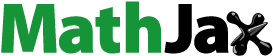
Abstract
Laying the ground for the agricultural sector to derive development is critical and urgent in relieving majority of the poor out of poverty trap. This in turn calls the modernization of the agriculture sector through agricultural technology adoption. The major objective of the study is to assess the perception and determinants of agricultural technology adoption in North Shoa zone, Amhara regional state, Ethiopia. Data were collected from 796 farming households from four districts namely, Angolela Tera, Menz Gera, Minijar Shenkora and Moretna Jiru. For analysis purpose, t-test and binomial logistic model was employed. The result indicates that the likelihood of adopting improved seed, chemical fertilizer and irrigation is higher among households with higher age, greater years of schooling, large farm size, large livestock ownership and many extension contacts. Additionally, the likelihood of adopting these agricultural technologies is higher for household participating in non-farm income generating activities, having membership status in various social group and having access to credit. The likelihood of adopting the prevailing agricultural technologies also found higher for male- headed households as compared to female-headed ones. Distance to the nearest market also negatively and significantly affects the decision to adopt various agricultural technologies. The study suggested that the awareness of farmers concerning the available agricultural technologies should be raised through membership of different social group and frequent extension contact. On the other, promoting farmers to participate in off-farm income generation activities and creating access to credit service can reduce the financial constraint in purchasing and possessing new agricultural technologies.
PUBLIC INTEREST STATEMENT
Capitalizing the agriculture sector through the adoption of new agricultural technology to drive development is both critically important and urgent for enhancing aggregate economic growth and improving the welfare of millions of extremely poor people especially in developing countries like Ethiopia. The decision to adopt a given or a combination of agricultural technologies affected by household specific, socio-economic, technology and institutional factors. However, there are a limited number of studies concerning the perception and determinants of agricultural technology adoption in Ethiopia. In this vein, this study tried to assess the perception and determinants of agricultural technology adoption in North Shoa Zone, Amhara Regional State, Ethiopia. The study helps policy makers to come up with more plausible policy options leading to improvement in agricultural technology adoption among rural households.
1. Introduction
Ensuring agricultural development is one of the vents through which developing countries can escape from the vicious circle of poverty. In predominantly agrarian developing countries like Ethiopia in which the contribution of agriculture to GDP is more than 40% and 85% to national employment (Dercon et al., Citation2012), there is no a mere solution than modernizing the agriculture sector. Therefore, sustaining the growth of the agricultural sector should be one of the central agenda in the developmental policy of Ethiopia and in order to ensure the fruits of development to be percolated to the grass root levels, a pronounced priority must be given to the development of the agricultural sector.
However, agricultural development should involve a process of transformation from traditionally oriented rural cultural towards an acceptance and reliance on science and technology mainly through the adoption of improved, scale-appropriate and ecofriendly technologies (Mottaleb, Citation2018). As such in most developing countries adoption of new agricultural technologies are preconditions for sustained improvements in the levels of agricultural output and productivity. Increased output in earlier years was achieved without the need for new technology simply by extending cultivation into unused but potentially productive lands. Almost all of these opportunities have by now been exploited, and there is little scope for further significant or sustainable expansion (Todaro & Smith, Citation2012). Therefore, actualizing a sustained development in the agricultural sector calls the need for new agricultural technology adoption.
The decision to adopt new agricultural technology can be affected by a number of factors such as household-specific factors (sex, age, education status, household size, farming experience), socio-economic factors (farm size, cost of adopting new agricultural technology, off-farm income, tropical livestock unit), technology factors i.e., the characteristics of the new technology, and institutional factors (membership of social group, access to extension service, access to credit, distance from the nearest market (Berhanu, Citation2018; Obayelu et al., Citation2017).
A number of studies has been conducted concerning the perception and determinants of agricultural technology adoption in Ethiopia for example, (Challa & Tilahun, Citation2014; Feyisa, Citation2020; Gebru, Citation2006; Milkias & Abdulahi, Citation2018). The above studies heavily rely in showing a single agricultural technology adoption decision and fails to account complementarity of agricultural technology adoption decision in establishing a causal effect relationship. According to (Abay et al., Citation2016) such kind of studies are often prone to methodological challenges related to the nature of adoption decisions, and hence may produce biased estimates.
Concerning the perception and determinants of agricultural technology adoption a number studies were undertaken. In this regard, a number of factors were identified as critical factors affecting the decision to adopt agricultural technology in many studies and showed inconsistent result. For instance, a number of studies reported that the decision to adopt a certain agricultural technology is highly likely among male-headed rural households as compared to their female counterpart (Amare & Simane, Citation2017; Launio et al., Citation2018; Solomon et al., Citation2014). On the contrary, the study by (Simtowe et al., Citation2016) showed the likelihood of adopting at least one variety of Psigeonpea variety diminishes with being a male farmer in Malawi. On the other hand, other group of studies found that sex of the household head was not statistically significant in influencing the decision to adopt agricultural technology (Challa & Tilahun, Citation2014; Feyisa, Citation2020; Gebru, Citation2006; Ponguane & Mucavele, Citation2018; Simtowe et al., Citation2011). The same is true for other determinants of agricultural technology adoption. Therefore, existence of inconsistent results on the determinants of agricultural technology adoption among rural households calls the need for further study on the isssue in order to come up with a better policy option targeting to improve the decision to adopt new agricultural technology among rural households.
In general, capitalizing the agriculture sector through the adoption of new agricultural technology to drive development is both critically important and urgent for enhancing aggregate economic growth and improving the welfare of millions of extremely poor people. Incognizant of this, the study is targeted towards assessing the perception and determinants of agricultural technology adoption in four districts of North Shoa, Amhara Regional State, Ethiopia. Unlike most studies on the determinants of agricultural technology adoption, which heavily rely on a single agricultural technology adoption decision, the application of a multiple agricultural technology adoption model is the main contribution of this study.
The study was organized in to five parts in which the first part of the study deals with introduction; the second part, deals with review of the related literature; the third part, deals with methods and materials; the fourth part, deals with result and discussion and the final part of the study deals with conclusion, recommendation and directions for future research.
2. Literature review
2.1. Agricultural technology adoption
The decision whether to adopt a given agricultural technology is not an overnight phenomenon. The adoption process of the new agricultural technology starts with the awareness of the adopter about the existence of the specific technology. In the next phase, the potential adopter analyzes the information about the new technology and potential adopter gets to understand the attributes of a technology further. In the third place, the potential adopter will make a trial or experimentation before adopting the technology. Based on perceived benefits of the technology, the individual goes through the fourth stage, which involves the actual technology adoption. Once the technology is adopted, the adopter may decide to continue using it or discontinue depending on the experience and benefits after adoption (Simtowe et al., Citation2016).
2.2. Determinants of agricultural technology adoption
A number of studies has been carried out on the determinants of agricultural technology adoption. Understanding the factors affecting the decision to adopt explicitly is vital to bring a profound change in the development of the agricultural sector and livelihood of the farm household. Additionally, understanding of the factors affecting a range of agricultural technology is crucial for development practitioners working on developing the agricultural sector and producers entangled in the production of agricultural technologies (Hall and Khan, Citation2003). In this study, following (Mwangi & Kariuki, Citation2015) the literature on the determinants of agricultural technology will be reviewed using the household-specific factors, socio-economic factors, technology factors and institutional factors as categories.
2.2.1. Householdspecific factors
Among household-specific factors the sex of the household head is one of the most widely used variable of interest in agricultural technology adoption. A review of literature on factors affecting adoption of new agricultural technologies in Ethiopia by (Berhanu, Citation2018), asserted that since the prevailing social set up of rural households placed a varying responsibility among male and female members, in most parts of rural Ethiopia women are disfavored groups of the society who couldn’t easily access technology information. As such, in many studies for instance, (it is obvious to find that being a female-headed household negatively affect the decision to adopt agricultural technology. The study on factors influencing adoption of selected peanut protection and production technologies in Northern Luzon, Philippines by (Launio et al., Citation2018) found sex of the household statistically significant in influencing the use of chemical fertilizer and pesticides in peanut farms. Male farmers are more likely use chemical fertilizers and pesticides than their female counterparts. In the same study, sex of the household is found to have no significant influence on the use of seed inoculant and seed treatment. Similarly, Solomon et al. (Citation2014) adoption of improved wheat varieties in robe and Digelu Tijo districts of Arsi zone in Oromia region, Ethiopia using a double hurdle approach also confirmed as sex have significant influence on the adoption of improved wheat variety. But, the study on the determinants of improved Pigeonpea adoption under partial population awareness in Malawi by (Simtowe et al., Citation2016) showed that the probability of adopting at least one improved Pigeonpea variety diminishes with being a male farmer. On the other hand, (Challa & Tilahun, Citation2014; Feyisa, Citation2020; Gebru, Citation2006; Ponguane & Mucavele, Citation2018; Simtowe et al., Citation2011) found as sex of the household head has no significant influence on the decision to adopt agricultural technology.
The other important household-specific variable capable of influencing agricultural technology adoption is age of the household head. Knowledge acquired and farming experience are highly tied with age of the farming household head. As such older farmers are better to evaluate the pros and cons of agricultural technology as compared to younger farmers. In this regard, (Beshir, Citation2014) found age of the farm household head is positively related to the probability of adoption of forage technology in North East highlands of Ethiopia. Feyisa (Citation2020) in his meta analysis concerning the determinants of agricultural technology in Ethiopia also found age of the household head have positive influence on farmer’s decision to adopt new/improved agricultural technology since older households might have better awareness about the benefit of new agricultural technologies from their life experiences. On the contrary, farmer’s age may negatively influence the adoption of agricultural technologies. It may be that older farmers are more risk averse and less willing to access information about new technologies since older farmers may incur higher search costs for the new technologies; hence lack information on the existence of new and improved agricultural technologies (Awotide et al., Citation2014; Milkias & Abdulahi, Citation2018; Simtowe et al., Citation2016).
Education status of the household is one of the most appealing variables in agricultural technology adoption studies. In many studies, education status of farmers has been assumed to have a positive influence on farmers’ decision to adopt new technology since education level of a farmer increases his ability to obtain, process and use information relevant to adoption of a new technology (Mwangi & Kariuki, Citation2015). For instance, (Abay et al., Citation2016; Challa & Tilahun, Citation2014; Simtowe, Citation2011; Simtowe et al., Citation2011) found that the education status of farmers increases the likelihood of adopting agricultural technology. This may be because advance in education status make farmers to be cautious in analyzing the cost and benefits of adopting new technology. In other study by (Ramaano, Citation2021) indicate that the higher the level of educational attainment, the greater the capacity of any member of a community to contribute productively to ordained policy and strategies designed to promote community development. That means education can serve as a spring board in changing the perception of farmers toward changes leading to overall development.
Household size which is mostly used as a proxy to labor availability. In a situation where the adoption of the agricultural technology places labor constraint during adoption process, households having large family size are advantageous. In this regard, household size is found to have positive significant influence on the adoption of inorganic fertilizer in Niger (Djibo & Maman, Citation2019). In contradict the study by Challa and Tilahun (Citation2014) in Ethiopia west Wollega district, showed that family size has a negative influence on the technology adoption of the households. This is because in case where the monetary outlay of adopting the new technology is high and most of the family members income heavily comes from the household head and one source, the likelihood of adopting the technology will diminish for households with large family size.
2.2.2. Socio-economic factors
Among the socio-economic factors, farm size plays a paramount role in adoption process of agricultural technologies. Some of the studies showed that farm size has positive influence on adoption decision. For instance, Milkias and Abdulahi (Citation2018) studied determinants of adoption of improved highland maize varieties in Toke Kutaye district, Oromia Regional State, Ethiopia and found a significant positive effect. Plenty of studies have found a positive and significant relation between farm size and agricultural technology adoption. Yigezu et al. (Citation2018) in their study on the adoption of zero tillage among wheat and barley producers in Syria found that the relative acreage of farmland dedicated to wheat production is key determinant of duration to adopt rather than the mere total farm acreage. Accordingly, farmers with large wheat acreage are likely to adopt zero tillage earlier relative to those with small wheat areas. Ponguane and Mucavele (Citation2018) in their study on the determinants of agricultural technology adoption in Chókwè district, Mozambique found that farm size had a significant and positive effect on improved seed and mechanization adoption. (Abay et al., Citation2016) in their study concerning understanding farmers’ technology adoption decisions with regard to input complementarity and heterogeneity in Ethiopia covering around 7500 farm households visited twice in two rounds (2011 and 2013) found that households with large farm size have higher propensity to adopt fertilizer, improved seed, and extension services, potentially because of economies of scale and risk aversion. Concomitantly, Langyintuo and Mekuria (2008) and Cavane et al. (Citation2013) reported that increase in farm size increases the likelihood of a household in adopting agricultural technologies because farmers with large farm size possession benefit from economies of scale and are market-oriented.
The cost of adopting new agricultural technology is one of the key variables in constraining the process of agricultural technology adoption. Obviously, in many studies high cost of agricultural technology places a great barrier to adoption. The study done by Djibo and Maman (Citation2019) on the determinants of agricultural technology adoption in Niger reported that high price of agricultural inputs place a negative effect on improved seed adoption and positive effect on inrganic fertilizer use. Challa and Tilahun (Citation2014) in their study on the determinants and impacts of modern agricultural technology adoption in west Wollega, Gulliso district, Ethiopia found that the household heads attitudes towards the fairness of the cost of inputs; that is the more the farmers think that cost of inputs (improved seed and fertilizers) as fair, the more they adopted the technology.
Farmers generating off-farm income is more likely to become adopter of modern agricultural technologies than the one who do not participate in off-farm income-generating activities. This may be due to the fact that off-farm income can be a vent through, which farmers come out of credit constraint in purchasing agricultural technologies in many developing countries. Ponguane and Mucavele (Citation2018) reported that farmers with other sources of income are more likely to adopt both mechanization and improved seed in Mozambique since farmers with off-farm can surpass financial constraints needed to purchase these agricultural technologies. Challa and Tilahun (Citation2014) also reported that having incomes other than farming activities has strong positive role on the decision of households in accepting new agricultural technologies.
2.2.3. Technology factors
The characteristics of the new technology is the most compelling in the process of agricultural technology adoption. The associated attributes of a technology are a precondition of adopting agricultural technology (Mwangi & Kariuki, Citation2015). (Doss, Citation2003)) also bolded the importance of characteristics of agricultural technology in making trial on a small scale first before adopting agricultural technologies completely. Mignouna et al. (Citation2011) in studying determinants of adopting Imazapyr-Resistant Maize (IRM) technology in western Kenya, argued that farmers who perceive the technology being consistent with their needs and compatible to their environment are likely to adopt since they find it as a positive investment.
2.2.4. Institutional factors
Belongingness to a particular social group is very important in a situation where information asymmetry concerning various agricultural technologies is evident since it paves the way for farmers to share information and learn from each other (Feyisa, Citation2020; Ketema et al., Citation2016). Additionally, Simtowe et al. (Citation2016), found that membership in social/faith group has a negative significant effect on adoption decision whereas membership in farmers club do not have a significant influence in the process of agricultural technology adoption.
Access to extension services is one of the institutional factors in technology adoption decision. Through informed consent about the existence, the use and benefit of new agricultural technology through extension agents’ farmers can make a decision in adopting agricultural technologies. In this regard, the positive effect of frequency of contacts with extension agents in raising the likelihood of adopting new agricultural technologies is propagated in many studies for instance, (Mignouna et al., Citation2011; Milkias & Abdulahi, Citation2018). Besides increasing the probability of acquiring up-to-date information on the new agricultural technologies, extension agents or agricultural development assistants can counter balance the negative effect of lack of years of formal education in the overall decision to adopt some technologies in many developing countries (Banabana-Wabbi, Citation2002).
Access to credit has been considered as one of the important variables of interest in agricultural technology adoption. Consistent with the economic constraint paradigm of adoption models Simtowe et al. (Citation2016) in their study about adoption of improved Pigeonpea in Malawi found that household that borrowed some money from the lending institution have higher propensity of adopting improved varieties of pigeonpea. Like off-farm income access to credit can somehow relieve the financial restraint imposed on farmers in purchasing agricultural technologies. In this regard, studies in Ethiopia done by (Abay et al., Citation2016; Feyisa, Citation2020; Milkias & Abdulahi, Citation2018) asserted that access to credit can counterbalance the financial constraint of farmers in purchasing agricultural technologies.
Distance from the nearest market has an enormous effect in agricultural technology adoption decision and marketing agricultural yield. Amare and Simane (Citation2017) found that distance from the nearest market has negative impact on the likelihood of choosing different agronomic practices and generalize that adoption of different technologies is booming in areas with developed rural infrastructure and markets and also where commercial agriculture prevails. Many studies in agricultural technology adoption have indicated a significant and negative effect of distance from the nearest market on farmers decision to adopt agricultural technology (Milkias & Abdulahi, Citation2018; Hagos & Zemedu, Citation2015). Contrarily, Feyisa (Citation2020) in his meta analysis concerning agrcultural technology adoption in Ethiopia found distance from market to have a significant and positive effect on the farmers’ decision to adopt agricultural technology.
3. Methods and materials
3.1. The study area
The study was undertaken in North Shoa Zone, Amhara Regional State, Ethiopia. North Shoa Zone (district), is among the 11 administrative districts of the Amhara region, where the capital city of the region, Bahir Dar, is based. The zone consists of 27 districts, and it has a wide range of cultures, agro-ecological diversities, resource endowments, dominant agricultural farming systems and other off-farm activities. According to the CSA estimates in 2019, the North Shoa has a total population of 2,263,097 whom 48.7% are females. From the total population, 81.2% is living in the rural area and 18.8% in urban areas. The total area of the district is nearly 17,347.19 square kilometers. Around 47.14% of the land is used for cultivation, 5.49% for grazing, 14.13% for plantation and the rest 33.24% is used for other purposes. The topology of the district covers 38.86% flat land, 25.89% mountains, 11.85% valley and 23.4% rugged (uneven ground). The altitude of the district ranges from 937 to 4000 meters above sea level. The district constitutes four agro-climatic zones namely Dega (32.02%), Woinadega (45.58%), Kola (21.95%) and Wrch/Frost (0.46%) (North Shoa Zone Agriculture and Rural Development Office, 2020).
3.2. Target population and sampling technique
The study was geared towards investigating the perception and determinants of agriculture technology adoption in case of North Shoa district, Amhara Regional State, Ethiopia. A multi-stage sampling technique was employed to collect data. Initially, in order to address the study objective out of twenty-seven districts within the zone four woredas (districts) namely, Angolela Tera, Menz Gera, Minijar Shenkora and Moretna Jiru were selected and a total sample of 796 was taken.
3.3. Conceptual modeling of households agricultural technology adoption decision
Following (Rahm & Huffman, Citation1984; Rosenbaum & Rubin, Citation1983; Wooldridge, Citation2001) farmers’ adoption decisions on varieties of agricultural technology are assumed to be based upon utility maximization. Define the varietal technologies by j, where for adoption of at least one agricultural technology and
otherwise. The non-observable underlying utility function, which ranks the preference of the
farmer is given by
. Thus, the utility derivable from the varietal technology depends on
, which is a vector of farm household-specific factors, socio-economic factors and institutional factors and technology factors i.e. attributes/characteristics associated with the agricultural technology. Although the utility function is unobserved, the relation between the utility derivable from a
agricultural technology is postulated to be a function of the vector of observed household-specific factors (sex, age, marital status, education status, household size, farming experience), socio-economic factors (farm size, cost of adopting new agricultural technology, off-farm income, tropical livestock unit), and institutional factors (membership of social group, access to extension service, access to credit, distance from the nearest market, and the technology factors i.e. specific characteristics of the agricultural technology and a disturbance term:
Since the utilities derived from adopting agricultural technologies by farm household is random, the farmer will adopt the specific agricultural technology or a bundle of agricultural technologies
or if the non-observable (latent) random variable
. The probability that
equals one i.e. the farmer adopts the specific agricultural technology or a bundle of agricultural technologies is a function of the vector of farm household-specific factors, socio-economic factors and institutional factors and technology factors:
Then, explicitly the probability of the farmer adopts the specific agricultural technology or a bundle of agricultural technologies is:
where X is vector of the explanatory variables i.e. farm household-specific factors, socio-economic factors and institutional factors and technology factors, and β is a
vector of parameters to be estimated,
is a probability function,
is error term, and
is the cumulative distribution function for
evaluated at
. The probability that a farmer will adopt the specific agricultural technology or a bundle of agricultural technologies is a function of the vector of explanatory variables and a vector of unknown population parameters and error term. Practically, without knowing the form of F, Equationequation (2)
(2)
(2) cannot be estimated directly, and it is the distribution of
that determines the distribution of F. If
is normal, F will have a cumulative normal distribution.
Setting to be the logistic distribution or assuming that
follows the logistic distribution gives rise to the logit model. The logistic distribution function is given by:
Here, the response probability i.e. the farmer adopts the specific agricultural technology or a bundle of agricultural technologies is evaluated as:
Similarly, the non-response probability i.e. the farmer does not adopt new agricultural technology is evaluated as:
Note that the response and non-response probabilities both lie in the interval , and hence, are interpretable.
The odds ratio for the logit model becomes:
is the ratio of the odds of . The natural logarithm of the odds (log odds) is:
Thus, the log-odds is a linear function of a vector of explanatory variables i.e. vector of farm household-specific factors, socio-economic factors and institutional factors and technology factors. The above transformation has certainly helped the popularity of the logit model. In order to estimate the logit model, maximum likelihood estimation technique is applied.
3.4. Measurement and definition of variables
The dependent variable takes the two values i.e. 1 for farmers adopting specific agricultural technology or a bundle of agricultural technologies and 0 for farmers who do not adopt adopting specific agricultural technology or a mix of agricultural technology.
The existing literature on agricultural technology adoption provides a wide range of factors influencing the process of adopting agricultural technology. Following the review made by (Berhanu, Citation2018; Obayelu et al., Citation2017) concerning the determinants of agricultural technology adoption and following the in-depth literature review made by the researchers for the purpose of this study, the decision to adopt specific agricultural technology or a bundle of agricultural technologies modeled as a function of household specific factors (sex of the of the HH head, age of the HH head, education status of the HH head, household size and farming experience of the HH head), socio-economic factors (farm size, participation in off-farm income generating activities and livestock owned by the HH) and institutional factors (membership of social group/agricultural cooperatives, access to extension service, access to credit and distance from the nearest market). The description of the potential explanatory variables, which are expected to influence the adoption of a specific agricultural technology or a mix of agricultural technologies with their respective expected sign is provided in .
Table 1. Definition and description of variables
4. Results and discussion
4.1. Agricultural technology adoption decision across sampled districts
indicates that among the prevailing agricultural technologies in this particular study adoption of chemical fertilizer takes the lion share in the adoption decision of the sampled households followed by improved seed and mix of improved seed and chemical fertilizer. Among the sampled districts in Menz Gera district do not adopt irrigation at all during 2019 cropping season. This situation directly related to the topography of the district especially the sampled villages, which is difficult to use irrigation. The decision of adopting a mix of agricultural technologies during cropping season is less prevalent in the study area. This may be due to the financial constraint faced by farming households in purchasing and possessing the prevailing agricultural technologies examined in this study.
Table 2. Agricultural technology adoption status across districts
4.2. Continuous variables by a single agricultural technology adoption status
4.2.1. Improved seed adoption status
depicts improved seed adoption status of farmers. Out of 796 farmers surveyed in the study, 344 (43.22%) of them adopt improved seed and the rest 452 (56.78%) do not adopt improved seed during 2019 cropping season. The average years of schooling for household heads is almost four years. The statistical test showed that there is a statistically significant difference among improved seed adopting households as compared to the non-adopters in terms of their years of schooling (p-value < 0.01). This signifies improving education status of households can improve the likelihood of adopting agricultural technologies since education can raise awareness and enhance the decision-making capability of in adapting new agricultural technologies. On the other hand, the average household size is 5.06 for improved seed adopters and 4.85 for the non-adopters. At 1% level of significance, household size for farmers adopting improved seed has a statistically significant difference as compared to farmers who do not adopt. The number of livestock owned measured using tropical livestock unit (TLU), have a statistical difference among adopters and non-adopters at 5% level of significance. Improved seed adopting households have larger livestock ownership (6.84 TLU) than the non-adopting households (5.74 TLU). Membership of in social grouping is higher among improved seed adopters (93.02%) as compared to non-adopters (81.64%) and showed a statistically significant difference at 1% level of significance. Access to market is measured by distance from the nearest market and found to be 9.37 km on average. Improved seed adopting households have the shorted distance to nearest market (2.31 km) as compared to non-adopters (14.75 km) and the difference is statistically significant at 1% level of significance. This implicates the existence of inverse relation between distance to nearest market and improved seed adoption decision of households.
Table 3. Characteristics of improved seed adoption status
4.2.2. Chemical fertilizer adoption status
Concerning chemical fertilizer adoption status as depicted in showed that 542(68.22%) adopt chemical fertilizer. Age of the household head, household size, years of farming experience of the household head, livestock owned is higher among chemical fertilizer adopting households and showed a statistically significant difference among adopters and non-adopters at 1% level of significance. Additionally, adoption of chemical fertilizer among farmers participating in off-farm income generating activities and among farmers with social membership status is higher among adopters as compared to non-adopters and it significant at 1% level of significance. Like improved seed, chemical fertilizer adopting households have the shorted distance to nearest market (7.40 km) as compared to non-adopters (13.60 km) and the difference is statistically significant at 1% level of significance.
Table 4. Characteristics of chemical fertilizer adoption status
4.2.3. Irrigation adoption status
According to , dealing irrigation adoption status of the sampled households, majority of the households 612(84.42%) did no adopt irrigation during 2019 cropping season. Around 90% of the households were male-headed and the proportion of male-headed households was higher among irrigation adopters (94.57%) as compared to the non-adopters’ sub-sample (88.24%). The test statistics indicate that there is significant difference among male and female-headed farmers in terms of irrigation adoption. Male-headed households have greater tendency of adopting irrigation as compared to the female-headed ones. Household size and livestock owned is higher among irrigation adopting households and showed a statistically significant difference among adopters and non-adopters at 1% level of significance. Surprisingly, frequency of extension contact is higher among irrigation non-adopting households (1.05 times per month) as compared to the adopting households (0.92 times per month). Like the other agricultural technologies’, shows the existence of inverse relation between distance to nearest market and irrigation adoption decision of households.
Table 5. Characteristics of irrigation adoption status
4.3. Determinants of adoption of agricultural technologies
According to , sex was found statistically significant in influencing the adoption of a mix of agricultural technologies i.e. a mix of improved seed and chemical fertilizer, a mix improved seed and irrigation and a mix of improved seed, chemical fertilizer and irrigation adoption at 5% levels of significance, respectively. Accordingly, the respective odds-ratio for sex of the household head in adopting the two mix of agricultural technologies are in favour of male-headed households. In this regard, male-headed farming households about 2.044 times more likely adopt a mix of improved seed and chemical fertilizer as compared to female-headed households. Again, the likelihood of adopting a mix of improved seed, chemical fertilizer and irrigation among male- headed households is about 5.294 times higher than female-headed households. The lower probability of adopting technologies among female-headed households may be attributed due the cultural and social barriers that limits females from access to land, information concerning a variety of agricultural technologies and their exposure much on non-agricultural activities. The finding of this study is in line with the study done by (Amare & Simane, Citation2017; Launio et al., Citation2018; Solomon et al., Citation2014).
Table 6. Odds-ratio (OR) on determinants of adoption of agricultural technology
The age of the household is statistically significant in influencing the household’s decision to adopt improved seed at 1% level of significance. The odds ratio for the age of the household head is 1.017 implying that as the age of the household head increases, the odds of adopting improved seed increases. The possible reason as to why older farmers adopt improved seed as compared to young farmers may be due to their resource endowment and experience even though their age limits them in searching information about various agricultural technologies. Similar studies (Beshir, Citation2014; Feyisa, Citation2020; Simtowe et al., Citation2016) were also found consistent with finding of this study.
Another critical factor influencing the adoption of agricultural technologies is education status of the farming household head measured in terms of years of schooling. Education is found statistically significant in affecting the adoption of small-scale irrigation at 5% level of significance. The likelihood of households in adopting irrigation increase with an increase in years of education. The possible reason for this may be due to the capability of education in raising the awareness and information processing of farmers about new agricultural technologies. Farmers having more years of schooling do not face difficulty in getting and processing information beside their ability in making thoughtful evaluation about new agricultural technologies than farmers with less years of schooling. The ease of adopting new technology is also higher among households with more years of schooling. This result is consistent with the findings of (Abay et al., Citation2016; Amare & Simane, Citation2017; Challa & Tilahun, Citation2014; Feyisa, Citation2020).
As one of the most appealing variables in agricultural technology adoption literature, farm size plays an indispensable role in the process of adopting new agricultural technology. Among the seven alternative agricultural technologies options in five of them farm size poses a negative and statistically significant effect in adoption decision at 1% and 5% level of significance. The likelihood of adopting new technology decreases in response to increase in land size. In a situation where financial constraint is greater, households with large farm size loose their appetite to invest in new agricultural technologies. Having small land size may also raise the incentive to adopt technology as means of boosting agricultural yield. This will be more appealing in situation where the new agricultural technology is land saving. Negative influence of farm size on technology adoption is also found in some studies done by (Amare & Simane, Citation2017; Deressa et al., Citation2010).
Participation in off-farm income generating activities is found the other important variable influencing the adoption of agricultural technologies. According to the logistic regression result, household’s participation in off-farm income generating activities have about 3.232, 1.818, 2.926 and 1.771 times more likely adopt irrigation, mix of improved seed and irrigation, mix of chemical fertilizer and irrigation and mix of improved seed, chemical fertilizer and irrigation technologies respectively as compared to household’s who do not participate in off-farm income generating activities. When there exist a number of income generating activities side to farming, the likelihood of adopting new technologies increase since this additional income from off-farm income generating activities can be used to purchase or possess the new technology. Similar to the finding of the study (Challa & Tilahun, Citation2014) also confirmed that households who participate in off-farm income-generating activities have a greater possibility to become adopters of new agricultural technology than households who do not participate in income-generating activities out-side farming.
Livestock ownership as a proxy for measuring wealth or household asset possession measured using TLU found to have positive and significant effect on the decision to adopt the prevailing agricultural technologies in this particular study. The likelihood of adopting technology is higher for households owning large livestock unit as compared to households owning small livestock unit. This is because households having large livestock unit will have better financial stand to afford and possess new agricultural technologies. In conformity, the study by (Abay et al., Citation2016; Feyisa, Citation2020) found the same result.
Membership within different social groups resulted a positive and statistically significant effect in adopting all agricultural technologies raised in the study indicating membership in different social groups raise the probability of adopting. Societal bond through membership of social groups can bridge the information asymmetry and reduces the cost of searching information concerning new technology. In a nutshell membership within social groups reduces the cost of acquisition of information about a new technology and enables farmers to learn the existence as well as the effective use of technology and this in turn promotes its adoption. Additionally, since farmers will only adopt the technology, they are aware of or have heard of it, access to information via membership within different social groups reduces the uncertainty about a technology’s performance hence may change individual’s assessment from purely subjective to objective over time (Banabana-Wabbi, Citation2002). Similar finding is obtained by (Feyisa, Citation2020).
Among the possible options that farming households used to curb down their financial constraint is credit. Providing access to credit can raise the likelihood of adopting agricultural technologies for households falling short of finance to purchase and possess new agricultural technologies. According to the above regression result access to credit is positive and statistically significant in affecting the decision to adopt chemical fertilizer at the 5% level of significance. The likelihood of adopting chemical fertilizer is higher for households with access to credit as compared to the one who do not have access. A number of studies also support this finding (Feyisa, Citation2020; Milkias & Abdulahi, Citation2018; Simtowe et al., Citation2016; Udima et al., Citation2017).
Distance to nearest market found to be negative and statistically significant in influencing the adoption of the prevailing agricultural technologies within the study. The likelihood of adopting various technologies is higher for households closer to the nearest market place as compared to households who are far away. The finding of the study match with (Beshir, Citation2014; Milkias & Abdulahi, Citation2018).
5. Conclusion, policy implication and direction for future research
5.1. Conclusion
Ensuring agricultural development is one of the vents through which developing countries can escape from the vicious circle of poverty. Therefore, actualizing a sustained development in the agricultural sector calls the need for agricultural technology adoption. This paper analyzes the perception and determinants of agricultural technology adoption in North Shoa zone, Amhara Regional State, Ethiopia. The collected data revealed that the decision to adopt the agricultural technologies namely improved seed, chemical fertilizer, irrigation and combination of these agricultural technologies in the study area were affected by household-specific factors (sex, age, education status, household size, farming experience), socio-economic factors (farm size, off-farm income, tropical livestock unit), and institutional factors (membership of social group, access to extension service, access to credit, distance from the nearest market). As such, the importance of adopting various agricultural technology is reinforced by the indispensable role of the agricultural sector in terms of employment, output and export along with its significant role in poverty reduction. Thus, for the agricultural sector to perform well, promoting the adoption of various agricultural technologies and relieving the various constraints binding farmers from adopting agricultural technologies is one of the vents through which growth within the agricultural sector is actualized.
5.2. Policy implication
The study suggested that in raising the awareness of farmers in adopting various agricultural technologies membership in different social group and frequent extension agents contact should be promoted. Promoting farmers to participate in off-farm income generation activities and creating access to credit service can reduce the financial constraint in purchasing and possessing new agricultural technologies. Thus, it is highly important for policy makers to ensure that farmers have access to credit in order to improve the pace of the adoption of various agricultural technologies. Producers or developers of various agricultural technologies should understand the ability and willingness of farmers in order to develop agricultural technologies that match the needs of them. Improving the educational status of farmers or bridging the knowledge gap of farmers through frequent extension contact should be taken in to account as another means to raise the rate of adoption of agricultural technology. Lastly, as the findings shows difference across each explanatory variables in different adoption decisions, policy makers should consider disaggregated interventions in this regard.
5.3. Direction for future research
In this study, a number of factors were identified in determining the decision to adopt agricultural technology but, in reality we may come across with many variables determining the decision to adopt various agricultural technologies. Thus, future researchers can introduce additional explanatory variables that may affect the decision to adopt. Additionally, undertaking a comparative study on the determinants of agricultural technologies can also be an another area of interest for future researchers.
Notes of Contribution
Solomon Estifanos, Tadesse Zenebe, Wudineh Ayalew and Markew Mengiste generated the idea, designed the study and collected the data. Solomon carried out the data analysis and write-up. Tadesse, Wudineh and Markew read, revised and approved the final manuscript.
Acknowledgements
The authors of this study wish to express their deepest gratitude to Debre Berhan University for its financial support in undertaking this study. It is our condolence to thank Debre Berhan University community who contributed a lot for the accomplishment of this study in one or another way. The authors would like to thank the anonymous reviewers and editors for their thoughtful and unreserved insights.
Disclosure Statement
The authors declare that they have no competing interest.
Additional information
Funding
Notes on contributors

Solomon Estifanos Massresha
Solomon Estifanos is an Economist. Currently, he is a lecturer at Debre Berhan University, Department of Economics and works as a private consultant and manager at Mesale Consultancy Service PLC based at Debre Berhan, Ethiopia. He obtained his MSc and BA degrees from Jimma University and Haramaya University, respectively. His research areas include poverty, food security, agricultural technology adoption, institutional quality, investment, environmental management and urbanization.
References
- Abay, K. A., Berhane, G., Taffesse, A. S., Koru, B., & Abay, K. (2016). Understanding Farmers’ Technology Adoption Decisions: Input Complementarity and Heterogeneity. ESSP Working Paper 82,Ethiopian Strategy Support Program (ESSP), Ethiopian Development Research Institute (EDRI), International Food Policy Research Institute (IFPRI).
- Amare, A., & Simane, B. (2017). Determinants of Smallholder Farmers’ Decision to Adopt Adaptation Options to Climate Change and Variability in the Muger Sub-basin of Upper Blue Nile Basin of Ethiopia. Agriculture & Food Security, 6(1), 64. https://doi.org/10.1186/s40066-017-0144-2
- Awotide, B., Abdoulaye, T., Alene, A., & Manyong, V. (2014). Assessing the extent and Determinants of doption of Improved Cassava Varieties in South-West Nigeria. Journal of Development and Agricultural Economics, 6(9), 376–19. https://doi.org/10.5897/JDAE2014.0559
- Banabana-Wabbi, J. (2002). Assessing Factors Affecting Adoption of Agricultural Technologies: The Csae of Integrated Pest Management (IPM) in Kumi District. MSc Thesis, Uganda.
- Berhanu, M. (2018). A Review on Factors Affecting Adoption of Agricultural New Technologies in Ethiopia. Journal of Agricultural Science and Food Research], 9, 3. https://www.longdom.org/open-access/a-review-on-factors-affecting-adoption-of-agricultural-new-technologiesin-ethiopia.pdf
- Beshir, H. (2014). Factors Affecting the Adoption and Intensity of Use of Improved Forages in North East Highlands of Ethiopia. American Journal of Experimental Agriculture, 4(1), 12–27. https://doi.org/10.9734/AJEA/2014/5481
- Cavane, E. A. P., Cugara, B., & Jorge, A. (2013). Adopção de tecnologias agrárias em Moçambique: Revisão, interpretação e síntese de estudos feitos. Projecto de investigação financiado pelo OMR. Maputo
- Challa, M., & Tilahun, U. (2014). Determinants and Impacts of Modern Agricultural Technology Adoption in West Wollega: The Case of Gulliso District. 4, 20. https://www.iiste.org/Journals/index.php/JBAH/article/view/15700/16097
- Dercon, S., Hoddinott, J., & Woldehanna, T. (2012). Growth and Cronic Poverty: Evidence from rural Communities in Ethiopia. Journal of Developement Studies, 48(2), 238–253. https://doi.org/10.1080/00220388.2011.625410
- Deressa, T., Hassan, R., & Ringler, C. (2010). Perception of and Adoption to Climate Change by Farmers in the Nile Basin of Ethiopia. Journal of Agricultural Science, 50(1), 1–9. https://doi.org/10.1017/S0021859610000687
- Djibo, O., & Maman, N. M. (2019). Determinants of Agricultural Technology Adoption: Farm Households Evidence from Niger. Journal of Development and Agricultural Economics, 11(1), 15-23. https://doi.org/10.5897/JDAE2018.0998
- Doss, C. R. (2003). Understanding Farm Level Technology Adoption: Lessons Learned from CIMMYT’s Microsurveys in Eastern Africa CIMMYT. Economic Working Paper 03–07.
- Feyisa, B. W. (2020). Determinants of Agricultural Technology Adoption in Ethiopia: A Meta Analysis. Cogent Food & Agriculture, 6(1), 1. https://doi.org/10.1080/23311932.2020.1855817
- Gebru, A. (2006). The Determinants of Modern Agricultural Inputs Adoption and Their Productivity in Ethiopia: The Case of Amhara and Tigray Regions. Unpublished Thesis Submitted to the School of Graduate Studies of Addis Ababa University.
- Hagos, A., & Zemedu, L. (2015). Determinants of Improved Rice Varieties Adoption in Fogera District of Ethiopia. Science, Technology and Arts Research Journal, 4(1), 221–228. https://doi.org/10.4314/star.v4i1.35
- Hall, B. H., & Khan, B. (2003). Adoption of new technology. doi:10.3386/w9730
- Ketema, M., Kebede, D., Dechassa, N., & Hundessa, F. (2016). Determinants of Adoption of Potato Production Technology Package by Smallholder Farmers: Evidences from Eastern Ethiopia. Review of Agricultural and Applied Economics, 19(2), 61–68. https://doi.org/10.15414/raae.2016.19.02.61-68
- Launio, C. C., Luis, J. S., & Angeles, Y. B. (2018). Factors Influencing Adoption of Selected Peanut Protection and Production Technologies in Northern Luzon, Philippines. Technology in Society, 55, 56–62. https://doi.org/10.1016/j.techsoc.2018.05.007
- Mignouna, B., Manyong, M., Rusike, J., Mutabazi, S., & Senkondo, M. (2011). Determinants of Adopting Imazapyr-Resistant Maize Technology and Its Impact on Household Income in Western Kenya. AgBioforum, 14(3), 158–163. http://hdl.handle.net/10355/12461
- Milkias, D., & Abdulahi, A. (2018). Determinants of Agricultural Technology Adoption: The Case of Improved Highland Maize Varieties in Toke Kutaye District, Oromia Regional State, Ethiopia. Journal of Investment and Management, 7(4), 125–132. https://doi.org/10.11648/j.jim.20180704.13
- Mottaleb, K. A. (2018). Perception and Adoption of a New Agricultural Technology: Evidence from a Developing Country. Technology in Society, 55, 126–135. https://doi.org/10.1016/j.techsoc.2018.07.007
- Mwangi, M., & Kariuki, S. (2015). Factors Determining Adoption of New Agricultural Technology by Smallholder Farmers in Developing Countries. Journal of Economics and Sustainable Development, 6(5), 208–216.
- Obayelu, A., Ajayi, O., Oluwalana, E., & Ogunmola, O. (2017). What does literature say about the determinants of adoption of agricultural technologies by smallholders farmers? Agricultural Research and Technology: Open Access Journal, 6, 1.
- Ponguane, S., & Mucavele, N. (2018). Determinants of Agricultural Technology Adoption in Chokwe District, Mozambique. Munich Personal RePEc Archive, MPRA Paper No. 86284.
- Rahm, M. R., & Huffman, W. E. (1984). The adoption of reduced tilage: The role of human capital and other variables. American Journal of Agricultural Economics, 66(4), 405–413. https://doi.org/10.2307/1240918
- Ramaano, A. I. (2021). Potential of ecotourism as a mechanism to buoy community livelihoods: The case of musina municipality, limpopo, south africa. Journal of Business and Socio-economic Development, 1(1), 47–70. https://doi.org/10.1108/JBSED-02-2021-0020
- Rosenbaum, P. R., & Rubin, D. B. (1983). The central role of the propensity score in observational studies for causal effect. Biometrika, 70(1), 41–55. https://doi.org/10.1093/biomet/70.1.41
- Simtowe, F. (2011). Determinants of agricultural technology adoption: The case of improved pigeonpea in tanzania. Munich Personal RePEc Archive(MPRA Paper No. 41329).
- Simtowe, F., Asfaw, S., & Abate, T. (2016). Determinants of Agricultural Technology Adoption under Partial Population Awareness: The Case of Pigeonpea in Malawi. Agricultural and Food Economics, 4(1), 7. https://doi.org/10.1186/s40100-016-0051-z
- Simtowe, F., Kassie, M., Diagne, A., Asfaw, S., Shiferaw, B., Silim, S., & Muange, E. (2011). Determinants of agricultural technology adoption: The case of improved pigeonpea varieties in tanzania. Quarterly Journal of International Agriculture, 50(4), 325–345.
- Solomon, T., Tessema, A., & Bekele, A. (2014). Adoption of Improved wheat varieties in robe and digelu tijo districts of arsi zone in oromia region, ethiopia: Adouble hurdle approach. African Journal of Agricultural Research, 9(51), 3692–3703.
- Todaro, M. P., & Smith, S. C. (2012). Economic Development (11 ed., pp. Addison–Wesley).
- Udima, T. B., Jincai, Z., Mensah, O. S., & Caesar, A. E. (2017). Factors Influencing the Agricultural Technology Adoption: The Case of Improved Rice Varieties (Nerica) in the Northern Region, Ghana. Journal of Economics and Sustainable Development, 8(8), 137–148.
- Wooldridge, J. M. (2001). Introductory econometrics: A modern approach (2 ed.).
- Yigezu, Y. A., Mugera, A., El-Shater, T., Aw-Hassan, A., Piggin, C., Haddad, A., & Loss, S. (2018). Enhancing adoption of agricultural technologies requiring high initial investment among smallholders. Technological Forecasting and Social Change, 134, 199–206. https://doi.org/10.1016/j.techfore.2018.06.006