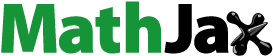
Abstract
This study addresses critical gaps in the existing literature by investigating housing affordability in developing countries and integrating quality considerations into standard affordability measures. Using survey data from a random sample of 670 households in Ho Chi Minh City, Vietnam, we conducted a distributional analysis to examine disparity in housing affordability and regression analysis to investigate factors influencing housing affordability. Our results reveal significant disparities in housing expenditure within HCMC households. On average, housing expenditure accounts for 12.78% of total household income, with renters bearing a disproportionate burden, as rent alone consumes a 27.19% of their income. The highest income quintile dedicates three times more to housing expenditure and consumption than the lowest quintile. The highest housing expenditure quintile covers 90% expenditure, while enjoying 50% of the housing consumption value. Regression results illustrate that housing expenditure and consumption are responsive to long-term income fluctuations, and more responsive to short-term income changes when comparing to results from the distributional analysis. Regressions also reveal no gender-based disparities in housing affordability. Our findings suggest a gender-neutral, affordable housing programs focusing on low-income renters, and the incorporation of flexible payment plans to address income fluctuations.
1. Introduction
Housing affordability presents a predominant policy challenge for large cities worldwide (Favilukis et al., Citation2023). A rapidly growing body of literature has emerged to address this critical issue (Lee et al., Citation2022; Malpezzi, Citation2023). Evidence suggested a decline in access to affordable housing, particularly for low and middle-income households (Lee et al., Citation2022). This challenge has been compounded by the escalation of house prices, the diminishing rates of home ownership, and the rising rents (Barrett, Citation2023).
Housing affordability issues addressed in the literature are also found in Ho Chi Minh City (HCMC), Vietnam. With a population of 9 million, HCMC is Vietnam’s primary economic and financial hub, accounting for 9.35 percent of the national population, according to (Vietnam, Citation2022). The 2019 Vietnam Population and Housing Census. Due to the city’s enormous population and ongoing influx of immigrants, the cost of housing has skyrocketed, placing an enormous financial burden on its residents. The presented situation poses a considerable challenge in attaining the targets set by Sustainable Development Goal 11, which seeks to ensure universal access to safe, affordable, and adequate housing for all individuals.
According to the 2019 Vietnam Population and Housing Census, the majority of households own their housing units, with an overall ownership ratio of 88.1%. However, there is a notable urban-rural disparity, as 77.9% of urban households own their homes, while in rural areas, the ownership rate is significantly higher at 93.6%. The remainder of the population resides in rented housing units or dwellings with unidentified ownership, with the rate of households in rented accommodation showing a considerable 5% points increase since 2009. The rate of rented households is higher in urban areas, which is 3.5 times higher than in rural regions, largely due to migration and urbanization. In highly industrialized cities such as Ho Chi Minh City, the prevalence of rented housing is remarkably elevated, reaching 32.8%.
Dominant housing types include townhouses and detached houses, while apartments constitute a mere 2.2% of the national housing stock, primarily concentrated in large cities. Urban areas exhibit a higher proportion of apartments at 5.8%, a trend driven by limited land availability and escalating land prices. The average housing area per capita stands at 23.2 m2/person, with urban areas averaging 24.5 m2/person and rural areas providing 22.5 m2/person. Regarding quality, housing units in Vietnam are usually assessed based on three fundamental components: load-bearing columns and walls, the roof, and enclosing walls, categorizing them as “solid houses and semi-solid houses” or “simple houses.” The overall prevalence of solid and semi-solid houses is 93.1%, with a breakdown of 90.3% in rural and 98.2% in urban areas such as HCMC.
In recent years, the Ho Chi Minh City (HCMC) government has adopted various measures to promote affordable housing and boost the housing stock. From 2013 to 2016, the government extended a loan program worth 1.3 billion USD to assist low-income households in purchasing houses. In 2020, it allocated an additional 130.4 million USD from the state budget, with about 43.4 million USD designated for the Bank for Social Policies and 87 million USD for four national banks to support housing loans. While these policies have yielded some positive outcomes, a significant proportion of HCMC’s populace still contends with inadequate housing. According to the 2019 Vietnam Population and Housing Census, approximately 690,000 urban households (equivalent to 3.2 million individuals) reside in substandard housing conditions, with an average living area of less than 6 m2 per person. Thus, despite the government’s efforts, more comprehensive and effective measures are required to address the persistent issue of housing deprivation in HCMC.
Housing costs in HCMC represent a significant financial strain for its residents compared to other countries. In 2020, the average household in HCMC allocate approximately 50.03% of their total disposable income on housing-related expenses, including rent, imputed rent, and maintenance and decoration costs. By contrast, housing expenditure in 2020 (including rent, imputed rent, gas, electricity, and water costs) in the United States accounted for 17.7% of disposable income, while in Australia, Canada, Japan, Korea, and the Slovak Republic, this figure ranged from 13.1% to 25%. It is generally accepted that countries where housing spending exceeds 30% of total income, face significant housing difficulties (Barrett, Citation2023). Thus, the proportion of housing expenditure in HCMC, which stands at 41%, highlights the formidable housing cost burden faced by its residents. This trend highlights the urgent need for comprehensive and effective measures to address the issue of housing affordability in HCMC.
The escalation of housing prices in HCMC has outpaced the growth of residents’ incomes. According to Numbeo, the house price-to-income ratio in 2023 is 35.17, indicating that it takes workers in HCMC 35 years of income to purchase a housing unit, a significantly more extended period than in other nations, where the process can take as little as 7 to 10 years. Additionally, HCMC’s housing conditions appear inferior to those in other countries. As of 2020, the number of bedrooms per capita in HCMC stood at 1.5 rooms per person, which is notably lower than the average bedroom rate of 2.3 rooms per person in EU countries. For example, this figure ranges from 2.9 rooms per person in Slovakia to 2.0 in Germany, Denmark, Finland, and Sweden. Consequently, residents of HCMC must contend with limited living space and disproportionately high housing costs compared to their peers in other nations.
Housing studies have centered on the critical aspect of securing housing that is both of high quality and affordability (Barrett, Citation2023). It is widely acknowledged that a single metric cannot fully encompass the myriad factors influencing households’ access to suitable housing (Robinson et al., Citation2006; UK Affordable Housing Commission, 2019). Among the most commonly employed metrics to gauge housing affordability are the price-to-income and expenditure-to-income ratios. The house price-to-income ratio serves as an indicator of housing costs in relation to income levels. The housing expenditure-to-income ratio is concerned with the proportion of household income dedicated to housing expenses (OECD, Citation2021; Robinson et al., Citation2006). Nonetheless, these ratios provide limited information into how affordability is distributed among various demographic groups (Leishman & Rowley, Citation2012; Meen, Citation2018). In addition, both the price-to-income and expenditure-to-income ratios do not cover the quality aspect of housing (Barrett, Citation2023).
Our study are based on a random sample of households in HCMC. Our examination involves two key housing affordability measures. First, we gauge housing expenditure, which encapsulates out-of-pocket housing expenses, covering items such as rent, mortgage payments, and maintenance and decoration costs. In tandem, we assess housing consumption, including rent (for renters) and imputed rent (for non-renters), and maintenance and decoration expenses. The research objectives of this paper are twofold. Firstly, we conduct a distributional analysis of these two crucial indicators. Secondly, we investigate the determinants of those two measures. Through the examination of housing consumption, we address a dimension of housing quality, echoing the perspective offered by Barrett (Citation2023), which may be overlooked when focusing solely on housing expenditures. The distributional analysis of both indicators enables an investigation into the heterogeneity within housing expenditure and opportunity costs, in line with the insights from Meen (Citation2018). Secondly, we examine the influencing factors of the two housing affordability measures, each with three variants including the housing expenditure/consumption, the expenditure/consumption per capita, and expenditure/consumption-to-income. To the best of our knowledge, no study has explored this specific issue in the context of Vietnam.
The remainder of the paper is structured as follows. Section 2 offers an overview of the literature relevant to the research objectives. In Section 3, we detail the identification strategy and data collection methods. Section 4 presents the distributional analysis of housing affordability measures, and Section 5 regression analysis results. Finally, Section 6 concludes the paper by summarizing the key insights and highlighting the implications of the study’s findings for future research and policy.
2. Literature review
Measuring housing affordability
Several measures of housing affordability exist, with most typically comparing housing costs to household incomes (Bentley, Citation2021). Among the most common are housing expenditure-to-income ratios, house price-to-income ratios, and residual income measurements (OECD, Citation2021; also see Meen, Citation2018; Ezennia & Hoskara, Citation2019; Robinson et al., Citation2006). It is widely recognized that no single measure comprehensively accounts for the various factors influencing households’ access to suitable housing (Robinson et al., Citation2006; UK Affordable Housing Commission, 2019).
The residual income measure diverges from others by emphasizing the amount of income remaining after housing expenses. Its focus is on determining whether a household has sufficient income left to cover essential non-housing expenses (Stone, Citation2006). Typically, it strives to establish a household’s basic non-housing spending needs and then evaluates whether the residual income can meet housing costs (Meen, Citation2018). This approach encounters challenges in establishing a uniform set of non-housing costs for diverse household types. Moreover, the determination of residual income cut-off points tends to be arbitrary. Additionally, this approach does not provide insights into housing quality (Murphy, Citation2014; OECD, Citation2021).
The house price-to-income ratio, on the other hand, is a straightforward indicator that relates housing costs to income levels. It quantifies the relationship between house prices and annual household incomes and is widely recognized as a simple rule-of-thumb measure of affordability (Robinson et al., Citation2006). However, at the aggregate level, such ratios offer limited insights into how affordability is distributed across various demographic groups (Leishman & Rowley, Citation2012). As such, they have limited practical utility for policy makers seeking to target housing support (OECD, Citation2021). Conversely, the housing expenditure-to-income ratio focuses on the proportion of household income allocated to housing expenses (OECD, Citation2021; Robinson et al., Citation2006). This measure offers a clearer perspective on low-income households, which tend to allocate a substantial portion of their incomes to housing costs, making it a common tool for assessing affordability.
Various studies have examined the composition of housing expenditure in empirical research. Rent, mortgage interest, property taxes, maintenance and repair costs, insurance fees, housing insurance, purchase fees, the operation of household appliances, as well as gas, electricity, water, and other costs, have been identified as components of housing expenditure in previous studies (Combs & Park, Citation1994; Fontes & Fan, Citation2006; Lino, Citation1990; Moon & Joung, Citation1997). However, some researchers exclude the costs of household appliances and equipment, as they are not considered expenses related to living space. Bui et al. (Citation2015) suggested that housing expenditure should only include rent and repair costs, while Tian et al. (Citation2017) proposed that electricity, water, property taxes, cleaning, and maintenance costs should also be considered as part of housing expenditure.
In addition, imputed rent has been suggested as an essential component of housing expenditure for homeowners, as it reflects the opportunity cost of living in their own housing units, similar to the rent paid by tenants. Some studies suggest that including imputed rent in housing expenditure would provide a more accurate measure of the opportunity costs of owning one’s own living space. Others include monthly interest and mortgage payments instead of imputed rent. However, Ulker (Citation2008) argued that these amounts do not accurately reflect consumption and should be replaced with rental equivalence, which represents the amount that homeowners would have to pay if they were renting their own home or imputed rent.
Permanent income and housing expenditure
Research on housing spending behavior is often based on the theoretical model of Muth (Citation1969). In this model, individuals are assumed to choose the housing expenditure that maximizes their utility, and thus the housing expenditure function mainly depends on rent, income, and travel costs from home to places of need.
However, some scholars argue that housing expenditure is a longer-term decision because it mainly relies on choosing the type of home to rent or purchase with long-term restrictions. As a result, a static model may not be applicable. Muth (Citation1974) developed a dynamic housing expenditure model where a household’s housing expenditure decisions are made throughout their time in a home, from the moment they move in until the time to relocate. This multi-stage utility maximization problem, akin to the single-period case, also features an income constraint with some modifications. In the single-period model, the household is assumed to consume all its income on housing and other goods. In the multi-stage model, the total income during the study period is given, including the property remaining at the end of the period. This anticipated residual asset is equivalent to the portion of income left after spending on housing and other goods. The dynamic model implies that the demand for housing services hinges on permanent income rather than income in a given period.
The rationale of the dynamic model is consistent with the concept of permanent income of Muth’s (1971), which is the income an individual or family expects to have during their lifetime. Permanent income is defined as the income that an individual or household expects to earn over their lifetime and reflects their expected wealth accumulation. However, measuring permanent income is challenging, and total expenditure is often used as a proxy for permanent income in empirical studies.
The relationship between income and housing expenditure has been extensively studied in the literature, and the findings are mixed. While some studies suggest that current income is the primary determinant of housing expenditure, others have used the concept of permanent income and found a positive relationship between permanent income and housing expenditure (Andel-Ghany & Sharpe, 1997; Fontes & Fan, Citation2006; Sanz, Citation2017). However, other studies reported no significant impact on housing expenditure, particularly for divorced or couple households. Tian et al. (Citation2017) argued that income has no effect on housing expenditure for immigrants, which suggests that the impact of income on housing expenditure may depend on the sample characteristics. Fan et al. (Citation2002) supported the view that permanent income negatively affects housing expenditure. Overall, the literature on the relationship between income and housing expenditure is inconclusive.
Other determinants of housing expenditure
Employment has been identified as a primary source of financing housing expenditure in the literature, with several studies highlighting its importance. Studies by Lino (Citation1990) and Nam and Park (Citation2015) confirmed that families with employed heads tend to have higher housing expenditures due to the secure source of income. In contrast, Spalkova and Spalek (Citation2014) found that households with more unemployed members tend to reduce their housing expenditures. Furthermore, working experience has been found to positively affect housing expenditure, as shown in the study by Kain and Quigley (Citation1975).
Although some studies have found no significant differences in housing expenditure across occupational groups (Fontes & Fan, Citation2006; Sharpe et al., Citation1995), many other studies have found that occupational categories do affect housing expenditure. Specifically, certain occupational groups tend to have higher or lower housing expenditures. For instance, Fontes and Fan (Citation2006) found that families with self-employed main income earners tend to have higher housing expenditures than those in agriculture, services, education, and management. In contrast, Vipond & Walker, Citation2009 found that unskilled workers spend less on housing.
Numerous household and individual characteristics have been found to affect housing expenditure beyond employment and occupation. Existing literature shows that higher education is associated with higher housing expenditure (Lino, Citation1990; Fan et al., Citation2002; Spalkova & Spalek, Citation2014; and Bui et al., Citation2015), with the exception of Sharpe et al. (Citation1995). The marital status of the primary income earner also has different impacts on housing spending. Fontes and Fan (Citation2006) revealed that families with single primary income earners tend to spend more on housing, while Spalkova and Spalek (Citation2014) found that married and divorced individuals have higher housing expenditures. However, some studies found no significant impact of marital status on housing expenditure (Abdel-Ghany & Sharpe, 1997).
While many studies have analyzed the effect of family structure on housing expenditure, particularly household size, number of adults, and number of children, the impact of these factors on housing expenditure remains an empirical matter due to differing results across studies. For example, some studies suggest that larger families tend to spend more on housing (Fontes & Fan, Citation2006), while others find that larger households spend less on housing (Bui et al., Citation2015; Nguyen et al., Citation2017). Some studies, such as those by Moon & Joung (Citation1997) and Lino (Citation1990), have found that household size has no impact on housing expenditure. Further analysis of household structure has shown that the impact of family size on housing expenditure differs for different types of families (Cage, Citation1994). Additionally, some studies suggest that the homeownership rate increases with household size (Fontes & Fan, Citation2006).
Apart from household size, the number of adults and children also affects housing expenditure. It is widely assumed that households with more adults will spend more on housing (Fontes & Fan, Citation2006). However, the impact of the number of children in the family on housing expenditure is more complex. While reasonable accommodation for children may lead to increased housing spending, families with young children may have higher expenditures and may spend less on housing (Fontes & Fan, Citation2006). In some cases, the two effects cancel each other out, resulting in the number of children having no impact on housing expenditure (Kain & Quigley, Citation1975; Sharpe & Abdel-Ghany, Citation1999). These results suggest that further research is needed to determine whether households with many children are likely to require housing assistance. The authors expect that households with a higher proportion of older people and children will spend more on housing.
Studies have also shown that the type of urban area in which a household resides is negatively related to housing expenditure (Spalkova & Spalek, Citation2014). Research by Tian et al. (Citation2017) found that rural-to-urban migrants who buy or rent houses have higher rates of housing expenditure, while those living in dormitories have lower rates. Fontes and Fan (Citation2006) suggest that households living in areas with more than 1.2 million population have lower housing costs than households in other areas. The authors expect that households in the inner city and those who own a house or live with other family members will spend more on housing expenditure.
In general, the drivers of housing expenditure vary across different housing markets, subjects, and periods. Most studies suggest that housing expenditure is affected by income, employment, or household demographics. Concerning income, some studies suggest that permanent income is a more appropriate measure than current income. This study focuses on the impact of permanent income, employment, and other factors on housing expenditure.
3. Methodology and data source
In this study, we use two indicators to assess housing affordability: housing expenditure and housing consumption. Housing expenditure includes all out-of-pocket expenses related to housing, covering rent (for tenants), mortgage payments (for homeowners), maintenance and repair costs, as well as expenses for decorations. While housing expenditure reveals the financial burden associated with housing, it may not provide a comprehensive reflection of housing quality. This limitation arises from the fact that individuals who own their homes outright or reside in houses owned by relatives do not incur rent expenses, resulting in lower expenditure.
To address the quality aspect of housing, we use the housing consumption indicator in this study. Although housing consumption is less common than expenditure, it has been mentioned in the literature (Aladangady, Citation2017; Shelton, Citation1994). This metric includes rent (for tenants), imputed rent (for non-renters), maintenance and repair costs, as well as expenses for decorations. The inclusion of imputed rent recognizes that individuals who do not pay rent may still incur an opportunity cost comparable to the rent they might pay if they were renters. Housing consumption do not replace housing expenditure but complement it, providing a more holistic perspective on housing affordability that accounts not only for the financial aspects but also for the inherent quality of housing, thus offering a more comprehensive understanding of the housing situation.
In our distributional analysis, we examine housing consumption and expenditure across different income quintiles. This approach allows us to understand how housing affordability measures vary among households with different income levels. Additionally, we construct Lorenz curves for both housing consumption and expenditure, offering a graphical representation of the distribution of housing affordability within the studied population. These analytical tools reveal the disparities in housing affordability.
To analyze the factors influencing housing affordability, we use an equation of the form
(1)
(1)
where
includes the two housing affordability measures, including expenditure and consumption. As mentioned, housing expenditure consists of mortgage payment (for non-renters) and rent (for renters), maintenance and repair costs, and decoration. For renters, we collected data on their monthly rent payments, while non-renters provided their monthly mortgage payments, if any. For other items in housing expenditure, we asked respondents how much their households spent on repair and maintenance and decoration in the past 12 months, then taking the monthly average. Imputed rent, which is used to calculate housing consumption, is collected by asking non-renters the estimates of their housing unit’s market rental value at the time of the survey.
In the regression equation, is the household’s permanent income,
the characteristics of the primary decision-making member, who is identified as the main income earner in the household, and
household characteristics. Characteristics of the main income earner include occupation, working experience, intention to change jobs, age, educational attainment, marital status, and gender. For household characteristics, we use household size, percentages of children and elder in the family, whether the household is doing home businesses at the housing unit, region (urban or suburban), and housing tenure.
For household characteristics, we collected data on household size, the percentage of children and elderly members, whether the household conducted home-based businesses, the region (urban or suburban), and housing tenure. Household members were defined as those residing at least six months within the last 12 months. Children were identified as those under six years old, while elderly members were aged above 60 at the time of the survey. We assessed home businesses by asking respondents whether their household operated a business at home. To assess household income, we asked respondents to choose from 18 income intervals, such as ‘0 to 5 million VND,’ ‘5 to 10 million VND,’ and so on. The use of income intervals helps mitigate potential self-reporting bias. We then calculated household income using the midpoint of the selected interval. For housing total expenditure, we requested respondents to estimate their households’ total monthly expenditure using an open-ended question. During the survey, these expenditure estimates were cross-referenced with reported income to identify any unexpected patterns or discrepancies.
The present study posits that the concept of permanent income advocated by Muth’s (1971) dynamic housing expenditure model is more fitting for HCMC. This assertion is grounded on the fact that housing expenditure is largely composed of rent and imputed rent, which are relatively fixed and thus impervious to short-term fluctuations in income. Additionally, given the high costs associated with relocating, housing expenditure is less responsive to changes in current income.
The correlation between certain forms of expenditure, particularly on consumer goods, and temporary income fluctuations is noteworthy (Winger Citation1968). Therefore, when estimating permanent income, using expenditure as a proxy may reduce income elasticity. Winger (Citation1968) also argued that total expenditure is not a reliable indicator of long-term income in housing studies due to the influence of household characteristics. To minimize such variations, we perform a regression of total expenditure on household and main income earner characteristics and utilize the resulting fitted values as a proxy for permanent income. This approach can be expressed mathematically in the form of an equation
(2)
(2)
where
is the expenditure of household
is current household income,
and
are characteristics of the main decision-making member and the household, as described earlier. Permanent income is then estimated by
(3)
(3)
The predicted values then become estimates of permanent household income after removing unexplained fluctuations. Note that these fitted values are used as a covariate in EquationEquation (1)(1)
(1) .
EquationEquation (2)(2)
(2) is estimated using the Ordinary Least Squares (OLS) estimation method. EquationEquation (1)
(1)
(1) comprises six variations with six dependent variables, encompassing two measures of housing affordability. Each measure includes three variations, including the natural logarithm of consumption/expenditure, consumption/expenditure per capita, and the percentage of consumption/expenditure in household income. EquationEquation (1)
(1)
(1) with housing consumption is also estimated using OLS. However, the three variations of housing expenditure are censored at zero, as a significant number of households report zero housing expenditure. Consequently, we employ a Tobit regression
(4)
(4)
Here, is the latent variable, assumed to be a linear function of
which includes all the groups of variables mentioned in Equationequation (1)
(1)
(1) , and
is the vector of coefficients to be estimated. In a Tobit model,
indicates the marginal effects of
on the latent variable
not
The marginal effects of
on
is given by
(5)
(5)
where
is the standard deviation of
which is estimated in a Tobit regression, and
indicates the cummulative standard normal distribution function. For Tobit regressions, we report the marginal effects on
instead of the estimated coefficients
We employed a systematic sampling method to select our sample of 670 households from a comprehensive list of approximately 2.55 million households in Ho Chi Minh City, sourced from the Vietnam Population and Housing Census. This systematic approach began with the random selection of an initial household, denoted as ‘household i,’ from a range of 1 to 3800. The value ‘3800’ was determined by dividing the total number of households in the list (2,550,000) by our target sample size of 670. Subsequently, we expanded the sample by including households at regular intervals of 3800 (i + 3800*n), where ‘n’ represents consecutive positive integers until the end of the comprehensive household list was reached. This systematic selection method was chosen to ensure that every household on the list had an identical probability of being chosen.
When addressing survey error, our use of the 2019 Vietnam Population and Housing Census provided a sampling frame closely aligned with the target population, minimizing coverage error. Our systematic sampling procedure, as explained earlier, helped eliminate sampling error. We minimized attrition by allowing respondents to pause and resume the interview at a convenient time for them. Upon obtaining consent, enumerators conducted interviews with the primary income earner or the household member responsible for managing expenditures. Respondents were fully informed about the study’s purpose and content and must sign consent forms before participation. It is important to note that, among the selected households, 40% participated in the survey, while non-responses were attributed to various factors, including instances where no one was present at the selected household after two visits, the inability to locate the address, or refusals to participate. In cases where addresses were not found, enumerators were instructed to approach households with address numbers that were closest to the originally selected ones. If a selected household was absent or refused to participate, enumerators would turn to the closest neighboring household. These replacements were made within the same neighborhoods, where households tend to share similar housing conditions and lifestyle, minimizing the potential bias and preserving the overall representativeness of the sample.
The survey was conducted using Survey Solutions, a computer-assisted personal interviewing (CAPI) system developed by the World Bank. Survey Solutions allows for real-time checks of response consistency across different sections of the questionnaire. Additionally, the platform records response times for each question, enabling us to identify any attempts by respondents to skip questions, which we can analyze. Each enumerator used a tablet to collect responses while also sharing their live locations, providing us with real-time information about their whereabouts during the survey. To ensure data accuracy, we compared demographic information from the survey with data from the 2019 Vietnam Population and Housing Census. We also cross-referenced this information with customer records from electricity and water utility providers, which we had access to, in order to identify any discrepancies. In cases where inaccuracies were identified, we ask the enumerator to revisit the household to validate or correct the responses.
The survey instrument used in this study was divided into three key sections. The initial section collected data on household demographics, including information about the primary income earner, as well as their spouse. The second section collects housing-related details, including ownership, expenditures, and conditions. Lastly, the third section was dedicated to quality control measures. It is worth noting that the data collection for this survey took place in Ho Chi Minh City (HCMC) from May to June 2020, during a period when the local housing market remained relatively unaffected by the COVID-19 pandemic and social distancing measures, maintaining its stability and positive trends.
Our survey statistics closely align with national and city-level data. According to the Vietnam Household Living Standard Survey (VHLSS) 2020 and General Statistics Office (GSO) HCMC, average monthly household income is 1,012 USD. In our survey, it is 952 USD, similar to the official figures. VHLSS reported HCMC households spending 589 USD per month; our survey is 500 USD (excluding rent), but adding rent brings it in line with population statistics. We do note a higher average household size (4.55) compared to VHLSS (3.6). This is due to our method of counting members who are permanently living in the households, whereas in some cases, respondents may report only registered household members (as in household registration books in Vietnam) in official surveys.
4. Distributional analysis of housing affordability
presents the summary statistics of the data used in this study. Approximately 57% of the primary income earners in the sample households are male, and 78% are married. The average age of the primary income earner is 44 years, and the average tenure with their current employer is 9.7 years. Educational attainment varies, with 29% completing high school, 25% completing secondary school, and 20% earning a university or higher degree. The sample is diverse in terms of occupation, with 23% working in unskilled labor, 25.5% in skilled labor, 15% in office jobs, and 11% being self-employed. Only 10% of the respondents indicated plans to change jobs in the near future.
Table 1. Summary statistics (N = 656).
The average household size in the sample is 4.5 members, with a monthly household income of US$953, of which US$500 is allocated to expenditures. A proportion of 10 %of the household members are over 60 years old, and 7 %are children under 6. In terms of housing tenure, 56.7 % of households own their housing units, 23 %reside in houses owned by relatives or other family members, and the remaining 20.3 percent are tenants.
provides a comprehensive overview of the housing affordability measures and their components. The average monthly housing expenditure stands at US$95, with a per capita housing expenditure of US$26. Housing expenditure comprises just 12.78% of the total household monthly income, a figure significantly lower than the 21% observed in OECD countries and well below the suggested 30% threshold for affordability as outlined by OECD (2019). This is partly due to the fact that only 20% of households in the sample are renters, and among homeowners, just 17 households are making mortgage payments. The majority of households either own their homes outright or reside in properties without rent. However, when we factor in imputed rent while excluding mortgage payments, the housing consumption rise to an average of US$390 per household per month, equating to US$95 in housing consumption per capita. As a result, housing consumption accounts for 50% of the monthly household income, highlighting the substantial opportunity costs associated with housing in HCMC.
Table 2. Measures of housing affordability and their components (US$/month).
The average monthly rent among renters is US$208. Housing expenditure constitutes only 12.78 percent of the household income, but rent alone accounts for 27.19 percent of renters’ household income. While housing expenditure may not appear significantly high for the overall sample, it places a substantial burden on renters. Similarly, imputed rent takes up 38.47 percent of homeowners’ and individuals living in relatives’ houses’ income. This imputed rent doesn’t immediately burden these groups but renders those without homeownership more financially vulnerable, making it challenging for young adults to establish new households. On average, mortgage payments constitute only 1.5 percent of income. However, it’s important to note that among homeowners, only 17 households are making mortgage payments, and for this specific group, mortgage payments account for a substantial 48 percent of their income. Maintenance and decoration costs represent a small proportion of total income, accounting for 3% and 1.2%, respectively. When comparing these figures to data from OECD countries, it is evident that home decoration expenditure in HCMC is approximately ten times lower than that in the OECD, whereas imputed rent in HCMC is around 30% higher than in the OECD. Furthermore, actual rent in HCMC is twice as high as in the OECD.
The distribution of housing tenure by income quintile, as depicted in , reveals distinct patterns. Homeownership rates range from 50% to 62% across quintiles, with the highest income quintile demonstrating the highest prevalence. However, no clear linear trend is evident between income and homeownership. In contrast, the proportion of renters shows relative stability in the first three lowest income quintiles but significantly decreases in the fourth and fifth quintiles, reflecting a decline in renting as income rises. Furthermore, the prevalence of living in relatives’ houses increases with ascending income quintiles, indicating a growing preference for this housing arrangement among wealthier households.
For the distributional analysis, the sample is stratified into income quintiles. In , we present housing affordability measures and household characteristics segmented by these quintiles. The highest income quintile exhibits an average monthly income of USD 2252, which is approximately seven times greater than the income in the first quintile. This difference indicates the significant income inequality within the dataset. The total household expenditure for the 5th quintile is approximately 3.6 times higher than that of the 1st quintile, implying a considerable divergence in spending capacity across income groups. When considering income on a per capita basis, the inequality appears less severe, but it remains substantial. It’s important to note that the 5th quintile has a larger household size, with an average of 5.79 members, compared to 3.55 in the 1st quintile. This implies that when income is distributed per capita, the impact of income inequality is somewhat mitigated, although it still prevails.
Table 3. Housing consumption and expenditure by income quintiles.
This inequality is mirrored in their housing consumption and expenditure, with the wealthiest quintile spending an average of USD 185.50 per month, while the lowest quintile spends only USD 64.14. The same pattern emerges in housing consumption, as the highest quintile allocates more of their resources to housing, averaging USD755 per month, compared to the lowest quintile’s USD241. For both housing expenditure and consumption, the values of the highest quintile are approximately three times higher than those of the lowest income quintile.
The inequality becomes more pronounced when considering the percentage of housing consumption and expenditures in household income. In terms of expenditure, the lowest quintile allocates approximately 20 percent of their household budget to housing, while the highest quintile spends only 8 percent. Remarkably, a substantial contrast exists between the two lowest quintiles, where this percentage is about 10%, and the higher quintiles, where it ranges from 18 to 20 percent. Housing consumption exhibit even greater disparity, consuming 75 percent of the lowest quintile’s income, while only constituting 34 percent of the highest quintile’s income. This emphasizes the high opportunity costs associated with housing in HCMC, particularly affecting households with lower incomes.
displays the Lorenz curves for housing expenditure and housing consumption. In , the concentration of housing expenditure is evident, with the first three expenditure quintiles allocating no expenses to housing, placing the entire burden on the two highest housing expenditure quintiles. Notably, the highest expenditure quintile bears approximately 90% of the total housing expenditure, highlighting a surprisingly high level of inequality in housing expenditure. This inequality is even more pronounced among the sub-sample of renters, as illustrates, with the Lorenz curve for renters significantly lower than that of non-renters. The Lorenz curve for renters exhibits a substantial reduction compared to that of non-renters, emphasizing the significant differences in housing expenditure distribution between these two groups. Among renters, a disproportionately small segment of households bears the financial burden of housing, further exacerbating the inequality within this sub-sample.
Housing consumption, inclusive of imputed rent, reveal a somewhat lower level of inequality, yet they still indicate significant disparities, as depicted in . demonstrates that the lowest housing consumption quintile accounts for just 4% of the total housing consumption, whereas the highest housing consumption quintile assumes the lion’s share at 52%. In this distribution, we can discern the potential role of housing consumption in reflecting housing quality and well-being, emphasizing substantial inequality in housing well-being across households. The pronounced variation in housing consumption among quintiles implies that certain households allocate a substantially larger proportion of their resources to housing, incurring higher opportunity costs. This, in turn, raises questions about how these disparities affect the quality of housing and overall well-being among different income groups.
The inequality in housing consumption is not uniform across all households. When we further dissect the data to differentiate between renters and non-renters, a striking difference emerges. illustrates a higher degree of inequality in housing consumption among renters, underscoring the pronounced disparities in how renters experience the consumption and potential opportunity costs associated with housing. This distinction emphasizes the varying degrees of inequality within different housing tenure groups and their respective well-being.
5. Regression results
presents the regression results for EquationEquation 1(1)
(1) . Note that we have two housing affordability measures, namely, housing expenditure and housing consumption, each with three variations (the natural logarithm, the per capita value, and the percentage in household income). Consequently, we conducted six separate regression analyses. The first three columns of the table pertain to housing consumption (Models 1–3), while the last three columns relate to housing expenditure (Models 4–6). Because housing expenditure being censored at zero, we employed Tobit regression for these analyses. The last three columns of the unconditioned marginal effects of variables on housing expenditure indicators.
Table 4. Regression results for housing consumption and expenditure.
The results of the three regression models indicate several significant factors influencing household housing expenditure in HCMC. However, these factors have varying effects on housing expenditure and consumption indicators. Specifically, a 1% increase in permanent income is associated with a 0.94% increase in housing expenditure but only a 0.54% increase in housing consumption. This suggests that as income rises, households allocate a larger portion of their budget to housing. This budget allocation increases at a rate that surpasses the rate of increase in housing consumption, where imputed rent plays a significant role. Models 2 and 5 also indicate a $0.65 per capita increase in housing consumption for every 1% income growth, in contrast to a $0.25 rise in housing expenditure per capita. Additionally, Models 3 and 6 demonstrate that a 1% increase in permanent income leads to a 0.44 percentage point increase in the share of housing consumption in income, while housing expenditure as a proportion of income remains unaffected. This suggests that housing expenditure increases proportionally with income. These findings indicate that income growth exacerbates disparities in both housing consumption and expenditure, posing challenges to the goal of achieving affordable housing for all.
This finding from regression analysis complies with previous studies such as Fan et al. (Citation2002), Fontes and Fan (Citation2006), and Bui et al. (Citation2015), but does not align with the distributional analysis in Section 4, which indicates that households with higher current income spend a significantly lower proportion of income on housing. The critical difference is that permanent income used in regression is income after removing random shocks. The difference between the two findings implies that a significant portion of the impact of income on housing expenditure is due to income fluctuations that are not permanent. In order words, households tend to adapt their housing expenses and costs in accordance with their present income rather than their future earning potential. This suggests that people are more inclined to make immediate adjustments rather than taking a more strategic and far-sighted approach to their housing expenditures.
However, removing the effect of random income shocks from the analysis provides a clearer picture of the long-term relationship between income and housing expenditure. The smaller impact of income on housing expenditure after removing the effect of random income shocks suggests that households tend to allocate a larger portion of their long-term income to housing than their short-term income. This could be because households, in the short term, prioritize spending on other items such as savings, investments, or other essential expenses that are not reflected in their long-term income fluctuations.
Using the exponential formula to calculate the effects of dummy variables, our analysis reveals that homeowners experience housing expenditures approximately 96% lower (
) than renters, while individuals living in relatives’ houses also face housing expenditures about 95% lower than renters. On the other hand, both of these groups incur housing consumption that are 50–60% higher than renters. These findings indicate that in comparison to renters, non-renters bear a significantly lighter burden of housing expenditure while enjoying a higher level of housing quality. Models 2 and 5 further illustrate that non-renters spend approximately $50 less per month on housing expenditure per capita than renters. Simultaneously, they enjoy housing consumption that is $30-37 higher per month. Non-renters also exhibit expenditure-to-income ratios that are 24–27 percentage points lower than renters while experiencing housing consumption values that are 20–29 percentage points higher in relation to their income.
Households with an additional member tend to experience a decrease in housing expenditure per capita by $2.9 per month, but this does not alter the total housing expenditure or its proportion to income. Moreover, an increase in household size reduces housing consumption per capita, yet leads to a rise in total housing consumption. As a result, housing consumption value increases by 5.7 percent with the addition of one more member. In summary, the number of household members does not significantly impact the burden of housing expenditure. This finding challenges previous research that suggested a negative relationship between household size and housing expenditure, as documented in studies by Bui et al. (Citation2015), Nguyen et al. (Citation2017), Abdel‐ghany, and Sharpe (Citation1997), Cage (Citation1994), and Combs and Park (Citation1994). Conversely, other studies, including Moon & Joung (Citation1997) and Lino (Citation1990), found no significant effect of household size on housing expenditure.
The presence of children and elderly members in a household has no significant impact on housing expenditure, its share of household income, or per capita housing expenditure. This suggests that the burden of housing expenditure remains consistent regardless of household composition, indicating that affordable housing policies should not be tailored to specific family structures. Nevertheless, an increase of one percentage point in the number of children in the household leads to a per capita reduction in housing consumption of $0.6 per month. Conversely, an additional percentage point of elderly members raises the proportion of housing consumption in household income by 0.296 percentage points. These findings indicate that household structure may result in different housing consumption values, but their housing expenditure burdens are not significantly different.
Households with home-based businesses at their residence exhibit significantly higher housing expenditure and consumption, with their per capita values and proportions in income also being notably elevated. This can be attributed to the specific location and housing conditions required for home businesses, which tend to be costlier. Notably, there is no discernible urban-rural disparity in housing expenditure, its per capita value, and its proportion in income. However, there is a substantial divergence in housing consumption values. Specifically, households in suburban areas of HCMC experience housing consumption that are 23.6 percent lower () than those in urban areas, with per capita housing consumption being $16.82 lower per month, and the proportion of housing consumption in income being 16.57 percentage points lower. This disparity can largely be attributed to imputed rent, which is significantly lower in suburban areas where homeownership rates are higher, resulting in reduced housing consumption. These results also indicate that while suburban areas may offer lower housing quality, they share a similar financial burden in terms of housing expenditure, implying that housing policies should not exclusively focus on metropolitan areas.
Some characteristics of the main income earner appear to be important factors in housing expenditure and consumption. There is no significant difference in housing expenditure and its burden in income between occupation categories of the main income earner, implying that housing affordability policies should not focus on any specific occulation categories. However, officer workers tend to have higher proportion of housing consumption in income compared to unskilled labor. Skilled workers tend to have higher housing consumption, housing consumption per capita, as well as housing consumption-to-income. The situation is similar for households with main income earners are self-employed and those belonging to “Others” occupation category, except that their housing consumption per capita is not significantly different from unskilled workers. This implies that households with main income earners of unskilled workers, office workers, workers in transportation and tourism, and those retired or unemployed, are experiencing lower housing quality and could be a target of housing policy.
The educational attainment of the primary income earner does not appear to influence housing expenditure, aligning with previous research findings in studies such as Sharpe et al. (Citation1995), Moon & Joung (Citation1997), and Nguyen et al. (Citation2017). However, it significantly impacts housing consumption and related indicators. Individuals with higher levels of education tend to exhibit notably higher housing consumption, increased housing consumption per capita, and a greater proportion of housing consumption in household income. This implies that higher education may lead to an overall rise in housing consumption, but it does not necessarily equate to a higher burden of housing expenditure for individuals with greater educational attainment.
The results of the regression analysis reveal that working experience and the intention to change jobs have no significant impact on housing expenditure. However, households with older main income earners tend to allocate more of their income to housing, resulting in higher per capita expenditure and a larger proportion of income dedicated to housing. This outcome aligns with previous studies by Fan et al. (Citation2002), Fontes and Fan (Citation2006), Cage (Citation1994), and Spalkova and Spalek (Citation2014). In contrast, other research, including studies by Sharpe et al. (Citation1995), Moon & Joung (Citation1997), Bui et al. (Citation2015), Lino (Citation1990), Sharpe and Abdel-Ghany (Citation1999), and Tian et al. (Citation2017), has reported no significant impact of age on housing expenditure. Additionally, the regression results indicate that older main income earners also tend to have higher housing consumption. Conversely, married income earners exhibit reduced spending on housing, along with lower housing consumption. Furthermore, female income earners do not significantly differ from their male counterparts in housing expenditure and costs. These findings highlight the significance of age and marital status as determinants of housing expenditure, while demonstrating that there is no gender-based disparity in the financial burden of housing expenditure and housing consumption.
6. Conclusion
This study is motivated by gaps in the existing literature, particularly the lack of research on housing affordability in developing countries and the common oversight in commonly used housing affordability measures, which often fail to account for housing quality. To address these issues, we conducted both a distributional and regression analysis based on a random sample of 670 households in HCMC. The distributional analysis involved an examination of housing consumption, reflecting housing quality and wellbeing, as well as housing expenditure, capturing the financial burden of housing. The regression analyses aimed to identify the factors influencing these housing affordability measures. For each of the housing cost and expenditure variables, we explored three variations, including the natural logarithm of the value, the per capita value, and its proportion in household income, resulting in a total of six separate regression models.
Our analysis reveals considerable disparities in housing expenditure and consumption among households in HCMC. While housing expenditure represents 12.78% of the average household income, this burden disproportionately affects renters, for whom rent alone constitutes a significant 27.19% of their household income. These figures highlight the substantial financial strain on renters in the city. Furthermore, the highest income quintile allocates roughly three times more to housing expenditure and consumption than the lowest quintile, exacerbating inequality. The disparity intensifies when assessing the percentage of household income dedicated to housing, with the lowest quintile allocating approximately 20% while the highest quintile spends only 8%. These findings emphasize that non-renting households shoulder a considerably lighter housing expenditure burden while enjoying higher housing quality. Non-renters also exhibit significantly lower expenditure-to-income ratios (24-27 percentage points less than renters) while experiencing housing consumption values that are notably higher (by 20-29 percentage points relative to their income). Consequently, implementing an affordable housing policy tailored to low-income renters could serve as a promising avenue for reducing inequality in housing expenditure and consumption.
The regression results indicate a notable relationship: both housing consumption and expenditure increase in parallel with income growth, but expenditure displays a steeper growth rate. This implies that with rising income, disparities in housing consumption and expenditure are amplified, potentially hindering the goal of ensuring affordable housing for all. In contrast to the distributional analysis, which reveals that individuals tend to make short-term income adjustments rather than adopting a long-term, strategic approach to managing their housing expenses, a significant challenge emerges in the pursuit of affordable housing. To address this challenge and enhance housing affordability, it is imperative to explore policies that incorporate flexible payment plans within affordable housing for rent initiatives. Such policies would adapt to tenant income fluctuations, bolster housing security, and provide a foundation for stable housing arrangements.
Using 10% level of significance, the regression findings reveal that the primary income earner’s occupation category does not significantly impact housing expenditure or its proportional burden on income. Moreover, higher educational attainment is associated with elevated housing consumption, but this does not necessarily result in increased housing expenditure for those with advanced education. The results also emphasize the significance of age and marital status in shaping housing expenditure, while indicating a lack of gender-based disparities in both the financial burden of housing expenditure and housing consumption. These findings suggest the importance of inclusive housing affordability policies, gender-neutral approaches, and targeted support for groups with lower educational attainment.
Future research in the field of housing affordability should consider a more comprehensive assessment of housing quality, incorporating factors such as housing conditions, amenities, and access to public services, which extend beyond the scope of housing consumption and expenditure. Additionally, addressing potential bias in self-reported imputed rent is essential, and exploring improved methods for collecting imputed rent or alternative measures is warranted to enhance the accuracy of housing quality assessment. Furthermore, the current study focuses exclusively on HCMC, which may not be representative of all regions in Vietnam. Therefore, future studies should consider a broader geographic scope, examining various regions within Vietnam to account for potential regional disparities in housing affordability and housing quality.
Disclosure statement
No potential conflict of interest was reported by the author(s).
Data availability statement
The dataset is owned by the funder, and its availability is subject to the funder’s discretion.
Additional information
Funding
Notes on contributors
Thuy Tien Huynh
Huynh Thuy Tien is a lecturer at the University of Economics and Law, Vietnam National University Ho Chi Minh City/VNU-HCM. Her research expertise spans various domains, including housing economics, housing policy analysis, and household behavior.
Dang Thuy Truong
Truong Dang Thuy currently serves as the Chair of the Department of Economics at the University of Economics Ho Chi Minh City. He is an economist and an active researcher with a focus on various aspects of household behavior, utility pricing, and public attitudes toward renewable energy.
References
- Abdel‐ghany, M., & Sharpe, D. L. (1997). Consumption patterns among the young-old and old-old data. Journal of Consumer Affairs, 31(1), 90–112. https://doi.org/10.1111/j.1745-6606.1997.tb00828.x
- Aladangady, A. (2017). Housing wealth and consumption: Evidence from geographically-linked microdata. American Economic Review, 107(11), 3415–3446. https://doi.org/10.1257/aer.20150491
- Barrett, P. (2023). Intersections between housing affordability and meanings of home: a review. Kōtuitui: New Zealand Journal of Social Sciences Online, 18(1), 27–44. https://doi.org/10.1080/1177083X.2022.2090969
- Bentley, A. (2021). Sticky rents and the affordability of rentals for housing in New Zealand. New Zealand Population Review, 47, 145–170.
- Bui, T. T. N., Le, T. T. N., & Daly, K. J. (2015). Microlevel impacts of remittances on household behavior: Viet Nam case study. Emerging Markets Review, 25, 176–190. https://doi.org/10.1016/j.ememar.2015.10.001
- Cage, R. (1994). How does rental assistance influence spending behavior? Monthly Labor Review, 117(5), 17–28.
- Combs, E. R., & Park, S. (1994). Housing affordability among elderly female heads of household in nonmetropolitan areas. Journal of Family and Economic Issues, 15(4), 317–328. https://doi.org/10.1007/BF02353808
- Combs, E. R., & Park, S. (1994). Housing expenditure/income ratios of older nonmetropolitan and metropolitan female heads of household. Housing and Society, 21(2), 90–100. https://doi.org/10.1080/08882746.1994.11430196
- Ezennia, S., & Hoskara, S. O. (2019). Methodological weaknesses in the measurement approaches and concept of housing affordability used in housing research: a qualitative study. PLOS One, 14(8), e0221246. https://doi.org/10.1371/journal.pone.0221246
- Fan, J. X., Abdel‐Ghany, M., & Lee, Y. G. (2002). Level of income and its distribution as determinants of Korean expenditure patterns. Family and Consumer Sciences Research Journal, 30(4), 526–539. https://doi.org/10.1177/1077727X02030004005
- Favilukis, J., Mabille, P., & Van Nieuwerburgh, S. (2023). Affordable housing and city welfare. The Review of Economic Studies, 90(1), 293–330. https://doi.org/10.1093/restud/rdac024
- Fontes, A., & Fan, J. X. (2006). The effects of ethnic identity on household budget allocation to status-conveying goods. Journal of Family and Economic Issues, 27(4), 643–663. https://doi.org/10.1007/s10834-006-9031-x
- Kain, J. F., & Quigley, J. M. (1975). Determinants of Housing Expenditures. In J. F. Kain, & J. M. Quigley (Eds.), Housing Markets and Racial Discrimination: A Microeconomic Analysis (pp. 154–189). America: NBER.
- Lee, Y., Kemp, P. A., & Reina, V. J. (2022). Drivers of housing (un)affordability in the advanced economies: a review and new evidence. Housing Studies, 37(10), 1739–1752. https://doi.org/10.1080/02673037.2022.2123623
- Leishman, C., & Rowley, S. (2012). Affordable housing. In: D. F. Clapham, W. A. V. Clark & K. Gibb (Eds.), The SAGE Handbook of Housing Studies (pp. 379–396). London: Sage.
- Lino, M. (1990). Factors affecting expenditures of single-parent households. Home Economics Research Journal, 18(3), 191–201. https://doi.org/10.1177/1077727X9001800301
- Malpezzi, S. (2023). Housing affordability and responses during times of stress: a preliminary look during the COVID-19 pandemic. Contemporary Economic Policy, 41(1), 9–40. Available from https://doi.org/10.1111/coep.12563
- Meen, G. (2018). How should housing affordability be measured. UK Collaborative Centre for Housing Evidence, 3, 3–29.
- Moon, S. J., & Joung, S. H. (1997). Expenditure patterns of divorced single-mother families and two-parent families in South Korea. Journal of Family and Economic Issues, 18(2), 147–162. [Mismatch]**** https://doi.org/10.1023/A:1024972223283
- Murphy, L. (2014). Houston, we’ve got a problem’: the political construction of a housing affordability metric in New Zealand. Housing Studies, 29(7), 893–909. https://doi.org/10.1080/02673037.2014.915291
- Muth, R. F. (1969). Cities and housing; The spatial ppattern of urban residential land use.
- Muth, R. F. (1974). Moving costs and housing expenditure. Journal of Urban Economics, 1(1), 108–125. https://doi.org/10.1016/0094-1190(74)90026-6
- Nam, S.-J., & Park, E.-Y. (2015). Relationship between the consumption status of households that include individuals with physical disabilities and their employment. Journal of Disability Policy Studies, 26(1), 33–43. https://doi.org/10.1177/1044207314526436
- Nguyen, D. L., Grote, U., & Nguyen, T. T. (2017). Migration and rural household expenditures: A case study from Vietnam. Economic Analysis and Policy, 56, 163–175. https://doi.org/10.1016/j.eap.2017.09.001
- OECD. (2021). OECD Affordable Housing Database: HC.1.5 Overview Of Affordable Housing Indicators. OECD Directorate of Employment, Labour and Social Affairs - Social Policy Division. [Title] (oecd.org).
- Robinson, M., Scobie, G., & Hallinan, B. (2006). Affordability of housing: concepts, measurement and evidence. Working Paper 06/03. Wellington: The Treasury. http://hdl.handle.net/10419/205579
- Sanz, G. (2017). The effect of rental assistance on household spending behavior Economics Undergraduate Honors Thesis, Stanford University
- Sharpe, D. L., & Abdel-Ghany, M. (1999). Identifying the poor and their consumption patterns. Family Economics and Nutrition Review, 12(2), 15–25.
- Sharpe, D. L., Abdel‐Ghany, M., & Silver, J. L. (1995). Spending patterns of lone‐parent and two‐parent Canadian families. Journal of Consumer Studies & Home Economics, 19(3), 289–298. https://doi.org/10.1111/j.1470-6431.1995.tb00551.x
- Shelton, G. G. (1994). Housing consumption: A research agenda. Housing and Society, 21(3), 75–90. https://doi.org/10.1080/08882746.1994.11430208
- Spalkova, D., & Spalek, J. (2014). Housing tenure choice and housing expenditures in the Czech Republic. Review of European Studies, 6(1), 23–33. https://doi.org/10.5539/res.v6n1p23
- Stone, M. (2006). What is housing affordability? The case for the residual income approach. Housing Policy Debate, 17(1), 151–184. https://doi.org/10.1080/10511482.2006.9521564
- Tian, X., Hu, J., Zhang, C., & Zhao, Y. (2017). Housing expenditure and home purchasing behaviors of rural-urban migrants in China. China Agricultural Economic Review, 9(4), 558–566. https://doi.org/10.1108/CAER-12-2016-0201
- Ulker, A. (2008). Household composition and housing expenditures in rental-occupied and owner-occupied markets. Family and Consumer Sciences Research Journal, 36(3), 189–207. https://doi.org/10.1177/1077727X07311686
- United Kingdom Affordable Housing Commission. (2019). Defining and measuring housing affordability – an alternative approach. Nationwide Foundation. Defining and measuring housing affordability – an alternative approach | Smith Institute (smith-institute.org.uk).
- Vietnam, G. S. Completed Results of the 2019 Viet Nam Population and Housing Census. (2022). 3–829, 1.
- Vipond, J., & Walker, J. B. (2009). The determinants of housing expenditure and owner occupation. Bulletin of the Oxford University Institute of Economics & Statistics, 34(2), 169–187. https://doi.org/10.1111/j.1468-0084.1972.mp34002001.x
- Winger, A. R. (1968). Housing and income. Economic Inquiry, 6(3), 226.