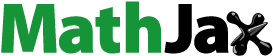
Abstract
Despite the extensive literature on rural poverty outcome, the labour employment channel has not been carefully investigated for rural Nigeria. The paper used the socioeconomic data from the three waves of the Nigerian General Household Survey Panel (2010/2011, 2012/3013 and 2015/2016 to examine the nature of household employment. By exploiting the panel nature of the data, we used a logit model and fixed effect approach to analyze the factors determining the employment expansion and earnings. From the descriptive statistics, agricultural employment sectoral share remains substantial but declined over the period considered. The study finds that much of the employment in rural Nigeria during the period 2010 to 2015 is farm self-employment. Though declining, it is two times the non-farm employment share and five times wage employment share. Wage employment is least and declining during the period covered. Findings from the econometric analysis suggest rural programmes that promote rural infrastructural, human capital development, networking, higher income and the diversity of income. It is an imperative policy goal to create an enabling environment for productivity and employment-intensive growth across all sectors of the economy particularly in agriculture. .
PUBLIC INTEREST STATEMENT
Nigeria’s population is projected to increase to 400 million by 2050. While it offers potential opportunity as a source of labor supply, there is the disturbing concern of a looming employment crisis. Already unemployment is worsening in the rural sector where poverty is a phenomenon. Employment and income opportunities for the rural population are channels for poverty reduction. To transform economic growth into more employment and income opportunities, reforms are needed across multiple labor subsectors including utilization of improved technologies, investment in skill and problem-solving levels for the labor force, lower transactions costs to connect and integrate economic activities, and more efficient allocation of resources.
1. Introduction
Previous studies have examined the drivers of household poverty in Nigeria using various indicators of consumption, income, and asset (see Odozi et al., Citation2015; Odozi & Oyelere, Citation2019; Olaniyan & Abiodun, Citation2005; Omonona, Citation2009). While these studies show that poverty is prevalent, there is little empirical information on the determinants of household employment and earnings in rural Nigeria. An understanding of rural labor markets requires considering employment from the perspective of a combination of livelihood opportunities. Labor is an important resource that is easily accessible to rural households in Nigeria as in many countries in Africa and much of it is self-employed labor. Employment offers the opportunity of raising rural incomes, asset build-up, and resilience to shocks of various kinds. Thus understanding the determinants of employment and earnings as channels for poverty reduction is informative.
The paper uses robust econometric techniques to analyze the determinants of employment and earnings in rural Nigeria. While this paper is similar to several studies done for Nigeria and the rest of Africa (Aminu, Citation2010; Awoyemi, Citation2011; Broeck & Kilic, Citation2018; Shittu, Citation2014), it contributes to the literature by identifying the determinants of labor employment holistically using a panel data. The multi‐topic nature of the data set also permits pairing our key response variables with relevant covariates at the household, individual and community levels. We focus more on the rural sector because it is the sector where poverty is a phenomenon. Hence, our study is relevant to the Sustainable Development Goals (SDGs) 1 to end extreme poverty by 2030 and boost shared prosperity of the bottom 40% of the population. It is also relevant to several rural transformative programsFootnote1 launched and targeted at the household by Nigeria’s government.
This paper proceeds as follows. Immediately after the introduction in section one is section two that gives a background of the rural labor market, section three gives the empirical strategy while section four presents the results and discussion while Section five presents conclusions, limitations, and further studies.
2. Background
As stipulated in the International Labour Organization Employment Policy Convention of 1964, countries are to pursue an active policy designed to promote full, productive and freely chosen employment.
In Nigeria several sectors and subsectors contribute to the country's economic activity and much of it is self-employment in the informal sector. Farm sector employment continues to play a central role in Nigeria’s rural economy,Footnote2 even though off-farm employment has been increasing (J. C. Odozi et al., Citation2018). The growing importance of off-farm employment in the informal sector can be viewed as a household diversification strategy against various risks and liquidity constraints that households experience while engaging in agriculture. According to Odozi et al. (Citation2010, p. 191), “most households cannot exit the farm sector entirely since participation in off-farm activities is equally constrained”.
Informal sector employment remains pervasive throughout the developing world. Based on the latest available data for 54 developing countries, in three-quarters of countries, more than half of those with jobs in non-agricultural sectors are informally employed (Sustainable Development Goals [SDGs], Citation2019 report). In Nigeria, the National Bureau of Statistics put the size of the informal sector as of 2010 at 54,643, 676 million persons (National Bureau of Statistics (NBS), Citation2010). Given the increasing economic downturn and the declining share of public and private sector formal wage employment over the last decade, the size of the informal sector has grown astronomically in Nigeria.
Various macroeconomic and within-sector reforms continue to contribute to the dynamics of the rural labor market. For example, the government-led-industrialization policy in the 1970s, the structural adjustment policy of the 1980s, and the diversified economy re-form of the late 1990s and early 2000s (see Okonjo-Iweala & Osafo-Kwaako, Citation2007). Poverty is a consequence of the inefficiency of the rural labor market and it is one of the biggest economic and social challenges that households frequently experience. Although economic growth as posited by economic theory is necessary for poverty reduction, the “employment absorption capacity of growth” (see Ajakaiye et al., Citation2016; Odozi et al., Citation2018) is also important. Furthermore, Odozi and Oyelere (Citation2019) document that despite positive economic growth between 2001 and 2014 after decades of economic stagnation, Nigeria has been experiencing negative economic growth since 2015 which stands in contrast to many other African countries that have experienced positive economic growth in recent years. They alluded that though poverty has been on a decline in Nigeria since the start of the twenty-first century, the percentage of people living in extreme poverty is still very high. With the development of the National Employment Policy produced in 2002, creating an enabling environment for productivity and employment-intensive growth across all sectors of the economy is an imperative policy goal.
3. Literature review
The International Labour Organization defines employment as all persons of working age working for pay, profit, or family gain and includes all persons having a job or enterprise (ILO, Citation2013a, Citation2013b). In Nigeria, the National Bureau of Statistics categorizes employment types as (1) paid employment (2) self-employment in farming, (3) self-employment in non-farming, (4) Paid apprentice, and (5) unpaid household worker. Within the context of a rural livelihood framework, households can be viewed as having earnings from various sources of rural employment.
Self-employment – entrepreneurs and survivalists – is pervasive in rural economies and consists of individuals who bear their own risk and are not remunerated by wage or salary but earnings from their own account economic activities. For example, “the farm household is characterized by a dual role in producing marketable output and coordinating consumption of its members through the allocation of time between work (on-farm and/or off-farm) and leisure” (Chang et al., Citation2012). Despite their roles, systematic empirical analysis of the determinants is still few.
The integrated consumption and labor supply behavior model has been extensively used in the study of rural employment. The model accommodates labor market imperfections and jointness of livelihood activities and decisions and is, therefore, able to analyze both the demand and supply aspects of the rural labor market. The variant of this model has been applied to estimate the demand and supply sides of rural employment independently under the model separation proposition. The wage determination model has also been applied in the analysis of rural employment where the dependent variable is typically logged hourly wages.
Several empirical studies exist for Nigeria and rest of the world using the aforementioned models. Aminu (Citation2010) used the wage determination model to examine the factors determining wage employment in private, public formal sectors, and informal sectors. The paper finds an increasing effect of an aged female household member, urban location, household size, and education on employment participation. Giannetti and Andrei (Citation2004) review the literature on the determinants of self-employment across Swedish municipalities. The study finds individual characteristics and business environment as the most important factors explaining self-employment. Using a rational choice approach to self-employment and linked to a reduced form model, Carlos et al. (Citation2011) postulated three categories of self-employment determinants: namely (i) monetary parameters such as initial wealth; (ii) individual abilities, tastes, and preferences; and (iii) institutions and macroeconomic conditions. Hung et al. (Citation2019) reviewed various literature and noted the following variables as important in explaining household income and employment (i) household size, dependency ratio, gender, age, and education of household head; (ii) owned farmland size per capita; the log of total values of all fixed assets; (iii) participation in non-farm self-employment and wage employment activities; (iv) the presence of means of transportation, paved roads, post offices, electricity, local markets, and (v) the provincial dummy variables which control for provincial fixed effects.
Broeck and Kilic (Citation2018) analyzed the determinants of employment within the context of off-farm employment growth. The paper aggregated off-farm wage and non-farm enterprise employment types to explain the determinants of off-farm employment across several countries in Africa including Nigeria. The paper highlights push and pull factors where push factors entail different forms of shocks, lack of agricultural productive assets, and social factors. The pull factors are characterized by available markets and opportunities, infrastructural facilities and supportive institutions, Human capital, initial non-productive asset holdings, access to credit and savings, and a larger social network.
Similarly, Ackah (Citation2013) examined the determinants of non-farm employment and finds a positive association with higher education levels, better access to infrastructure, and public services (road, market, energy, water, etc.). Himanshu et al. (Citation2013) show that diversification into rural non-farm employment increased household income using both long-term survey data in Palanpur, a village in western Uttar Pradesh, and the NSS data. Imai et al. (Citation2015) in their study looking at non-farm employment and poverty examined female and male wage rates, household composition, education attainment among other factors as determinants of non-farm employment. They find wages to be positively significant in affecting non-farm employment. Chang et al. (Citation2012) in their study investigated how changes in economic conditions and government policies affect off-farm labor supply. They find how farm households respond to lower-income payments by increasing their off-farm labor supplies. Laszlo (Citation2005) focused on non-farm self-employment ventures in rural Peru and documents the important roles of education and local market development. From the review of empirical studies, several factors have been identified as determinants of employment and earnings at the micro level. This paper adds to the literature by examining the determinants of rural labour employment in rural Nigeria from a holistic perspective. Furthermore, the idea of social capital as an important factor determining earnings and employment in developing countries like Nigeria is missing from previous studies. Hence an important contribution of this paper is the inclusion of social capital variable in an attempt to fill the gap in knowledge.
4. Materials and methods
4.1. Analytical framework
The study employs household-level data and an integrated model of consumption and labor supply behavior to determine the drivers of employment and earnings. Household is classified as employedFootnote3 using common questions across all three waves that ask” whether persons worked for (1) someone (2) worked on a farm owned or rented (3) worked on their account. The working-age population (15–65) was included using the age variable of respondents. There was no clear variable to identify persons who were recently laid off, absent from work temporarily such as persons who were sick, on vacation, on maternity leave, on strike, or temporarily laid off. First, we estimate the determinants of employment at the extensive margin defined as individuals or households who participated in the farm, wage, and non-farm employment during a reference period. EquationEquation 1(1)
(1) captures the employment model at the extensive margin of household i at time t.
In Equationequation 1(1)
(1)
the probability of household or individual i in employment.
is a dummy variable and it takes the value of 1 if a household is employed and 0 otherwise. Yi captures household characteristics to control for household preferences and includes age and level of education of the household head, gender, and household size. We use years of household schooling as a proxy for human capital and skills. Cj is the vector of community characteristics. We also included the dependency ratio to account for the non-working population. The dependency ratio was computed as the ratio of the population below 5 plus above 65 years to the population between 15 and 65 years. Other variables included are community labor wages. The dependency burden is seen as important because it creates subsistence pressure to work more hours. Education creates status considerations and an aversion to some types of employment. It thus may reduce the labor supply. We estimate equation one using logit regression. Given the difficulty in interpreting logit estimates directly, we calculate marginal effects. To analyze employment at the intensive margin, that is the determinants of earnings and hours worked we make use of a Heckman selection model and a fixed-effects (FE) approach. The selection equation is captured with Equationequation (2)
(2)
(2) and the outcome equation is captured with Equationequation (3)
(3)
(3) .
Equationequation 2(2)
(2) ,
captures labor force participation of household heads i, and
captures hours worked by household head or earnings. EquationEquation 2
(2)
(2) captures the selection of households into employment. For this equation, the dependent variable is a dummy variable and it takes the value of 1 if a household is in employment and 0 otherwise. The second equation is the outcome equation using earnings and hours of work.
are state fixed effects,
are the interaction between zone and time fixed effects and
represents the error term. The Heckman methodology has been commonly used to eliminate the potential self-selection bias that arises if those participating are systematically different from those who are not participating. For instance, in our case, there could be unobserved effects, i.e. earning or hours worked is only observed when an individual selects to be in the labor force. Heckman model thus ensures unbiased estimates of β through incorporating the omitted variable (termed as the inverse Mills Ratio) into the model to control for selectivity bias issues. This is the key advantage over Ordinary Least Squares (OLS) estimation. The limitation of Heckman methodology however in addressing other sources of selectivity bias, endogeneity, for example, leads us, for more robust analysis, to use the fixed effects (FE) approach. Using panel data and a fixed effect strategy attenuates biases in coefficients and increases the likelihood that estimated effects are consistent (Odozi & Oyelere, Citation2021). The FE model can be specified as follows.
where a vector of individual and household regressors that affect hours is worked and
represents time-varying local government area characteristics such as the rainfall levels and population density.
are time-invariant household-specific effects that could be correlated with the observed covariates.
are year fixed effects;
are zone time trends,
is the idiosyncratic error term.
is the parameter of interest to be estimated and captures the effects of the regressors on earnings and hours worked, respectively. The fixed effect approach accounts for time-invariant characteristics of households that could be correlated with the regressors and our variable of interest-hours worked and earnings.
4.2. Data
The main source of data is the Nigerian General Household Survey Panel (GHS-Panel). As noted on the World Bank’s Central Microdata Catalog website, the GHS-Panel is implemented in collaboration with the World Bank Living Standards Measurement Study (LSMS) team as part of the Integrated Surveys on Agriculture (ISA) program and was revised in 2010 to include a panel component (GHS-Panel). The survey was undertaken by the National Bureau of Statistics in partnership with the Federal Ministry of Agriculture and Rural Development (FMARD), the National Food Reserve Agency (NFRA), the Bill and Melinda Gates Foundation (BMGF), and the World Bank (WB). All sample households were administered a multi-topic Household Questionnaire. The information is provided for post-planting/pre-harvest and post-harvest outcomes. There are four waves currently of the panel (2010, 2012, 2015, and 2018) and the GHS-Panel is a nationally representative survey of approximately 5,000 households, which are also representative of the geopolitical zones in Nigeria at both the urban and rural level. We used the labor module questions to determine the employment of individuals. The module provides information on whether an individual was employed during the last 7 days. The present study focuses on a subsample of individuals in rural areas aged 15 or over who were in work (even if unpaid) at the time of the survey. The data set also includes several specific household and individual characteristics. We also use the information on rainfall and population density in our analysis.
4.3. Description of variables and summary statistics
In , all variables used in the analysis are presented with their definitions and nature, Wwhile presents the correlation matrix for core variables. presents the summary statistics of the variables. The continuous variables are Log earnings; log hours worked, age household size, number of children below 5 years of age, number of children between 5 and 14 years of age and number of adults between 15 and 65 years of age, years of school, rainfall population density and labor wage. Except for rainfall, population density, and labor wage which are at the LGA level, the other continuous variables are at the household level. Employment category variables are dichotomous. We construct infrastructure and social capital indices using various community-level infrastructural and social capital variables by applying principal component analysis. The broad concept of infrastructure and social capital is given by the first principal component of the underlying variables. This is necessary since some of the variables interrelate with each other. In empirical estimation, such correlations make it difficult to obtain reliable estimates of the individual coefficients of variables representing different kinds of infrastructure. We also used the same approach to construct an income diversification index.
Table 1. Definition and nature of variables used
Table 2. Correlation matrix of core variables
Table 3. Summary statistics of variables included in the regressions
5. Results and discussion
5.1. Descriptive statistics
presents the employment share in percentage across various sectors and years in rural Nigeria. From the table, agriculture is the predominant sector in terms of the number of households employed in rural Nigeria. Column 5 of the table presents the pooled average across the 3 years (2010, 2012, and 2015) considered. For agriculture, the pooled average share is 70.31%. While the agricultural employment share for 2010 is marginally below the pooled average for agriculture, it declined by 3.43% points in 2015, from 71.33% to 67.9%. Buying and selling employment comes after agriculture. Although not shown in , 31.4% of urban households were employed in buying and selling (trade) compared to the rural estimate at 8.28% in 2010. The urban estimate is over three times higher. We aggregate the various employment types into wage Employment, farm self-employment, Non-Farm Wage Employment (NFWE), and Non-Farm Self-Employment (NFSE). From the Table, Farm self-employment is the dominant employment type, followed by non-farm self-employment and wage employment in that order. We graph the mean earnings for these aggregates for each year and across years as shown in .
Table 4. Percentage employment share, 2010–2015 (RURAL)
shows the graph of the distribution of Log earnings of rural households using the non-parametric kernel density estimates. Thus, shows the Kernel density distribution of mean earning by aggregate employment types for each year (2010, 2012, and 2015) and the pooled average across years (2010–2015). The whole idea of the graph is to visualize the trend across the entire range of the distribution. The Epanechnikov kernel is used because it is optimal among non-negative kernels in minimizing the integrated mean square error (Mills and Hazarika, 2003). From the figure, relative to other aggregate employment types, a slight rightward shift is observed for Non-Farm Wage Employment (NFWE) across the entire range of earnings. Studies have shown that mean earnings in non-agriculture are higher than for agriculture.
5.2. Determinants of employment types and earnings
presents the marginal estimates of the logit regression for aggregate employment types: (1) Wage employment, (2) Farm self-employment, (3) Non-farm self-employment and for the pooled sample, all at the extensive margin. The marginal estimates are presented in columns (1), (2), (3), and (4). The wage employment (column 1) reveals a 0.17% probability increase in wage employment that is statistically significant at p < 0.01 when households increased schooling by 1%. Also, we find a 0.32% increase in wage employment that is statistically significant at p < 0.01 for a unit expansion in infrastructure. In column 1, we find the age of the household head and income diversification index to be negatively significant at p < 0.05 and p < 0.01 respectively. This suggests that the more the age of household head, the less likely households will engage in wage employment. For farm self-employment estimates in column (2), labor wage and rainfall were positively significant at p < 0.01 and p < 0.1. Other variables are income diversification, social capital and the number of adults per household are significantly positive at p < 0.01, p < 0.1, and p < 0.05 respectively. Variables that are significant but negative are age (p < 0.01), infrastructure index (p < 0.01), and years of schooling (p < 0.01). For non-farm self-employment estimates in column (3) infrastructure was positively significant at p < 0.01. Non-farm self-employment increased by 0.28% with a unit expansion in infrastructure. Exposure to shock also led to more non-farm self-employment by increasing employment by 0.09%. Other variables that are positively correlated with non-farm employment include rainfall (p < 0.01) while increasing labor wage reduced non-farm self-employment at p < 0.1.
Table 5. Logit regression correlates of employment (Extensive margin)
presents the log earning and log hours worked regression results for the Heckman and Fixed Effects models model using only the pooled sample. These estimates provide results that focus on the intensive margin of employment. For the Heckman models, we report only the outcome estimates and not the selection estimates. The Wald test of the independence of equations suggests that conducting a Heckman selection model may not be necessary as we fail to reject the hypothesis that p = 0. In columns (1) and (2) we present estimates for household head log earning under the Heckman and fixed-effects models. In columns (3) and (4) the estimates for the log hours worked are presented. The results in columns (1) and (2) suggests that an increase in age as a measure of experience, years of schooling, a household with children less than 5 years correlated with a significant increase in earnings while social capital increased the hours worked. These findings are generally consistent with prior expectations. Aminu (Citation2010) finds an increasing effect of aged female household members, urban location, household size, and education on wage employment participation in private, public formal sectors, and informal sectors. Also, Giannetti and Andrei (Citation2004) find individual characteristics and business environment as the most important factors explaining self-employment. Van den Broeck and Kilic (Citation2018) noted the importance of push and pull factors in determining rural employment. The pull factors are characterized by available markets and opportunities, infrastructural facilities and supportive institutions, Human capital, initial non-productive asset holdings, access to credit and savings, and a larger social network while the push factors include shocks, lack of agricultural productive assets, and social factors. Ackah (Citation2013) finds a positive association between non-farm employment and higher education levels, better access to infrastructure, and public services (road, market, energy, water, etc.). Himanshu et al. (Citation2013) show that diversification into rural non-farm employment increased household income. Imai et al. (Citation2015) find wage to be positively significant in affecting non-farm employment. Chang et al. (Citation2012) find how farm households respond to government policies measured as lower-income payments by increasing their off-farm labor supplies. Our results point to factors such as years of schooling, level of infrastructure, social capital and income diversification, labor wage as drivers of increased rural employment and earnings. Education represents the accumulation of human capital, which increases the value of labor both on and off the farm. Education has a clear impact on access to nonfarm employment and acts as an exit path from low productivity economic activity to a higher one. The aforementioned empirical studies reviewed in this paper provide evidence that nonfarm employment is positively associated with higher education levels and better access to infrastructure. Without good roads, communications networks, and electricity, those in self-employment suffer higher transaction costs. Such cost also reflects why some households may achieve higher earnings and some others do not.
Table 6. Determinants of household head earnings and hours worked
6. Conclusion
Employment is undeniably a major challenge in Nigeria and therefore an issue of public policy. Poverty on the other hand is enormous and Nigeria is in the top position as the country with the most people in extreme poverty in the world (World Poverty Clock). To understand how rural employment fits into the overall livelihood strategies of households we used the Nigerian General Household Survey Panel (GHS-Panel) data. We find agriculture as the predominant employment in terms of the number of households employed in rural Nigeria. By aggregating the various employments in our data set into wage employment, farm self-employment, and non-farm self-employment, we find farm self-employment as the dominant employment type, followed by non-farm self-employment and wage employment. As noted in the background section, a key characteristic of the rural economy is the central role played by agriculture. In our regression analysis, we focused on two main questions: what drives employment in rural Nigeria (extensive margin)? Second, what drives employment at the intensive margin namely, earnings and hours worked by the household head? Our results suggest important factors such as years of schooling, level of infrastructure, social capital and income diversification, labor wage as drivers of increased rural employment and earnings. The econometric analysis, therefore suggests rural programmes that promote rural infrastructural, human capital development, networking, higher income and the diversity of income. It is an imperative policy goal to create an enabling environment for productivity and employment-intensive growth across all sectors of the economy particularly in agriculture. It is similarly important to have a holistic view on employment and earnings, that is being able to participate in more than one jobs, or being able to move to higher paying jobs. This paper is however limited in that the aggregate distinction of employment types namely, farm self-employment, wage employment, and non-farm employment conceal a range of economic activities in the rural sector. Future research should explicitly consider employment types to capture rural heterogeneous employment.
Disclosure statement
No potential conflict of interest was reported by the author(s).
Additional information
Funding
Notes on contributors
John Chiwuzulum Odozi
Abigail Gbemisola Adeyonu holds a Ph.D. in Agricultural Economics and a Lecturer I in the Department of Agricultural Economics and Extension, Landmark University, Nigeria where she teaches courses in economics and research methodology at undergraduate and graduate levels. She has researched and published on various issues relating to livestock economics, development economics which focus on rural areas.
John Chiwuzulum Odozi holds a Ph.D. in agricultural economics and is a Senior Lecturer in the Department of Agricultural Economics and Extension, Ajayi Crowther University, Nigeria. He is also a Senior Research Fellow at the French Institute for Research in Africa, University of Ibadan, Nigeria. His research focuses on issues about the economics of agriculture with a special focus on rural income growth and food security and how climate and non-climate shocks affect the rural population.
Notes
1. Among many programs, Nigeria’s government launched the Agriculture Transformation initiative and the Growth Enhancement Support program (GES). The government also initiated the Commercial Agricultural Credit Scheme (CACS) and the Nigerian Incentive-Based Risk Sharing for Agricultural Lending (NIRSAL).
2. Though large enterprises exist, they are few and employ labor on long-term contracts ranging from a season to many years. Small enterprises/farms also engage hired labor on a day−to−day basis or for a particular task.
3. Employment refers to all persons of working age who worked for pay, profit, or family gain during a specified reference period. It also includes all persons who had a job or enterprise but were absent from that job or enterprise during that period temporarily (e.g., persons who during the reference period were sick, on vacation, on maternity leave, on strike, or were temporarily laid off) (ILO, Citation2013a, Citation2013b).
References
- Ackah, C. (2013). Nonfarm employment and incomes in rural Ghana. Journal of International Development, 25(3), 325–16. https://doi.org/10.1002/jid.1846
- Ajakaiye, O., Jerome, A. T., Jerome, D. X., & Alaba, O. A. (2016).Understanding the relationship between growth and employment in Nigeria. understanding the African lions- growth traps and opportunities in six dominant African economies. World Institute for Development Economic Research (WIDER) Working Paper series 124/2015.
- Aminu, A. (2010) Determinants of participation and earnings in wage employment in Nigeria. 5th IZA/World Bank Conference: Employment and Development Cape Town, South Africa May 03-May4, 2010
- Awoyemi, T. T. (2011). Rural non-farm incomes and poverty reduction in Nigeria AERC Research Paper 224 African Economic Research Consortium, Nairobi
- Broeck, V., & Kilic, T. (2018) Dynamics of off-farm employment in Sub-Saharan Africa: A gender perspective. World Bank Group Policy Research Working Paper. 8540
- Carlos, B., Tilman, B., & Nina, W. (2011) Self-employment and conflict in Colombia. Working Paper. Discussion papers // German Institute for Economic Research, No. 1098
- Chang, Y., Huang, B., & Chen, Y. (2012). Labor supply, income, and welfare of the farm household. Labour Economics, 19(3), 427–437. https://doi.org/10.1016/j.labeco.2012.03.004
- Giannetti, M., & Andrei, S. (2004). On the determinants of entrepreneurial activity: Social norms. Economic Environment, and Individual Characteristics Swedish Economic Policy Review, 11(2), 269–313. https://doi.org/10.2139/ssrn.554511
- Himanshu, L. P., Murgai, R., & Stern, N. (2013). Nonfarm diversification, poverty, economic mobility, and income inequality: A case study in village India. Agricultural Economics, 44(4–5), 461–473. https://doi.org/10.1111/agec.12029
- Hung, M. N., Nguyen, T. A., & Read, R. (2019). Investigating the determinants of household welfare in the Central Highland, Vietnam. Cogent Economics & Finance, 7(1), 1684179. https://doi.org/10.1080/23322039.2019.1684179
- ILO. (2013a). Decent work indicators: Guidelines for producers and users of statistical and legal framework indicators. International Labour Organization.
- ILO. (2013b). National practices in the measurement of the economically active population, employment, unemployment, and time‐related underemployment. International Labour Organization.
- Imai, K. S., Gaiha, R., & Ganesh, T. (2015). Does non-farm sector employment reduce rural poverty and vulnerability? Evidence from Vietnam and India. Journal of Asian Economics, 36, 47–61. https://doi.org/10.1016/j.asieco.2015.01.001
- Laszlo, S. (2005). Self-employment earnings and returns to education in rural Peru. Journal of Development Studies, 41(7), 1247–1287. Taylor & Francis. https://doi.org/10.1080/00220380500170915
- National Bureau of Statistics (NBS)(2010). National manpower stock and employment generation survey: Household and micro enterprise (informal sector)
- Odozi, J. C., Awoyemi, T. T., & Ehirim, C. (2015). The contributions of economic growth and redistribution to poverty reduction in Nigeria. International Journal of Agricultural Development, 18(1), 2052–2063. http://ijard.com/journalarticles/vol18(1)2015/8._john_Odozi_2014_2015_05_29_16_37_21_678.pdf
- Odozi, J. C., Awoyemi, T. T., & Omonona, B. T. (2010). Household poverty and inequality: The implication of migrants’ remittances in Nigeria. Journal of Economic Policy Reform, 13(2), 191–199. https://doi.org/10.1080/17487871003700788
- Odozi, J. C., Ikhimalo, O. P., Awaebe, J., & Agbugui, M. (2018, October 10). Employment in the Nigerian agricultural labour market: Barriers and forecast. SocArXiv Papers. https://doi.org/10.31235/osf.io/s9hgv.
- Odozi, J. C., & Oyelere, U. R. (2019). Conflict exposure and economic welfare in Nigeria (No. 334). Global Labour Organization Discussion Paper series No.334.
- Odozi, J. C., & Uwaifo Oyelere, R. (2021). Does violent conflict affect labor supply of farm households? The Nigerian experience. Agricultural and Resource Economics Review, 1–35. https://doi.org/10.1017/age.2021.14
- Okonjo-Iweala, N., & Osafo-Kwaako, P. (2007). Nigeria’s economic re- forms progress and challenges. Working paper 6. Brookings Global Economy and Development. The Brookings Institution
- Olaniyan, O., & Abiodun, S. (2005) Human capital, capabilities, and poverty in rural Nigeria Research Report submitted to the African Economic Research Consortium (AERC), for the Second Phase Collaborative Poverty Research Project. African Economic Research Consortium.
- Omonona, B. T. (2009) Quantitative analysis of rural poverty in Nigeria. Nigeria Strategy Support Program (NSSP)Background Paper No. NSSP 009. IFPRI
- Shittu, A. M. (2014). Off-farm labor supply and production efficiency of farm households in rural Southwest NigeriaAgricultural and Food Economics 2(8), 1–21. http://doi.org/10.1186/s40100-014-0008-z
- The Sustainable Development Goals. (2019). Report https://unstats.un.org/sdgs/report/2019/The-Sustainable-Development-Goals-Report-2019.pdf