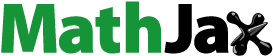
Abstract
Poverty and inequality remain a development challenge for most fishery-dependent households. This has prompted the current interest in the ocean-based economic model as Kenya became the first to host a global conference on the sustainable blue economy which was held in Nairobi in November 2018. To provide a more comprehensive understanding of the challenges surrounding the blue economy approach, we used a fractional response model to analyze drivers of marine fishery dependence. Our case study, involving 384 randomly selected households from Coastal lowlands of Kenya, specifically Kilifi County, revealed that marine fishery is the highest livelihood option exhibiting gender differences. The livelihood participation rates were approximately 68%, 36%, 31%, 25%, and 9% for marine fishery and related activities, agriculture, self-employment (excluding agriculture and marine fishery), wage employment, and remittances respectively. Many livelihood options were pursued independently implying a low level of diversified livelihood strategies in the region. The analysis of the determinants of the marine fishery dependence indicated that education level, agricultural productive assets, access to credit, group membership, security of tenure, flood shock, and fish price shock significantly influenced marine fishery dependence. The study therefore recommends government intervention in marine governance and development programs such as infrastructural development, capital availability and extension services. This will enable the dependent households to diversify to alternative livelihood options and contributes to sustainable marine fishery dependence.
PUBLIC INTEREST STATEMENT
Marine fishery is one of the major economic activities along the coastal region of Kenya. Despite its vast potential to sustaining livelihoods and contributing to national economy, majority of the marine fishery-dependent households are poor. Therefore, it was of great interest to explore factors associated with marine fishery participation. This paper established that participation in marine fishery dependence is influenced by socioeconomic, institutional and climatic factors. To attain sustainable livelihood option among fishing communities the study recommends government intervention in marine resource governance, capital availability, and infrastructural development.
1. Introduction
Marine resources contribute to food security, livelihood, and mitigation of climate change as well as enhanced economic growth through trade (Amevenku et al., Citation2019; Ding et al., Citation2017; Funge‐Smith & Bennett, Citation2019; Satumanatpan & Pollnac, Citation2020). They also serve as the safety net for the poor, providing nutrition and income, particularly in the period of financial hardships. Estimates show that marine resources generate employment for millions of coastal inhabitants and nearly 1 billion people worldwide (Fabinyi et al., Citation2019; Salas et al., Citation2019; Teh & Sumaila, Citation2013). Therefore, the contribution of marine fishery activities to the national economy is inevitable and multifaceted. Apart from improving food security, they contribute to the gross domestic product (GDP) and they are the source of hard currency (FAO, Citation2014).
In Kenya, marine resource generates employment to over 2 million Kenyans through fishing activities, boat building, fish processing, equipment repair, and tourism (CGOK, Citation2018; Muigua, Citation2018). The fishing activities in Kenya’s coastal lowlands are mainly small scale and rely heavily on traditional and simple methods. Also, since the marine fishery-dependent households have low literacy levels and the fishing proceeds are affected by seasons, they are more vulnerable to shocks. The higher vulnerability in fishing-communities results in a poverty-environment trap, which is higher among the poor compared to non-poor (Kiwanuka-Tondo et al., Citation2019; Narain et al., Citation2008; Nguyen et al., Citation2018; Soltani et al., Citation2014). More importantly, marine fishery dependence is under the threat of climatic and idiosyncratic shocks. These include fluctuating prices, changing economic policies, rising sea levels, aridity of the climate, and biological constraints (Somoebwana et al., Citation2021). Therefore, poverty in fishery could be explained by biological limits and natural shocks of the marine fishery resource.
Many of the coastal residents depend on marine resource due to landlessness and insecure land right systems (Van Hoof & Steins, Citation2017). The squatter problem has become a development concern in the coastal region of Kenya, which traces its way back to the colonial administration. It is worth noting that the enactment of the Registration of Documents Ordinance, together with Crown Land Ordinances of 1902 and 1915 redefined crowned land owned by natives. This resulted in a declined community land. With the lack of efficient ownership rights, Africans at the coast remained dispossessed and have been forced to live as tenants at will. Further, individualization of land tenure and other post-independence laws and policies created rural elite phenomenon and intense squatter problem. The land transfer was grounded within the willing seller-willing buyer principle that favored politicians leading to the unfair allocation of land and land grabbing concept of the 1980s (Githunguri, Citation2017). Kenya has begun to embrace the blue economy concept for sustainable development. Therefore, an effective legislative framework that enhances the restoration of an individual’s entitlement to land is inevitable. Through this, marine fishery-dependent households can diversify to alternative livelihood strategies and contribute to a sustainable fishery.
As the Kenyan population increases, the availability of agricultural land struggles to keep up with its demand. As a result, the importance of the marine resource base for sustainable development and income generation for the households to meet their basic needs has increased. This has inspired a wide range of empirical studies in Kenya to understand marine resource opportunities and challenges facing the blue economy approach (Cinner et al., Citation2012; Benkenstein, Citation2018). However, these studies failed to explore fishery dependence and how the shift to blue revolution affects fish biomass concerning both the incidental and target catch. More so, the coastal lowland of Kenya is affected by historical land injustices. Nevertheless, the previous studies failed to take into account the nexus between marine fishery dependence and land tenure. Given the higher incidence of shocks among natural resource-dependent households, over-dependency on marine resources may make households vulnerable to poverty (Somoebwana et al., Citation2021). Therefore, this paper aims at contributing to a better understanding of factors influencing marine fishery dependence, with a special focus on land tenure and shocks. The information obtained is critical to comfort policies pioneered towards promoting inclusive economic growth through sustainable and diversified livelihood options. Further, in natural resource literature, we are not aware of any previous study using the fractional response model (FRM) for analysis of dependency. FRM offered a vital framework for handling the proportional dependent variable and overcoming the observed limitations in linear regression models, Tobit model and non-linear models.
The remainder of the article is structured as follows; the second section presents literature review; the third section presents material and methods for the study; the fourth section presents the discussion of the econometric results, and the fifth section presents the conclusions and policy implications of the study.
2. Literature review
This section presents a review of the past research and findings on the determinants of marine fishery dependence. Degen et al. (Citation2010) and Cinner et al. (Citation2012) found that fishers’ number has increased gradually in Kenya. This is because of the poverty that is linked to poor education, inadequate employment opportunities, lack of access to credit, poor agro-ecological conditions, and unfavourable climate. In this sense, failure to diversify to alternative livelihood options is usually observed especially in regions where there is marginalization and lack of social protection of the fishing communities. This implies that weak institutional framework to capacitate the rural livelihoods may be the missing link towards diversified and sustainable livelihood options (Cinner et al., Citation2012). Further, Daw et al. (Citation2012) established that failure to exit marine fishery is attributed to the occupational attachment, ethnicity, household size, education, age and attitudinal factors.
A growing body of empirical literature suggests that natural resource dependence is associated with socio-economic characteristics, distance to the extracting ground, and asset value (Amevenku et al., Citation2019; Nguyen et al., Citation2015, Citation2018; Tamale et al., Citation2017). In addition, market access, financial capital, access to health, and membership to formal or informal institutions are likely to affect the dependence on these resources (Béné & Friend, Citation2011; Soltani et al., Citation2012). Hence, given climate variability, institutional bottlenecks, inadequate infrastructure, and land degradation (Neiland & Béné, Citation2013), the households may be attracted to the fishery resource as a result of both the short-term and long-term shocks. These conditions create a downward spiral of overexploitation, which leads to poverty, and poverty results in overexploitation (Cinner et al., Citation2012; Somoebwana et al., Citation2021; Stanford et al., Citation2013).
3. Materials and methods
3.1. Description of the study area
The study was carried out in Kilifi County, which is among the six counties in Kenya’s coastal region. Kilifi County lies in the East and South of the Indian Ocean and Mombasa County, respectively (). It is characterized by a bimodal rainfall pattern averaging between 300 mm and 1,300 mm. The main topographic features of the County include coastal plain, foot plateau, coastal range, and Nyika plateau (CGOK, Citation2013). With poor agro-ecological zones and massive blue economy investment potential arising from the Indian Ocean coastline of 265 km, fishing and tourism have emerged as the dominant economic activities in the area. Most importantly, the marine fishery dependence has been long linked to the Bajun ethnic group that is being regarded as the fishers’ par excellence. However, since the 1960s, Mijikenda has engaged in this economic activity (Degen et al., Citation2010). This is due to a significant level of poverty-related to historical injustices of land and social exclusion. Therefore, in an effort to improve the welfare of the dependent households, studying the dimensions of the marine fishery dependence in the County is vital. This will help in formulating appropriate policy options that take into account socio-economic constraints, land tenure, and multiple shocks observed. As a result, the wealth generated from fishery can contribute to rural development through fishing proceeds and employment multiplier effects due to the cash crop nature of fish (Béné et al., Citation2007).
3.2. Research design, sampling, and data management
The cross-sectional research design was used in this study to explore the drivers of marine fishery dependence among households in Kilifi County. Apart from enabling assessment of the link between covariates and the outcome, this approach allowed a simultaneous comparison of different variables in the study sample and is relatively faster and inexpensive.
The selection of the respondents for this study was arrived at using a multistage sampling technique. Kilifi County was purposively selected in stage one due to its large Indian Ocean coastline, the higher rate of historical land injustices in the region, and significant poverty level (CGOK, Citation2018). The second stage involved a purposive sampling method of four Sub-Counties (Malindi, Kilifi North, Magarini, and Kilifi South) since they are located along the Indian Ocean. Later four wards were purposively selected from the four Sub-Counties (Shella-Malindi, Watamu-Kilifi North, Gongoni-Magarini, and Shimolatewa-KilifiSouth) from a population of 35 because they offer important ground for artisanal fishing. In the last stage, simple random sampling was used to select 384 households spread over the 4 wards () (Somoebwana et al., Citation2021). The household sample size was determined usingEquationEq. (1(1)
(1) (Cochran, Citation1977)
Table 1. Distribution of sample size
where is the minimum estimated sample size, z is the value of the t-distribution, which equals to the alpha of 0.05 = 1.96, p represents population proportion estimate, and e is the margin of error. When the population proportion is unknown, p is put at 0.5 and e at 0.05 (Cochran, Citation1977).
The primary data were collected through face–face interviews with the household heads. However, this was after the questionnaire was subjected to a pre testing procedure to determine its validity and competency. We ensured quality control in data by carefully selecting and training the enumerators to equip them with knowledge sufficient to portray the research objectives. More so, to avoid biased data collection process, respondents who took part in the pre-test exercise were considered ineligible in the actual household survey. Further, the descriptive and fractional response models were applied in this study to gain clear insights into the dimensions of marine fishery dependence (Chegere, Citation2018). Data were analyzed using Stata econometric software.
3.3. Marine fishery dependence
The level of marine fishery dependence of a particular household was expressed as the proportion of the income derived from the ocean fishery and related (fishing, fish trading and processing, boatbuilding, and selling of fish equipment) to the total household income, as described in EquationEq. (2)(2)
(2) .
where Y* is the marine fishery dependence ranging between 0 and 1 with a higher value indicating high dependence (Nguyen et al., Citation2018). The numerator represents household income generated from marine fishery and related activities, while the denominator reflects total household income; (net income from crop production, livestock rearing, fishery and related activities, business), wages, salary, and remittances (Mathenge et al., Citation2010).
3.4. Analytical framework
In determining the factors influencing marine fishery dependence, the present study used a fractional response model (FRM). Most importantly, FRM was preferred to linear regression models such as Ordinary Least Square (OLS) because they are not fit to estimate fractional dependent variables and, therefore, may produce estimates outside the unit interval (Chegere, Citation2018). The non-linear models such as logit and probit transformations may be applicable in this case; however, they do not consider observations that lie at the boundaries and hence may result in truncation problem. Further, the Tobit model may seem appropriate for bounded dependent variables; however, in proportional data, the values outside the unit interval are not feasible. Therefore, FRM remains the best model for handling the proportional dependent variable and to overcoming the observed limitations in other econometric models. According to Papke and Wooldridge (Citation1996), the FRM model is a synthesis and an extension of quasi-maximum-likelihood (QML) methods and generalized linear models (GLM) as described below
where yi is the dependent variable defined as (0 ≤ yi ≤ 1), xi represents explanatory variables for household i, and G(.) is the logistic regression function that will be estimated directly using QML based on Bernoulli log-likelihood function defined as
Given Bernoulli distribution is a linear, exponential family (LEF), the estimator of QML, θ will be given by
Therefore, θ is normally distributed, and the method can generate consistent estimates of the fractional response variable. The variables in the fraction response model are presented in from the review of past studies;
Table 2. Variables used in the fractional response model
4. Results and discussion
4.1. Descriptive statistics on marine fishery dependency
The total number of households that participated in marine fishery and related activities was approximately 68%, about 36% pursued agriculture, and 31% participated in self-employment (non-farm and non-fishery). Further, approximately 25% of the households participated in wage employment (non-farm and non-fishery), while households with income from remittances were about 9%. In terms of the level of dependency, marine fishery and related activities, agriculture, self-employment, wage employment, and remittances had about 0.63, 0.02, 0.12, 0.19, and 0.01, respectively. Diversification is a critical approach to ensure a consistent flow of income. However, most of the households who participated in marine fishery and related activities find it difficult to find the next best diversification livelihood option due to insecure land ownership rights and lower education levels. This is clearly indicated by a higher dependence on marine fishery compared to other livelihood options.
The willingness to pursue additional livelihood options among the households was low. Cultural preference and the time-consuming nature of the fishing activities hampered the households to practice diversified strategies. Most importantly, the dependency on marine fishery extends beyond personal income and employment. The conducted interviews suggest that there is a working culture in this particular livelihood strategy defined by reciprocity and interpersonal relationships, where individuals engage in marine fishery through social ties, and form a significant part of their identity. Additionally, most of the households that were dependent on the ocean fishery were Bajuns and were culturally bounded by self-employment. They felt that marine fishery provided a sufficient form of self-employment and did not like wage employment due to too much control from employers. These elements offered a critical pathway in the survival and growth of the fishing industry in the coastal region (Carter & Garaway, Citation2014). With the government at the heart of transforming the sector and organizing beach management units (BMUs), reciprocal interdependencies have become more evident.
Further, gender disaggregated analysis on the livelihood options was conducted. Results indicated that marine fishery and related activities is a male dominated livelihood option with males taking the largest share at 79.69% while females at 20.31%. This could have been attributed to the cultural belief that fishery suits males since it requires higher strength. Males were also more likely to engage in wage employment than females at 78.57% and 21.43%, respectively. Further, the participation rate of males and females in self-employment was 81.51% and 18.49% accordingly. The higher likelihood of males to engage in wage employment and self-employment is because of their exposure to education and entrepreneurial behaviors than females who for a long time have been marginalized. However, it is worth mentioning that females were more likely to participate in agriculture at 79.14% compared to males at 20.86. This is because of higher tendency of females to provide unpaid farm labor and ensuring food security for households.
4.2. Descriptive statistics of the variables used in the econometric model
shows descriptive statistics for the dependents and non-dependents households in the marine fishery for continuous variables. There are significant differences with regard to some of the covariates. On average, dependent households have a lower level of education at a mean of about 7.9 compared to 12.5 for non-dependent households. Majority of the dependent households tend not to value education and usually drop out of school because marine fishery serves as an immediate and direct source of income (Ndhlovu et al., Citation2017; Nguyen et al., Citation2018), which reduces the individual’s incentive to pursue further education for future employment.
Table 3a. Socioeconomic characteristics of households stratified by participation in marine fishery (continuous variables)
Participating households in marine fishery and related activities have significantly fewer agricultural productive assets averaging KES 10,417.2 compared to non-participating households, KES 71,321.3 as can be seen in . Asset poverty usually compels individuals to diversify into common pool resources (Nguyen et al., Citation2020), and as such, they pursue the livelihood option as a safety net in response to idiosyncratic and climatic shocks. Further, there are significant differences with respect to distance to the marine and distance to the fishery market. Households that are closer to the ocean and market tend to engage more in the marine fishery and related activities due to social identity and reduction in transaction costs. On the other hand, an increase in the distance lowers the expected net economic value, which ultimately discourages the decision to participate (Kyando et al., Citation2019).
reports findings for the socioeconomic characteristics of households stratified by participation in marine fishery for categorical variables. The results indicate that there are also significant differences in credit access. Households that have poor access to credit are more likely to participate in marine fishery and related activities. The logic follows that lack of access to credit limits capital availability and investment in entrepreneurial activities, making common pool resource only viable livelihood option for the affected households. This can be further affirmed by lower proportion of security of tenure for the participating households, 36%, preventing the right to land usage, loan acquisition and long-term investment. Further, group membership was higher among the participating households compared to those that do not pursue the livelihood option at about 86% and 4%, respectively. Participation in local institutions affects the decision to participate in fishery resource due to peer influence and awareness of economic surplus extracted from the commons.
Table 3b. Socioeconomic characteristics of households stratified by participation in marine fishery (categorical variables)
The differences in shocks relating to rainfall, flood, and price are also significant between the two groups. Price uncertainty and the fluctuation nature of the marine fishery returns due to seasons and climatic conditions expose these households to financial and weather shocks. In response to these shocks, households usually take advantage of their right of opportunity-freedom of the commons by increasing fishing activities (Jentoft et al., Citation2010). In such instances, coping strategies such as adopting illegal gears, exploiting threatened and protected species, or extending fishing periods are deployed. This results in overexploitation, which exposes poor households to heavy risks given that majority of the dependent households do not enjoy the insurance offered by land ownership against a sudden loss of livelihood option. Efforts have been put to organize the marine fishery under beach management units (BMU) with the primary objective of achieving capacity building and reduce sensitivity to shocks through information sharing and provision of marketing facilities. However, more than half of the conducted interviews publicly criticized BMUs due to their failure to mobilize resources and instead focusing on restrictive fishing regulations that are not even equally implemented.
4.3. Econometric analysis of the factors influencing marine fishery dependence
To determine the factors influencing marine fishery dependence, the fractional response model was estimated. The results are presented in . The Akaike information criterion (AIC) and Bayesian information criterion (BIC) were 208 and 271, respectively. The likelihood ratio test of the model had a p-value of less than 0.001, indicating a better fit (Shin & McCann, Citation2018). Pseudo R2 of 65% was also higher compared to the set statistical threshold of 20%, and hence marine fishery dependence is well explained by the proposed covariates (Power et al., Citation2015). The result in indicates that several factors significantly influenced the level of marine fishery dependence among households. They include education level, group membership, credit, the security of tenure, flood, fish price, and value of agricultural productive assets.
Table 4. Fractional response model results on factors influencing marine fishery dependence
The education level of the household head was negatively associated with marine fishery dependence at 1% significant level. Lower education level limits opportunity in formal employment and fishing provides a livelihood option as it does not require higher levels of education. This finding is consistent with previous studies (Amevenku et al., Citation2019; Daw et al., Citation2012; Garekae et al., Citation2017; Selig et al., Citation2019), where education had a negative effect on both relative and absolute environmental income attributed to higher education opening up alternative employment opportunities in public and private sector jobs. Moreover, education also enhances income diversification and labour diversity (Do et al., Citation2019; Jin et al., Citation2018), since it enriches access to information and skills and hence off fishery-related employment opportunities.
The amount of agricultural productive assets had a positive and significant effect on the marine fishery dependence at 5% significant level. The results have been attributed to the majority of the households diversifying into agricultural production. However, agriculture was pursued in this region, mainly for subsistence with pockets of commercialized agricultural production. This is to serve as a coping strategy against the perceived risk and a livelihood diversification approach in sustaining consumption. The finding is consistent with prior evidence (Ndhlovu et al., Citation2017; Nguyen et al., Citation2018), where a positive relationship between asset values and natural resource dependence implies an attempt by the households to build adaptive capacity. This is because the fishery is associated with a variety of disruptions, which could result in fluctuations in the income derived. Therefore, increased asset value is critical as a coping strategy and in sustaining consumption among dependent households.
Group membership in agricultural-related activities positively and significantly influenced the level of marine fishery dependence at 1% significant level. Beach management units (BMU) are the main groups in the region related to agriculture and, therefore, positively influenced marine fishery dependence. The reason for this is that beach management units are directly related to marine fishery, and its membership is critical in providing information, skills, knowledge, fishing gears, and market resources that enable fishers to be more efficient. Additionally, membership to beach management units enhanced repeated social cohesion and peer influence, which influences members’ behaviour to participate in common economic activity (Alexander et al., Citation2018).
Access to credit negatively and significantly influenced marine fishery dependence at 5% significant level. Credit access increases financial resources, reduces cash constraint, and enhances participation in off-fishery investment opportunities, reducing dependence on marine fishery. The results are in line with the work of Kimengsi et al. (Citation2019) and Amevenku et al. (Citation2019), who found that lack of access to credit increases dependence on natural resources. The reason for this is that access to credit prompts households to pursue more lucrative livelihood strategies that are not labour intensive. It has been established that households that have efficient access to credit tend to promote nonfarm activities rather than increasing investment in fishing (Amevenku et al., Citation2019). Similarly, Kleih et al. (Citation2013) reported that small-scale and medium actors in the fishery sector in Egypt have poor access to credit due to the information gap and lack of suitable collateral.
Security tenure of land negatively and significantly influenced the level of marine fishery dependence at 10% significant level. Ceteris paribus, the security of tenure, decreases marine fishery dependence by the probability of 0.5424%. Land ownership right prompts households to invest in agriculture and off-fishery employment opportunities. Security of tenure provides the right to usage, which encourages individuals to engage in entrepreneurial livelihood strategies outside the marine fishery such as business investment and intensive agricultural production. Another possible explanation relates to the land and squatter issue facing the coastal community. The problem was contributed by the colonial era, non-recognition of the land tenure security system, and hence dispossession of indigenous people by successive regimes. Despite the enactment of the 2010 constitution, the squatter problem continues to persist due to a lack of independent enforcement mechanisms and political goodwill (Githunguri, Citation2017; Klopp & Lumumba, Citation2017). The political destabilizing effect in land reforms in Kenya’s coastal region has increased land inequality among households. As a result, local individuals are exposed to land-related violence, social division, and impeded economic growth, which has subsequently enhanced dependence on natural livelihood options. The result is consistent with the work of Teshager Abeje et al. (Citation2019), who established that dependence on one particular livelihood option is significantly influenced by insecure land ownership rights.
Marine fishery dependence was further influenced by shocks related to flood at 10% significant level. The results indicated that households that experienced floods have a higher likelihood of dependence on marine fishery and related activities. This is particularly true in Malindi during the rainy season when river Sabaki moves into the Indian Ocean. Mixing of waterfronts with different density raises the sea level and result in high and strong tides disrupting fishing activities. Similar findings were found by Nguyen et al. (Citation2018), who founded that households that are dependent on natural resources have faced a significantly higher number of shocks, such as floods. Ndhlovu et al. (Citation2017) argued that the small-scale sector is vulnerable to climate change and through flooding events and fluctuating water levels.
Further, Price shock had a positive and significant effect on marine fishery dependence at 1% significant level. The reason for this is due to the seasonality nature of the livelihood option. Marine fishery catches depend on seasons with kusi season, which occurs from April to July, being the poorest. During this period, rough seas and heavy rainfall hamper the supply of fish and subsequently result in a higher price. On the contrary, kaskaz season, which occurs from August to March, is suitable for fishing activities since the ocean is relatively calm. However, due to the higher supply of fish, price decreases, which ultimately reduces both absolute and relative income from marine fishery and related activities. The findings indicated how the vicious cycle between weather shocks and price uncertainty results in natural resource dependence. Given credit constrain, majority of the households in the region used risk-sharing strategies such as advance payment to cope with flood events and price fluctuation in the fishing industry (Somoebwana et al., Citation2021).
5. Conclusion and policy implications
This study examined the drivers of marine fishery dependence among households in the coastal lowlands of Kenya using the fractional response model. The key factors influencing marine fishery dependence were education level, access to credit, security of tenure, group membership, agricultural productive assets, flood shock, and fish price shock. Given that poor households mainly depend on marine fishery and related activities there is the need for government intervention in non-formal education programs, financial support, beach management units, and infrastructural development. Public policies built around these aspects will enable adequate marine conservation, good financial management, loan acquisition, and entrepreneurial behaviours. As a result, the dependent households will be able to diversify to alternative livelihood options that will subsequently increase their adaptive capacity and achievement of the blue economy approach.
Further, efficient land right system is considered critical for sustainable livelihood options. Slow adjudication has been observed to jeopardize settlement programs that have subsequently contributed to insecure land tenure in the Country. Therefore, the establishment of the administrative and legal framework that addresses historical claims and regions with squatter problems is critical to solving the landlessness problem. More generally, reducing or alleviating chronic poverty in fishery requires public services, social protection, and enhancing of individual and collective assets. This implies that more comprehensive governance option remains significant for responsible fishery and improving household welfare in the coastal lowlands of Kenya.
This study can be extended to other regions in Kenya to allow for generalization of the research findings for effective policy recommendation critical to contribute to the achievement of blue economy approach. Areas for further research include determining factors influencing vulnerability of the coastal fishing communities and evaluating the effect of migrant fishers on local fishers’ income and wellbeing.
Acknowledgements
The authors would like to sincerely thank the African Economic Research Consortium (AERC) for funding this research work. They would also like to acknowledge the chairpersons of Beach management units and residents of Kilifi County for guidance, support, and cooperation during data collection.
Additional information
Funding
Notes on contributors

Mohamed Idris Somoebwana
Mohamed Idris Somoebwana is a student at Egerton University, Kenya pursuing Master of Science degree in Agricultural and Applied Economics. He is a beneficiary of the African Economic Research Consortium (AERC) scholarship and shared facility program occurred between September and December 2018 in the University of Pretoria, South Africa. His research areas include rural development, environmental economics, agricultural marketing, climate change, food security and nutrition, agricultural project analysis and agricultural innovation. This study provides information on the factors influencing participation on marine fishery and related activities. Its aim is to provide effective policy recommendations for building sustainable livelihood options among fishing communities.
References
- Alexander, S., Bodin, Ö., & Barnes, M. (2018). Untangling the drivers of community cohesion in small-scale fisheries. International Journal of the Commons, 12(1), 519–15. https://doi.org/10.18352/ijc.843
- Amevenku, F. K. Y., Asravor, R. K., & Kuwornu, J. K. M. (2019). Determinants of livelihood strategies of fishing households in the Volta Basin, Ghana. Cogent Economics & Finance, 7(1), 1595291. https://doi.org/10.1080/23322039.2019.1595291
- Béné, C., & Friend, R. M. (2011). Poverty in small-scale fisheries: Old issue, new analysis. Progress in Development Studies, 11(2), 119–144. https://doi.org/10.1177/146499341001100203
- Béné, C., Macfadyen, G., & Allison, E. H. (2007). Increasing the contribution of small-scale fisheries to poverty alleviation and food security ( No. 481). Food & Agriculture Organization.
- Benkenstein, A. (2018). Prospects for the Kenyan blue economy, 1–9. Retrieved from https://www.africaportal.org/publications/prospects-kenyan-blue-economy/
- Carter, C., & Garaway, C. (2014). Shifting tides, complex lives: The dynamics of fishing and tourism livelihoods on the Kenyan coast. Society & Natural Resources, 27(6), 573–587. https://doi.org/10.1080/08941920.2013.842277
- CGOK. (2013). First Kilifi county integrated development plan 2013–2017, finance and economic planning, County Government of Kilifi.
- CGOK. (2018). Kilifi county integrated development plan 2018–2022, economic planning and finance, County Government of Kilifi.
- Chegere, M. J. (2018). Post-harvest losses reduction by small-scale maize farmers: The role of handling practices. Food Policy, 77(2018), 103–115. https://doi.org/10.1016/j.foodpol.2018.05.001
- Cinner, J. E., McClanahan, T. R., Graham, N. A., Daw, T. M., Maina, J., Stead, S. M., Wamukota, A., Brown, K., & Bodin, Ö. (2012). Vulnerability of coastal communities to key impacts of climate change on coral reef fisheries. Global Environmental Change, 22(1), 12–20. https://doi.org/10.1016/j.gloenvcha.2011.09.018
- Cochran, W. G. (1977). Sampling Techniques. John Wiley & Sons.
- Daw, T. M., Cinner, J. E., McClanahan, T. R., Brown, K., Stead, S. M., Graham, N. A., & Maina, J. (2012). To fish or not to fish: Factors at multiple scales affecting artisanal fishers’ readiness to exit a declining fishery. PloS One, 7(2), e31460. https://doi.org/10.1371/journal.pone.0031460
- Degen, A., Hoorweg, J., & Wangila, B. C. (2010). Fish traders in artisanal fisheries on the Kenyan coast. Journal of Enterprising Communities: People and Places in the Global Economy, 4(4), 296–311. https://doi.org/10.1108/17506201011086101
- Ding, Q., Chen, X., Hilborn, R., & Chen, Y. (2017). Vulnerability to impacts of climate change on marine fisheries and food security. Marine Policy, 83(2017), 55–61. https://doi.org/10.1016/j.marpol.2017.05.011
- Do, T. L., Nguyen, T. T., & Grote, U. (2019). Nonfarm employment and household food security: Evidence from panel data for Rural Cambodia. Food Security, 11(3), 703–718. https://doi.org/10.1007/s12571-019-00929-8
- Fabinyi, M., Dressler, W., & Pido, M. (2019). Access to fisheries in the maritime frontier of palawan province, Philippines. Singapore Journal of Tropical Geography, 40(1), 92–110. https://doi.org/10.1111/sjtg.12260
- FAO. (2014). The state of food and agriculture 2014. Rome: FAO. Available online at http://www.fao.org/3/a-i3720e.pdf. Accessed on 25 march 2019.
- Funge‐Smith, S., & Bennett, A. (2019). A fresh look at inland fisheries and their role in food security and livelihoods. Fish and Fisheries, 20(6), 1176–1195. https://doi.org/10.1111/faf.12403
- Garekae, H., Thakadu, O. T., & Lepetu, J. (2017). Socio-economic factors influencing household forest dependency in Chobe Enclave, Botswana. Ecological Processes, 6(1), 40. https://doi.org/10.1186/s13717-017-0107-3
- Githunguri, F. W. (2017). Evaluation of the mandate of the relevant institutions in addressing the squatter problem in Kenya (Doctoral dissertation, Strathmore University). Available online at https://su-plus.strathmore.edu/bitstream/handle/11071/5224/Evaluation%20of%20the.%20mandate%20of%20the%20relevant%20institutions.pdf?sequence=1&isAllowed=y. Accessed on 25 march 2019.
- Jentoft, S., Onyango, P., & Islam, M. M. (2010). Freedom and poverty in the fishery commons. International Journal of the Commons, 4(1), 1. https://doi.org/10.18352/ijc.157
- Jin, S., Waibel, H., Min, S., & Huang, J. (2018). Livelihood responses of smallholder farmers in southwest china to the decline in rubber prices. In 30th International Conference of Agricultural Economists Vancoucer, USA, 1-26.
- Kimengsi, J. N., Pretzsch, J., Kechia, M. A., & Ongolo, S. (2019). Measuring livelihood diversification and forest conservation choices: Insights from Rural Cameroon. Forests 2019, 10(81), 1–16. https://doi.org/10.3390/f10020081.
- Kiwanuka-Tondo, J., Semazzi, F., Pettiway, K., Casadevall, S. R., & Ricart Casadevall, S. (2019). Climate risk communication of navigation safety and climate conditions over lake victoria basin: Exploring perceptions and knowledge of indigenous communities. Cogent Social Sciences, 5(1), 1588485. https://doi.org/10.1080/23311886.2019.1588485
- Kleih, U., Linton, J., Marr, A., Mactaggart, M., Naziri, D., & Orchard, J. E. (2013). Financial services for small and medium-scale aquaculture and fisheries producers. Marine Policy, 37(2013), 106–114. https://doi.org/10.1016/j.marpol.2012.04.006
- Klopp, J. M., & Lumumba, O. (2017). Reform and counter-reform in Kenya’s land governance. Review of African Political Economy, 44(154), 577–594. https://doi.org/10.1080/03056244.2017.1367919
- Kyando, M. T., Nyahongo, J. W., Røskaft, E., & Nielsen, M. R. (2019). Household reliance on environmental income in the Western Serengeti Ecosystem, Tanzania. Environment and Natural Resources Research, 9(1), 54–63. https://doi.org/10.5539/enrr.v9n1p54
- Mathenge, M., Place, F., Olwande, J., & Mithoefer, D. (2010). Participation in agricultural markets among the poor and marginalized: Analysis of factors influencing participation and impacts on income and poverty in Kenya. Unpublished Study Report Prepared for the FORD Foundation.
- Muigua, K. (2018). Harnessing the blue economy: challenges and opportunities for Kenya. kmco.co.ke. Available at http://kmco.co.ke/wp-content/uploads/2018/12/Harnessing-the-Blue-Economy-Challenges-and-Opportunities-for-Kenya-24th-December-2018.pdf. Accessed on 22 March 2019.
- Narain, U., Gupta, S., & Van’t Veld, K. (2008). Poverty and resource dependence in Rural India. Ecological Economics, 66(1), 161–170. https://doi.org/10.1016/j.ecolecon.2007.08.021
- Ndhlovu, N., Saito, O., Djalante, R., & Yagi, N. (2017). Assessing the sensitivity of small-scale fishery groups to climate change in Lake Kariba, Zimbabwe. Sustainability, 9(12), 2209. https://doi.org/10.3390/su9122209
- Neiland, A. E., & Béné, C. (Eds.). (2013). Poverty and small-scale fisheries in West Africa. Springer Science & Business Media.
- Nguyen, T. T., Do, T. L., Bühler, D., Hartje, R., & Grote, U. (2015). Rural livelihoods and environmental resource dependence in Cambodia. Ecological Economics, 120(2015), 282–295. https://doi.org/10.1016/j.ecolecon.2015.11.001
- Nguyen, T. T., Do, T. L., & Grote, U. (2018). Natural resource extraction and household welfare in Rural Laos. Land Degradation & Development, 29(9), 3029–3038. https://doi.org/10.1002/ldr.3056
- Nguyen, T. T., Nguyen, T. T., & Grote, U. (2020). Multiple shocks and households’ choice of coping strategies in Rural Cambodia. Ecological Economics, 167(2020), 106442. https://doi.org/10.1016/j.ecolecon.2019.106442
- Papke, L. E., & Wooldridge, J. M. (1996). Econometric methods for fractional response variables with an application to 401 (k) plan participation rates. Journal of Applied Econometrics, 11(6), 619–632. https://doi.org/10.1002/(SICI)1099-1255(199611)11:6<619::AID-JAE418>3.0.CO;2-1
- Power, R. A., Steinberg, S., Bjornsdottir, G., Rietveld, C. A., Abdellaoui, A., Nivard, M. M., & Cesarini, D. (2015). Polygenic risk scores for schizophrenia and bipolar disorder predict creativity. Nature Neuroscience, 18(7), 953–955. https://doi.org/10.1038/nn.4040
- Salas, S., Chuenpagdee, R., & Barragán-Paladines, M. J. (2019). Drivers and prospects for the sustainability and viability of small-scale fisheries in Latin America and the Caribbean. In Viability and sustainability of small-scale fisheries in Latin America and The Caribbean, 87(1) (pp. 543–559). Cham.
- Satumanatpan, S., & Pollnac, R. (2020). Resilience of small-scale fishers to declining fisheries in the Gulf of Thailand. Coastal Management, 48(1), 1–22. https://doi.org/10.1080/08920753.2020.1689769
- Selig, E. R., Hole, D. G., Allison, E. H., Arkema, K. K., McKinnon, M. C., Chu, J., & Ingram, J. C. (2019). Mapping global human dependence on marine ecosystems. Conservation Letters, 12(2), e12617. https://doi.org/10.1111/conl.12617
- Shin, D. W., & McCann, L. (2018). Analyzing differences among non-adopters of residential stormwater management practices. Landscape and Urban Planning, 178(2018), 238–247. https://doi.org/10.1016/j.landurbplan.2018.06.003
- Soltani, A., Angelsen, A., & Eid, T. (2014). Poverty, forest dependence and forest degradation links: Evidence from Zagros, Iran. Environment and Development Economics, 19(5), 607–630. https://doi.org/10.1017/S1355770X13000648
- Soltani, A., Angelsen, A., Eid, T., Naieni, M. S. N., & Shamekhi, T. (2012). Poverty, sustainability, and household livelihood strategies in Zagros, Iran. Ecological Economics, 79(2012), 60–70. https://doi.org/10.1016/j.ecolecon.2012.04.019
- Somoebwana, M. I., Ayuya, O. I., & Mironga, J. M. (2021). Marine fishery dependence, poverty and inequality nexus along the coastal lowlands of Kenya. National Accounting Review, 3(2), 152–178. https://doi.org/10.3934/NAR.2021008
- Stanford, R. J., Wiryawan, B., Bengen, D. G., Febriamansyah, R., & Haluan, J. (2013). Exploring fisheries dependency and its relationship to poverty: A case study of West Sumatra, Indonesia. Ocean & Coastal Management, 84(2013), 140–152. https://doi.org/10.1016/j.ocecoaman.2013.08.010
- Tamale, A., Ejobi, F., Muyanja, C., Naigaga, I., Nakavuma, J., Drago, C. K., & Amulen, D. R. (2017). Sociocultural factors associated with fish consumption in lake albert fishing community: Guidelines for lead and mercury. Cogent Environmental Science, 3(1), 1304604. https://doi.org/10.1080/23311843.2017.1304604
- Teh, L. C., & Sumaila, U. R. (2013). Contribution of marine fisheries to worldwide employment. Fish and Fisheries, 14(1), 77–88. https://doi.org/10.1111/j.1467-2979.2011.00450.x
- Teshager Abeje, M., Tsunekawa, A., Adgo, E., Haregeweyn, N., Nigussie, Z., Ayalew, Z., & Berihun, D. (2019). Exploring drivers of livelihood diversification and its effect on adoption of sustainable land management practices in the Upper Blue Nile Basin, Ethiopia. Sustainability, 11(10), 2991. https://doi.org/10.3390/su11102991
- Van Hoof, L., & Steins, N. A. (2017). Mission report kenya: Scoping mission marine fisheries Kenya (No. C038/17). Wageningen Marine Research.