integration test
In the case of a mixed order integration of variables provided such series are not I(2) or more; the ARDL cointegration technique is the appropriate method. As a result, the next process after the stationarity is the co-integration test. By cointegration, a long run relationship between independent and dependent variables can be examined in this study. The test procedure adopts the error correction model (ECM) framework and also makes use of the Wald/F-statistic. In order to avoid spurious results, the ECM framework is used. This is because it accounts for previous disequilibrium is corrected in current periods. In this regard, the co-integration test was conducted so as to determined the estimate of the short- and long-run relationship of the variables. The relationship between the lending rate and the independent is established. If there is no long-run relationship but the variables are co-integrated, the ARDL technique is applied. In the presence of long-run relationship after the bounds test, the error correction model is applied.
2.4.3. Autoregressive distributed lag (ARDL) model
The research utilized the Autoregressive Distributed Lag (ARDL) model, also called bounds testing method as was suggested by Pesaran et al. (Citation2001), to examine the relationship between lending rate and monetary policy rate. The presence, direction and extent of a long-run relationship between the dependent variable and the various independent variables is established as well. It provides a very valuable vehicle for testing for the presence of long-run relationships between economic time series (Giles, Citation2013). The model is autoregressive because the dependent variable is explained in part by the lagged values of itself. It also has a distributed lag component in the form of successive lags of the explanatory variables (Giles, Citation2013). In case, there is an autocorrelation, the customary ARDL requirement needs to be augmented with a satisfactory number of lagged changes in the repressors before estimation and interpretation are carried out (Pesaran et al., Citation2001).
The ARDL model is stated as:
The regress and a vector of a series of explanatory variables in equation (3.3) are given by and
, respectively. n represents the number of regressors and k implies the lag order.
is the error term and Δ is the difference operator.
and
are the lags of the independent and the dependent variables.
represents the coefficient of the vector of independent regressors. The null hypothesis of no cointegration is tested against the alternative hypothesis of the presence of cointegration relationship. Lower bounds of the cointegration test are integrated of order zero {I (0)}.
2.4.4. ARDL bounds testing procedure
According to Giles (Citation2013) the bounds test method is preferred because it can be used with a mixture of I (0) and I (1) data. It also involves a single-equation set-up, making it simple to interpret and implement. Finally, different variables can be assigned different lag-lengths as they enter the model. In order to determine the existence of a long run relationship between the dependent and independent variables, the bounds test is most appropriate. The lag length was automatically selected by eviews 9 according to the Akaike Information Criterion (AIC). The null hypothesis for this test is no long-run relationship between the dependent and the various independent variables. To make a decision, the F statistic is compared with the lower and upper bounds. If the F statistic is less than the lower bound at the specified significance level, fail to reject the null hypothesis. This means there is no long-run relationship. If the F statistic is greater than the lower bound but less than the upper bound, the test is inconclusive. If the F statistic is greater than the upper bound, reject the null hypothesis. This means there is a long run relationship between the dependent and the independent variables.
3. Results and discussion
3.1. Interpretation of descriptive statistics
The basic characteristics of the variables in the study are presented in
Table 1. Measures and Expected Effects of Study Variables
Table 2. Descriptive Statistics
Evidenced from the table, the mean of average lending rate, treasury bill, monetary policy rate inflation and money supply are all positive. It shows the average of each of the variables under study. The values of the average lending rates fluctuated between 3.1676 and 3.6889 and their dispersion around the mean was 0.1240. Monetary policy rates oscillated between 2.5258 and 3.3141 and a variability of measure of 0.2484 from a mean of 2.8820. All the variables but Treasury bill rate and money supply are negative skewed. Both negative and positive skewness implies the tails of the distribution are fat in those areas. However, it can also be implied that inflation rates are normally distributed because the value deviates from a kurtosis if 3. Average lending rate, policy rates, monetary policy rates, Treasury bill rates and money supply according to the kurtosis rule are not normally distributed with values of, 2.4761, 1.8127, 1.7661 and 1.7560, respectfully.
3.2. Time series properties
Most econometric studies show that macroeconomic data which are analyzed with time series are non-stationary at levels, Engel and Granger (Citation1987). This then presupposes that most Ordinary Least Square Regression carried out may not be reliable in the absence of diagnostic checks. Hence the need for diagnostic checks of all the variables to ensure that they become the best linear unbiased estimators. This section therefore examines the time series properties of the data by presenting the Augmented Dickey-Fuller (ADF) and Phillips-Perron test (PP). The Phillips-Perron test was conducted in addition to the ADF test to check the robustness of the unit root test results. The PP test modifies the ADF test and rectifies serial correlation and heteroscedasticity in the error term. The test regression included an intercept (constant) and a linear trend for both logarithmic levels and the logarithmic first difference of the variables using E-views 9.0.
3.2.1. Unit root test
In order to prevent spurious results, stationarity test is performed on each of the variables. A summary of the unit root test for both ADF and Phillips-Perron of the variables used in the study is illustrated in The result of the unit root as shown in indicated all values of the series according to ADF and Phillips-Perron test (PP) are not stationary in their levels both at constant without trend and constant with trend. First difference is therefore performed to check the presence of unit roots.
Table 3. Result of The Unit Root Test (Levels)
The test statistic of lnALR, lnMPR, lnINF, lnM2+, lnTBR-91day are found in the rejection area at the various significance level. It is shown in the table as the ADF values were less than critical value at 1%, 5% and 10% It also implies that the null hypothesis is rejected indicating the absence of unit root. The absence of unit root in the test statistic implies an existence of I (0) and I (1).
3.2.2. Unit root test at first difference
At first difference, the series were stationary at a 1% significance level for all the variables. This was however done because at levels, the series were not stationary hence the need to be differenced again to attain stationarity. At the critical values (−3.46178, −2.875128, −2.574090), the t statistic was greater with or without linear trend. Therefore, the null hypothesis of stationarity can be accepted which presupposes that at first difference we failed to reject stationarity. It is also evidence from the table that all variables were stationary at first difference i.e. I (1). With the presence of stationarity on all the variables, ARDL framework will then be applied to test for Co-integration amongst variables amongst others. Also, it indicates the absence of I (2), thereby making the ARDL test most appropriate.
3.3. Co-integration test
A linear combination of variables that are I (1) which produces a stationary series need to be co-integrated (Olokoyo, Citation2011). The relationship amongst the variables may be farther apart in the short run but will draw close and move together in the long run. Therefore, to establish whether this relationship exist the Co-integration test was applied. The bounds test approach was therefore necessary to find the relationship between lending rates and monetary policy rate, hence the response of lending rates to changes in the policy rate. It can be inferred from , that the value of the computed F-statistic is 8.346446 which is greater than the lower and upper bound levels at all the critical values at 10%, 5% and 1% significance level. Simply put, the computed of F value of 8.346446 is greater than 4.401 and 5.06 at 5% and 10% significance level, respectively. The null hypothesis is therefore rejected since there is a long-run relationship among the variable. This therefore certifies the Co-integration test among the variable when lending rate is used as the dependent variable.
Table 4. Result of the Unit Root Test (First Difference)
3.4. Estimated long run ARDL result
The long-run relationship is estimated by selecting ARDL (1, 0, 3, 0, and 0) based on Akaike Information Criterion (AIC). The empirical evidence of the response of average lending rates to changes in policy rate is reported in . The dependent variable of the equation is the average lending rates.
Table 5. ARDL Bounds Test for Co-integration Relationship
Table 6. Estimated long run ARDL Result
illustrates the long run relationship between the variables. It can be observed from the result that monetary policy rate and money supply (lnM2+) were significant at all the alpha levels but more so at 99.9% confidence interval to be precise. However, the money supply (lnM2+) was negatively significant. This shows that graphically, the regression does not pass through the origin. However, there is a 0.03% decline in average lending rates when the supply of money (m2+) increases at a 1% significance level in the long run. A tight or contractionary monetary policy embarked on by the central bank reduces the supply of money. Inferring from the law of demand and supply, a reduction in the supply of money implies a shortage hence an increase in prices. The cost of funds as an element of price also increases. The findings are supported by Amidu (Citation2006) whose study indicated an expansion of the economy through increase in money supply, induces bank to increase credit portfolios. All things being equal, increase in credit portfolio implies a surplus thus cost of funds reduces, as backed by the loanable funds theory.
Inflation was also significant at 5% significance level, indicating that an increase in inflation will lead to a 0.083% increase in average lending rates. This finding is consistent with other researchers and Amidu (Citation2006) who stated the reduction in demand for bank credit by firms due to low productivity caused by high inflation. Put differently, at high lending rates, demand for credit falls all other things being equal. However, inflation rate was insignificant in his study and it can be attributed to the use of prime rate instead of policy rate to influence lending behaviour and Baoko, Acheampong and Ibrahim (Citation2017) also suggested Amidu’s use of OLS produced biased results more so with a short dimension between 1998 and 2004. It is noted that a unit change in monetary policy rate increases the average lending rates by 0.32% in the long run.
Therefore the policy rate adequately served its signalling purpose by being a reference cap for the average lending rate. Thus, its significance at a 1% significance level indicated it importance as well as the response of lending rates to it changes as represented by a large coefficient fraction compared to the other variables.
Increasing Treasury bill rate by 1% leads to 0.13% increase in average lending rate at a 95% confidence interval. Aside monetary policy rate, Treasury bill rate account for the second largest fraction of influence on lending rates (0.13%). The study result is in consonance with the findings of Garr (Citation2013) which stated that there is an upsurge in cost of funds when government rely heavily on domestic banking sector for debt financing.
It also increases competition for funds through the issuance of treasury bills, because banks prefer to lend to the government which is relatively safe. Hence in order to lend to individuals whom are deemed riskier, the rates should be higher than the Treasury bill rates. Also, Bernanke and Blinder, (Citation1992) research findings used three (3) month Treasury Bills rate to capture exogenous shifts in monetary policy and realized that it reduced bank loans due to high lending rates.
3.5. Estimated short run error correction model (ECM)
From the bounds test conducted earlier in , the result indicated a long-run relationship between the variables. Engel and Granger (Citation1987) however suggested that a valid error correction is represented in the variables when the series are co-integrated. Hence the dependent variable is able to respond to shocks in the independent variable and reconciles the short run behavior of the variable to its long run behavior. In addition, the coefficient of the error correction model measures the speed of adjustment in gradually restoring equilibrium in the dynamic model from deviations in the long run equilibrium.
CM = lnALR-(0.3197*lnMPR+ 0.1385*lnTBR -0.0304*lnM2_+0.0826*lnINF + 1.8621)
It can be deduced from that the estimated coefficient of the ECM term is −0. 197,663. It is negatively and significant at a 99.9% confidence interval which presupposes its efficiency in restoring itself to equilibrium. Also, it will take a speed of 19.7663% to restore any disequilibrium of within a period of one month. 19.7663% represent a relative weak speed of adjustment in the short run. The relatively low pass- suggests rigidity in the banking system which may be due to underdevelopment of the financial system.
Table 7. Short iiRun iiResult
The strength of the relationship of the model is described by the coefficient of determination of 94.35%. The R-squared of 94.35% explains the variations in the average lending rate caused by unit changes in the independent variables in the equation. This implies that the regression has a good fit, a strong relationship amongst the variables and hence very reliable. The study further realized the insignificance of Treasury bill on average lending rate in the short run. It was however negatively insignificant with about two lags period which implied the inability of the effect or treasury bill changes to be felt immediately in the short run. All things being held constant the higher the credit risk the higher the lending rates as cited by the loan pricing theory.
Therefore, inferring from the loan pricing theory, the insignificance of Treasury bill in the short run is similar to Garr (Citation2013) insignificance of Treasury bill rate on credit risk. However, the negative relationship can be explained in relation to the diversification of banks portfolio. In order to diversify their portfolio and reduce risk in general, banks reduce lending rate to risky individuals to minimize their risk of default. Ceteris paribus, the lower the price the higher the demand for loans by the private sector. Therefore, by this diversification efficient banks will still be able to make a lot of returns on their assets. Also due to relationship lending and the number of customers banks trust and have built a long-term relationship with, the reduce the rates to maintain their relationship with them. The insignificance of this relationship buttresses the collusive banking theory and the underdevelopment of the banking sector.
However, monetary policy rate, inflation and money supply were significant at 5% alpha level in the short run. The coefficient of money supply has a negative elasticity of −0.006% and it is statistically different from zero at 5%level of significance. The money supply is however significant at both long run and short run and exerts negative pressure on both instances. In the short run a unit increase in money supply reduces average lending rate by 0.006. This is theoretically backed by Mishkin (2007) the author of “The economics of money, banking, and financial markets”. He stated that through the liquidity effect, an expansionary monetary policy will cause real interest rate to fall. Empirically, Hung and Pfau (Citation2009) findings also concluded that increasing money supply led to a decline in cost of credit thereby increasing credit supply
The inflation rate were relatively more significant at a 5% significance level(0.0146) in the long run than in the short run(0.0190).
4. Conclusion
This study sought to ascertain response of changes in monetary policy on average lending rates and also to determine other macroeconomic variable that affected lending rates. The study used monthly data in Ghana between the period of 2002 to 2017 on average lending rates, monetary policy rate, Treasury bill rate, money supply and inflation. Monetary policy rate (MPR) was a proxy for monetary policy tool which was established in the year 2002. The MPR adopted was to signal all rates in the economy in view of stabilizing inflation and output. The Inflation targeting implemented by the central bank in 2007 after a transition from monetary aggregate was highly consistent with the policy rates. Per the loanable funds theory as stated earlier, money supply aside being a monetary policy tool as well, its fluctuation directly affects the lending rates as cited by many researchers.
The average lending rates was a representation of lending rates of all banks in the country as at the time of the study. The Treasury bill rate was included basically because in order to achieve an effective monetary policy objective, it must be consistent with the fiscal tool. It was also used to represent a macroeconomic variable just as inflation rate. The findings of the study found a positive response of average lending rates to changes in monetary policy rate. MPR was significant in both long and short run and had a large marginal effect on lending rates compared to all the other variables in the equation. However, in the short run the speed of adjustment was relatively slow. This implied a more rigid downward adjustment of average lending rate to changes in all the variables. A study by Matemilola, et al. (2015) attributed the asymmetry response to the underdevelopment of the financial sector.
The study explained by the positive effect of inflation and Treasury bill rates on lending rates in the long run. That notwithstanding the unavailability of monthly data on GDP hindered an extensive macro-economic analysis. The interest rate pass-through effect varies depending on the type of interest rate used. It is evidenced from literature, more especially the works of Amidu (Citation2006) that the prime rate and inflation rate were insignificant in affecting lending rate in the long run. Therefore, some level of improvement and benefit has been observed with the use of MPR. In conclusion, efforts geared towards strengthening and creating a vibrant banking and financial system as a whole would further enhance the effectiveness of monetary policy.
5. Recommendations
Loanable funds should be increased through diversification of the financial market alongside encouraging a strong competition amongst bank to make the banking sector healthy. The development of the money and capital market will make the collusive banking theory invalid. Therefore, banks will feel pressured to reduce lending rates when these measures are put in place.
The wide interest rate spread is indicative of inefficiency in the banking sector and the level of development in the financial sector. Therefore, efforts that will be put in place to narrow the margin will ensure the complete pass through effect of the interest rates.
Again, competition in the banking sector encourages banks to take on more diversified risk (i.e. investing in real estate etc.) and make them less fragile to shocks. In order to erode the margin, competition should be encouraged, and legal and regulatory institutions should also be improved.
On the macro level, a reduction in inflation will also reduce the interest rate spread. Boyd et al. (Citation2001) show that countries with high inflation have underdeveloped financial systems and that banks with higher inflation rates are positively associated with net interest margins.
Additional information
Funding
Notes on contributors

Simon Akumbo Eugene Mbilla
Simon Akumbo Eugene Mbilla is a Ghanaian and lectures at the University of Education Winneba. He holds a Master’s Degree in Commerce (Accounting) from University of Cape Coast, Ghana. He is currently pursuing his Ph.D Degree in Accounting from the University of KwaZulu-natal, South Africa.
Peter Akurigo Atindaana
Peter Akurigo Atindaana is a Ghanaian, a chartered accountant and holds a Master’s Degree in Economics from Ghana Institute of Management and Public Administration, Department of Economics, Ghana.
Samuel Gameli Gadzo
Samuel Gameli Gadzo is a senior lecturer at the University of Education Winneba and a chartered accountant. He is currently pursuing his Ph.D Degree in Economics from the University of Cape Coast.
Abiola Adeniyi
Abiola Adeniyi is a Head of Department for Business Studies at Regentropfen College of Applied Science, Bolgatanga. He holds an Mphil Degree in Accounting from KNUST, Ghana.
Idrisu Salifu
Idrisu Salifu is a Ghanaian, He holds a Master’s Degree in Economics and now pursuing his second Master’s Degree in Integrated Coastal Zone Management from University of Cape Coast, Ghana.
The group research covers Economics, Accounting, Finance, Environmental and Natural Resource Management.
References
- Abdul-Hanan, A. (2016). Agricultural credit and technical efficiency in Ghana: Is there a nexus? Agricultural Finance Review, 76(2), 309–16. https://doi.org/10.1108/AFR-01-2016-0002
- Abradu-Otoo, P., Amoah, B., & Bawumia, M. (2003). An investigation of the transmission mechanisms of monetary policy in Ghana: A structural vector error correction analysis. Bank of Ghana, Working Paper, 2
- Adeola, O., & Ikpesu, F. (2016). An empirical investigation of the impact of bank lending on agricultural output in Nigeria: A vector autoregressive (VAR) approach. The Journal of Developing Areas, Special Issue on Dubai Conference Held in April 2016, 50(6), 90–103.
- Adoah, I. (2015). Determinants of universal bank lending rate in Ghana (Doctoral dissertation, University of Ghana)
- African Development Bank Group. (2013). Agricultural value chain financing (AVCF) and development for enhanced export competitveness. African Development Bank.
- Akosah, N. K. (2015). Empirical appraisal of fiscal stability: the case of Ghana. Journal of Economic Studies
- Amidu, M. (2006). The link between monetary policy and banks lending behaviour: the Ghanaian case. Banks & bank systems, 1(4), 38–48
- Aryeetey, E., & Baah-Boateng, W. (2015). Understanding Ghana's growth success story and job creation challenges (No. 2015/140). WIDER Working Paper
- Baoko, G., Acheampong, I. A., & Ibrahim, M. (2017). Determinants of bank credit in Ghana: A bounds-testing cointegration approach. African Review of Economics and Finance, 9(1), 33–61
- Barrett, C. B., Bachke, M. E., Bellemare, M. F., Michelson, H. C., Narayanam, S., & Walker, T. F. (2011). Smallholder participation in contract farming: Comparative evidence from five countries. World Development.
- Begum, I. A., Alam, M. J., Buysee, J., Frija, A., & Huylenbroeck, G. H. (2012). Contract farmer and poultry farm efficiency in Bangladesh: A data envelopment analysis. Applied Economics, 44(28), 3737–3747. https://doi.org/10.1080/00036846.2011.581216
- Bernanke, B., and Blinder, A. (1992). The Federal funds rate and the channels of monetary transmission. American Economic Review, 82, 901–921
- Birthal, P. S., Jha, A. K., Tiongco, M. M., & Narrod, C. (2008). Improving farm-to-market linkages through contract farming: A case study of smallholder dairying in India. International Food Policy Research Institute.
- Bolwig, S., Gibbon, P., & Jones, S. (2009). The economics of smallholder organic contract farming in tropical Africa. World Development, 37(6), 1094–1104. https://doi.org/10.1016/j.worlddev.2008.09.012
- Boyd, J. H., Levine, R., & Smith, B. D. (2001). The impact of inflation on financial sector performance. Journal of monetary Economics, 47(2), 221–248
- Castro, G., & Santos, C. (2010). Bank interest rates and loan determinants. Banco de Portugal Economic Bulletin, 65–86
- Chen, Z. K., Joshi, K. P., Cheng, E., & Birthal, S. P. (2015). Innovations in financing of agri-food value chains in China and India; Lessons and policies for inclusive financing. China Agricultural Economic Review, 7(4), 616–640. https://doi.org/10.1108/CAER-02-2015-0016
- Chow-Lin, A. (1971). Best linear unbiased interpolation, distribution, and extrapolation of time series by related series. The Review of Economics and Statistics, 53(4), 372–375
- Coll, A. A., Santander, E., & Torres, E. (2005). The bank lending channel in Venezuela: Evidencefrom bank level data.
- Demirguc-Kunt, A., Klapper, L., Singer, D., & Oudheusden, P. V. (2015). The global findex database 2014; measuring financial inclusion around the world. Policy Research Working Paper 7255; World Bank Group.
- Denton, F. T. (1971). Adjustment of monthly or quarterly series to annual totals: An approach based on quadratic minimization. Journal of the American Statistical Association, 66, 92–102
- Dorward, A., Kydd, J., & Urey, I. (2003). A policy agenda for pro-poor agricultural growth. World Development, 32(1), 73–89. https://doi.org/10.1016/j.worlddev.2003.06.012
- Eaton, C., & Shepherd, A. W. (2001). Contract farming: Partnerships for growth. FAO Agricultural Services Bulletin 145.
- Engel, R., F., & Granger, C. W. J (1987). Conintegration and error-correction representation, estimation and testing. Econometrics, 55, 251–276
- Essama-Nssah, B. (2006). Propensity score matching and policy impact analysis; A demonstration in E views. World Bank Policy Research Working Paper 3877, 1–59.
- FAO. (2015). Country fact sheet on food and agriculture policy trends. FAPDA.
- Folawewo, A. O., & Tennant, D. (2008). Determinants of interest rate spreads in Sub-Saharan African countries: A dynamic panel analysis. In A paper prepared for the 13th Annual African Econometrics Society Conference (pp. 9–11)
- Fraenkel, J. R., & Wallen, N. E. (2000). How to design and evaluate research in education. McGraw-Hill.
- Garcia, Z. (2006). Agriculture, trade negotiations and gender. The Food and Agriculture Organization.
- Garr, D. K. (2013). Determinants of credit risk in the banking industry of Ghana. Developing Country Studies, 3(11), 64–77
- Giles, D. E. (2013). Autoregressive distributed lag models–part ii–bounds tests. Econometrics Beat
- Guirkinger, C., & Stephen, R. B. (2008). Credit constraints and productivity in Peruvian agriculture. Agricultural Economics, 39(3), 295–308.
- Hanif, N., Iqbal, J., & Malik, J. (2013). Quarterisation of national income accounts of Pakistan
- Havi, E. D. K., & Enu, P. (2014). The effect of fiscal policy and monetary policy on Ghana’s economic growth: which policy is more potent. International Journal of Empirical Finance, 3(2), 61–75
- Hung, L. V., & Pfau, W. D. (2009). VAR analysis of the monetary transmission mechanism in Vietnam. Applied Econometrics and International Development, 9(1), 165–179
- Iyoha, M. A., & Oriakhi, D. (2002). Explaining African economic growth performance: the case of Nigeria. Revised Interim Report on Nigerian Case Study, Prepared for the African Economic Research Consortium Research Project, Explaining African Economic Growth Performance
- Izahr, A., & Tariq, M. (2009). Impact of institutional credit and agricultural production in India during psot reform period. MPRA Paper, 17075.
- Jack, G. A., Carlos, A., & Jaques, H. (2007). Agro-industrial chain management: Concept and applications. Agricultural Management, Marketing and Finance Occasional Paper, Food and Organization of the United Nations.
- Jeong, H.-W. (1995). Liberal economic reform in Ghana:. jstor, 82–104.
- Kalim, R., & Shahbaz, M. (2009). Impact of foreign direct investment on stock market development: the case of Pakistan. In Global Conference on Business and Economics, ISBN (pp. 978–0)
- Kalimangasi, N. N., Kihombo, A., & Kalimangasi, N. (2014). Contribution of contract cocoa production on improving livelihood of smallholder farmers’. International Journal of Scientific and Research Publications, 4(10).
- Kamya, I. R. (2015). Development aid, agricultural value chains and farmers’ benefits: The case of vanilla growers in Kasese, Uganda. EASSRR, 31(1), 1–20.
- Kemal, A. R., & Arby, M. F. (2004). Quarterisation of annual GDP of Pakistan. Pakistan Institute of Development Economics
- Kopparthi, S. M., & Kagabo, N. (2012). Is value chain financing a solution to the problems and challenges of access to finance of small-scale farmers in Rwanda? Managerial Finance, 38(10), 993–1004. https://doi.org/10.1108/03074351211255182
- Kopparthi, S. M., & Kwizera, A. (2016). Impact of agricultural value chain financing on smallholder famers’ livelihoods in Rwanda case study; Rwanda rice value chain. International Journal of Information Research and Review, 3331–3340.
- Kovanen, A. (2011). Monetary policy transmission in Ghana: Does the interest rate channel work?
- Kumar, P. (2006). Contract farming through agribusiness firms and state corporation. Economic and Political Weekly, 41(52), 5367–5375.
- Lartey, L. (2018). The Signalling Effect of Monetary Policy Rate on Lending Rates in Ghana
- Lee, H., & Billington. (1995). The evolution of supply-chain management models ad practice. Hewlett-Packard.
- Matemilola, B. T., Bany-Ariffin, A. N., & Muhtar, F. E. (2015). The impact of monetary policy on bank lending rate in South Africa. Borsa Istanbul Review, 15(1), 53–59
- Miller, C., & Jones, L. (2010). Agricultural value chain finance; Tools and lessons. Food and Agriculture Organization of the United Nations and Practical Action Publishing Ltd.
- Mishkin, F. S. (1995). Symposium on the monetary transmission mechanism. Journal of Economic perspectives, 9(4), 3–10
- Mishkin, F. S. (2007). Housing and the monetary transmission mechanism (No. w13518). National Bureau of Economic Research
- Miyata, S., Minot, N., & Hu, D. (2009). Impact of contract farming on income: Linking small farmers, packers, and supermarkets in China. World Development, 37(11), 1781–1790. https://doi.org/10.1016/j.worlddev.2008.08.025
- Mwambi, M. M., Oduol, J., Mshenga, P., & Mwanarusi, S. (2016). Does contract farming improve smallholder income? The case of avocado farmers in Kenya. Journal of Agribusiness in Developing and Emerging Economies, 6(1), 2–20. https://doi.org/10.1108/JADEE-05-2013-0019
- Nasir, J. (2007, October 17). Downside of informal agricultural credit. Retrieved October 27, 2017, from dawn.com: www.dawn.com
- Nguyen, T. A., Dzator, J., & Nadolny, A. (2015). Does contract farming improve productivity and income of farmers? A review of theory and evidence. The Journal of Developing Areas, 49(6), 531–538. https://doi.org/10.1353/jda.2015.0094
- Oladokun, O. N., Larbani, M., & Mustafa, O. M. (2015). The problems facing the agricultural sector in Nigeria and the prospect of Muzara’ah and supply chain model. Humanomics, 31(1), 18–36. https://doi.org/10.1108/H-11-2012-0022
- Olokoyo, F. O. (2011). Determinants of commercial banks’ lending behavior in Nigeria. International journal of financial research, 2(2), 61–72
- Osinubi, T. S., & Akinyele, A. O. (2006). Commercial bank lending rates and the real sector of the Nigerian economy. The IUP Journal of Bank Management, 3, 27–48.
- Pesaran, M. H., Shin, Y., & Smith, R. J. (2001). Bounds testing approaches to the analysis of level relationships. Journal of applied econometrics, 16(3), 289–326
- Quartey, P., & Afful-Mensah, G. (2014). Financial and monetary policies in Ghana: A review of recent trends. Review of Development Finance, 4(2), 115–125
- Rahji, M., & Adeoti, A. I. (2010). Determinants of agricultural credit rationing by commercial banks in South Western Nigeria. International Research Journal of Finance and Economics, 37, 7–14.
- Rosenbaum, P., & Rubin, D. (1983). The central role of the propensity score in observational studies for causal effects. Biometrika, 70(1), 41–55. https://doi.org/10.1093/biomet/70.1.41
- Saigenji, Y., & Zeller, M. (2009). Effect of contract farming on productivity and income of small holders: The case of tea production in North-western Vietnam. International Association of Agricultural Economists Conference.
- Service, G. S. (2014). Ghana living standards survey round 6. Accra.
- Simmons, P. (2002). Overview of smallholder contract farming in developing countries. ESA Working Paper, FAO.
- Stigler, G. J. (1951). The division of labor is limited by the extent of the market. The Journal of Political Economy, 59(3), 185–193. https://doi.org/10.1086/257075
- Wainaina, P. W., Okello, J. J., & Nzuma, J. (2012). Impact of contract farming on smallholder poultry farmers’ income in Kenya. International Association of Agricultural Economists Triennial Conference.
- Wanjaiya, W. (2015). The impact of monetary policy on bank lending rate in Kenya and the signaling effect on the international investment community (Doctoral dissertation, University of Nairobi)
- Warning, M., & Key, N. (2002). The social performance and distributional consequences of contract farming: An equilibrium analysis of the Arachide de Bouche Program in Senegal. World Development, 30(2), 255–261. https://doi.org/10.1016/S0305-750X(01)00104-8
- World Bank. (2007). Agriculture for development: World development report 2008.