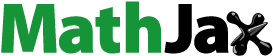
Abstract
What are the characteristics of recipient countries that attract more climate finance in mitigating and adapting to climate change? In this study, we address this question by looking at recipients in 43 Sub-Sahara African countries for the period 2006–2017, and implement several panel regression techniques, including system generalized methods of moments estimations to address potential endogeneity concerns. We also performed sensitivity analysis using panel quantile regressions. The findings show that Sub-Sahara African countries with higher population growth rate, higher poverty levels, better ease of doing business profile, weaker governance policies, weaker control of corruption, stronger rule of law enforcement, deepened social inequality, and better ICT usage, have attracted more climate finance. Policy implications of the study are discussed.
PUBLIC INTEREST STATEMENT
Global warming is one of the greatest concerns of humans. Despite African countries not contributing much to climate change, these countries suffer the most from it as they do not have the proper means to mitigate and adapt to climate change. Therefore, industrialized economies who contribute the most to climate change, have pledged climate funds to assist less developed countries circumvent the adversities of climate change even though there is much debate on whether the current levels of climate assistance is enough for mitigation and adaptation purposes. Our study examines the recipient characteristics of climate finance for a sample of 43 African countries using data collected between 2006-2017. In order of importance, we identify that SSA countries with higher ICT usage, higher social inequality, higher rule of law, higher ease of doing business and lower corruption, whilst other more traditional characteristics such as population size and poverty play less of a role. Policy implications of these findings are discussed.
1. Introduction
Sustainable development goal 13 echoes that all countries must make urgent and stringent effort to mitigate against and adapt to climate change and its associated impact. To deal with the climate canker, the United Nations Framework Convention on Climate Change (UNFCCC) divided countries into Annex I and Non-Annex I countries, where industrialized economies are categorized under Annex I, whilst developing economies fall under Non-Annex I. Subsequent to the Conference of party 15 (COP15) held in Copenhagen in 2009, a sub-group of Annex I countries (called Annex II countries) have dedicated themselves to providing the climate finance needed to aid Non-Annex I countries to mitigate and adapt to climate change (Pauw, Citation2017). Initially, US$ 30 billion was pledged between 2010 and 2012 to “fast-start” finance (Zhang & Pan, Citation2016) and the accumulative climate finance grew from US$ 584 to US$ 680 between 2013 and 2014 (Kawabata, Citation2019) and the Annex II countries have devoted themselves to mobilizing $100 billion per annum by 2020 (Nakhooda et al., Citation2014).
Amongst the non-Annex 1 countries, Sub-Sahara Africa (SSA) region hosts the most vulnerable economies to climate change even though African nations contribute the least to global greenhouse gas emissions. Considering that SSA countries are highly dependent on rain-fed agriculture with low ability to adapt to climate change; the continent is particularly vulnerable to the adverse impacts of climate change by way of flooding, drought and/or disease (Cooper et al., Citation2008; Asante & Amuakwa-Mensah, Citation2015). The “GDP worth” of exposure of African countries vulnerability to extreme climate patterns is expected to increase from $895 billion in 2018 to $1.4 trillion in 2023—which is approximately half of the continents total gross domestic product (GDP) (Dahir, Citation2018). Moreover, the continent could still face climate change adaptation costs of US$ 50 billion per year by 2050 to keep temperatures at relevant levels (Hedger & Nakhooda, Citation2015). Multilateral and bilateral climate finance directed to the region estimated at USD 4.5 billion for 665 projects has been approved for usage in SSA since 2003 and a majority of the approved funding is for adaptation purposes and less so for mitigation and forest conservation (Climate finance Regional Briefing, Citation2018).
Approximately 43 countries in SSA have received some climate finance, yet about half (49%) of the region’s approved funding is in the hands of the top ten recipient countries, and more fragile and war-torn countries in the region like Liberia, Chad, Burundi and Somalia, which are more vulnerable to climate change, receive less climate finance (Watson & Schalatek, Citation2019). Moreover, at regional level, Africa has the lowest number of approved requests in comparison to other regions such as Latin American and Asia-Pacific regions, and in general, the amount of climate funding allocated to African countries has been criticized as being insufficient to meet the mitigation and adaptation goals (Afful-Koomson, Citation2015; Fonta et al., Citation2018; Ngwenya & Simatele, Citation2020).
Gilder and Rumble (Citation2020) recently argue that the availability of climate finance tends to be more donor-centric than recipient focused and suggest that the scope of climate funding to African countries can be improved if both donor and recipient countries can have a common understanding of what is expected from each party. An important policy question in this regard is, “What do donor countries look for when seeking to fund climate financing to African countries?” “Are there certain recipient characteristics donors focus on when deciding to allocate climate finance to African countries?”
The objective of our study is to analyze the recipient characteristics of climate finance for 43 Sub-Saharan (SSA) countries by contextualizing the 4P’s (poverty, population, policy and proximity) aid donor allocation framework of Clist (Citation2011) in perspective of climate finance allocation to SSA countries. As far as we are concerned, only the previous studies of Dolsak and Crandall (Citation2013), Nakhooda et al. (Citation2013), Barrett (Citation2014), Halimanjaya (Citation2015), Robertsen et al. (Citation2015), and Samuwai and Hills (Citation2018) have studied the characteristics of climate finance recipients in developing countries and the consensus drawn from these previous studies is that aid donors do not base their financing on recipient needs but tend to focus on the country hosts “merits”. Notably, much of this previous literature focuses on developing countries at large or Asian-Pacific countries (Dolsak & Crandall, Citation2013; Halimanjaya, Citation2015; Nakhooda et al., Citation2013; Samuwai & Hills, Citation2018) whilst little evidence exists exclusively for SSA countries (Barrett, Citation2014; Robertson et al., Citation2015).
Our study extends on this previous literature for SSA countries and contributes to the existing literature in three ways. Firstly, we focus on a sample of 43 African countries and our study covers a longer time series span (i.e. 2006–2017) and a larger scope of climate finance (i.e. both bilateral and multilateral funding) compared to previous works. Secondly, we supplement the traditional 4P’s model framework adopted in Robertson et al (Citation2015) study with three dimensions of “readiness”, namely, political readiness, social readiness and economic readiness; which our study finds to be significant contributors to attracting climate finance in SSA countries. Lastly, as part of our sensitivity analysis we use quantile regressions which are more powerful and informative estimators compared to the traditional Ordinary Least Squares (OLS) estimators used in previous studies.
Against this background, we present the rest of the manuscript as follows. Section 2 of the paper discusses the associated literature review. Section 3 presents the empirical data and outlines the methodological framework of the paper. The empirical findings of the paper are present in section 4, whilst the study is concluded in section 5 of the paper.
2. Literature review
The UNFCCC was established in 1992 during the Rio De Janeiro Earth Summit in Brazil to address the threat of climate change. Article 4.3 of UNFCCC intimates that Annex 1 countries must raise bilateral and multilateral climate finance to help Non-Annex 1 countries mitigate and adapt to climate change. UNFCCC estimates that between USD 156 billion and USD 165 billion is needed for mitigation-related climate change financing (MCCF) yearly until 2030.
The Kyoto protocol of 1997 held under the COP3 mentioned that all Annex 1 countries should reduce global GHG by 5 percent as compared to 1990 level (Harvey et al., Citation2018). Non-Annex 1 countries that have contributed very little to total GHG were excluded from the target. For the Kyoto protocol to be implemented, 55 percent of high emission countries were required to ratify it. In that year, United States of America failed to do so and prevented the operationalization of the protocol until 2004 when Russia ratified; and finally brought the protocol into full force (Harvey et al., Citation2018).
During the 2007 COP13, the UNFCCC concluded that climate change was a certainty and the “Bali Action Plan” or “Bali Road Map” was introduced as a new international agreement to replace the Kyoto Protocol which expired in 2012 (Gross, Citation2009). The Copenhagen accord of 2009 held under the COP15 set targets of holding the increase in global warming by 2.6 degrees Celsius by 2100 and commitments were made to provide US$ 100 billion in climate finance annually by 2020 (Zhang et al., Citation2017).
Furthermore, the Cancun Agreements of 2010 (i.e. COP 16) established a green climate fund and further postulated that polluter countries should contribute to climate finance based on their current and historical GHG emission i.e. “polluter pays” principle (Schalatek et al., Citation2012). The Paris agreement of 2015 (i.e. COP 21), dubbed as the most successful climate meeting, submitted an “Intended Nationally Determined Contribution” (INDC) emphasizing on the financing obligations of industrialized countries to less-developed economies, although no consensus was reached on how the financial responsibilities will be shared amongst the developed countries (Zhang and Pan, Citation2016).
Robertsen et al. (Citation2015) noticed that a majority of climate funds were allocated to lower middle-income countries, next to high income countries, followed by upper middle income and low/poor income countries. Nakhooda et al. (Citation2013) found the flow of climate finance to be related to the strength of recipient country institutions, such that higher income countries, which have stronger institutions and higher levels of development, can better manage their climate funds.
Fuchs et al. (Citation2014) showed that climate finance increases when donor countries wealth increases, and countries which donors have independent aid agencies also attract more climate finance. Based on their study, the authors conclude that donor interest play a major role as to how climate finance is extended.
Barrett (Citation2014) found that vulnerability is not a determining factor in attracting climate finance in Malawi, rather climate finance goes to higher income areas that are better able to operationalize the funding or to areas where agriculture is important. Furthermore, Barrett (Citation2014) asserts that even if donors direct funds to more vulnerable countries, these funds may not get to their destinations in the vulnerable areas of the country.
Dolsak and Crandall (Citation2013) find that home-host familiarity relations such as colonial history, bilateral trade and bilateral aid have influenced the allocation of Clean Development Mechanism (CDM) project financing. The authors also find that host countries which have submitted national communications to the UNFCCC secretariat as well host countries which have been previously engaged in Activities Implemented Jointly (AIJ) mechanism were most likely to receive CDM funding.
Halimanjaya (Citation2015) found that developing countries with higher CO2 intensity, larger carbon sinks, lower per capita GDP and good governance tend to be selected as recipients of climate mitigation finance. In making a comparison between climate finance and official development aid (ODA), Halimanjaya (Citation2015) find that carbon emissions; infant mortality and corruption affect ODA and climate finance allocation/distribution differently.
Samuwai and Hills (Citation2018) recently examined the impact of “readiness” on climate finance recipients for Asian-Pacific countries and the authors use principal component analysis to form a “readiness index” based on (i) policies and institutions, (ii) knowledge management and learning (iii) fiscal policy environment. Overall, higher levels of readiness are a more important factor in attracting climate finance in comparison to other plausible determinants such as population, per capita GDP levels and levels of governance.
Our study builds upon the studies of Samuwai and Hills (Citation2018) and extends on the definition of “readiness” in context of “three dimensions” proposed in Sarkodie and Strezov (Citation2019), i.e. “political readiness”, “social readiness” and “economic readiness”. Moreover, our study also considers other plausible determinants such as population, per capita GDP and institutional quality which are frequently used in previous literature. In also differing from previous studies, we make use the more efficient generalized methods of moments (GMM) estimators which are time variant and panel in nature, and we further employ quantile regression estimators as part of our sensitivity analysis to explore the non-monotonic dependence of the explanatory variables on climate finance.
3. Empirical specification and data
We borrow our empirical specification by following Clist (Citation2011) “Two-Part” or “Cragg” model framework 4P’s (i.e. Poverty, population, proximity and policy). The first stage of the model determines which countries receive aid and the second part of the model is a linear regression that looks at how much aid the recipients receive. Overall, the “4P model” can be represented a cross sectional regression with a dependent variable of aid and the 4P’s being dependent variables:
Clist (Citation2011) highlighted that, due to the proximity variables such as language, distance and colony which are time invariant, it will not allow for time series analysis. In this study, we drop the proximity variables and replace it with “readiness” variables, i.e. (i) political readiness (ii) social readiness (iii) economic readiness. On this backdrop, the empirical specification employed in this study is given as follows:
The description of the variables, sources and expected values are as follows:
Climate Finance: Climate finance data used in this study is sourced from DAC donors’ finance of various development projects categorized under OECD Rio Marker Creditor Reporting System (OECD, Citation2018). Total commitment data is used in this study because it covers a longer time period and wider scope of finance compared to strictly bilateral finance commonly used in previous studies (Robertson et al. 2015; Samuwai & Hills, Citation2018). From the summary statistics reported in , an average of USD 371 million is extended to SSA yearly by way of climate finance.
Population: Clist (Citation2011) points out that population is added to the model in other to check biasedness of the model towards high population countries. This bias is expected because high population countries normally have lower levels of development and so will receive more climate finance. On that backdrop, population is expected to impact positively on climate finance received. Population growth is sourced from Notre Dame Global Adaptation index (ND-GAIN) data. On the average, population of SSA grew by 2.4 percent during the study period.
Poverty: Clist (Citation2011) and Robertsen et al. (Citation2015) used GDP per capita as a measure of poverty which is sourced from World Bank’s Development Indicators. GDP per capita serves as an indicator of adaptive capacity and a measure of a country’s ability to cope with the adverse impacts of climate change. A country’s ability to cope with climate change is related to its level of development, highly developed countries have more resources with which to adapt to climate change. Since countries with lower adaptive capacity require more climate finance, so a negative relation between GDP per capita and climate finance is expected (Robertsen et al., Citation2015; Halimanjaya, Citation2015). GDP per capita for SSA averaged 2,425, which by World Bank classifications corresponds to a lower middle-income region.
Political Readiness: Under the policy variable, the study looked at effectiveness of government policy in each country. If governments are able to effectively and efficiently roll out policies, they will be able to turn climate finance received into desirable outcome in the mind of donors (Clist, Citation2011; Robertsen et al., Citation2015). Based on that, a positive relation is expected between government policy and climate finance received. Clist (Citation2011) accessed government policy variable from Freedom House Freedom Index and Political Terror Scale. Robertsen et al. (Citation2015) parted away from Clist (Citation2011) and employed Polity IV overall Polity2 score, because policy data used by Clist is more applicable for Western Democracy than SSA. However, Halimanjaya (Citation2015) used Kaufman institutional measure which is made up of rule of law, regulatory quality, control of corruption, voice and accountability, government effectiveness and political stability. This study employed a policy variable similar to that used by Kalimanjaya (2014) from ND-GAIN which is governance readiness (GRit) index compiled using four main indicators; political stability and non-violence, control of corruption, regulatory quality and rule of law. On the average general governance readiness of SSA is very low, less than 0.5, implying that governance readiness of SSA towards climate mitigation and adaptation is very low.
Table 1. Summary statistics
Besides the governance readiness index, we also include the individual dimensions of government readiness (i.e. control of corruption, regulatory quality and rule of law). Control of corruption was couched by assessing the views of experts worldwide on how public power is exercised for private gain. Prior studies have showed that corruption has a significant negative impact on climate finance (Habib & Zurawicki, Citation2002). Regulatory quality examined the perception of people on government’s ability to formulate and implement policies to promote growth and development of the private sector. Gani (Citation2007) showed that countries with stronger institutions can easily deploy adaptation actions and adaptation-related policies, and thus can attract more climate finance. Finally, rule of law captures expert views on people’s confidence in the rules of society and their preparedness to abide by it. Some of such rules include property rights, quality of contract enforcement, efficiency and effectiveness of police service and high courts. Burnside and Dollar (Citation2004) intimated that rule of law is a significant factor in driving climate finance flows into a country. SSA countries are performing better in promoting rule of law and strengthening regulatory quality as compared to their fight against corruption (refer to summary statistics).
Social Readiness: Social readiness index compiled by ND-GAIN used four main indicators; Education, social inequality, Information Communication Technology (ICT) infrastructure and innovation. Education indicator is measured as the ratio of number of enrolled people in tertiary education to the number of people in the age group that officially corresponds to tertiary education attendees. Mercer (Citation2010) posits that a well-established educational sector helps build adaptive capacity and adaptive solution for climate change with ease and attracts more climate finance. Social inequality indicator is measured using the country’s poorest quintiles share in national income and consumption. The reason is that Tol et al. (Citation2004) iterated that poorest households are the most vulnerable to climate related problems and so needs more climate finance.
ICT infrastructure index by ND-GAIN was built using four main sub-indicators; mobile phone subscription per 100 persons, fixed broad band subscription per 100 persons, fixed phone subscription per 100 persons and the percentage of individuals using internet. Information on all four indicators were collated from the annual ICT Development Index (IDI) database. This social indicator is very important because Pant and Heeks (Citation2011) argued that ICT infrastructure enhances knowledge integration and learning which is a key driver of adaptive capacity. Furthermore, technical support is provided for Early Warning Systems (EWS) which strengthens local organizations implementing adaptation policies and hence attracts more climate finance. Due to data unavailability innovation is not added in our study, leaving three social readiness variables; education, social inequality and ICT infrastructure. Based on prior studies, a positive relation between social readiness variables and climate finance is expected, all variables were sourced from ND-GAIN. On the average 42 percent of people living in SSA are currently living in extreme poverty based on the social inequality statistics. On the average, only 6 percent of the people in the tertiary age bracket are undergoing tertiary education on the continent with access to internet averaging 26 percent from the summary statistics table.
Economic Readiness: Economic readiness (ERit) index of a country by ND-GAIN is measured using “Ease of Doing Business (DB)” indicator by World Bank to show how various countries are able to attract adaptation investment (Chen et al., Citation2015). The index on doing business is generated from 10 topics using 40 indicators. The topics constitute of how construction permits are dealt with, ease of starting business, how to register property, accessibility of electricity, investor protection, getting credit, tax payment, contract enforcement, trading across borders, insolvency resolution, and enforcing contracts. ND-GAIN computed economic readiness by ranking countries according to percentile on each topic, and then DB scores generated by averaging the percentile rankings of all 10 topics. This data is sourced from ND-GAIN and expected to show a positive impact, since countries with very high ability to attract adaptation investment will be able to attract more climate finance. Readiness index ranges from 0 to 1, where close to 1 represented higher readiness level and close to 0 represented poor readiness level of a country. SSA is showing on average an index of 0.253 for economic readiness, which shows that most countries in the region are displaying less readiness to adapt to climate change and so needs more finance to do that. Only a few countries like Mauritius and South Africa showed economic readiness above 0.5, indicating that most countries in SSA needs to be educated more to be ready.
Data collected was for 43 SSA countries for the period 2006 to 2017. Countries that have several missing data points were not included in the study. However, countries with one or two missing data points were included to yield an unbalanced panel. summarizes the descriptive statistics of the time series whilst the correlation matrix is presented in . It is important to note that none of the correlation coefficients are of values high enough to suspect multicollinearity amongst the variables.
Table 2. Pairwise correlations
3.1. GMM estimation technique
This study employed a dynamic panel model propounded by Arellano and Bover (Citation1995) and Blundell and Bond (Citation1998) known as System Generalised Method of Moment (GMM-SYS) estimator. This estimator is employed because it is more efficient and lagged levels of the explanatory variables have also been found to be poor instruments for the first-differenced regressors. System GMM estimator obtains two equations from the level equation (model 3), one differenced equation and the other level equation to generate additional instruments. The level variables in the second equation use their own first differences as instruments for the model. The System GMM technique employed in this study is represented in model (3).
Where
represent the coefficients of the predictor variables under study.
,
represent the error term,
represents individual country effect,
represents time specific effect and
represents random disturbance term. Lagged dependent variable is included as a regressor to capture the persistence of the dependent variable.
System GMM outperforms most panel models in a number of ways in estimating growth models and other models like model (3). Firstly, reverse causality relationship is expected between some of the independent variables and the dependent variable. For example, poverty can help attract more climate finance, whereas climate finance can also help reduce poverty which is an endogeneity problem. System GMM allows for dealing with endogeneity or simultaneity problem in the model (Alguacil et al., Citation2011). Secondly, system GMM has been found to have higher efficiency and lower bias as compared to other estimators like differenced GMM estimator when certain persistence exists in a series (Alguacil et al., Citation2011). This is because Blundell and Bond (Citation1998) mentioned that differenced GMM estimates behave poorly when the explanatory variables show a strong autoregressive relation between some of the variables.
Notwithstanding, system GMM also exhibits some weaknesses which has to do with the efficacy of their instruments and the accuracy of their assumption that the errors do not contain serial correlation. Most studies tests the validity of the instruments using Arellano-Bond test and Sargan test of overidentifying restrictions. To circumvent the problem of overidentifying restrictions, the study employed robust standard errors and so only reported results for Arellano and Bond test. Robustness and sensitivity of our result is tested using panel quantile regression analysis.
In this study, some variables have more data points than others making our panel an unbalanced panel. The Breitung, Im-Pesaran-Shin (IPS) and Fisher test of unit root are the main tests for unbalanced data set. IPS test statistic uses averages of bias adjusted t-statistics for each panel (stata.com). Fisher-type panel unit root is more explicit than IPS and Breitung. Choi (Citation2001) intimated that for finite and infinite samples, the inverse-logit and inverse-normal transformations can be used. From the panel unit root test results reported in Appendix 1, it is clear that all the variables are stationary at levels using all the Fischer type tests including the inverse-logit and inverse-normal transformations.
4. Empirical findings
In this section of the paper we present the empirical findings from our analysis. presents the GMM regression estimates and we present four different estimated models to ensure robustness of our estimates. Model 1 is the regression inclusive of population, per capita GDP and the individual measures of government readiness; Model 2 is the regression inclusive of population, per capita GDP, government readiness index and the individual measures of social readiness; Model 3 is the regression inclusive of population, per capita GDP, government readiness index, social readiness index and economic readiness index; Model 4 is the regression inclusive of all dependent variables. presents the pooled OLS and fixed effects regression estimates as additional robustness checks.
Table 3. System GMM results for Sub-Sahara Africa
Table 4. Pooled OLS and fixed effect models
Judging from the GMM estimation results reported in , there is consistency in estimated coefficient estimates across all four estimated regression models. For instance, in models 1–4, the coefficient estimate on the population variable is positive and statistically significant at all critical levels whist the coefficients on the poverty variable are negative and statistically significant at all critical levels. Similarly, in models 2, 3 and 4, the government readiness index produces negative coefficient estimates which are statistically significant at all levels of significance whilst all individual measures of economic readiness (i.e. social inequality, ICT use, education), in models 2 and 4 produce positive and statistically significant estimates. Moreover, the coefficient estimates of the individual measures of government readiness reported in models 1 and 4, produces negative and significant statistics for the control of corruption and regulatory quality variables whilst the coefficient estimates on rule of law are positive and statically significant. Also note that the coefficient estimates on the social readiness index and the economic index readiness variables reported in models 3 and 4, both produce positive estimates, although the results from model 3 produces statically significant estimates. Finally, judging by the magnitude of the coefficient estimates in models (1)—(4), the strongest determinant of climate finance is government readiness, which is followed by education, social readiness, rule of law, control of corruption, economic readiness, ICT usage, social inequality, regulatory power, population and lastly per capita GDP.
When making a comparison of the GMM estimates to OLS and fixed effects model estimates presented in , we note very few discrepancies in the signs, significance and magnitudes of the coefficient estimates. Common to all three estimators are positive coefficients on population, rule of law, social inequality, ICT use and economic readiness and negative coefficients on poverty, government readiness and control of corruption. Also judging by the coefficient estimates, the measures of “political and economic readiness” have higher elasticities when compared to other determinants of climate finance. For the remaining variables (i.e. education, social readiness and regulatory quality) there are discrepancies in either the significance of the variable (education) or the coefficient sign on the variable (i.e. social readiness and regulatory quality).
Altogether our findings can be summarized as follows. SSA countries characterized with (i) less government readiness (ii) higher education (iii) higher social readiness (iv) stronger rule of law (v) lower control of corruption (vi) economic readiness (vii) higher ICT usage (viii) higher social inequality (ix) lower regulatory power (x) higher populations and (xi) lower per capita GDP levels. These findings are comparable to those obtained in previous studies. For instance, Robertsen et al. (Citation2015) find higher populated countries to receive more aid and climate finance, Samuwai and Hills (Citation2018) found a significant and negative coefficient on the per capita variable implying poor countries receive more climate finance, whereas Halimanjaya (Citation2015) and Nakhooda et al. (Citation2013) found that countries with higher corruption and higher quality of institutions attract more climate finance. Pant and Hicks (Citation2011) find that ICT infrastructure enhances knowledge integration and learning which is a key driver of adaptive capacity and a motivator of increase in climate fund. Chen et al. (Citation2015) also finds that better ICT infrastructural facilities provide good technical support in terms of EWS development which strengthens local organizations implementing adaptation policies and hence attracting more climate finance. Moreover, Masse et al. (Citation2020) find that “climate finance readiness” a significant factor in increasing efficiency of climate finance received by developing countries but not as important as population or poverty.
4.1. Sensitivity analysis: Quantile regression estimates
In this section of the study, a sensitivity analysis using a simultaneous quantile regression is employed to control for distributional heterogeneity. The panel quantile regressions allow for the estimation of the conditional mean function on a full range of conditional quantile “points” hence providing a more complete picture relationship between the dependent and independent variables. Consider the following conditional quantile function:
Where Y is the dependent variable with dimension matrix N × 1, X is a set of k independent variables with dimension matrix N × k and βτ is the distribution function of Y with a dimensional matrix k × 1 vector. Note that since conditional distribution function βτ can be obtained as:
And therefore, solving the sample analogue defines the estimator of β as:
Where yi and xi are ith element of Y and X, respectively, θτ(u) = u(τ—I(u < 0)), I is an indicator function, and 0 < τ < 1. Our study focuses on four values of τ, i.e. τ = 0.25, 0.50, 0.75 and 0.90 which represent the 25th, 50th, 75th and 90th distributional quantiles, respectively.
From the regression results reported in , the coefficient signs on the variables remaining more-or-less consistent with those found for the GMM, OLS and Fixed effects estimators, although the magnitude and levels of significance differ across different quantile distributions. Altogether we find negative coefficients across all quantiles for five variables, namely, (i) poverty, (ii) control of corruption, (iii) education, (iv) governance readiness index, and (v) social readiness index whilst finding positive coefficients across all quantiles for six variables, namely, (i) pollution, (ii) regulatory quality, (iii) rule of law, (iv) social inequality, (v) ICT use, (vi) economic readiness.
Table 5. Panel quantile regression for Sub-Sahara Africa
Out of the five variables with negative coefficient estimates, only poverty, governance readiness index, social readiness index produces negative and statistically significant estimates across all quantiles with the strength of the estimates increasing as one moves up the quantiles. For the remaining variables with negative coefficients, control of corruption produces significant estimates in the 50th, 75th and 90th quantiles whilst education produces a significant samite in the 50th quantile. These later findings imply that only countries with moderate to high levels of corruption moderate levels of education attract climate finance.
Out of the 6 variables with positive coefficient estimates, rule of law and ICT use; produces statistically significant estimates across all quantiles with the strength of the relationship increasing as one moves up the quantiles. For the remaining coefficients with positive estimates, population growth produces significant estimates at the 25th, 50th and 75th quantiles; regulatory quality and economic readiness at 50th and 75th and 90th quantiles; social inequality at 75th and 90th quantiles. Moreover, we find it important to note that for the population variable, the magnitude of coefficient estimates is the largest at the 50th quantile, implying that economies with moderate population growth receive the most climate financing amongst the SSA countries. Similarly, for the economic readiness variable, the largest coefficient estimate is found at the 75th quantile, implying that economies with moderately-high “ease of doing business” levels attract the most climate finance.
In collectively summarizing the findings from our analysis, we concluded that countries in the SSA region who attract the most climate funding are those characterized by (i) less government readiness (ii) higher education (iii) higher social readiness (iv) stronger rule of law (v) lower control of corruption (vi) economic readiness (vii) higher ICT usage (viii) higher social inequality (ix) lower regulatory power (x) higher populations and (xi) lower per capita GDP levels. Note that these determinants have been placed in order of strength of regression coefficients.
5. Conclusion
Since 2005, the UNFCCC has shown commitment to providing funding for developing countries to mitigate and adapt to climate resilient economies. Whilst funding commitments have significantly grown over the last two decades, African countries have received the least climate funding assistance for adaptation and mitigation purposes, despite contributing the least to global greenhouse gas emissions and being the most vulnerable to climate change effects. Using 43 SSA countries as a case study, we sought to shed light on the recipient characteristics which climate finance donors consider in extending finance to African host countries. To this end, we augment the 4P framework for donor aid allocation with three dimensions of “readiness” (i.e. political readiness, social readiness and economic readiness) and estimate the panel models using GMM and quantile regression estimators applied to annual time series covering the period 2006–2017.
Common to most previous literature, our results find that population is a significant determinant of climate finance received in SSA economies. Our quantile regression estimates particularly depict an inverted U-shaped relationship between climate finance and population such that African countries with moderate populations receive the most climate assistance. Another common finding in our study is that African countries with lower per capita GDP (poorer countries), lower control of corruption (moderate to high levels of corruption) as higher regulatory quality and rule of law (moderate to high institutional quality) attract the most climate.
Our study also presents new empirical evidence on government readiness, social readiness and economic readiness (ease of doing business) as significant determinants of climate finance received. Judging from the magnitude of the coefficient estimates, our study finds that a country’s ICT usage, social inequality (part of social readiness), rule of law (part of government readiness) and ease of doing business (measure of economic readiness) are the most responsive determinants of climate finance received in the SSA region even when compared to “traditional” determinants such as population, poverty and institutional quality.
The United Nations Development Programme (UNDP) has developed an institutional and policy framework for climate finance readiness which they define as “the capacity of countries to plan for, access, deliver, and monitor and report on climate finance”. A number of “climate finance readiness” programmes have been designed to support African countries in planning for and accessing climate funding from potential donors. From our study it is evident that policymakers should not only focus on the “institutional quality readiness” of climate finance but must also focus on other dimensions of climate finance readiness, in particular, political readiness in the form of institutional quality and levels of corruption, as well as social and economic readiness, in the form of social inequality, technological usage and ease of doing business.
Additional information
Funding
Notes on contributors
Andrew Phiri
Dr. Isaac Doku is currently a post-doctorate student with the department of economics at Nelson Mandela University who has research interests are in environmental economics, applied econometrics and macroeconomics.
Professor Ronney Ncwadi is a full professor and director of the school of Economics, Development and Tourism at Nelson Mandela University. He is a member of several international organisations, including the Pan African Entrepreneurship Research Council, United States, and the Athens Institute for Education and Research, Greece. He is also a member of the BRICS Academic Forum. He has had a number of articles published both in national and international journals, and has presented papers at academic conferences.
Professor Andrew Phiri is an associate professor in Economics at the Nelson Mandela University and has research interests in macroeconomics, applied econometrics, financial economics and international economics.
References
- Afful-Koomson, T. (2015). The green climate fund in Africa: What should be different? Climate and Development, 7(4), 367–17. https://doi.org/10.1080/17565529.2014.951015
- Alguacil, M., Cuadros, A., & Orts, V. (2011). Inward FDI and growth: The role of macroeconomic and institutional environment. Journal of Policy Modeling, 33(3), 481–496. https://doi.org/10.1016/j.jpolmod.2010.12.004
- Arellano, M., & Bover, O. (1995). Another look at the instrumental variable estimation of error-components models. Journal of Econometrics, 68(1), 29–51. https://doi.org/10.1016/0304-4076(94)01642-D
- Asante, F. A., & Amuakwa-Mensah, F. (2015). Climate change and variability in Ghana: Stocktaking. Climate, 3(1), 78–99. https://doi.org/10.3390/cli3010078
- Barrett, S. (2014). Subnational climate justice? Adaptation finance distribution and climate vulnerability. World Development, 58, 130–142. https://doi.org/10.1016/j.worlddev.2014.01.014
- Blundell, R., & Bond, S. (1998). Initial conditions and moment restrictions in dynamic panel data models. Journal of Econometrics, 87(1), 115–143. https://doi.org/10.1016/S0304-4076(98)00009-8
- Burnside, C., & Dollar, D. (2004). Aid, policies, and growth: Revisiting the evidence. The World Bank.
- Chen, C., Noble, I., Hellmann, J., Coffee, J., Murillo, M., & Chawla, N. (2015). University of Notre Dame global adaptation index country index technical report. ND-GAIN: South Bend, IN, USA.
- Choi, I. (2001). Unit root tests for panel data. Journal of International Money and Finance, 20(2), 249–272. https://doi.org/10.1016/S0261-5606(00)00048-6
- Climate finance Regional Briefing. (2018). Climate finance regional briefing: Middle East and North Africa (2018). Retrieved November 9, 2019, from https://climatefundsupdate.org/publications/climate-finance-regional-briefing-middle-east-and-north-africa-2018
- Clist, P. (2011). 25 years of aid allocation practice: Whither selectivity? World Development, 39(10), 1724–1734. https://doi.org/10.1016/j.worlddev.2011.04.031
- Cooper, P., Dimes, J., Rao, K., Shapiro, B., Shiferaw, B., & Twomlow, S. (2008). Coping better with current climatic variability in the rain-fed farming systems of sub-Saharan Africa: An essential first step in adapting to future climate change? Agriculture, Ecosystems & Environment, 126(1–2), 24–35. https://doi.org/10.1016/j.agee.2008.01.007
- Dahir, A. (2018). Africa’s fastest growing cities are the most vulnerable to climate change globally. World Economic Forum, Quartz publishers. December 21.
- Dolsak, N., & Crandall, E. (2013). Do we know each other? Bilateral ties and the location of clean development mechanism projects. Climate Change, 118(3–4), 521–536. https://doi.org/10.1007/s10584-013-0694-7
- Fonta, W., Ayuk, E., & Van Huysen, T. (2018). Africa and the green climate fund: Current challenges and future opportunities. Climate Policy, 18(9), 1210–1225. https://doi.org/10.1080/14693062.2018.1459447
- Fuchs, A., Dreher, A. & Nunnenkamp, P. (2014). ‘Determinants of donor generosity: a survey of the aid budget literature’ World Development 56, 172–199. https://doi.org/10.1016/j.worlddev.2013.09.004
- Gani, A. (2007). Governance and foreign direct investment links: Evidence from panel data estimations. Applied Economics Letters, 14(10), 753–756. https://doi.org/10.1080/13504850600592598
- Gilder, A., & Rumble, O. (2020). Improving sub-Saharan African access to climate change finance: An alternative view. SAIIA Policy Briefing No. 194, April.
- Gross, M. (2009). Climate crunch year. Current Biology, 19(14), 537–539. https://doi.org/10.1016/j.cub.2009.07.011
- Habib, M., & Zurawicki, L. (2002). Corruption and foreign direct investment. Journal of International Business Studies, 33(2), 291–307. https://doi.org/10.1057/palgrave.jibs.8491017
- Halimanjaya, A. (2015). Climate mitigation finance across developing countries: What are the major determinants? Climate Policy, 15(2), 223–252. https://doi.org/10.1080/14693062.2014.912978
- Harvey, C. A., Saborio-Rodríguez, M., Martinez-Rodríguez, M. R., Viguera, B., Chain-Guadarrama, A., Vignola, R., & Alpizar, F. (2018). Climate change impacts and adaptation among smallholder farmers in Central America. Agriculture & Food Security, 7(1), 57. https://doi.org/10.1186/s40066-018-0209-x
- Hedger, M., & Nakhooda, S. (2015). Finance and intended nationally determined contributions (INDCs): Enabling implementation. Overseas Development Institute.
- Kawabata, T. (2019). What are the determinants for financial institutions to mobilize climate finance? Journal of Sustainable Finance and Investment, 9(4), 263–281. https://doi.org/10.1080/20430795.2019.1611148
- Masse, J., Gerber, P., Halpern, C., & Baedeker, T. (2020). Review: Climate finance readiness of the animal protein sector: Overview of experience in linking the sector to climate finance, and options to address bottlenecks. Animal, 14(3), 491–499. https://doi.org/10.1017/S1751731120001755
- Mercer, J. (2010). Disaster risk reduction or climate change adaptation: Are we reinventing the wheel? Journal of International Development: The Journal of the Development Studies Association, 22(2), 247–264. https://doi.org/10.1002/jid.1677
- Nakhooda, S., Norman, M., Barnard, S., Watson, C., Greenhill, R., Caravani, A., & Banton, G. (2014). Climate finance: Is it making a difference. A review of the effectiveness of multilateral climate funds. Overseas Development Institute.
- Nakhooda, S., Watson, C., & Schalatek, L. (2013). The global climate finance architecture. Overseas Development Institute.
- Ngwenya, N., & Simatele, M. (2020). The emergence of green bonds as an integral omponent of climate finance in South Africa. South African Journal of Science, 116(1–2), #6522. https://doi.org/10.17159/sajs.2020/6522
- OECD. (2018). Climate finance from developed to developing countries. Retrieved November 9, 2019, from http://www.oecd.org/environment/cc/Climate-finance-from-developed-to-developing-countries-Public-flows-in-2013-17.pdf
- Pant, L., & Heeks, R. (2011). ICT-enabled development of capacity for climate change adaptation. Centre for Development Informatics. Institute for Development Policy and Management, University of Manchester.
- Pauw, P. (2017). Mobilising private adaptation finance: Developed country perspectives. International Environmental Agreements: Politics, Law and Economics, 17(1), 55–71. https://doi.org/10.1007/s10784-016-9342-9
- Robertsen, J., Francken, N., & Molenaers, N. (2015). Determinants of the flow of bilateral adaptation-related climate change financing to Sub-Saharan African countries. LICOS Discussion Paper Series No. 373-2015, November.
- Samuwai, J., & Hills, J. (2018). Assessing climate finance readiness in the Asia-PAcific region. Sustainability, 10(4), 1–18. https://doi.org/10.3390/su10041192
- Sarkodie, S., & Strezov, V. (2019). Economic, social and governance adaptation readiness for mitigation of climate change vulnerability: Evidence from 192 countries. Science of the Total Environment, 656, 150–164. https://doi.org/10.1016/j.scitotenv.2018.11.349
- Schalatek, L., Nakhooda, S., & Bird, N. (2012). The Green Climate Fund. In Overseas development Institute and Heinrich Böll Stiftung North America.
- Tol, R. S., Downing, T. E., Kuik, O. J., & Smith, J. B. (2004). Distributional aspects of climate change impacts. Global Environmental Change, 14(3), 259-272. https://doi.org/10.1016/j.gloenvcha.2004.04.007
- Watson, C., & Schalatek, L. (2019). Climate finance fundamentals 3: Thematic briefing - adaptation finance. Overseas Development Institute and Heinrich Böll Stiftung North America.
- Zhang, W., & Pan, X. (2016). Study on the demand of climate finance for developing countries based on submitted INDC. Advance in Climate Change Research, 7(1–2), 99–104. https://doi.org/10.1016/j.accre.2016.05.002
- Zhang, Y., Chao, Q., Zheng, Q., & Huang, L. (2017). The withdrawal of the U.S. from the Paris Agreement and its impact on global climate change governance. Advances in Climate Change Research, 8(4), 213–219. https://doi.org/10.1016/j.accre.2017.08.005