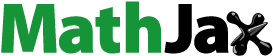
Abstract
This study examines the impacts of multiple agricultural technology adoption on poverty in rural Amhara region, Ethiopia. The study is based on Ethiopian socio economic survey of 2015/16. A total of 656 farm households were included. The study employed Multinomial Logit model to identify the determinants of adoption and Multinomial Endogenous Switching Models to measure the effect of adoption on poverty. The results revealed that educational level of the household head, family size, off-farm participation, livestock, extension visit, credit access, advisory service, plot distance, distance from market, distance from zonal town, and remittances are the major determinants of agricultural technology adoption decisions. The impact results revealed that adoption of multiple technologies increases consumption expenditure significantly, thereby reduces poverty. Moreover, adoption in package provides higher consumption than in isolation. Therefore, the study suggests that policies that promote wider adoption of alternative agricultural technologies at the regional and country level help to reduce poverty.
JEL Classifications:
PUBLIC INTEREST STATEMENT
In our country Ethiopia, agriculture is at the heart of the economy, but the sector’s productivity is very low due to lower rate of technology adoption. To this end, poverty is a critical problem in the country. An adoption of agricultural technology plays a significant role to improve productivity and thereby reduces poverty. Thus, this study tried to examine the effects of agricultural technology adoption in rural Amhara region. The study found that adoptions of alternative technologies significantly increases consumption and thereby reduces poverty. Therefore, a wider promotion of agricultural technology adoption helps to reduce poverty.
1. Introduction
In the 21st century, the agricultural sector remains fundamental for poverty reduction, economic growth, and environmental sustainability in many developing countries (McCalla, Citation2001). In Ethiopia, where agriculture is the base of the economy - it accounts for over 34.1% of Gross domestic product, employs over 79% of the population, accounts for 79% of foreign earnings, provides raw material and capital for investment and to market and covers over 90 percent of smallholder agricultural production (Diriba, Citation2020). The sector is a key for stimulating growth and overcoming poverty. However, the contribution of agriculture is declining overtime because the sector is still rain-fed subsistence production, in many areas traditional farming practices still dominate, highly affected by climate variability, droughts and often characterized by low use of modern technology. These constraints have kept the sector’s potential crop production and productivity very low (Abewa et al., Citation2020). Therefore, a majority of the country’s population is highly challenged by development problems of poverty, food insecurity and deprivation for a long time. Therefore, to overcome these developmental challenges, increasing agricultural production and productivity has become the priority. Because increasing agricultural productivity has the potential to significantly impact the welfare of millions of smallholder holder farmers by improving productivity, increasing income and consumption in the country (Belay & Mengiste, Citation2021).
In Ethiopia where land is scarce, the feasible way to aagricultural production and productivity is through increasing productivity by investing in agricultural technologies or improving the level of technical efficiency (Mohammed, Citation2014). Thus, in the country, agricultural production and productivity is highly dependent on the generation and delivery of modern agricultural technologies. The technologies incorporated new varieties, farm management practices, fertilizer, chemicals, and often irrigation led to improvement in agricultural production (Tefera et al., Citation2016).
In recognizing such roles of agricultural technology, the government of ethiopia has been underway in designing and implementing different policies to increase agricultural production and productivity through promoting agricultural technologies starting from the imperial regime. Following this, there are several studies in the country that have been evaluated the impacts of agricultural technology adoption on poverty. For example, (Sebsibie et al., Citation2015; Abewa et al., Citation2020; Belay & Mengiste, Citation2021; Natnael, Citation2019; Belete & Melak, Citation2018; Ayenew et al., Citation2020; Shita et al., Citation2020) found that adoptions of agricultural technology significantly reduces household poverty through raising productivity, income and consumption of the poor. Despite, the government contributed directly to poverty reduction strategies by introducing new improved farm technologies, still the uptake of the technology is very low and in result, production and productivity remains to be very low (Keba, Citation2019; Natnael, Citation2019; Tefera et al., Citation2016). Therefore, the main objective of this study is to examine the determinants of agricultural technology adoption and its impact of single and combined technology on poverty reduction in rural Amhara national region of Ethiopia.
The rest of the paper is organized as follows; Part 2 provides relevant literatures, Part 3 describes the methodology of the study, Part 4 presents the results of the study and Part 5 provides conclusion and recommendation of the study.
2. Literature
Agricultural technology improves agricultural productivity, raises farmers’ income and consumption; it should be the major factor in reducing poverty and food insecurity. There are different studies in different parts of the country that explored the negative relationship between adoption directly increases productivity and poverty. For example:
Habtewold (Citation2021) examined the the impact of adopting climate-smart agricultural technology on the multidimensional poverty status of rural households in Ethiopia. The study found that the impact is significantly higher in severely deprived households. It is also observed that the reduction in multidimensional poverty due to the technology is through an increase in income/consumption via improvement in production gain. The impact channels more through the non-food expenditure pathway.
Belete and Melak (Citation2018) investigated the impact of adoption of small-scale irrigation technologies on child nutritional wellbeing of farm households in the Amhara national region of Ethiopia. The results of the average treatment effect on treated participants suggest that adoption of small-scale irrigation technologies has a positive impact on improving the adopters’ nutritional status. Agricultural technology adopting households have significantly lower acute malnutrition status than those of non-adopting households even after controlling for the potential heterogeneity.
Ayenew et al. (Citation2020) conducted a study on the effect of improved wheat variety adoption on household’s welfare in Ethiopia. The study results revealed that adoption decision and intensity of adoption of farm households have determined by credit access, extension visits, soil fertility, plot size, off-farm employment, age of household head, distance from input market, and farm experience. And adoption of improved wheat varieties has a positive and significant effect in enhancing farm household’s welfare.
Shita et al. (Citation2020) analyses the impact of agricultural technology adoption on income inequality. The estimated results revealed that adoption of agricultural technologies such as chemical fertiliser and improved seeds significantly increase total household income but worsen income distribution. Wordofa et al. (Citation2021) investigates the impact of improved agricultural technology use on farm household income in eastern Ethiopia. The results of the study show that households using improved agricultural technologies had, higher annual farm income compared to those households not using such technologies. Overall, the empirical works that have been conducted shows that agricultural technology adoption is an essential strategy for increasing agricultural productivity, achieving food self-sufficiency and poverty reduction among smallholder farmers.
However, many of the aforementioned studies, shows the impact of single agricultural technology on poverty (for example, Sebsibie et al., Citation2015; Natnael, Citation2019; Ayenew et al., Citation2020; Wordofa et al., Citation2021), many studies have been employed particularly PSM and OLS model for impact measurement (for example, Habtewold, Citation2021; Natnael, Citation2019; Sahu & Das, Citation2015; Sebsibie et al., Citation2015; Shita et al., Citation2020; Wordofa et al., Citation2021). But these models are subject to selection bias, endogeneity problem and heterogeneity problem, which may not show the real impact on the outcome variable. Therefore, this study contributes to the existing literature by examining the impact of multiple technology adoption on poverty. In response to the impact evaluation pitfalls of selection bias, endogeneity and unobserved heterogeneity problem, this study employed an endogenous switching regression model. Thus, this study tests whether adoption of alternative agricultural technologies has an impact on poverty reduction proxied by household consumption expenditure per adult equivalent in the study area.
2.1. Working hypothesis
Many impact evaluation studies showed that a direct and indirect positive welfare effects of technology adoption on the farm households. On the other hand, impacts of adoption depends on the poverty status of households that the impact may not have an identical effect on poor and non-poor farmers (Dercon et al., Citation2007). Based on the various literatures on the subject and theoretical and analytical framework defined in this study, the following working hypothesis was tested:
The adoption of alternative agricultural technologies increases the consumption expenditure of farm households in the study area. Hence, there is a strong case that farmers could have more consumption due to adoption of different agricultural technologies.
3. Methodology
3.1. Description of study area
The State of Amhara consists of 10 administrative zones, one special zone, 105 Woredas, and 78 urban centers. Amharic is the working language of the state. The capital city of the State of Amhara is Bahir-Dar. The Sate of Amhara is located in the north western and north central part of Ethiopia. The State shares common borders with the state of Tigray in the north, Afar in the east, Oromiya in the south, Benishangul/Gumuz in the south west, and the Republic of Sudan in the west. The State of Amhara covers an estimated area of 170,752 square kilometers. 90% of the total populations are rural residents which heavily depend on agriculture. The Amhara Highlands receive 80% of the total rainfall of Ethiopia and is the most fertile and hospitable region of Ethiopia. Most of the Amhara Region is mountainous and is covered with large trees and forest (Ethiopian Government Portal, Citation2019).
3.2. Description of data
The study employed secondary data sources. The study used survey data collected from 656 farm households drawn from rural Amhara regional state collected by Ethiopian Socioeconomic Survey (ESS, Citation2015/16). The data is regionally adequate and representative, and covered a wide range of topics.
3.3. Methods of data analysis and model specification
In order to evaluate the impact of adoption of agricultural technology on poverty can be modeled in the setting of a two stage framework. In the first stage, the study models the farmers’ choices of agricultural technologies using a MNLFootnote1, and the second stage regression estimates the determinants of total consumption (proxy for poverty) conditional on specific criterion function using MESMFootnote2 by adding inverse mills ratio as a correction term computed from the MNL model in order to reduce the bias from not accounting selection in to the adoption decisions procedure is estimated simultaneously. Based on (Kassie et al., Citation2015), this way helps to get consistent and efficient estimates of the selection process and correction for the outcome equation for evaluating single and combined choices, even IIA is severely violated.
3.3.1. Multinomial logit model and its modeling
According to Verbeek (Citation2008), the common starting point is a random utility framework, in which the utility of each alternative is a linear function of observed characteristics plus an additive error term. Economic theory dictates that farmers adopt a single or a combination of technologies that can maximize their utility. This implies adoption occurs if the utility of the chosen package is higher than the utility of the other alternatives. However, the utility that gained from adopting agricultural technology is not observed but only its choice of technology, one can assume a random utility model which states conditional probability choice given farmers choice. To formalize this, consider the following latent variable:
Where: Aij* is a latent variable, which describes the ith farmer’s behavior in adopting the alternative package of technology J (j = 1, 2, … … .m) with respect to another alternatives K. Z’s are a vector of observed independent variables (household characteristics, farm-level factors, institutional factors, biophysical factors and technology aspects) and are unobserved characteristics which are relevant to the farm household’s decision maker but are unknown to the researcher such as expectation, skill, perception and motivation.
The farm household i will choose a package of j- technologies with respect to adopting any other technologies of k if it provides greater expected utility than any other alternative k, k ≠ j, i.e.
if =
(
*—
*) < 0.
It is assumed that the covariate vector of Zi is uncorrelated with the unobserved error term, i.e. E(
|
) = 0. Assuming that
are independent and Gumbel (identically) distributed (independence across utility functions and identical variance), that is under the independence of irrelevant alternatives (IIA) hypothesis; this model leads to the selection of a multinomial logit model.
Under adoption of multiple agricultural technologies, the number of alternatives that can be chosen is more than two; we can apply the multinomial discrete choice model to estimate simultaneously the effects of the explanatory variable on the adoption of different agricultural technologies. The variable Aij is a multiple choice variable and can be consistently estimated using a limited dependent variable (Maddala, Citation1986). There are two mostly usable multinomial probabilistic choice models, namely multinomial logit (MNL) and probit (MNP).
This study applied the MNL model over MNP since the model is simple to calculate the choice probability and computers can maximize the resulting likelihood function even for a large number of choices. And also the result obtained from the model is more stable than MNP when IIA fulfilled. (Kropko, Citation2008) also shows the MNL model nearly always provides more accurate and realistic results than MNP even if the IIA assumption is severely violated.
The probability of choosing alternative packages of J using MNL (Pij) can be computed as:
Where j =1,2 … J
3.3.2. Multinomial Endogenous Switching Regression Model (MESM)
This modeling is adapted from Asfaw and Shiferaw (Citation2010) and Kassie et al. (Citation2015). To evaluate the impact of agricultural technology adoption on poverty reduction, this study used MESM. The study estimated two outcome equations for with and without the applied technologies using MESR model. The model examines the relationship between the outcome variable (Yj) and covariate variables (Xj) for each of the chosen packages. Yj is observed when these alternative packages “j” has been adopted. In the study, there are eight technology combination choices, perhaps the simplest approach in multinomial data is to nominate one of the response categories as a baseline cell. The base category for this study is not-adoption any of agricultural technologies (F0M0H0), is denoted as j = 1. In the remaining combinations (j = 2, 3, 4 … … .m), at least one agricultural technology is adopted. To evaluate the poverty implications of Agricultural technology adoption for each regime combination of j strategy is defined as m regime given as:
Where are the poverty effect of farm household i in regime j and j (1, 2, … .m), Xi’s are a set of exogenous variables included in
, and
denotes error terms that capture the uncertainty faced by farmers and it is unobserved, and satisfies E(
) = 0 and constant variance of δj2.
In order to get consistent estimates, one can take into account the correlation between the error terms from the multinomial logit model estimated in the first stage and the error terms from outcome equation
. If the error terms are η’s and μ’s are not independent (E (
=0)) and identically distributed (with 0 mean and constant variance), a consistent OLS estimation of parameters requires the inclusion of the selection correction terms of the alternative choices in equation (4).
The consistent estimates can be obtained by estimating the following models:
Here, ν is the error term with an expected value of zero, is the covariance between
and
, λ is the inverse Mills ratio (for controlling selection bias) computed from the estimated probability (Pij) that the ith farm household chooses of package j.
3.3.3. Conditional expectations, treatment and heterogeneity effects
Switching regression models allows us to estimate adequate counterfactual situations, and one can estimate the average treatment effects of adoption (Kassie et al., Citation2015). Thus, the counterfactual is the effect of the treatment “adoption of strategy j” for adopters on the poverty outcome which would have obtained if the returns on their characteristics had been the same as the returns on the characteristics of the non-adopters, and vice versa. From equation (5), the following conditional expectations for each outcome variable can be computed:
Adopters with the adoption of j combination (actual): -
Adopters had they decided not to adopt (counterfactual):
Non-Adopters without technology adoption (actual):
Non- Adopters had they decided to adopt (counterfactual):
The conditional expectation outcome is used to calculate the transitional heterogeneity which is the difference between treatment effects on treated (TT) and untreated (TU). Since it helps to compare the expected consumption expenditure of the adopter with non-adopter, and in the counterfactual, adopted if did not adopt, and non-adopted if adopted. On the other way, to see whether the effect is larger or smaller for the actual adopter or for non-adopter in the counterfactual case. Base heterogeneity can also calculate for both adopter and non-adopter based on the prevalence some sort of difference like skill, knowledge, motivation among them. The following table tells us the treatment and heterogeneity effect. An efficient method to estimate MESM is obtained by using the “semi-log” command in STATA (Teklewold et al. (Citation2013). The conditional expectation, transitional and heterogeneity effect are presented in as follows.
Table 1. Conditional expectation, Transitional and Heterogeneity effect
3.4. Methods of poverty measuring
The study used total consumption expenditure per adult equivalent proxy to measure household poverty status. Poverty comparison also involves choosing a poverty line. This study used the national poverty line, which has been determined as total consumption poverty line. According to (National planing commision, Citation2017), the estimated national annual total consumption expenditure line is 7184 Birr per annum. Thus, the study used poverty line of 7184 birr per adult per annum. Those who spend below the threshold (7184 birr) are considered as poor. The assigned values equals to 1 for poor and 0 for non-poor households. The study also used poverty indices to estimate poverty headcount, gap and severity, as presented by (Foster et al., Citation2010).
3.5. Description of variables, measurement and expected sign
4. Results and discussion
4.1. Descriptive analysis
This study classified as adopters as households who used at least one technology in one of crop fields. The eight possible agricultural technology packages are summarized in . Of the total 656 sampled farm households, about 21.04% are non-adopters (F0M0H0), whereas 7.77%, 17.84% and 2.74% of them adopted only one technology such as adopters of inorganic fertilizer (F1M0H0), organic fertilizer (F0M1H0), herbicide (F0M0H1) respectively, and 19.82%, 8.84% and 3.35% of them are adopted a combination of two technology packages such as adopters of inorganic and organic fertilizer (F1M1H0), inorganic fertilizer and herbicide (F1M0H1), organic fertilizer and herbicide (F0M1H1) respectively, and lastly 18.6% of the farm households simultaneously adopted all of the three packages (F1M1H1).
Table 2. Description, measurement and expected sign of variables
Table 3. Description of Alternative Technology Packages
presents the descriptive statistics of the variables. The explanatory variables’ mean value of non-adopters (F0M0H0) is used as a base category to compare with mean values of alternative adopters of inorganic fertilizer (F1M0H0), organic fertilizer (F0M1H0), herbicide (F0M0H1), inorganic and organic fertilizer (F1M1H0), inorganic fertilizer and herbicide (F1M0H1), organic fertilizer and herbicide (F0M1H1) and a combination of organic fertilizer, organic fertilizer and herbicide (F1M1H1). The result shows that the mean comparison test between adopters and non-adopters are significantly larger for adopters and the values are different across the different alternatives.
Table 4. Descriptive summary of selected variables used in estimation
For instance, a set of household characteristics like gender of the household head (adopters are more of male headed), regarding education, adopters have high education level. Moreover, on average adopters have large family size than non-adopters. On the other way, the mean age of the household head for adopters is lower than the non-adopters.
Total farm size, livestock wealth measured in TLU, and off-farm employment are used as a means to describe the economic status of the household. Average farm size for non-adopter is lower than adopters. The mean livestock wealth and the proportion of households with off-farm activity participation for adopters are higher than non-adopters.
Moreover, Institutional factors such as extension contact, farm cooperatives, credit access, and saving are other factors which affect the adoption decision. The first two are related with farmers’ access to information on different packages and its profitability while access to credit and saving indicates farmers’ ability to finance their purchase of modern technology under cash constraints. The result shows these support and accesses are higher for adopters than non-adopters.
4.2. Econometric analysis
4.2.1. Factors determining the adoption of agricultural technology
The result from the MNL model is presented in . The base category is non-adoption (F0M0H0), where results of alternative packages are compared. The model fits the data reasonably well. The result indicates that the coefficient of age of household head is negative and significant for the adoption of full technology (F1M1H0) at 10% level of significance. The marginal effect indicates that as age of the household increases by a year, then the probability of adopting F1M1H0 decreases by 0.23%, other things unchanged. This implies that young farmers are more likely to adopt F1M1H0 since young farmers may have formal education than the non-adopter, less risk averse, more willing, and have greater flexibility in accepting new ideas, and consistent with Keba (Citation2019) and Abewa et al. (Citation2020).
Table 5. Maximum Likelihood Estimates for the multinomial Logit model
The coefficient of family size is positive and significant for the adoption of organic fertilizer (F0M1H0) and a combination of organic fertilizer and herbicide technology (F0M1H1) at 1%. The marginal effect shows that as the family member of the household increases by a unit, then the probability of adopting F0M1H0 and F0M1H1 increases by 4% and 0.6%, respectively, other things remain constant. This is because of the adoption of farm technology requires and attracts more labor force for agricultural activities, consistent with Shita et al. (Citation2020) and Habtewold (Citation2021). On the other hand, the coefficient of family size is also negative and significant for the adoption of inorganic fertilizer (F1M0H0), herbicide (F0M0H1), and full technologies (F1M1H1). The marginal effect shows that as the family member of the household increases by a unit, then the probability of adopting F1M0H0, F0M0H1, and F1M1H1 by 1.9%, 1.12% and 1.52%, respectively, other things remain constant. This may be because of the household with more family member weakens their economic status, and thus adoption decreases, this is in line with Sahu and Das (Citation2015).
Technological innovations and their adoption have also changed the way farm households regard employment choices (Fernandez-Cornejo et al., Citation2007). Various studies of technology adoption viewed off-farm income as influencing adoption of agricultural technology by providing additional income to finance purchase of agricultural technologies. In fact, agriculture is the source of livelihood for of the majority of people leaving in rural areas, some of the farmers are participated in non-farm working side by side to the agriculture activity. But, this is not to mean that adoption displaces labor significantly. Besides, in the country like Ethiopia, there is also a surplus labor which accommodates the activities and may not negatively affect the output (Nasir & Hundie, Citation2014). Thus, farmer’s already working off-farm may be more disposed to adopt agricultural technologies as it can enhance farm production by providing the finance needed for farm inputs and technologies. Consistently, the coefficient of off-farm employment participation in this study is positive and significant for the adoption of inorganic fertilizer (F1M0H0) at 1%. The marginal effect shows that as the household participated in off-farm activities increases the probabilities of adopting F1M0H0 by 19.6%, other things remain constant. This is because of participating in off-farm activities can generate income and solve the problem that the farm household’s face while intending to purchase farm technologies. This is consistent with Ayenew et al. (Citation2020).
The coefficient of TLU is positive and significant for the adoption of inorganic fertilizer (F1M0H0), organic fertilizer (F0M1H0), herbicide (F0M0H1), and full technology (F1M1H1).The marginal effect shows that as the household has a flock of livestock increases the probability of adopting F1M0H0, F0M1H0, F0M0H1 and F1M1H1 by 1.4%, 1.2%, 1.05% and 2%, respectively, other things unchanged. This is because of farmers who possess a flock of livestock are more likely to adopt than the have-not as it helps to get improved technology (as income means and source of organic fertilizer). This is consistent with Feyisa (Citation2020) and Belay and Mengiste (Citation2021).
The coefficient of distance to market is negative and significant for the adoption of a combination of inorganic fertilizer and herbicide (F1M0H1) at 5%. The marginal effect shows that as the distance to market increases by one kilometer, then the probability of adopting F1M0H1 decreases by 1%. The coefficient of distance to zonal town is negative and significant for the adoption of inorganic fertilizer (F1M0H0), organic fertilizer (F0M1H0), a mix of organic and inorganic fertilizer (F1M1H0) and full technology (F1M1H1). The marginal effect shows that as the distance to zonal town increases by one kilometer, then the probability of adopting F1M0H0, F0M1H0, F1M1H0 and F1M1H1 decreases by 0.1%, 0.1%, 0.8%, 0.8%, respectively. And the coefficient of distance to all weather roads is negative and significant for the adoption of organic fertilizer (F0M1H0) and full technology (F1M1H1). The marginal effect shows that as the distance to all weather roads increases by 1 km then the probability of adopting F0M1H0, F1M1H1 decreases by 0.7% and 0.3%, respectively, other things remain constant. This is because of that those framers with better access to the zonal town, the market and main road may buy (sell) agricultural inputs (outputs) on time and with a reasonable price are more likely to adopt farm technologies. The result is consistent with Ayenew et al. (Citation2020) and Belay and Mengiste (Citation2021).
The coefficient of extension visit is positive and significant for the adoption of all technology packages at 1%. The marginal effect shows that as the farm households get an extension visit increases the probability of adopting F1M0H0, F0M1H0, F0M0H1, F1M1H0, F1M0H1, F0M1H1 and F1M1H1 by 10.4%, 36.3%, 81.4%, 32.6%, 7.6%, 6.7% and 26.3%, respectively, other things remain constant. The coefficient of advisory service is positive and significant for the adoptions of inorganic fertilizer (F1M0H0) and a combination of organic and inorganic fertilizer (F1M1H0). The marginal effect shows that as the farm households get access of advisory services increases the probability of adopting F1M0H0 and F1M1H0 by 7.9% and 5.3%, respectively, other things remain constant. This is because the extension visit and advisory services helps the farmers to raise their awareness about the characterization and attributes of the technology, use and their impact. Extension gives detailed information, training and advisory services about the source, use and importance of the technologies to the farmers and engaging in input distribution. Advisory service on the other hand indicates that having access to regular and frequent advisory services by development agents, farm cooperatives and meetings plays a fundamental role in the dissemination and adoption of farm technology. This is in line with Belete and Melak (Citation2018), Belay and Mengiste (Citation2021), and Habtewold (Citation2021).
The coefficient of access to credit is a positive and significant for the adoptions of organic fertilizer (F0M1H0), herbicide (F0M0H1) and a mix of inorganic fertilizer and herbicide (F1M0H1). The marginal effect shows that as the farm households get access of credit increases the probability of adopting F0M1H0, F0M0H1 and F1M0H1 by 10.5%, 76% and 7.3%, respectively, other things remain unchanged. This is because of credit access solves income problems that household could face while they want to purchase agricultural technologies; and hence paves the way for timely application of modern farm inputs, consistent with Abewa et al. (Citation2020) and Shita et al. (Citation2020).
The coefficient of tenure security is positive and significant for the adoption of a mix of organic and inorganic fertilizer (F1M1H0) at 5%. The marginal effect shows that as the households have the rights of their own plots increases the probability of adopting F1M1H0 by 12.7%, other things remain constant. This is due to the fact that when farmers are secured for their own land, the more likely participated in the adoption practice since they can make long-term investment, consistent with Mohammed (Citation2014).
Finally, the coefficient of plot distance from the homestead is negative and significant for the adoptions of F1M0H0, F0M1H0, F1M1H0 and F1M0H1. The marginal effect shows that as the distance of plots increase by one kilometer, then the probability of adopting F1M0H0, F0M1H0, F1M1H0 and F1M0H1 decreases by 3.9%, 6.3%, 5.4% and 6.8%, respectively. This is potentially because of as the distance to plot is far away from the homestead, the less likely will be on time plot preparation, weeding, harvesting and input utilization and hence farm households are less likely to adopt agricultural technologies.
The coefficient of plot potential wetness index is positive and significant for the adoption of full technologies at 1%. The marginal effect shows that a unit increases in the plot potential wetness index increase the probability of adoption by 3.9%. This is because of as the wetness of the plot increases (maintains vascular plant species richness, soil pH, groundwater level and soil moisture) the more likely the adoption of the agricultural technology. These findings are consistent with Sebsibie et al. (Citation2015) and Mesele (Citation2019).
4.2.2. The impact of agricultural technology adoption on poverty
The impact of agricultural technology adoption on consumption expenditure (poverty proxy) is presented in . The study compares the value of consumption expenditure under the actual case that had adopted and the counterfactual case which had not adopted. The table presents the conditional average treatment effects of adoption of a combination of alternative packages on total consumption expenditure per adult equivalent.
Table 6. Conditional Average Treatment Effects of Adoption on consumption expenditure (ETB)
The actual value indicates that adopters of alternative technologies have higher total consumption expenditure per adult equivalent than they had not adopted. This confirms that adoption gives larger total consumption per adult relative to non-adoption. That is, adopters who actually adopted increase their consumption expenditure per adult, and, if those who do not currently adopted were to adopt it, their consumption expenditure per adult would increase as well. Within adopters, the highest annual total consumption expenditure per adult equivalent are obtained when farm households adopt a full technology (F1M1H1) which is 4918.85 birr; a combination of herbicide (F0M0H1) which is 4595.76 birr; followed by adoptions of organic fertilizer and herbicide (F0M1H1) which is 4060.80 birr. The second highest annual total consumption expenditure per adult are found when farm households adopt a combination of organic and inorganic fertilizer (F1M1H0) which is 3893.26 birr, followed by adoptions of inorganic fertilizer (F1M0H0) which is 3868.87 birr, adoptions of inorganic fertilizer and herbicide (F1M0H1) which is 3681.52 birr. The lowest is obtained when adopters adopt organic fertilizer (F0M1H0) which is 3592.74 birr. But, all the adopters obtained higher consumption expenditure per adult as compared to the non-adopters (F0M0H0) which is 3388.32 birr. Moreover, the highest value of consumption expenditure is obtained from the joint adoption of alternative agricultural technologies than adopting in single technology, implicating complementarity in benefits.
According to the result of average treatment effect on treated (ATET)Footnote4, farm households who actually adopted alternative agricultural technologies significantly would have lower consumption expenditure per adult equivalent respectively if they had not adopted. On the other hand, the results of average treatment effect on untreated (ATEU)Footnote5 indicates, farm households who did not adopt (F0M0H0) would significantly increase their total consumption expenditure per adult if they had decided to adopt alternative agricultural technologies. This indicates that non-adopters who actually had not adopted would gain a higher percentage of weight if they were to adopt it, compared to the weight gained by their counterparts who actually had adopted. But the actual values indicate adopters have lower consumption expenditure per adult equivalent than they had not adopted.
The significant values of base heterogeneity for non-adopter (BH0) and adopters (BH1) presented in , shows that there is some sort of heterogeneity among adopters and non-adopters. For example, the negative values of BH0 of non-adopter imply that adopters who actually adopted would have more total consumption expenditure than who had not adopted, and the negative value of BH1 of adopters indicates that non-adopters would have more total consumption expenditure than those who actually had adopted. The negative significant transitional effect (TH) values confirmed that the effect of adoption would be significantly lower for the farm households who actually adopted relative to those who had not adopted, if they had adopted.
4.2.3. Poverty measures by technology adoption
This study used the national poverty line which has been determined as total consumption poverty line of 7184 birr per adult per annum (National planning commissions, 2017). presents the incidence, gap, and severity of poverty. The result shows that poverty headcount, gap and severity were lower among adopters than non-adopters; implicating that adoption had a poverty reduction effect.
Table 7. Poverty Measures by Technology Adoption
Thus, the result of the study confirms that adoption of alternative agricultural technologies have a positive impact on households total consumption expenditure per adult equivalent, and confirms the potential direct role of adopting agricultural technologies on reducing poverty, as higher farm consumption expenditure from adopting agricultural technology translate into lower consumption poverty. So, adoptions of alternative agricultural technologies can be taken as a favourable way to lift out poor farm households from poverty. The study’s finding is consistent with the works of Sahu and Das (Citation2015), Sebsibie et al. (Citation2015), Ayenew et al. (Citation2020), and Natnael (Citation2019), Biru et al. (Citation2020), Belay and Mengiste (Citation2021) and Wordofa et al. (Citation2021).
5. Conclusion and recommendation
The study examined the impact of adoption of multiple agricultural technologies on poverty in rural Amhara region of Ethiopia. The study used the Ethiopian socioeconomic survey data and 656 farm households were selected. The study used multinomial logit to identify the factors of adoption and multinomial endogenous switching regression model to measure the welfare effect of adoption. Using a multinomial logit model, the study found that households decision to adopt multiple agricultural technologies are positively and significantly affected by household size, off-farm activity, livestock wealth, farmers contact with extension and advisory service, farmers having credit access, farmers secured for their own land, and plot potential wetness index. On the other hand, the adoption decision is negatively and significantly affected by age of the family head, household size, distance [from market, zonal town and road] and plot distance from the homestead. Using a multinomial endogenous switching regression model, the study found that, adopters have higher annual total consumption expenditure per adult equivalent and have lower headcount, depth and severity than the non-adopters. And also, adoption of combined technology packages provides higher annual total consumption per adult as compared to adopting a single technology. Finally, the results verify that the positive and significant impact of adoption of alternative agricultural technologies on poverty reduction.
Thus, in terms of policy implication polices for strengthening and promoting the adoptions of alternative agricultural technologies increases the consumption of the farm household and thereby reduces poverty. Specifically, policy iinterventions should revisit how devising access to extension visit and advisory services to increase the awareness of farmers about the characteristics, the use and impact of agricultural technologies; how credit is accessed, used and returned by farmers; how the government increases the access of infrastructure like roads for the farm households, and secures the land ownership right for the far households so as to encourage adoption widely. Moreover, off-farm activity participation is positively associated with the adoption decision of fertilizer technology through proving an extra income to buy agricultural technologies. Thus, intervention should be there to encourage off-farm works without affecting the agricultural production and productivity. Moreover, there should be due attention to rural households to have and promote a better targeting of agricultural technology adoption and in turn to reduce poverty and to improve food security of the rural society.
Acknowledgements
The author would like to thank Debre Berhan University for material support.
Additional information
Funding
Notes on contributors
Mesele Belay Zegeye
Mesele Belay Zegeye, the author of this article, is a lecturer at Debre Berhan University, Ethiopia. He earned BA in Economics and MSc in Development Economics from Debre Berhan University. His research interest includes impact and effectiveness of policies, poverty, food security, technology adoption, and agricultural innovations and extensions, and agricultural productivity.
Notes
1. Multinomial Logit.
2. Multinomial Endogenous Switching Model.
3. Adoption refers a farm household who used at least one technology in one of crop fields.
4. ATET is the difference in expected outcome of adopters when they decided to not to adopt..
5. ATEU is the difference in expected outcome of non- adopters when they decided to adopt..
References
- Abewa, D., Merie, H. E., & Kassa, M. (2020). Prevalence and associated factors of agricultural technology adoption and teff productivity in Basso Liben District, East Gojjame Zone, North west Ethiopia. bioRxiv. https://doi.org/10.1101/2020.10.28.358770
- Asfaw, S., & Shiferaw, B. A. (2010). Agricultural technology adoption and rural poverty: Application of an endogenous switching regression for selected East African Countries ( No. 308-2016-5081).
- Ayenew, W., Lakew, T., & Kristos, E. H. (2020). Agricultural technology adoption and its impact on smallholder farmer’s welfare in Ethiopia. African Journal of Agricultural Research, 15(3), 431–20. https://doi.org/10.5897/AJAR2019.14302
- Belay, M., & Mengiste, M. (2021). The ex‐post impact of agricultural technology adoption on poverty: Evidence from north Shewa zone of Amhara region, Ethiopia. International Journal of Finance & Economics, 25, 1–11. https://doi.org/10.1002/ijfe.2479
- Belete, B., & Melak, S. (2018). Impacts of small-scale irrigation technology on the nutritional well-being of children in the Amhara national region of Ethiopia. Ethiopian Journal of Economics, 27(1), 29–56.
- Biru, W. D., Zeller, M. and Loos, T. K. (2020). The Impact of Agricultural Technologies on Poverty and Vulnerability of Smallholders in Ethiopia: A Panel Data Analysis. Social Indicators Research, 147(2), 517–544.
- Dercon, S., Hoddinott, J., & Woldehanna, T. (2007). Growth and Poverty in Rural Ethiopia: Evidence from 15 Communities 1994–2004. Background Paper for the Chronic Poverty Report 2008/09. Chronic Poverty Research Centre.
- Diriba, G. (2020). Agricultural and Rural Transformation in Ethiopia: Obstacles, Triggers and Reform Considerations.
- ESS. (2015/16). Ethiopia Socioeconomic Survey, Wave 3 (ESS3) 2015-2016. https://datacatalog.worldbank.org/dataset/ethiopia-socioeconomic-survey-2015-2016
- Fernandez-Cornejo, J., Mishra, A. K., Nehring, R. F., Hendricks, C., Southern, M., & Gregory, A. (2007). Off-farm income, technology adoption, and farm economic performance ( No. 1477-2016-121070). United States Department of Agriculture (USDA).
- Feyisa, B. W. (2020). Determinants of agricultural technology adoption in Ethiopia: A meta-analysis. Cogent Food & Agriculture, 6(1), 1855817. https://doi.org/10.1080/23311932.2020.1855817
- Foster, J., Greer, J., & Thorbecke, E. (2010). The Foster–Greer–Thorbecke (FGT) poverty measures: 25 years later. The Journal of Economic Inequality, 8(4), 491–524. https://doi.org/10.1007/s10888-010-9136-1
- Habtewold, T. M. (2021). Impact of climate-smart agricultural technology on multidimensional poverty in rural Ethiopia. Journal of Integrative Agriculture, 20(4), 1021–1041. https://doi.org/10.1016/S2095-3119(21)63637-7
- Kassie, M., Teklewold, H., Marenya, P., Jaleta, M., & Erenstein, O. (2015). Production risks and food security under alternative technology choices in Malawi: Application of a multinomial endogenous switching regression. Journal of Agricultural Economics, 66(3), 640–659. https://doi.org/10.1111/1477-9552.12099
- Keba, A. (2019). Review on Adoption of Improved Agricultural Technologies in Ethiopia. Policy, 4(1), 11–19. doi: 10.11648/j.hep.20190401.12
- Kropko, J. (2008). Choosing between multinomial logit and multinomial probit models for analysis of unordered choice data. https://doi.org/10.17615/wz24-qq92
- Maddala, G. S. (1986). Limited-dependent and qualitative variables in econometrics (No. 3). Cambridge university press.
- McCalla, A. F. (2001). Challenges to world agriculture in the 21st century. J. Agricultural Resource and Economics, 4, 3. University of California, Davis.
- Mesele, B. Z. (2019). The Impact of Agricultural Technology Adoption on Poverty Reduction in Rural Ethiopia (thesis). Debre Berhan University- Health Science National Academic Digital Repository of Ethiopia. https://doi.org/10.20372/nadre/6315.
- Mohammed, A. (2014). Adoption of Multiple Sustainable Agricultural Practices and Its Impact on Household Income: Evidence from Maize-Legumes Cropping System of Southern Ethiopia. International Journal of Agriculture, 196–203.
- Nasir, M., & Hundie, B. (2014). The effect of off farm employment on agricultural production and productivity: Evidence from Gurage Zone of Southern Ethiopia. Journal of Economics & Sustainable Development, 5(23), 85–98.
- National Planning Commission. (2017). Ethiopia’s progress towards eradicating poverty. An Interim Report on 2015/16 Poverty Analysis Study.
- Natnael, A. B. (2019). Impact of Technology Adoption on Agricultural Productivity and Income: A case study of Improved Teff Variety Adoption in North Eastern Ethiopia. Agri Res & Tech: Open Access J, 20(4), 556139. https://doi.org/10.19080/ARTOAJ.2019.20.556139
- Portal, E. G. (2019). Amhara Regional State, retrieved from:
- Sahu, S. K., & Das, S. (2015). Impact of agricultural related technology adoption on poverty: A study of select households in India. Working Paper 131/2015. India Studies in Business and Economics. Springer, Singapore.
- Sebsibie, S., Asmare, W., & Endalkachew, T. (2015). Agricultural technology adoption and rural poverty: A study on smallholders in Amhara regional state, Ethiopia. Ethiopian Journal of Economics, 23(683–2017–950), 117–156. doi: 10.22004/ag.econ.259413
- Shita, A., Kumar, N., & Singh, S. (2020). The impact of agricultural technology adoption on income inequality: A propensity score matching analysis for rural Ethiopia. International Journal of Information and Decision Sciences, 12(1), 102–114. https://doi.org/10.1504/IJIDS.2020.105013
- Tefera, T., Tesfay, G., Elias, E., Diro, M., & Koomen, I. (2016). Drivers for adoption of agricultural technologies and practices in Ethiopia. CASCAPE project report no. NS_DfA_2016_1, Addis Ababa/Wageninge.
- Teklewold, H., Kassie, M., & Shiferaw, B. (2013). Adoption of multiple sustainable agricultural practices in rural Ethiopia. Journal of Agricultural Economics, 64(3), 597–623. https://doi.org/10.1111/1477-9552.12011
- Verbeek, M. (2008). A guide to modern econometrics. John Wiley & Sons.
- Wordofa, M. G., Hassen, J. Y., Endris, G. S., Aweke, C. S., Moges, D. K., & Rorisa, D. T. (2021). Adoption of improved agricultural technology and its impact on household income: A propensity score matching estimation in eastern Ethiopia. Agriculture & Food Security, 10(1), 1–12. https://doi.org/10.1186/s40066-020-00278-2