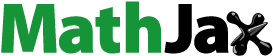
Abstract
The study examines the impact of regulatory & institutional quality (IQ) on FDI inflows, focusing on select factors to explain evolving FDI patterns in India over 2006–2019. India, one of the top 5 FDI attracting nations in the Asian region, is taking various measures to improve IQ and encourages FDI. The reforms facilitating the Ease of starting business and reduction in EPU significantly and positively impacts FDI; however, the measures easing trade across border and resolving insolvency has a positive but insignificant impact of FDI. In addition, deteriorating Labor Freedom significantly inhibits FDI. The results demonstrate that improvement in IQ positively influences FDI; however, the IQ impact is insignificant in some cases due to the weak institutional structure. The study suggests that IQ factors tend to be pivotal in attracting FDI inflows, but India is yet to arrive.
Public Interest Statement
The study examines the impact of India’s institutional quality (IQ) on FDI inflows, focusing on select factors over 2006–2019. India, one of the top 5 FDI attracting nations in the Asian region, takes various measures to improve IQ and attract more FDI. Our finding demonstrates that reforms facilitating the Ease of starting a business and reduction in EPU significantly and positively impacts FDI; however, the measures easing trade across the border and resolving insolvency has a positive but insignificant impact on FDI. Thus improvement in IQ positively influences FDI; however, the IQ impact is insignificant in some cases due to the weak institutional structure. The findings have implications for Indian policymakers to enhance good governance via adequate institutional reforms and strengthen the gaps in existing policies to attract a substantial portion of FDI flowing in the Asian region.
1. Introduction
Over the years, researchers have shown keen interest in exploring key country-specific factors that play significant roles in attracting inward FDI. Traditionally, studies emphasized macroeconomic determinants such as market size, exchange rates, inflation, international trade, and GDP as the major factors responsible for country-specific variation in FDI inflow (Caves, Citation1971; Chakrabarti, Citation2001; Dunning, Citation1980; Liu et al., Citation2001). However, since the late 90s, North’s (Citation1990) notable contribution underlining the importance of institutions and their interplay in the economy has served as a foundation to examine the link between institutions and FDI inflow (Aziz, Citation2018; Blonigen & Piger, Citation2014; Globerman & Shapiro, Citation2003; Loree & Guisinger, Citation1995). Researchers acknowledge that good functioning institutions protecting the interests of market entities significantly attract foreign investments (Masharu & Nasir, Citation2018 which is a catalyst to the economic development process (Acemoglu et al., Citation2002; Williamson & Kerekes, Citation2011).
The FDI literature ruminates numerous aspects while examining institutions-FDI links. Several studies have considered factors such as corruption, bureaucracy (Bénassy‐Quéré et al., Citation2007; Brada et al., Citation2019), minority stakeholder’s protection (Appiah-Kubi et al., Citation2020; Aziz, Citation2018; Serfraz, Citation2018), political instability (Globerman & Shapiro, Citation2002), international trade regulations (Corcoran & Gillanders, Citation2015), contract enforcement (Contractor et al., Citation2020) and economic freedom (Xu, Citation2019) to examine the country-specific institutional and regulatory climate influencing the FDI inflows. Infrastructural limitations restraining cross-border trade and the uncertainty related to monetary, fiscal, trade, and other macroeconomic policies (Q. Nguyen et al., Citation2018) are also notable factors influencing firms’ investment decisions in a host country (Lanz & Miroudot, Citation2011). Poor institutions are like the imposed tax, which increases investment costs, thus impeding foreign investment (Buchanan et al., Citation2012).
The world FDI inflows to developing Asian economies have steadily increased from 21% to 31% over 2006–2019, with significant differences across nations. Over the period of study, India’s share in the developing Asian FDI has marginally increased from 6.9% to 10.7%, whereas in the world FDI, from 1.4 % to 3.3%. India has consistently been among the top 5 nations to receive maximum FDI (2006–2019), but the overall share in total FDI has been more pronounced for China (28%), Hong Kong (20%) and Singapore (13%) vis-a-vis India (8%). Over the last two decades, economies worldwide have introduced numerous policy measures to liberalize, promote and facilitate foreign investment. During this phase, the share of total national policies liberalizing and promoting investment (83%) far outnumbered restrictive policies (17%), imposing limitations for FDI (UNCTAD, Investment Policy Monitor database). Notwithstanding the widespread deregulation and liberalization measures, institutional and regulatory barriers widely persist across nations. Developing economies take the lead in establishing institutional frameworks promoting free-market mechanisms, privatization and foreign investments (WIR (World Investment Report), Citation2019).
Numerous researches in FDI focuses on region-specific institutional quality (IQ) studies, for instance, OECD nations (Kim & Choi, Citation2020), emerging economies (Abraha & Hyder, Citation2021; Bhasin and Garg (Citation2020), developing and developed regions (Jude & Levieuge, Citation2017; Peres et al., Citation2018). Recent studies have also examined IQ concerning developing Asian regions like SAARC (Adeel-Farooq et al., Citation2020), South Asian economies (Layla et al., Citation2020) and individual nations, for instance, Vietnam (Bổn, Citation2020) and Iraq (Asaad & Marane, Citation2020) to attract FDI, as the US-China trade war and Covid-19 pandemic has forced foreign investors to relocate out of China, preferably in the labor-intensive developing Asian nations (Stangarone, Citation2020; Sun, Citation2019). However, there is little attention in the research on the institutional quality-FDI link in India. The institutional environment of developing countries is predominantly characterized by evolving institutions that are more receptive to change (Fon et al., Citation2021). Over the period, the Indian government have employed various institutional measures to seize a larger share of FDI directed towards the developing Asian economies. Hence the study attempts to address a fundamental question: “To what extent are FDI inflows in India motivated by the changes in institutional and regulatory quality influencing the business environment?”
The empirical contribution lies in testing the theoretical framework using a sample of top 20 investing nations contributing 93.6% of the total FDI inflow in India over the period 2006−2019. Besides considering the state-of-the-art FDI policy frameworks, MNCs looking to invest are increasingly paying attention to the host countries’ macroeconomic stability and institutional predictability. Numerous regional and world level studies have investigated the Institution-FDI association, but very few concerning India. The present study fills in the gap and contributes to the existing literature by examining a range of institutional factors where the Indian government has made persistent efforts to drive the business reforms and improve the IQ: starting a business, labor freedom, resolving insolvency, trading across bordersFootnote1 and EPU.Footnote2 The decision to focus our empirical examination on India is based on two reasons. First, considering the fact that IQ is crucial to attracting foreign investors, the Indian government has introduced considerable reforms to strengthen the same by improving select institutional and regulatory factors over the period. Second, due to the ongoing US-China trade war and the Covid-19 pandemic, several firms are contemplating re-location out of China to the other neighboring Asian nations.Footnote3 It is thus imperative to investigate how influential and successful these reforms have been in attracting the FDI by improving the institutional quality.
The institutional factors in the study comprise variables that are readily observable through written documents or rules and are determined and executed through formal positions, such as government authority. The study employs panel regression under both static (fixed and random effects) and dynamic conditions. Dynamic modelling employs the GMM estimation model of Arellano and Bover (Citation1995) and Blundell and Bond (Citation1998), which adequately deals with concerns related to endogeneity, omission of relevant variables, measurement error and simultaneity. The findings have implications for Indian policymakers to enhance good governance via adequate institutional reforms and strengthen the gaps in existing policies to attract a substantial portion of FDI flowing in the Asian region. Institutional reforms are vital for developing nations with constrained fiscal space and dependence on foreign investment to advance growth prospects (IMF, Citation2014). Hence, the study is equally relevant for the policymakers of other nations in drawing inferences about the extent to which institutional reforms with adequate changes enhance the business environment and promote investment.
The paper is structured as follows: Section 2 reviews the literature on the determinants of FDI. The methodology and data are presented in Section 3, while Section 4 reports and discusses the empirical results, and section 5 concludes.
2. Literature review
A large body of empirical literature in international business has examined the influence of institutional factors in determining FDI attractiveness. North (Citation1990) defined institutions as humanly invented constraints that structure political, economic and social interaction or simply as “rules of a game”. Contextually, the institutions within the economy aim to reduce uncertainty by establishing a stable framework bounded by rules for human interactions. North’s (Citation1990) institutional framework consists of formal rules (policies, reforms, laws), informal constraints (customs, norms, self-imposed code of conduct) and enforcement mechanisms. The formal rules represent the overall business climate for firms to operate and gain legitimacy (Meyer et al., Citation2011; Ostrom, Citation2009). Studies suggest that institutional and regulatory environment quality reduces the cost of doing business and increases firms profitability, thereby influencing foreign investments (Corcoran & Gillanders, Citation2015; Jandhyala, Citation2015; Sabir et al., Citation2019; Trevino et al., Citation2008). With institutional reforms becoming a tool for growing international competitiveness, governments worldwide have been reforming their domestic institutional (legal, political, economic, and cultural) structures to attract foreign investors (Contractor et al., Citation2020; Hitt, Citation2016). An unreliable institutional environment begets uncertainty and paints an unpredictable and insecure environment for foreign investors (Buthe & Milner, Citation2008; Jensen, Citation2003). Caggiano et al. (Citation2017) suggest that during high EPU, investors are more likely to opt for a wait-and-see attitude by postponing their investments, adversely impacting production in the host nation. Hence, the governments rendering a stable political environment with reliable and predictable market-based institutions favorably attract more FDI in their regions (Q. Nguyen et al., Citation2018; Sethi et al., Citation2003).
Empirical studies investigating the influence of a country’s regulations and norms on FDI are increasing but are geographically scattered, tackling broadly the regions and collective markets and few concerning individual nations. This study investigates the impact of India’s regulations and business climate in attracting worldwide FDI. Kurul and Yalta (Citation2017) assert that some institutional factors matter more than others in drawing more FDI inflows. Their empirical findings based on 113 developing countries over 2002–2012 suggest that institutional measures concerning control of corruption, government effectiveness, and voice and accountability positively influence FDI flow.
Tun et al. (Citation2012) affirm that nations with better institutional quality draw more investment due to reduced business and uncertainty costs. The results reveal that institutional quality, represented by bureaucratic quality, law and contract enforcement efficiency and reduction in corruption and expropriation risk are crucial factors influencing FDI inflows in 77 developing countries over 1981–2005. Corcoran and Gillanders (Citation2015) use the World Bank’s measures of institutional quality- ease of doing business (EODB) determinants to capture the business environment in Sub Saharan Africa, OECD and the rest of the world over 2004–2009. The results suggest that in the world’s most deprived Region-Sub-Saharan Africa (SSA) and the top income group-OECD, the quality of the business environment is insignificant. However, nations may reap benefits by improving their ease of trading across borders in the rest of the world. However, a similar study conducted by Anggraini and Inaba (Citation2020), using the world’s bank EoBD score and its determinants, investigating the institutional quality of 166 countries over 2009–18, suggest that the overall EoDB score is significant across all countries (high-income, middle-income, low-income, and SSA countries), except for OECD nations. The other most significant EoDB determinants include starting a business (high-income and OECD countries), paying taxes (middle-income countries), enforcing contracts (SSA countries), and getting credit (low-income countries). Sarpong et al. (Citation2020) suggest a 0.78 percentage point increase in FDI vis-à-vis one percentage point improvement in the ease of starting a business in SSA.
Aziz and Mishra (Citation2016) emphasize building a more efficient institutional environment in Arab nations to attract more foreign investments. The study points that government instability generating uncertainty about the nation’s economic and political principles and widespread corruption impacting investment returns has significantly affected FDI in Arab economies. Kofarbai and Bambale (Citation2016) highlight impaired electricity and inefficient tax administration as the major institutional bottlenecks hindering FDI inflow in Nigeria.
The FDI literature significantly highlights the importance of institutional quality in attracting foreign investments in developed and developing nations. As evident, due to the paucity of studies investigating developing Asian economies IQ, particularly India’s, the present study attempts to investigate the impact of Institutional factors driving inward FDI, thereby suggesting the way forward to policymakers to strengthen India’s position in attracting the much needed FDI boosting the country’s economic growth. Given the current global economic scenario, India must compete with its regional competitors in attracting investments moving out of China, mainly to boost the “Make in India” initiative.
3. Methodology
3.1. Data and variables
The analysis considers a sample of the top 20 investing nations contributing 93.6% of the total FDI inflow in India over the period 2006−2019. Appendix () lists the nations along with their investment contributions. With 280 observations across 20 cross-sections, the study analyzes the association between institutional factors and FDI, given a set of control variables that include other business environment aspects. The study reports trade openness and Population Growth in % terms and all other variables in the natural log form. below provides information about the data used in the study.
Table 1. Summary description of the variables
3.1.1. Foreign Direct Investment (FDI)
The study employs the natural log value of FDI inflows instead of FDI stock as the dependent variable to ensure consistency in measuring the dependent variable. Measurement of FDI stock flowing from different investing countries tends to be heterogeneous with many zeroes and negative values (Dauti, Citation2015; Globerman & Shapiro, Citation2002). Scarce data related to India’s FDI stock from its investing partners also makes FDI flows a more apposite variable (Bhasin & Manocha, Citation2016; Dorakh, Citation2020).
3.1.2. Institutional quality variables
The institutional quality relates to well-functioning institutions in the host nation that reduce transaction costs and enhance profitability for the investors and, therefore, a significant determinant for FDI inflows (Sabir et al., Citation2019). The institutional quality indicators used in the study are obtained from multiple sources: Ease of doing business from the World Bank, Economic Freedom from the Heritage Foundation, and Economic Policy Uncertainty www.polityuncertainty.com provided by Baker et al. (Citation2016).
3.1.2.1. The World Development Indicators
The World Bank’s ease of doing business (EODB) database captures 12 development indicators that best measure the functioning of the nation’s regulatory and institutional quality (such as courts, credit bureau), shaping the business environment in which a representative firm operates (Lehne et al., Citation2014; World Bank, Citation2015). Regulatory quality relates to governments ability to formulate and implement sound policies to promote private sector development and uniform rules of economic engagement. The indicators measure the complexity and cost of regulatory processes and the strength of legal institutions by enabling a comparison of absolute change in regulatory business environments across economies over time. Each Doing Business indicator score ranges between 0 and 100, where 0 represents the lowest and 100 the best performance. The score bench-mark economies with respect concerning regulatory best practice, showing the proximity to the best regulatory performance on each Doing Business indicator.
3.1.2.2. Ease of starting a business(EoSB)
The EoSB score is a proxy for entry-focused regulations imposed by the government concerning the multinational enterprises (MNEs) entry into a new market or starting a business (Djankov et al., Citation2002). The score reflects the ease of starting a new business based on the time and cost involved in complying with the regulatory procedures, such as the number of formal procedures, the actual time required in business days to complete such procedures and the minimum paid-in capital needed to start a firm. The EoSB scores for India have significantly improved over time, from 26.8 (2006) to 81.2 (2019). A higher score indicates lower entry barriers; thus, we expect the variable to correlate with FDI inflow positively.
3.1.2.3. Trading across borders (TAB)
The trading across border score, a proxy for the country’s border trade facilitation, measures the time and cost associated with the logistical process of exporting and importing goods. The score considers the documentary and border compliance involved in the overall process. The number of forms, procedures, delays, and tariffs concerning cross border trade is lower in Sri Lanka and Thailand than in India (Prasad et al., Citation2014). Trevisani (Citation2019) highlights Brazilian efforts to overcome regulatory impediments at ports and airports to encourage trade-related FDI. However, over time, the scores for India has improved from 53.2(2006) to 82.2(2019). Ease of trading across borders favors FDI decision by enabling the geographical spread of supply chains ranging from R&D to supply of components, assembly or supporting services (Contractor et al., Citation2020; Jovanovic & Jovanovic, Citation2018). A higher score indicates a lower logistical cost of trading goods; we expect the variable to correlate with FDI inflow positively.
3.1.2.4. Resolving insolvency (RI)
Resolving insolvency score is a proxy for exit-focused regulations or laws imposed by the government to facilitate or delay firms’ insolvency (Djankov et al., Citation2008). The score measures the efficiency of insolvency or bankruptcy laws and the length of administrative procedures required by a firm to undergo an insolvency process while exiting the host market. Studies view the presence of entry and exit regulations as mobility barriers affecting investment strategies (Contractor et al., Citation2020; Jeong et al., Citation2018). The higher score reflects the ease of exiting the host country’s market, and the score for India has improved from 32.3 (2006) to 40.8 (2019). We expect the variable to be positively associated with FDI inflows.
3.1.2.5. The Heritage Foundation economic freedom indicators
The Economic Freedom indicators by Heritage Foundation measures the degree to which the policies and institutions of countries support economic freedom. Economic freedom is a good proxy for measuring a nation’s institutional quality (Gwartney et al., Citation2014; Pääkkönen, Citation2010). An effective legal system, fewer regulations and free competition in an economically free society are fundamental factors attracting more volume of FDI (Ajide & Eregha, Citation2015; Iamsiraroj, Citation2016; Sayari et al., Citation2018). The economic freedom score ranges between 0 and 100, with 0 being the lowest and 100 the highest level of economic freedom.
3.1.2.6. Labor Freedom(LF)
Labor Freedom is considered a proxy for labor market regulations imposed by the governments of the respective nations. A flexible labor market influences FDI inflow through the cost and productivity channels, reflecting the quality of labor market institutions (Hodgson & Stoelhorst, Citation2014; Mina, Citation2020). Labor Freedom score measures the flexibility of the labor market by weighing businesses ability to freely contract or dismiss redundant workers, a vital determinant of business productivity and overall economic growth. The government’s rigid labor regulations prevent employers and employees from negotiating changes in the terms and conditions of work. A rise in the labor market regulatory burden is reflected in higher unit labor costs, which impedes FDI inflow (Bayraktar-Sağlam & Sayek Böke, Citation2017). The evidence from China, Brazil, Portugal, and middle-income nations suggest that stringent labor market regulations adversely affect the operational flexibility of MNCs and hence the FDI inflows (Almeida & Poole, Citation2017; Rong et al., Citation2020; Walsh & Yu, Citation2010). Over the years, India’s LF score dwindles from 62.30 (2006) to 41.80 (2019). The study expects low LF to influence FDI inflow negatively.
3.1.2.7. Economic Policy Uncertainty (EPU)
EPU is widely considered a proxy for a country’s macroeconomic institutions, as it captures the uncertainty related to government policies and regulatory frameworks such as monetary, fiscal, trade, and investment policies (Jiang et al., Citation2019; Q. Nguyen et al., Citation2018; Syed & Bouri, Citation2021). A stable political environment with reliable and predictable government policies and market-based institutions, rightly called “good governance”, impacts firms operational costs and profitability in a host nation (Fan et al., Citation2009; Root & Ahmed, Citation1978; Sethi et al., Citation2003). EPU index corresponds well with events associated with periods of acute policy uncertainty such as spikes occurring around elections, wars, debt ceiling deliberations, variations in government policies and regulations, implementation of new reforms, particularly with reservations from the opposition party. Literature suggests that policy uncertainty in a host nation plays a vital role in attracting investments (Al-Thaqeb & Algharabali, Citation2019; Demir & Ersan, Citation2017). The high EPU warns foreign investors, sensitive to changes in future economic policies, to wait before making costly-to-reverse investment decisions in the host country (Belke et al., Citation2018; Canh et al., Citation2020; Zhao et al., Citation2020). India’s significantly high EPU index (185.5) corresponds to 2011–12, known as the years of policy paralysis, but henceforth, the EPU has significantly reduced (73.1 in 2019). Indian policymakers aim to reduce ambiguity and arbitrariness in the policy implementation, thereby enhancing policy certainty to foster the country’s wholesome investment climate. The study expects a fall in EPU over time to positively influence FDI inflow in India.
3.1.3. Control variables
3.1.3.1. Sum of the Gross Domestic Product (GDPsum)
The sum of the Gross Domestic Product of the host and source countries intends to capture the horizontal market size (Bayraktar, Citation2013). The measure of market size is the natural log value of GDP in US dollars. If the source country views the host as an extended market for its products and services, the variable is expected to affect the dependent variable, FDI inflow, positively. Numerous studies have used GDPsum as a macroeconomic variable in explaining inward FDI (Bae & Keum, Citation2013; Regelink & Elhorst, Citation2015; Yokota & Tomohara, Citation2009).
3.1.3.2. Absolute difference in the GDP per capita (GDPpc)
The absolute difference in the GDP per capita between home and the host nation is considered a proxy for measuring the skill differential and capturing the vertical FDI drive (Bhasin & Manocha, Citation2016; Navaretti & Venables, Citation2020). Literature suggests that low production costs and qualified labor force encourage vertical FDI (Lankes & Venables, Citation1997), implying that relatively cheap skilled labor found in skilled labor-abundant countries attracts vertical FDI. The variable’s coefficient from the estimated model would either confirm the Linder hypothesis or the Heckscher-Ohlin (H-O) perspective. We expect a positive coefficient, confirming the H-O theory asserting that countries take advantage of their relative factor endowments and vice-versa. In context to the endowment theory, vertical FDI is typically measured as aggregate exports from the host country to the other nations (Braconier et al., Citation2005). Considering the influence of human capital (skilled labor) on improving productivity and increasing capital inflow, India, a significant service exporter with a large skilled labor force, has tremendous potential to attract a sizable volume of FDI. The study expects the variable to be positively related to FDI inflow, contributing immensely to the service sector.
3.1.3.3. Trade Openness (TO)
The literature on trade liberalization suggests that trade openness, symbolizing nations liberal economic orientation drives FDI inflows (Akbar & Akbar, Citation2015; Lin & Ye, Citation2018). The ratio of exports plus imports to GDP is used as a proxy for trade openness. Besides encouraging resource-seeking inward FDI, an open economy also encourages efficiency-seeking FDI to the service sector, such as business services and communications (Mina, Citation2020).
3.1.3.4. Population growth (PopGr)
Population growth is considered a proxy for the availability of human capital in the host country. Coale and Hoover (Citation2015) highlight three significant effects of population growth. The study suggests that the increasing “children to working adults” ratio and a decreasing “capital to labor” ratio divert household’s income from saving towards consumption; moreover, the growing demand for government expenditure towards health and education veers limited resources from relatively more productive enterprises. The host country’s human capital plays a crucial role in determining FDI inflows (Borensztein et al., Citation1998); however, the excessive population growth burdens the available infrastructure. The rapid population growth raises alarming economic situations such as high unemployment, lack of infrastructure and restricting the government from providing basic facilities such as health and education to the fast-growing population (Musambachime, Citation1990). It may further result in low-quality human capital, dissuading investors from investing in the country. The study assumes that the variable will have a positive coefficient if India successfully attracts FDI in labor-intensive sectors. Though, beyond a certain limit, human capital expansion negatively affects FDI (Hayat, Citation2018).
3.2. Econometric estimation
The study estimates balanced data from the panel of 20 countries to investigate the impact of select institutional factors on FDI inflow in India by employing Fixed effect (FE) and Random effect (RE) for static estimation and system Generalized Methods of Moments (GMM) for dynamic estimation over the period 2006–2019.
The OLS estimation is prone to heteroscedasticity in pooled time-series and cross-sectional settings as it considers common intercept (βit = β), ignoring the individual cross-sectional effects (Al Nasser, Citation2007). The technique also violates certain basic assumptions, such as orthogonality. The normalcy of the data tested by the Jarque-Bera test suggests whether or not the data is normally distributed. Wooldridge (Citation2010) suggests multicollinearity between variables if the correlation coefficient exceeds 0.70 between two variables; thus, the VIF test is recommended to identify the same. The stationarity of variables at level tested through Levin, Lin, and Chu (LLC) unit root test suggest the applicability of FE/RE models for estimation. The FE and RE modelling eases the limitations of pooled OLS technique to a certain extent. The preponderance of FE model is based on its ability to control unobserved time-invariant heterogeneity across units and provide robust results by omitting variable biases (Hausman & Taylor, Citation1981). FE model examines the difference between country-specific intercepts and is appropriate to analyze a specific set of entities (Baltagi, Citation2008). It considers the within-group variation (cross-section) by assuming constancy of (λi) over time and subsumes the unobserved heterogeneity under the constant term (α + λi).
On the other hand, RE model is suitable to draw inferences about entities randomly drawn from a large sample. RE considers between-group variation (time) and adds the unobserved heterogeneity to the error term (ԑit = λi + uit). Breusch-Pegan Lagrange Multiplier test recommends the applicability of the RE model over pooled OLS. The violation of the homoscedasticity assumption in the variance of the error term suggests heteroscedasticity. The robust standard error technique is employed to estimate unbiased standard errors and make the model robust due to heteroscedasticity in the FE and RE models. The Hausman specification further specifies acceptance of RE and FE model. The null of the Hausman test proposes that the “difference in coefficients are not systematic”, and a significant chi-square (χ2) rejects the null and accepts FE model and vice versa. Further, the endogeneity in the model, due to the lagged FDI inflow coefficient significantly influencing the dependent FDI variable, as highlighted by the previous studies (Aziz & Mishra, Citation2016; Saini & Singhania, Citation2018), suggests applying a dynamic panel model. The inconsistent estimates and incorrect inferences in the presence of endogeneity bias lead to misleading and inappropriate results (Baltagi, Citation2008).
The dynamic panel estimators proposed by Arellano and Bond (Citation1991) find its acceptance in a wide range of economic applications. The present study estimates the dynamic relationship between FDI inflows and regressors, including a lagged value of a dependent variable. The coefficients of lagged dependent variable, allowing for dynamics in the process, is crucial for consistent estimation of parameters. However, lagged dependent variables lead to certain econometric issues, autocorrelation among regressors, and heterogeneity among the individuals. The unobservable time-invariant properties of cross-sections, captured by the error terms with the likelihood of correlation with regressors, causes estimation issues.
Arellano and Bond (Citation1991) proposed the GMM estimator, where lagged values of explanatory variables are used as instruments to tackle the endogeneity bias. The generalized method of moments (GMM) model is suitable in this study due to the small T, and large N. A small T can lead to asymptomatically inconsistent estimates from the FE model (Nerlove, Citation1967; Nickell, Citation1981). EquationEquations 1(1)
(1) and Equation2
(2)
(2) represent the fundamental static & dynamic nature of model estimating FDI inflow determinants:
Static Model
Dynamic Model
In the above specified model FDIit represent FDI of country i and time t with i = 1, …, N, and t = i, …, T,
is the first lag of the dependent variable FDI inflows, Xjit represents independent variable, Zlit represents institutional variable, eit is disturbance term with unobserved country-specific effects, υi and uit are idiosyncratic error where ʋi ~IIN (0; σʋ2) and ui (IIN (0; σu2) and
are the parameters to be estimated in the model.
Arellano-Bond estimation starts by transforming regressors, typically by differencing and using the GMM technique known as difference GMM (Hansen, Citation1982). The difference GMM estimator employs first-differences of dependent variable and regressors to transform the regression, remove fixed country-specific effects, making regressors time-invariant. However, the lagged levels of the regressors may sometimes be imperfect instruments for the first-differenced regressors, decreasing the efficiency. Blundell and Bond (Citation1998) argue that the lagged-level instruments in Arellano–Bond estimator are subject to weakness due to a robust autoregressive process or a high ratio of panel-level variance to idiosyncratic error variance. Hence to increase the model’s estimation efficiency, Blundell and Bond (Citation1998) proposed a system GMM estimator that uses moment conditions. System GMM is a blend of level and difference dynamic equations that improve on difference-GMM by supplementing the equation in the first differences with the equation in levels and correcting measurement errors in the other regressors (Blundell & Bond, Citation1998).
The FDI literature widely supports the use of system GMM to address possible endogeneity problems such as reverse causation, simultaneity biases and omitted variables issues (Abbas et al., Citation2021; Islam et al., Citation2020; Sabir et al., Citation2019; T. T. Nguyen et al., Citation2021). The study employs a two-step system GMM estimation procedure. In one-step estimation, the error terms are assumed to be independent and homoscedastic across the country and time. At the same time, two-step estimator uses residuals of the first step to estimate the variance-covariance matrix of residuals consistently, relaxing the assumption of homoscedasticity (Favarra, Citation2003). Windmeijer (Citation2005) suggest that two-step GMM with lower bias and corrected standard errors is better than one-step GMM in estimating coefficients.
The system GMM panel estimation is based on two basic assumptions: the absence of second-order autocorrelation or serial correlation in the error term and the validity of additional instruments to explain the model. Arellano-Bond autocorrelation tests, AR (1) and AR (2), are used to investigate first-order and second-order autocorrelation. The test for AR1 should be significant as it indicates the first-order autocorrelation in residual terms; however, there should not be the second-order autocorrelation AR (2). GMM uses multiple lags as instrument variables which make the system over-identified; thus Sargan-Hansen test of over-identification is used to validate the instruments in a statistical model.
4. Result & discussion
We analyze the data with standard procedures (pooled OLS, fixed and random effect models, and GMM). The first method employed is pooled OLS estimation. Jarque-Bera test indicates normalcy of the data. With a p-value of 0.426, the null of Jarque Bera stating “data is normally distributed” cannot be disproved. The static model is considered as data is non-poolable on the ground of heterogeneity. The FE and RE models are tested for the presence of cross-sectional dependency. The results of the Pesaran CD test clearly show the presence of cross-sectional dependency (p-value = 0.000); thus, sys-GMM, a more reliable technique than standard GMM in the presence of heterogeneous error cross-sectional dependence, is employed in the study. Descriptive statistics are reported in and . displays the mean, standard deviation and minimum and maximum values for each variable. The correlation matrix presented in does not suggest a strong correlation between explanatory variables; however, the variables indicating mild correlation: EOSB & PopGr (0.814), EOSB & RI (0.668) and EPU & PopGr (0.524), are treated in two separate models. Wooldridge (Citation2010) suggests the presence of multicollinearity between variables if the correlation coefficient exceeds 0.70 between two variables. The VIF (Variance Inflation Factor) estimates the influence of multicollinearity on the variance of coefficients in any estimated regression model. A VIF >10 indicates multicollinearity; however, studies largely suggest a more conservative threshold of 5 or even 2.5 for better estimation (Gareth et al., Citation2013; Kawiana et al., Citation2020). Hence, based on VIF statistics (mean VIF = 5.34) ( and ), the study considers correlated variables separately in two distinct models. The unit-root test examines the stationarity of variables as non-stationary data results in unreliable and spurious findings (Aziz & Mishra, Citation2016). The study employs Levin et al. (Citation2002) LLC unit root test for all the variables, disproving common unit root in the study (). Breusch-Pegan test recommends the applicability of the RE model over pooled OLS.
Table 2. Descriptive statistics
Table 3. Correlation matrix
Table 4. Panel regression results for Model 1
Table 5. Panel regression results for Model 2
Table 6. VIF results
Table 7. Unit root test
report the panel regression results of FE, RE (static) and GMM(dynamic) estimation for models 1 and 2, respectively, for 14 years (2006–2019), capturing both institutional and control variables influencing FDI inflow in India. Model 1 & Model 2 specifications are stated below:
Model 1
Static Eq:
Dynamic Eq:
Model 2
Static Eq:
Dynamic Eq:
The FE estimator is preferred over RE estimator when the study draws inferences based on a specific set of entities rather than a randomly drawn sample (Baltagi, Citation2008; Wooldridge, Citation2010). Hausman test reflects the incidence of a systematic difference in the coefficients of RE vs FE estimators (p-value = 0.020) for both the models, rejecting the null hypothesis in support for FE estimators to be appropriate for the study. However, the presence of autocorrelation (D-W test, p-value>0.05) and endogeneity (cov (x,ε)≠ 0) suggest the use of dynamic model. Hence, the study also employs system GMM estimator, capturing the dynamic nature of FDI by including lagged FDI as an explanatory variable. The System GMM technique adequately deals with issues concerning endogeneity, simultaneity, omission of relevant variables, measurement error and sample selectivity (Aziz & Mishra, Citation2016). The Sargan and Hansen tests rule out the over-identification problem, supporting the validity of instruments; furthermore, the Arellano-bond autocorrelation rules out second-order autocorrelation, supporting GMM estimation. Based on the Hausman test, the study considers GMM specifications appropriate for interpretation. Post-estimation tests, AR (1) is significant and AR (2) is insignificant, implying the presence of first and absence of second-order autocorrelation in data. Wald test statistics give significant values of χ2, rejecting the null hypothesis that estimated coefficients are jointly and significantly different from zero, meaning that the model has predictive power. Rejection of over-identifying restrictions using the Sargan-Hansen test suggests that all instruments are valid to explain the model. Windmeijer’s finite-sample correction is used for finer control over the instrument matrix. The lagged FDI inflow is used as an instrument in both the models. Past FDI literature suggests the demonstration effect of existing FDI as a crucial factor attracting more FDI (Aziz & Mishra, Citation2016; Mina, Citation2020). It connects well with the argument that MNEs are more inclined to invest in nations that already have a sizeable FDI. This implies that the MNEs success in host countries is a decisive factor attracting further investment by foreign firms. The study employs lagged FDI variable as an instrument in the study.
Model 1 () represents the select control variables: GDPsum, GDPpc, PopGr, TO and the institutional/regulatory variables: TAB and RI that can influence FDI inflow. The GMM estimation indicates a significant positive relationship between explanatory variables lagged FDI, GDPsum, TO, TAB and the FDI inflow, whereas RI fails to impact FDI inflows significantly. There is a significant negative relationship between FDI inflow and PopGr.
Model 2 ( and ) comprises GDP, GDPpc, TO as macroeconomic variables, whereas the LF, EPU and EoSB are the institutional or regulatory variables that can influence FDI inflow. The GMM estimation indicates a significant positive relationship between the following explanatory variables- lagged FDI, GDP, TO, EoSB, EPU and the FDI inflow, whereas LF negatively impacts FDI inflows.
The explanatory variable- lagged FDI inflows is positive and significant in the GMM specification across model 1 (β = 0.287, p-value = 0.000) and model 2 (β = 0.283, p-value = 0.000). Results indicate that the existing FDI is a crucial determinant to attract more FDI. Thus India holds a fair chance to attract more FDI contingent to the already amassed sizeable investment by the foreign investors. A similar finding is reported by Aziz (Citation2018).
The ease of starting a business relates to a good regulatory environment characterized by simpler and shorter procedures for starting businesses, capable of attracting more investments. Since 2015, India has initiated sustained business reforms easing the setting up of a business in the nation by fully integrating multiple application forms into a general incorporation form and abolishing fees for incorporation of Company in India. GMM results reports EoSB to be a significant variable positively influencing FDI inflows (β = 0.002, p-value = 0.089).
The EPU index shows that the uncertainty in India is declining over time, which has a positive and significant impact on FDI inflows (β = 0.424, p-value = 0.000). Theoretically, as the EPU index rises, it signifies higher policy uncertainty for the country in question, thus resulting in an inverse relation with FDI. However, for the purpose of our analysis, to satisfy the stationarity conditions, we have considered a change in EPU from period t-1 to period t for the sample period. As India’s policy uncertainty recorded by the index has consistently fallen at an increasing rate within the sample period, the data points carry a negative sign indicating a fall from period t-1 to period t. This, paired with the theoretical negative β, results in a positive coefficient in our equation. The observation is in line with the assumption that low domestic EPU positively influences FDI inflows. Since early 2015, there has been a continuous decline in domestic policy uncertainty, contrary to the rising global policy uncertainty, especially in the US, UK, and China. Indian policymakers have fostered a healthy investment climate by allowing consistency in actual policy with the forward guidance to reduce EPU. The results are similar to the study reported by Barrero et al. (Citation2017).
The variable resolving insolvency is positive but insignificant in GMM specification (β = 0.005, p-value = 0.610) specifications. India aims to facilitate more accessible insolvency settlement by adopting a new insolvency and bankruptcy code that promotes reorganization proceedings for corporate debtors and facilitates the debtor’s business during insolvency proceedings. However, the regulations not allowing dissenting creditors to receive the same liquidation amount as under reorganization still does not fully solve the problem.
Trading across border variable is positive but insignificant in GMM specification (β = 0.130, p-value = 0.768). Since 2008, the Government of India has been undertaking sustained business reforms to facilitate trading across the border by enabling post-clearance audits, integrating trade stakeholders in a single electronic platform, upgrading port infrastructures and enhancing the electronic submission of documents. However, more institutional efforts are required to influence FDI inflows significantly.
Rising deterioration in the Labor Freedom is found to have a significant negative impact on FDI inflows (β = −4.038, p-value = 0.019) in GMM specification. The study calculates the deterioration of LF score by subtracting the yearly LF score from 100 (100 represents most freedom). In labor-intensive sectors, the quality of labor market restrictions related to the ease of hiring and firing policies, wage determination flexibility, redundancy costs, and severance requirements significantly influence labor costs and incentivize FDI inflows. The Labor Freedom in India has significantly declined from 62.30 to 41.80 over the study period and consequently the FDI inflows, particularly in the labor-intensive manufacturing sector.
The sum of the Gross Domestic Product of the host and source countries intends to capture the horizontal market size. The variable is found to be positive and significant in both the GMM models specifications viz., Model 1 GMM (β = 0.695, p-value = 0.060), Model 2 GMM (β = 0.801, p-value = 0.000) specification. Results indicate that India has made proper utilization of the vast market size to attract more FDI inflows. This finding is consistent with Regelink and Elhorst (Citation2015).
The absolute difference in the GDP per capita between the home and the host nation helps measure the skill differential and captures the vertical FDI drive. As factor costs are assumed to be determined by factor endowments thus, skilled labor would be relatively cheap in skilled-labor abundant countries. This variable’s positive and significant coefficient in GMM model 2 specifications viz., (β = 0.567, p-value = 0.005) indicates that the human capital (skilled labor) in India can attract a high share of FDI inflows in the service sector. Mina (Citation2020) also reports similar results.
The population growth variable is negative and insignificant in GMM (β = −0.382, p-value = 0.073) specification. The rising population diverts the nation’s income towards ensuring capital for new workers rather than raising capital per worker, resulting in lower productivity among the labor force. However, India may significantly enhance the output per capita by increasing the capital per worker ratio to attract FDI, particularly in the manufacturing sector. The result is consistent with the findings of Bhasin and Manocha (Citation2016).
The trade openness variable is positive and significant in both in both the GMM model specifications viz., Model 1 (β = 0.631, p-value = 0.000), Model 2 (β = 0.432, p-value = 0.006) specifications. The liberal trade orientation of nations helps potential foreign investors understand the local conditions of their international trade partners. Therefore, countries that are more open to trade (fewer restrictions) with sizeable trade volume will likely attract more FDI. This result is in line with (Lin & Ye, Citation2018).
5. Conclusion & policy implications
Building on the North’s Institutional framework that the host country’s institutional quality affects profitability and institutionally strong nations can attract more foreign investors by offering high returns, the study empirically examines the impact of institutional quality on FDI inflows in India by focusing on select institutional and regulatory factors. In recent years, the Indian Government has been proactively involved in reducing regulatory complexity, strengthening legal institutions and streamlining administrative systems to create a conducive business environment for foreign investors. The results of this study have several implications for policymakers. Over the years, India has successfully improved institutional quality by offsetting high entry (EoSB) and exit barriers (RI), easing trade across borders, overcoming labor market rigidities, and reducing domestic policy uncertainty to facilitate business operations and decrease business costs. Most of the reforms have positive implications for foreign investors but have yet to show a significant impact on FDI inflows. India needs to facilitate business operations and protect foreign investors by further enhancing the institutional quality and significantly promoting free-market mechanisms (liberal labor laws) and an effective legal system (cross border insolvency framework) to attract more inward FDI. Labor cost, one of the significant components of a cost function, and the poor quality of a state’s legal system significantly deter Investment. The low-quality human capital also significantly dissuades investors from investing in the country. The advancement in human capital development with a stronger focus on quality education and health services is likewise an indispensable and essential condition to attract FDI in India. Indian policymakers should sequence their human development policy measures with the institutional ones, beginning with a focus on labor laws, trade facilitation services and legal frameworks ensuring transparency and legal certainty of business activities, consequently driving economic growth.
Our research has certain limitations. For instance, the theory of economic regulations indicates that regulatory reforms may also emerge from constituents’ demands. It implies that besides being the passive recipient of regulatory changes, MNCs may also actively model regulations. Future research may accordingly investigate the process of regulatory changes to identify MNCs’ role in regulatory reforms. Additionally, future research needs to identify the relevance of sector-specific regulatory and institutional factors to gain a holistic view of a country’s business environment.
Disclosure statement
No potential conflict of interest was reported by the author(s).
Additional information
Funding
Notes on contributors

Leena Ajit Kaushal
Leena Ajit Kaushal is currently working as Assistant Professor in the area of Economics at Management Development Institute, Gurgaon, India. Her teaching interests include Macroeconomic Environment ߪ Policy, Foreign Direct Investments and International Economics. Her research interest lies in the field of Sustainable Development, Foreign Direct Investment, Macroeconomic policy and Sharing Economy. She is Associate Fellow of the Higher Education Academy (HEA), UK and possesses over 15 years of experience across a range of verticals which include University teaching in multinational and cross-cultural teaching environment, Investment banking industry and research fellowship with UGC grant commission. She is credited with research publications in various refereed national and international journals. She has published books on Managerial Economics by Thompson Publishing, ‘Global Entrepreneurship and New Venture Creation in the Sharing Economy’ by IGI Global and FDI-The Indian Experience by BEP publishers. Her email address [email protected]
Notes
References
- Abbas, Q., Junqing, L., Ramzan, M., & Fatima, S. (2021). Role of governance in debt-growth relationship: Evidence from panel data estimations. Sustainability, 13(11), 5954. https://doi.org/10.3390/su13115954
- Abraha, D., & Hyder, A. S. (2021). “Theoretical background”, transformation of strategic alliances in emerging markets (Vol. I, pp. 101–112). Bingley: Emerald Publishing Limited.
- Acemoglu, D., Johnson, S., & Robinson, J. A. (2002). Reversal of fortune: Geography and institutions in the making of the modern world income distribution. The Quarterly Journal of Economics, 117(4), 1231–21. https://doi.org/10.1162/003355302320935025
- Adeel-Farooq, R. M., Khan, J., Nawaz, A., & Akram, K. (2020). Governance and FDI in selected SAARC economies. Pakistan Journal of Social Sciences (PJSS), 40(3), 1317–1327. http://pjss.bzu.edu.pk/website/journal/article/5f74953d9d345/page
- Ajide, K. B., & Eregha, P. B. (2015). Foreign direct investment, economic freedom and economic performance in sub-saharan Africa. Managing Global Transitions: International Research Journal, 13(1), 43–57. https://www.fm-kp.si/zalozba/ISSN/1581-6311/13_043-057.pdf
- Akbar, M., & Akbar, A. (2015). An empirical analysis of foreign direct investment in Pakistan. Studies in Business and Economics, 10(10), 5–15. https://doi.org/10.1515/sbe-2015-0001
- Al Nasser, O. M. (2007). The determinants of the US foreign direct investment: Does the region matter? Global Economic Review, 36(1), 37–51. https://doi.org/10.1080/12265080701217181
- Almeida, R. K., & Poole, J. P. (2017). Trade and labor reallocation with heterogeneous enforcement of labor regulations. Journal of Development Economics, 126(C), 154–166. https://doi.org/10.1016/j.jdeveco.2017.01.004
- Al-Thaqeb, S. A., & Algharabali, B. G. (2019). Economic policy uncertainty: A literature review. The Journal of Economic Asymmetries, 20(C). https://doi.org/10.1016/j.jeca.2019.e00133
- Anggraini, R. F., & Inaba, K. (2020). The impact of the ease of doing business on foreign direct investment. The Ritsumeikan Economic Review: The Bi-Monthly Journal of Ritsumeikan University,69(3), 393–421.
- Appiah-Kubi, S. N. K., Malec, K., Maitah, M., Kutin, S. B., Pánková, L., Phiri, J., & Zaganjori, O. (2020). The impact of corporate governance structures on foreign direct investment: A case study of West African countries. Sustainability, 12(9), 3715. https://doi.org/10.3390/su12093715
- Arellano, M., & Bond, S. (1991). Some tests of specification for panel data: Monte Carlo evidence and an application to employment equations. The Review of Economic Studies, 58(2), 277–297. https://doi.org/10.2307/2297968
- Arellano, M., & Bover, O. (1995). Another look at the instrumental variable estimation of error-components models. Journal of Econometrics, 68(1), 29–51. https://doi.org/10.1016/0304-4076(94)01642-D
- Asaad, Z. A., & Marane, B. M. (2020). The influence of human development, institutional quality and ISIS emergence on foreign direct investment in Iraq. Technium Social Sciences Journal,10(1), 318–332. https://techniumscience.com/index.php/socialsciences/article/download/1402/499
- Aziz, O. G. (2018). Institutional quality and FDI inflows in Arab economies. Finance Research Letters, 25(C), 111–123. https://doi.org/10.1016/j.frl.2017.10.026
- Aziz, O. G., & Mishra, A. V. (2016). Determinants of FDI inflows to Arab economies. The Journal of International Trade & Economic Development, 25(3), 325–356. https://doi.org/10.1080/09638199.2015.1057610
- Bae, C., & Keum, H. (2013). The impact of FTAs on FDI in Korea. KIEP Research Paper. World Economy Update, 13–19. https://papers.ssrn.com/sol3/papers.cfm?abstract_id=2320947
- Baker, S. R., Bloom, N., & Davis, S. J. (2016). Measuring economic policy uncertainty. The Quarterly Journal of Economics, 131(4), 1593–1636. https://doi.org/10.1093/qje/qjw024
- Baltagi, B. (2008). Econometric analysis of panel data (1). John Wiley & Sons.
- Barrero, J. M., Bloom, N., & Wright, I. (2017). Short and long run uncertainty (No. w23676). National Bureau of Economic Research.
- Bayraktar, N. (2013). Foreign direct investment and investment climate. Procedia Economics and Finance, 5, 83–92. https://doi.org/10.1016/S2212-5671(13)00013-0
- Bayraktar-Sağlam, B., & Sayek Böke, S. (2017). Labor costs and foreign direct investment: A panel VAR approach. Economies, 5(4), 36. https://doi.org/10.3390/economies5040036
- Belke, A., Dubova, I., & Osowski, T. (2018). Policy uncertainty and international financial markets: The case of Brexit. Applied Economics, 50(34–35), 3752–3770. https://doi.org/10.1080/00036846.2018.1436152
- Bénassy‐Quéré, A., Coupet, M., & Mayer, T. (2007). Institutional determinants of foreign direct investment. World Economy, 30(5), 764–782. https://doi.org/10.1111/j.1467-9701.2007.01022.x
- Bhasin, N., & Garg, S. (2020). Impact of institutional environment on inward FDI: A case of select emerging market economies. Global Business Review, 21(5), 1279–1301. https://doi.org/10.1177/0972150919856989
- Bhasin, N., & Manocha, R. (2016). Do bilateral investment treaties promote FDI inflows? Evidence from India. Vikalpa, 41(4), 275–287. https://doi.org/10.1177/0256090916666681
- Blonigen, B. A., & Piger, J. (2014). Determinants of foreign direct investment. Canadian Journal of Economics/Revue Canadienne D’économique, 47(3), 775–812. https://doi.org/10.1111/caje.12091
- Blundell, R., & Bond, S. (1998). Initial conditions and moment restrictions in dynamic panel data models. Journal of Econometrics, 87(1), 115–143. https://doi.org/10.1016/S0304-4076(98)00009-8
- Bổn, N. V. (2020). The effect of FDI on private investment in the southeast region of Vietnam. Dalat University Journal of Science, 10(4), 32–42. https://doi.org/10.37569/DalatUniversity.10.4.765(2020)
- Borensztein, E., De Gregorio, J., & Lee, J. W. (1998). How does foreign direct investment affect economic growth? Journal of International Economics, 45(1), 115–135. https://doi.org/10.1016/S0022-1996(97)00033-0
- Braconier, H., Norbäck, P. J., & Urban, D. (2005). Multinational enterprises and wage costs: Vertical FDI revisited. Journal of International Economics, 67(2), 446–470. https://doi.org/10.1016/j.jinteco.2004.08.011
- Brada, J. C., Drabek, Z., Mendez, J. A., & Perez, M. F. (2019). National levels of corruption and foreign direct investment. Journal of Comparative Economics, 47(1), 31–49. https://doi.org/10.1016/j.jce.2018.10.005
- Buchanan, B. G., Le, Q. V., & Rishi, M. (2012). Foreign direct investment and institutional quality: Some empirical evidence. International Review of Financial Analysis, 21(C), 81–89. https://doi.org/10.1016/j.irfa.2011.10.001
- Buthe, T., & Milner, H. V. (2008). The politics of foreign direct investment into developing countries: Increasing FDI through international trade agreements? American Journal of Political Science, 52(4), 741–762. https://doi.org/10.1111/j.1540-5907.2008.00340.x
- Caggiano, G., Castelnuovo, E., & Figueres, J. M. (2017). Economic policy uncertainty and unemployment in the United States: A nonlinear approach. Economics Letters, 151(C), 31–34. econlet.2016.12.002
- Canh, N. P., Binh, N. T., Thanh, S. D., & Schinckus, C. (2020). Determinants of foreign direct investment inflows: The role of economic policy uncertainty. International Economics, 161(C), 159–172. https://doi.org/10.1016/j.inteco.2019.11.012
- Caves, R. E. (1971). International corporations: The industrial economics of foreign investment. Economica, 38(149), 1–27.
- Chakrabarti, A. (2001). The determinants of foreign direct investments: Sensitivity analyses of cross‐country regressions. kyklos, 54(1), 89–114. https://doi.org/10.1111/1467-6435.00142
- Coale, A. J., & Hoover, E. M. (2015). Population growth and economic development. Princeton University Press.
- Contractor, F. J., Dangol, R., Nuruzzaman, N., & Raghunath, S. (2020). How do country regulations and business environment impact foreign direct investment (FDI) inflows? International Business Review, 29(2), 101640. https://doi.org/10.1016/j.ibusrev.2019.101640
- Corcoran, A., & Gillanders, R. (2015). Foreign direct investment and the ease of doing business. Review of World Economics, 151(1), 103–126. https://doi.org/10.1007/s10290-014-0194-5
- Dauti, B. (2015). Determinants of foreign direct investment in transition economies, with special reference to Macedonia. Evidence from gravity model. The South East European Journal of Economics and Business, 10(2), 7–28. https://doi.org/10.1515/jeb-2015-0007
- Demir, E., & Ersan, O. (2017). Economic policy uncertainty and cash holdings: Evidence from BRIC countries. Emerging Markets Review, 33(C), 189–200. https://doi.org/10.1016/j.ememar.2017.08.001
- Djankov, S., Hart, O., McLiesh, C., & Shleifer, A. (2008). Debt enforcement around the world. Journal of Political Economy, 116(6), 1105–1149. https://doi.org/10.1086/595015
- Djankov, S., La Porta, R., Lopez-de-Silanes, F., & Shleifer, A. (2002). The regulation of entry. The Quarterly Journal of Economics, 117(1), 1–37. https://doi.org/10.1162/003355302753399436
- Dorakh, A. (2020). FDI determinants in the European Union: Empirical application. Scientific Annals of Economics and Business, 67(2), 219–232. https://doi.org/10.47743/saeb-2020-0012
- Dunning, J. H. (1980). Toward an eclectic theory of international production: Some empirical tests. Journal of International Business Studies, 11(1), 9–31. https://doi.org/10.1057/palgrave.jibs.8490593
- Fan, J. P., Morck, R., Xu, L. C., & Yeung, B. (2009). Institutions and foreign direct investment: China versus the rest of the world. World Development, 37(4), 852–865. https://doi.org/10.1016/j.worlddev.2008.07.016
- Favarra, G. (2003). An empirical reassessment of the relationship between finance and growth, No. 3-123, International Monetary Fund, pp. 14–16.
- Fon, R. M., Filippaios, F., Stoian, C., & Lee, S. H. (2021). Does foreign direct investment promote institutional development in Africa? International Business Review, 30(4), 101835. https://doi.org/10.1016/j.ibusrev.2021.101835
- Gareth, J., Daniela, W., Trevor, H., & Robert, T. (2013). An introduction to statistical learning: With applications in R. Spinger.
- Globerman, S., & Shapiro, D. (2002). Global foreign direct investment flows: The role of governance infrastructure. World Development, 30(11), 1899–1919. https://doi.org/10.1016/S0305-750X(02)00110-9
- Globerman, S., & Shapiro, D. (2003). Governance infrastructure and US foreign direct investment. Journal of International Business Studies, 34(1), 19–39. https://doi.org/10.1057/palgrave.jibs.8400001
- Gwartney, J., Lawson, R., & Hall, J. (2013). Economic Freedom Dataset, published in economic freedom of the world: 2013, annual report. Fraser Institute. https://www.fraserinstitute.org/sites/default/files/economic-freedom-of-the-world-2013.pdf
- Hansen, L. P. (1982). Large sample properties of generalized method of moments estimators. Econometrica, 50(4), 1029–1054. https://doi.org/10.2307/1912775
- Hausman, J. A., & Taylor, W. E. (1981). Panel data and unobservable individual effects. Econometrica:Journal of the Econometric Society, 1377–1398.
- Hayat, A. (2018). FDI and economic growth: The role of natural resources? Journal of Economic Studies, 45(2), 283–295. https://doi.org/10.1108/JES-05-2015-0082
- Hitt, M. A. (2016). International strategy and institutional environments. Cross Cultural & Strategic Management,23(2), 206–215.
- Hodgson, G. M., & Stoelhorst, J. W. (2014). Introduction to the special issue on the future of institutional and evolutionary economics. Journal of Institutional Economics, 10(4), 513. https://doi.org/10.1017/S1744137414000393
- Iamsiraroj, S. (2016). The foreign direct investment–Economic growth nexus. International Review of Economics & Finance, 42(C), 116–133. https://doi.org/10.1016/j.iref.2015.10.044
- IMF. (2014). Fiscal Policy & Long-Term Growth, IMF Policy Paper, Washington DC. https://www.imf.org/external/np/pp/eng/2015/042015.pdf
- Islam, M. A., Khan, M. A., Popp, J., Sroka, W., & Oláh, J. (2020). Financial development and foreign direct investment—The moderating role of quality institutions. Sustainability, 12(9), 3556. https://doi.org/10.3390/su12093556
- Jandhyala, S. (2015). International and domestic dynamics of intellectual property protection. Journal of World Business, 50(2), 284–293. https://doi.org/10.1016/j.jwb.2014.10.005
- Jensen, N. M. (2003). Democratic governance and multinational corporations: Political regimes and inflows of foreign direct investment. International Organization, 57(3), 587–616. https://doi.org/10.1017/S0020818303573040
- Jeong, H. G., Lee, B., & Pek, J. (2018). Factors influencing ASEAN FDI and the policy implications. World Economy Brief, Korea Institute for International Economic Policy, 8(21). https://think-asia.org/handle/11540/8687
- Jiang, Y., Zhou, Z., & Liu, C. (2019). Does economic policy uncertainty matter for carbon emission? Evidence from US sector level data. Environmental Science and Pollution Research, 26(24), 24380–24394. https://doi.org/10.1007/s11356-019-05627-8
- Jovanovic, B., & Jovanovic, B. (2018). Ease of doing business and FDI in the ex-socialist countries. International Economics and Economic Policy, 15(3), 587–627. https://doi.org/10.1007/s10368-017-0377-3
- Jude, C., & Levieuge, G. (2017). Growth effect of foreign direct investment in developing economies: The role of institutional quality. The World Economy, 40(4), 715–742. https://doi.org/10.1111/twec.12402
- Kawiana, I. G. P., Riana, I. G., Rihayana, I. G., & Adi, I. N. (2020). How transformational leadership intensify employee performance mediating by job satisfaction. MIX: Jurnal Ilmiah Manajemen, 10(3), 454–468. https://doi.org/10.22441/mix.2020.v10i3.010
- Kim, S., & Choi, B. (2020). The impact of the technological capability of a host country on inward FDI in OECD countries: The moderating roles of institutional quality. Sustainability, 12(22), 9711. https://doi.org/10.3390/su12229711
- Kofarbai, H. Z., & Bambale, A. J. A. (2016). Investment climate and foreign direct investment in Nigeria: The mediating role of ease of doing business. Journal of Energy and Economic Development, 2(2), 10. https://www.proquest.com/openview/c7705b005e1af4a4518294362f5addf1/1?pq-origsite=gscholar&cbl=2042854
- Kurul, Z., & Yalta, A. Y. (2017). Relationship between institutional factors and FDI flows in developing countries: New evidence from dynamic panel estimation. Economies, 5(2), 17. https://doi.org/10.3390/economies5020017
- Lankes, H. P., & Venables, A. J. (1997). Foreign direct investment in Eastern Europe and the former Soviet Union: Results from a survey of investors. In S. Zecchini (Eds.), Lessons from the economic transition (pp. 555–565). Dordrecht: Springer. https://doi.org/10.1007/978-94-011-5368-3_32
- Lanz, R., & Miroudot, S. (2011). Intra-firm trade: patterns, determinants and policy implications (OECD Trade Policy Papers, No. 114). Paris: OECD Publishing. https://doi.org/10.1787/5kg9p39lrwnn-en
- Layla, F., Majumder, S. C., Appiah, B. K., Martial, A. A. A., Randolphe, K. G., & Cardorel, O. C. (2020). A panel dynamic analysis on inward FDI and institutional quality in South Asia and South East Asia. Asian Economic and Financial Review, 10(6), 654. https://doi.org/10.18488/journal.aefr.2020.106.654.669
- Lehne, J., Mo, J., & Plekhanov, A. (2014). What determines the quality of economic institutions? Cross-country evidence(Working Paper No. 171). European Bank for Reconstruction and Development. http://dx.doi.org/10.2139/ssrn.3121122
- Levin, A., Lin, C. F., & Chu, C. S. J. (2002). Unit root tests in panel data: Asymptotic and finite-sample properties. Journal of Econometrics, 108(1), 1–24. https://doi.org/10.1016/S0304-4076(01)00098-7
- Lin, S., & Ye, H. (2018). Foreign direct investment, trade credit, and transmission of global liquidity shocks: Evidence from Chinese manufacturing firms. The Review of Financial Studies, 31(1), 206–238. https://doi.org/10.1093/rfs/hhx087
- Liu, X., Wang, C., & Wei, Y. (2001). Causal links between foreign direct investment and trade in China. China Economic Review, 12(2–3), 190–202. https://doi.org/10.1016/S1043-951X(01)00050-5
- Loree, D. W., & Guisinger, S. E. (1995). Policy and non-policy determinants of US equity foreign direct investment. Journal of International Business Studies, 26(2), 281–299. https://doi.org/10.1057/palgrave.jibs.8490174
- Masharu, U., & Nasir, M. A. (2018). Policy of foreign direct investment liberalisation in India: Implications for retail sector. International Review of Economics, 65(4), 465–487. https://doi.org/10.1007/s12232-018-0306-y
- Meyer, K. E., Mudambi, R., & Narula, R. (2011). Multinational enterprises and local contexts: The opportunities and challenges of multiple embeddedness. Journal of Management Studies, 48(2), 235–252. https://doi.org/10.1111/j.1467-6486.2010.00968.x
- Mina, W. (2020). Do GCC market-oriented labor policies encourage inward FDI flows? Research in International Business and Finance, 51. https://doi.org/10.1016/j.ribaf.2019.101092
- Musambachime, M. (1990). The impact of rapid population growth and economic decline on the quality of education: The case of Zambia. Review of African Political Economy, 17(48), 81–92. https://doi.org/10.1080/03056249008703863
- Navaretti, G. B., & Venables, A. J. (2020). Multinational firms in the world economy. Princeton, NJ: Princeton University Press.
- Nerlove, M. (1967). Experimental evidence on the estimation of dynamic economic relations from a time series of cross-section. The Economic Studies Quarterly (Tokyo. 1950), 18(3), 42–74. https://www.jstage.jst.go.jp/article/economics1950/18/3/18_3_42/_pdf
- Nguyen, Q., Kim, T., & Papanastassiou, M. (2018). Policy uncertainty, derivatives use, and firm-level FDI. Journal of International Business Studies, 49(1), 96–126. https://doi.org/10.1057/s41267-017-0090-z
- Nguyen, T. T., Huynh, T. L. D., & Wong, W. K. (2021). Factors driving openness in China trade: Corruption, exchange rate volatility, and macro determinants. Review of Pacific Basin Financial Markets and Policies, 24(2), 1–25. https://doi.org/10.1142/S0219091521500168
- Nickell, S. (1981). Biases in dynamic models with fixed effects. Econometrica: Journal of the Econometric Society, 49(6), 1417–1426. https://doi.org/10.2307/1911408
- North, D. C. (1990). Institutions, institutional change and economic performance. Cambridge University Press.
- Ostrom, E. (2009). Understanding institutional diversity. Princeton university press.
- Pääkkönen, J. (2010). Economic freedom as driver of growth in transition. Economic Systems, 34(4), 469–479. https://doi.org/10.1016/j.ecosys.2010.03.001
- Peres, M., Ameer, W., & Xu, H. (2018). The impact of institutional quality on foreign direct investment inflows: Evidence for developed and developing countries. Economic research-Ekonomska Istraživanja, 31(1), 626–644. https://doi.org/10.1080/1331677X.2018.1438906
- Prasad, H. A. C., Sathish, R., & Singh, S. S. (2014). India’s merchandise exports: Some important issues and policy suggesfions. Department of Economic Affairs, GOI, Wp. No. 3-DEA.
- Regelink, M., & Elhorst, J. P. (2015). The spatial econometrics of FDI and third country effects. Letters in Spatial and Resource Sciences, 8(1), 1–13. https://doi.org/10.1007/s12076-014-0125-z
- Rong, S., Liu, K., Huang, S., & Zhang, Q. (2020). FDI, labor market flexibility and employment in China. China Economic Review, 61. https://doi.org/10.1016/j.chieco.2020.101449
- Root, F. R., & Ahmed, A. A. (1978). The influence of policy instruments on manufacturing direct foreign investment in developing countries. Journal of International Business Studies, 9(3), 81–94. https://doi.org/10.1057/palgrave.jibs.8490670
- Sabir, S., Rafique, A., & Abbas, K. (2019). Institutions and FDI: Evidence from developed and developing countries. Financial Innovation, 5(1), 1–20. https://doi.org/10.1186/s40854-019-0123-7
- Saini, N., & Singhania, M. (2018). Determinants of FDI in developed and developing countries: A quantitative analysis using GMM. Journal of Economic Studies, 45(2), 348–382. https://doi.org/10.1108/JES-07-2016-0138
- Sarpong, B., Nketiah-Amponsah, E., & Owoo, N. S. (2020). Health and economic growth nexus: Evidence from selected sub-Saharan African (SSA) countries. Global Business Review, 21(2), 328–347. https://doi.org/10.1177/0972150918778966
- Sayari, N., Sari, R., & Hammoudeh, S. (2018). The impact of value added components of GDP and FDI on economic freedom in Europe. Economic Systems, 42(2), 282–294. https://doi.org/10.1016/j.ecosys.2017.03.003
- Serfraz, A. (2018). Foreign direct investment inflows and labor productivity in Pakistan: A sector-wise panel cointegration analysis. Asian Journal of Economics and Empirical Research, 5(1), 1–18. https://doi.org/10.20448/journal.501.2018.51.1.18
- Sethi, D., Guisinger, S. E., Phelan, S. E., & Berg, D. M. (2003). Trends in foreign direct investment flows: A theoretical and empirical analysis. Journal of International Business Studies, 34(4), 315–326. https://doi.org/10.1057/palgrave.jibs.8400034
- Stangarone, T. (2020). Rather Than COVID-19, is the US-China Trade War the Real Threat to Global Supply Chains? East Asian Policy, 12(3), 5–18. https://doi.org/10.1142/S1793930520000197
- Sun, I. Y. (2019). Why factories leaving China aren’t going to India. Economic Times. https://economictimes.indiatimes.com/markets/stocks/news/why-factories-leaving-china-arent-going-to-india/articleshow/71462428.cms?from=mdr
- Syed, Q. R., & Bouri, E. (2021). Impact of economic policy uncertainty on CO2 emissions in the US: Evidence from bootstrap ARDL approach. Journal of Public Affairs, 1–10. https://doi.org/10.1002/pa.2595
- Trevino, L. J., Thomas, D. E., & Cullen, J. (2008). The three pillars of institutional theory and FDI in Latin America: An institutionalization process. International Business Review, 17(1), 118–133. https://doi.org/10.1016/j.ibusrev.2007.10.002
- Trevisani, P. (2019). Brazil opens up an economy long shielded from competition. Wall Street Journal. https://www.wsj.com/articles/brazil-opens-up-an-economy-long-shielded-from-competition-11569244957
- Tun, Y. L., Azman-Saini, W. N. W., & Law, S. H. (2012). International evidence on the link between foreign direct investment and institutional quality. Engineering Economics, 23(4), 379–386. https://doi.org/10.5755/j01.ee.23.4.2569
- Walsh, J. P., & Yu, J. (2010). Determinants of foreign direct investment: A sectoral and institutional approach (IMF Working Paper, WP/10/187). Washington: Asia Pacific Department, IMF. https://www.imf.org/external/pubs/ft/wp/2010/wp10187.pdf
- Williamson, C. R., & Kerekes, C. B. (2011). Securing private property: Formal versus informal institutions. The Journal of Law and Economics, 54(3), 537–572. https://doi.org/10.1086/658493
- Windmeijer, F. (2005). A finite sample correction for the variance of linear efficient two-step GMM estimators. Journal of Econometrics, 126(1), 25–51. https://doi.org/10.1016/j.jeconom.2004.02.005
- WIR (World Investment Report). (2019). https://unctad.org/webflyer/world-investment-report-2019
- Wooldridge, J. M. (2010). Econometric analysis of cross section and panel data. MIT press.
- World Bank. (2015). Doing business 2016: Measuring regulatory quality and efficiency.World Bank. Retrieved from https://www.worldbank.org/en/events/2015/11/11/doing-business-2016-report
- Xu, T. (2019). Economic freedom and bilateral direct investment. Economic Modelling, 78(C), 172–179. https://doi.org/10.1016/j.econmod.2018.09.017
- Yokota, K., & Tomohara, A. (2009). A decomposition of factors influencing horizontal and vertical FDI: A separate analysis. Eastern Economic Journal, 35(4), 462–478. https://doi.org/10.1057/eej.2008.45
- Zhao, C., Liu, Z., & Ding, Y. (2020). How COVID-induced uncertainty influences Chinese firms’ OFDI binary margins. Emerging Markets Finance and Trade, 56(15), 3613–3625. https://doi.org/10.1080/1540496X.2020.1855139
Appendix
Table A1 Top 20 Investing nations in India (2006–2019)