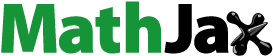
Abstract
The impact of microfinance institution (MFIs) activities on household revenue in Northern Ghana is of significant importance, particularly in light of the high levels of poverty in the region. The objective of this research is to explore the factors that influence the amount of loans granted to beneficiaries of three microfinance systems, namely the informal, semi-formal, and formal institutions, in the Upper West Region. This seeks to identify the factors that influence households’ credit access from microfinance systems. To achieve this objective, primary data collected through a questionnaire was analysed using the Ordinary Least Squares (OLS) estimation technique. The model was initially run using data from the three microfinance systems and then separately estimated for each system. The study found consistency in the variables that affect the amount of loans received by borrowers across all systems. Age, household size, interest rate charged by the institution, and group membership were found to have a negative relationship with the amount of loan received by borrowers. The study recommends that microfinance institutions in the Upper West Region focus on reducing the geographical distance between the institution and potential borrowers, providing access to microfinance information and education, and extending repayment periods to increase the loan amount borrowers receive. Moreover, the study suggests that microfinance institutions in the region should factor in the influence of interest rates on loan accessibility and adopt measures to counteract any negative consequences.
Keywords:
Reviewing Editor:
Introduction
Expanding the reach of financial services is of utmost concern to international development partners, as demonstrated by the establishment of the ‘Alliance for Financial Inclusion (AFI)’ in 2008. This unique alliance aims to empower policymakers around the world and provide high-quality financial services to socio-economic groups that have hitherto been marginalised from accessing such services. As a result, financial inclusion has become a top priority for most governments, and many policies and practices have been implemented to increase access to financial services for excluded groups (Arun & Kamath, Citation2015). Moreover, the ninth Sustainable Development Goal (SDG) of the United Nations is committed to fostering comprehensive and sustainable industrialization, with a particular emphasis on financial inclusion and credit provisions to small enterprises as a means of broadening access to financial services. Nevertheless, despite global attempts to improve financial inclusion, several underlying bottlenecks, such as exorbitant transactional fees, inadequate infrastructure development, escalating poverty, and financial service costs, continue to impede credit access in nations in sub-Saharan Africa (Batuo, Citation2015; Chikalipah, Citation2017; Demirgüç-Kunt et al., Citation2020). Additionally, in developing countries, limited household credit further constrains initiatives to promote financial inclusion due to the scarcity of credit for household consumption (Gozgor et al., Citation2019).
The global market for microfinance services has shown a noticeable upsurge within the last 20 years. The need for microfinance services grew at a minimum rate of 19% between 1997 and 2013, according to the microfinance barometer. As a result, over 3000 microfinance institutions were established, and by 2016, they were serving an astounding 200 million borrowers (Buera et al., Citation2018). Microfinance beneficiaries worldwide were 139.9 million in 2018 alone, according to the 2019 Microfinance Barometer study. This is an 11.5% annual growth rate since 2014.
Microfinance has gained a great deal of attention in the discourse around global development and provides hope to rural households looking to improve their standard of living. Because of their capacity to uplift marginalised communities and spur economic growth—particularly in the face of enduring poverty, restricted access to traditional financial services, and other socioeconomic obstacles—microfinance organisations have garnered attention. Even though the microfinance sector is expanding significantly in Sub-Saharan Africa, it is commonly acknowledged as one of the global economic regions marked by high income disparity and poverty (Agyire-Tettey et al., Citation2018).
D’Espallier et al. (Citation2017), find that 23% of the world’s MFIs manage without subsidies. Overall, the lack of subsidies worsens social performances of MFIs, and strategies to achieve financial self-sufficiency differ substantially across regions. They indicated that African and Asian MFIs compensate for non-subsidization by charging higher interest rates and that in Eastern Europe and Central Asia, unsubsidized MFIs find it more suitable to target less poor clients with unsubsidized Latin American MFIs tend to reduce their share of female borrowers.
In Ghana, microfinance is a well-established sector that provides a variety of financial services and products to the impoverished, particularly those involved in small-scale farming and other rural activities (World Bank, Citation2018). The sector is made up of various providers, including formal banks, credit unions, cooperatives, NGOs, MFIs, and rural and community banks (RCBs) (Bank of Ghana, Citation2020). Microfinance has been identified as a critical approach for reducing poverty and promoting economic growth in Ghana (Amankwah-Amoah, Citation2016; Amidu et al., Citation2022; Obeng-Odoom, Citation2019). According to the Ghana Statistical Service (GSS, Citation2022), only 18.1% of the active labour force in Ghana is employed in the formal sector, while the majority (81.9%) work in the informal sector, which includes agriculture and rural activities. Agriculture accounts for about 18.24% of the gross domestic product (GDP) and employs about 39.49% of the labour force (GSS, Citation2022).
Microfinance is a form of financial inclusion that provides access to credit and other financial services to low-income individuals and groups excluded from the formal banking sector (Dorfleitner et al., Citation2022). In many developing nations, including Ghana, microfinance has gained widespread acceptance as a method for reducing poverty and promoting economic growth (Osei-Assibey & Dikgang, Citation2020). According to the Bank of Ghana (Citation2020), as of December 2019, there were 319 licenced microfinance institutions (MFIs) operating in Ghana, serving about 2.5 million clients with a total lending portfolio of GH¢1.8 billion.
However, the microfinance sector in Ghana has faced several challenges and risks that have undermined its performance and sustainability. Some of these include poor governance and management practices, a lack of regulation and supervision, macroeconomic instability, high operational costs, low repayment rates, fraud and corruption, competition among providers, and customer dissatisfaction (Boateng et al., Citation2016). These factors have contributed to the failure or collapse of many MFIs in recent years. For instance, Boateng et al. (Citation2016) investigated four cases of MFIs that collapsed in the Ashanti Region of Ghana between 2013 and 2015. They found that these MFIs engaged in risky, unethical, and illegal practices, such as overlending, diversion of funds, and falsification of records. They also faced external pressures, such as macroeconomic shocks, panic withdrawals, and regulatory actions that exacerbated their financial distress.
One of the major challenges facing the microfinance industry in Ghana is the high level of non-performing loans (NPLs), which are loans that are overdue or defaulted on by borrowers. According to the Bank of Ghana (Citation2020), the NPL ratio of MFIs was 19.9% as of December 2019, which was higher than the average NPL ratio of 13.6% for all deposit-taking institutions in Ghana. High NPLs can adversely affect the profitability, liquidity, solvency, and sustainability of MFIs, as well as their social impact and reputation.
The Upper West region of Ghana ranks among the highest in the country for the incidence of poverty. A report by GSS (Citation2022) indicates that the region has a poverty incidence of about 45.2%, which is above the national average of about 23.4%. However, the contribution of the region to national poverty stands at only 10%. The Upper West region currently has four (4) rural banks and six (6) cooperative credit unions operating in the region. It is therefore important that conscious efforts be made to enhance the livelihood of individuals and households in the region and, in so doing, bridge the inequality gap and ultimately reduce the extent of poverty in the region.
A study by Kotir and Obeng-Odoom (Citation2009) revealed that micro-credit beneficiaries in the Upper West Region allocate a substantial portion of the loans towards household consumption. The contribution of microcredit to household productivity and welfare was determined to be moderate, while its effect on rural community development was modest. However, some scholars remain doubtful regarding the relevance of microfinance in the development process. Despite acknowledging the crucial role of microcredit in poverty reduction, several studies conclude that modern microfinance institutions have not been as effective as expected. Finch and Kocieniewski (Citation2022) even suggest that microfinance has exacerbated the debt burden of poor borrowers in developing economies rather than alleviating their poverty. They further argue that household characteristics, such as education and wealth, influence the accessibility and repayment of microfinance loans, as well as the risk of exploitation by unscrupulous lenders.
Moreover, it should be noted that previous research on microfinance in the region has not provided a comprehensive analysis of all three (3) microfinance systems (Kotir & Obeng-Odoom, Citation2009; Sekyi, Citation2017). The focus has mainly been on formal microfinance systems, while the informal or traditional ones have been overlooked. Additionally, most studies on microfinance and credit have concentrated on the demand for credit and access to it, with little attention paid to the amount of credit received (Asiamah et al., Citation2021; Tura et al., Citation2017; Waje, Citation2020).
This study seeks to address the research gap by examining the factors that influence the magnitude of loans granted to recipients through the three microfinance systems (informal, semi-formal, and formal) currently operational within the specified geographical area of interest. It is anchored on the notion that an applicant might only receive a small proportion of the amount they apply to receive and could, in effect, be less effective in consumption smoothing and household investments. It is therefore important to understand what determines the amount of loan they receive. The current study shows that factors, such as age, distance to a microfinance institution, educational status, access to microfinance information, interest rates, and repayment period could be very vital in determining the amount of credit applicants receive. This expands on the literature on the socioeconomic characteristics of an individual that influence their access to credit. The findings of this study have important implications for both theory and policy. It adds to the literature on microfinance in sub-Saharan Africa, particularly the Upper West Region. The findings will reveal what determines access to credit and thus could prove important to policymakers in their attempt to use microfinance to alleviate poverty in the region, especially as the region is ranked among the highest in the country for the incidence of poverty. A report by GSS (Citation2022) indicates that the region has a poverty incidence of about 45.2%, which is above the national average of about 23.4%.
The remainder of the paper is structured as follows: There is a review of the theoretical and empirical literature on microfinance in the next section; methodology and data sources are covered next; the penultimate section is devoted to analysis and discussion of results; and the final section draws some conclusions.
Literature review
Credit access for households, from both informal and formal institutions, has been recognised as having a considerable impact on their well-being. The availability of credit provides immediate benefits, such as smoothing consumption patterns, enabling investment, and promoting growth in incomes, ultimately leading to an overall improvement in household welfare. Access to financial services has two dimensions: the demand for and supply of credit, as argued by (Stijn, Citation2005). The supply hinges on financial intermediation. Several theories, including the information asymmetry theory and the transaction cost theory, could explain the supply of credit. According to the information asymmetry theory, there is incomplete information, which leads to an information dilemma. Ex-ante or ex-post consequences can be applied to information difficulties in the financial market. Adverse selection and moral hazard are the outcomes of ex-ante information difficulties in the financial market, whereas ex-post information problems necessitate costly compliance verifications or assurance services.
Stiglitz (Citation1990) find three financial problems that arise in a financial market. They include (i) determining the extent of default risk (the problem of screening); (ii) the cost to guarantee that credit contracts are adhered to (the incentives challenge); and (iii) the cost of monitoring credit recipients to ensure repayment of loans (the enforcement challenge). According to information theory, the provision of financial services is an effort to reduce these costs, at least in part, by facilitating easier access to information. Leland and Pyle Citation(1977), for instance, saw financial market intermediaries as a coalition that reduces information asymmetries and promotes information exchange to increase access to information.
In the context of the current study, financial institutions, in extending credit, have to avoid the identified problems and will thus pay close attention to the socio-economic characteristics of borrowers and other cultural factors. These factors include the age of the borrower, educational status, distance to the institution, and household size, among others. They could also use interest rates to regulate borrowers who enter the market for loans.
Determinants of microfinance participation
Research into microfinance has revealed that various factors impact households’ ability and willingness to participate in microfinance programs. These factors can generally be classified into socio-demographic, economic, communication, and operational categories that are directly linked to credit-granting institutions. Certain credit-granting institutions impose minimum prerequisites that clients must fulfill to participate effectively in the market, which can at times impact credit demand.
Socio-demographic variables
The literature concerning credit demand often entails discussion of socio-demographic determinants, including the age of the head of household, education level as measured by years of schooling, dependency ratio, and marital status.
Egyir (Citation2010), in his study of rural women in Ghana’s challenges to microfinance, argues that socio-cultural factors and religious constraints are not insurmountable obstacles to accessing microcredit. The study indicated that the identified limitations are the result of misconceptions, insufficient information, and low levels of education. They implied that sociocultural barriers are no longer barriers for rural women in Ghana to seek credit. However, it is imperative to acknowledge that this might not universally hold true for all women in rural areas, as some communities in Ghana may face cultural barriers to the advancement of women. Additionally, women may not receive microfinance as capital for investment but as a credit facility that might bring shame to their families. They attribute this to a lack of formal education and poor access to knowledge about the programmme.
Credit market participants must possess a certain degree of education to comprehend the intricacies of the system, particularly the loan procedures. This implies a clear correlation between one’s education and the household’s microcredit demand. In line with this, several researchers have observed this phenomenon in diverse locations and time periods. John Ewoi et al. (Citation2023), using a sample of 204 respondents in Monbasa, Kenya, studied the impact of education on loan accessibility from microfinance institutions. The result of their study indicates that education is positively related to credit access. They argue that people with higher education were more likely to receive credit relative to those with less education. Boussetta (Citation2021) argues that microfinance can reduce poverty by improving education levels, especially in high- and middle-income countries. However, he also acknowledges that households with higher levels of education and wealth may have greater access to credit and may be more willing to borrow to finance expenditures, while households with lower levels of education and wealth may face greater constraints in accessing credit and may be less likely to borrow.
Asiamah et al. (Citation2021) found that formal education was a significant determinant of credit requests and a negative determinant of credit constraints in Ghana. Tang and Guo (Citation2017) attempted to determine the credit demand and constraints among households in rural areas. They found that education significantly influenced demand for credit and significantly influenced credit constraints. Similarly, Sekyi (Citation2017), in their study of credit access and constraints among farmers in the Upper West region of Ghana, found that education was a significant and positive determinant of access to credit among farmers. Tura et al. (Citation2017), in a study in Central Ethiopia, to determine factors that influenced wheat farmers demand and access to credit, found that the amount of loan requested was positively related to the level of education. People with higher levels of education were more likely to request larger amounts of loans from microfinance institutions.
In contemporary times, advocates for the development of rural areas have shifted their focus towards a more nuanced examination of gender roles in the transformation of rural economies. This shift in focus has resulted in researchers dedicating their efforts to investigating the impact of gender on the crucial process of accessing investment capital. It is interesting to note that, despite the importance of this aspect, empirical studies on the demand for microcredit tend to neglect it in their findings. Some of these studies (De Andrés et al., Citation2021; Tura et al., Citation2017) show that men have a higher risk appetite, which affects their willingness to borrow.
Awunyo-Vitor and Abankwah (Citation2012) argue that females control fewer assets and involve themselves in small-scale businesses with relatively low output levels that require no external capital sources. Therefore, there is a need to further explore the impact of gender on the availability and use of investment capital in rural areas. Moro et al. (Citation2017), however, did not find any form of discrimination with respect to gender on access to credit, even though they argue that female entrepreneurs were less likely to request loans as compared to their male counterparts. Akudugu (Citation2012) reveals that microfinance institutions often prioritise the productivity of rural women, resulting in increased accessibility to credit for females. Aristei and Gallo (Citation2016) examine the prevalence of gender disparities in firms’ financial access, utilising firm-level data for 28 transitional European countries. They demonstrate that female-led firms are more likely to face financial constraints and higher probabilities of credit denial than male-led firms. These disparities persist despite controlling for observed firm characteristics, indicating gender-based discrimination in credit access.
The age of the household head is an essential socio-demographic factor that has a significant impact on the household’s demand for microfinance, according to Akudugu (Citation2012). In contrast to the life-cycle hypothesis, which suggests that younger individuals have a greater inclination to invest and, therefore, a higher demand for credit, Akudugu argues that the understanding of credit conditions improves as time passes, resulting in a significant positive correlation between the age of the borrower and credit demand. Tang and Guo (Citation2017) discovered that age serves a crucial role in determining demand for loans and constraints among rural Chinese farmers, with older farmers being more likely to borrow. Similarly, Sekyi (Citation2017) found a positive relationship between age and credit access among farmers in the Upper West region of Ghana.
Scholars who model the demand for loans and its determining factors have given significant attention to the consideration of household composition. Some scholars use the dependency ratio (Tura et al., Citation2017), while others, like Mpuga (Citation2010), use family status. Larger sizes of households require extra resources for investments to support the family, thus showing a positive correlation with credit demand. In addition, the findings of Mpuga (Citation2010) confirm that those who are married experience more significant social problems, which in turn compel them to seek credit. However, the cross-national results of Balogun and Yusuf (Citation2011) show that families with higher dependency have relatively low demand for loans from commercial banking institutions, NGOs, and government institutions. Mishra and Bhardwaj (Citation2022) quantile regression analysis in India found that the dependency ratio affects access to credit because it reflects the number of dependents of the borrower and thus affects the ability to repay the loan. Thus, it can be concluded that the consideration of household composition is a crucial determinant of household demand for credit.
Quoc (Citation2012) conducted an investigation in Vietnam to determine the underlying factors that impact the decision to borrow and the amount borrowed. To achieve this, the study used the double hurdle model and the Heckman selection model. The results demonstrated that the accessibility of formal credit among rural households was significantly influenced by various determinants, including household capital endowments, marital status, family size, distance to the market center, and location.
Baiyegunhi et al. (Citation2014) delved into the subject of smallholder farmers’ credit access in the Eastern Cape Province of South Africa. To accomplish this, they employed logit regression models, which enabled them to gain a deeper understanding of the various factors that influence this process. Their empirical findings revealed that a range of factors, including gender, education level, household income, asset value, savings, dependency ratio, repayment capacity, and social capital, all have a significant impact on these farmers’ ability to access credit markets.
Household economic indicators
Economic factors that indicate the demand for credit by households are wide and varied. These indicators include household earnings, their involvement in livelihood strategies, and their productive capacity. Thus, variables that are often captured include income level, participation in alternative livelihood strategies, enterprise size, value of assets, labour, and production purpose.
As noted by Okurut et al. (Citation2005), in Uganda, credit demand increases in direct proportion to household expenditure. When spending increases, households require more resources than are available, leading to the use of borrowed funds to finance their expenditures. Nwaru et al. (Citation2011) determined that in Nigeria, farm income has a direct positive relationship with the demand for informal credit, as people with higher incomes try to increase their bank savings to access loans. Being able to repay a loan was therefore a trait of high-income earners. Some individuals supplement the seasonal pattern of their farming income with an additional source of income, while others use it as collateral to secure credit. Financial institutions have the conviction that such livelihood strategies could lead to an increase in household income, resulting in their ability to repay loans in the case of default. Studies show that involvement in other income-generating activities is positively correlated to credit demand (Awunyo-Vitor & Abankwah, Citation2012).
Kiplimo et al. (Citation2015) conducted a thorough investigation into the various factors that have an impact on the ability of smallholder farmers in Kenya to obtain credit. Their research involved the utilisation of a binary logit model, which allowed for a comprehensive exploration of this complex issue. The results of their study demonstrated that several important variables, including education level, primary occupation, group membership, household income, distance to market, and access to extension services, all play a significant role in determining whether or not these farmers are able to obtain the credit they need.
Anang and Asante (Citation2020) conducted an analysis of data gathered from households in northern Ghana with the aim of investigating the determining factors of loan access among poor households. Their study revealed that several determinants, such as cattle ownership, gender, farm capital, household size, technology adoption, contact with extension services, awareness of lending institutions, and location of the farm, were significant influencers of credit access. In addition, cattle ownership, gender, farm capital, household size, and technology adoption had a noteworthy influence on the size of loans obtained.
Mduduzi and Bianca (Citation2017) conducted research in South Africa to look at the variables influencing impoverished households’ ability to obtain loans. Their research revealed that the propensity of impoverished households in South Africa to borrow was significantly influenced by several variables, including the age of the household head, race, educational attainment, gender, job, and geographic area. A study conducted by Onyeneke and Iruo (Citation2012) identified various factors that influence credit access, including farm experience, family size, and poultry stock. These findings are in line with the results reported by Kuwornu et al. (Citation2012), Dzadze et al. (Citation2012) in Ghana, and Onyeneke and Iruo (Citation2012) in Nigeria. Dzadze et al. (Citation2012) further highlighted the significance of exposure to agricultural extension services in credit accessibility. Similarly, Kuwornu et al. (Citation2012) determined that annual income and household size are crucial determinants of credit accessibility. It is important to note that formal credit institutions require collateral as a prerequisite for obtaining credit, as reported by Okpukpara (Citation2010).
The review of the literature on household characteristics that affect access to credit leads to the following hypothesis:
H1: Household characteristics have a significant effect on amount of credit received.
External factors
This section gives a brief review of the external factors and issues of information asymmetry that could influence an individual’s demand for and access to credit. These factors mostly relate to factors outside the control of the participants. Participants in the credit market must possess knowledge regarding the functioning of the market. Consequently, effective communication plays a crucial role in the analysis of microcredit demand. Social groups often provide a means for households to become aware of market developments. Other variables that are often considered include proximity to financial institutions and access to information.
Amidu (Citation2006) investigated the relationship between bank credit lending behaviour in Ghana and monetary policy. The author discovered that a financial institution’s ability to provide credit on demand is influenced by its size and liquidity. Larger banks have the capability to attract more deposits and extend more credit. To prevent default, banks have implemented varied mechanisms, including collateral and interest rate policies. However, Awunyo-Vitor and Abankwah (Citation2012) report that certain households are not able to provide the necessary collateral to obtain loans, resulting in a decrease in credit demand. Owusu-Antwi and Antwi (Citation2010) agree that relatively larger collateral requirements are one of the primary bottlenecks faced by households in terms of credit demand.
Numerous studies, including Balogun and Yusuf (Citation2011), Nwaru et al. (Citation2011), and Owusu-Antwi and Antwi (Citation2010), have emphasised the influence of interest rates on microcredit demand. However, their findings differ regarding the direction of influence on credit demand. Balogun and Yusuf (Citation2011) indicate a significant positive effect of interest on credit demand from commercial banks but a negative effect of interest rates on credit from moneylenders. The demand for credit is affected by various factors, such as interest rates, collateral requirements, and credit availability, as reported by numerous studies, such as Nwaru et al. (Citation2011). Also, (Owusu-Antwi and Antwi Citation2010) suggest an inverse relationship between interest rates and households’ demand for loans. Amonoo et al. (Citation2003) contend that credit demand is negatively impacted by interest rates and suggest reducing them to increase loan demand. These findings suggest that some findings do not align with the theoretically negatively established relationship between interest rates and loan demand.
Kebede et al. (Citation2023) find that corruption reduces both the number of active borrowers and the average loan per borrower, reduces both coverage and the amount of credit extension, and facilitates an increase in loans to female borrowers.
Literature on the factors determining microfinance demand points to various factors that influence consumer demand for microfinance products. However, it is crucial to note that such studies mainly focus on the factors affecting access to credit and demand for credit. Little is, however, known about what determines the amount of credit a borrower receives when they apply for credit. There is therefore a need to study what determines the amount of credit one receives when they apply for credit, as it is very important in determining the extent of consumption smoothing that households can engage in. This study therefore investigates the determinants of the amount of credit among borrowers in the Upper West Region.
The review of the literature on the communication and economic factors that affect access to credit leads to the following hypothesis:
H2: External factors have a significant effect on amount of loan received.
Methodology
Research design
This study utilised a cross-sectional and analytical research design. The study focused on the outcome of the determinants that affect the amount of credit extended to individual recipients from the triad of microfinance systems that are presently in operation within the confines of the study area. As a natural consequence of the study’s design, the population that was sampled consisted solely of households that had at least one member who was the beneficiary of any one of the three microfinance systems investigated.
Sampling procedure
A multi-stage sampling method was used in this research to obtain a sample that accurately represents the target population. A multi-stage sampling process involves three distinct stages. The initial phase involved the selection of districts and municipalities; the second phase involved the selection of microfinance institutions; and the third and final phase focused on the selection of beneficiaries from different microfinance systems. The research area was selected to be the Upper West Region due to the region’s high rate of poverty. The Upper West Region has the highest incidence of poverty at 45.2% above the national poverty incidence of 23.4%, even though it contributes to <10% of poverty in Ghana (GSS Citation2022). Wa Municipal, Lawra District, and Sissala East District were chosen using simple random sampling during the first stage of the multi-stage sampling method with the aim of providing equal opportunities for their inclusion in the study.
For the second stage, the formal (Sissala Rural Bank, Nandom Rural Bank, Nandom Rural Bank-Wa Branch), semi-formal (Sissala East Co-operative Credit Union, Wa Co-operative Credit Union), and informal (Susu associations) microfinance institutions were selected on the basis of their predominance in the chosen districts and municipality. The categorization of the financial institutions is done based on the level of control of these institutions by the central bank. Rural banks are classified by the Bank of Ghana as formal financial institutions. Cooperative credit unions, by nature, are guided by the Non-banking Financial Institutions Act and are not considered of formal institutions but semiformal due to the level of regulation. Susu associations are, however, considered informal institutions since they are largely not regulated.
In the third and final stage, the beneficiaries were chosen by means of simple random sampling. The members in the informal, semi-formal, and formal microfinance systems were selected using the technique of simple random sampling. An all-encompassing list of beneficiaries within the various institutions was procured, guaranteeing equal opportunities for those participants in the microfinance institutions. shows the sample frame.
Table 1. Sampling frame.
Sample size determination and data collection
The determination of the necessary size of the sample for a research project depends on available data, categorical or quantitative, and various equations can be used for this purpose. The present investigation utilizes the formula propounded by Krejcie and Morgan (Citation1970) as follows:
(1)
(1)
From EquationEquation (1)(1)
(1) , n represents the sample size required, N is the population which the sample size is drawn from.
at a 1 degree of freedom is the chi square value. From a standard chi-square table, 95% confidence level with a 1 d.f equates to 3.84. P represents the proportion of the population used in the study.
is the margin of error which is set at a default of 0.05.
This then yields;
(2)
(2)
The present study involved the acquisition of data from 360 households that have benefitted from microfinance, with 120 beneficiaries being chosen from each of the microfinance systems that were under study. It is noteworthy to mention that the sample population of 4500 participants, who have been associated with microfinance institutions for a minimum of four years, were included in this study. Primary data was the primary source of information for this study. Respondents were selected from informal, semi-formal, and formal microfinance institutions located in the region. A household survey, using semi-structured questionnaires was conducted to obtain data from beneficiary households.
Method of data analysis
A log-log model was used to model the objectives, requiring the transformation of both dependent and explanatory variables by using the natural logarithm. The reason for using the log-log model stems from its suitability for non-linear relationships, along with the assumption that the transformation of variables into logs enables the parameters to become linear, which aligns with OLS assumptions. Additionally, the use of log streamlines the interpretation of the coefficients of regression as elasticities.
Theoretically stated as:
(3)
(3)
From EquationEquation (3)(3)
(3) , the variables are defined as follows:
represents the amount of loan received by each beneficiary,
are factors derived from literature to influence the credit amount received (including the age of the beneficiary, beneficiary’s gender, household size of the beneficiary, number of years spent in school),
represents the estimated parameters and
represents the estimated error term
Drawing upon the theoretical framework outlined in EquationEquation (3)(3)
(3) , the present study specifies the empirical model as follows.:
(4)
(4)
The variables, along with their corresponding units of measurement, are outlined in . An overall model was estimated using EquationEquation (4)(4)
(4) that combines all three microfinance systems (i.e. informal, semi-formal, and formal).
Table 2. Definition of variables.
Results and discussions
Descriptive statistics
outlines the summary statistics of all the variables used in the study. The statistics outlined include the mean values, standard deviations, and minimum and maximum values of variables. The average distance of microfinance institutions from borrowers is about 2 km. While some borrowers have to move about less than a kilometre to access microfinance services, others have to move about 25 km to access microfinance services. The average age of borrowers, according to the sampled population, is 44 years, with a minimum age of 20 years and a maximum age of 70 years. The average household size is 5 persons per household, with a minimum number of 2 persons and a maximum number of 15 persons per household. The average amount of loan extended to borrowers for the sample is about 1160.40 Ghana cedis. A minimum of 50 cedis is extended to some borrowers, while others receive a maximum amount of 10,000 cedis.
Table 3. Variables summary statistics.
The average number of years of education for borrowers in the sampled population is about 4 years. While some borrowers have never had any form of formal education, others have up to 24 years of formal education. The average interest rate is about 59.9% per annum, with a minimum of 30% charged and a maximum of about 120% per annum charged for some microfinance institutions. This high average is accounted for by the high rates that are usually charged at informal microfinance institutions. The data also shows that the average repayment period for loans extended is 5 weeks. While some loans are extended with a minimum repayment period of a week, others have a repayment period of about 24 weeks.
Correlation analysis
presents the results of a pairwise correlation analysis. The results of the correlation analysis indicate that the variables are not very highly correlated to suspect the presence of serial correlation among the variables.
Table 4. Pairwise correlation.
Test for endogeneity and multicollinearity
Suspicion of endogeneity existing between the amount of credit and household income arose during the utilisation of the OLS model. To examine the endogeneity, a control function approach has been adopted. The findings of the residual analysis suggest the presence of endogeneity. The loan amount model included the residual, which was insignificant, indicating no endogeneity between the loan amount and income following the correction. Moreover, the Variance Inflation Factor (VIF) method was utilised to evaluate multicollinearity among the variables. The mean VIF of 2.75 indicates no evidence of the existence of multicollinearity among the explanatory variables.
Determinants of loan amount received
Log-log regression was utilized to estimate the factors influencing the extent of loan granted to beneficiaries. Model one was estimated involving all the three microfinance systems (i.e. informal, semi-formal, and formal). The results of the estimated coefficients are presented in .
Table 5. Results of determinants of loan amount received.
The study’s findings show that distance to the institution has a positive significant impact on the amount of credit received. A 1% increase in the distance to access loans leads to an approximate increase of 0.174% in the loan amount. This outcome corroborates the research by Sekyi (Citation2017), which also found a positive relationship between distance and loan amount. Sekyi (Citation2017) revealed that rural households travelling an extra kilometre to access credit from a credit institution experience a GHS 13.72 increase in the loan amount, which is consistent with the study by Tura et al. (Citation2017) that found a positive and significant relationship between distance and credit accessibility. However, the research by Chauke et al. (Citation2013) contradicts this finding, stating that households located far away from the institution they borrowed from are discouraged from borrowing from such credit institutions due to an increase in transaction costs.
Estimated household income has a significant effect on the amount of credit received, with a statistical significance of 1% and a positive relationship. A 1% increase in household income leads to an increase of 0.404% in the loan amount. This is because projected household income can serve as collateral, increasing household credibility and allowing them to borrow more. This finding was reported by Henri-Ukoha et al. (Citation2011) and Lotto (Citation2019), who found that the amount of credit taken by households’ is affected by the income of the household.
The beneficiary’s age significantly determines the credit amount, with a statistical significance of 10%. A 1% increase in the borrower’s age results in a 0.527% reduction in the amount of loans received. Therefore, as the borrower’s age increases, the amount of the loan taken decreases. This finding was confirmed by Sekyi (Citation2017) and Tura et al. (Citation2017), who found an inverse relationship between age and credit demand. The elderly are at risk, which can prevent them from participating in the credit market. In addition, seniors often rely on inconsistent sources of income, such as Social Security benefits, which make them less secure and lower their credit scores.
The gender impact on loan amount received is of significant statistical importance, with male beneficiaries receiving higher loan amounts than female participants. This finding agrees with previous empirical literature (Bendig et al., Citation2009; Mpuga, Citation2010), which suggests that participants who are male have a higher risk-bearing ability, resulting in an increased demand for credit. It, however, contradicts the finding of Kebede et al. (Citation2023) that, facilitated by corruption, MFIs grant more loans to female borrowers.
In addition, the preponderance of male-headed households in the study area may explain the greater control over economic resources that males possess relative to females.
Household size is negatively related to the amount of credit provided by microfinance institutions, as increasing household size leads to a decrease in the amount of credit received. Specifically, a percentage increase in household size causes a decrease of 0.881% in the amount of loan received. This finding is consistent with Ma-Azu (Citation2015) on the determinants of borrowing and its impact on food security among households in Karaga District, Northern Region, Ghana. Bendig et al. (Citation2009) further explain that large households spend a large portion of their income and have less leverage to save, therefore less leverage. As a result, he has no choice but to borrow a small amount because he does not have enough collateral to borrow a large amount.
The dependency ratio is another factor that significantly affects the credit amount received in microfinance systems. In particular, it has a significant positive effect on the size of loans obtained at the 5% level. As a result, a percentage point increase in the dependency ratio leads to an increase of 0.254 percentage points in the amount of loans issued. This finding may be due to the increase in household consumption expenditure, which may lead to the need to borrow to supplement income. Anang and Asante (Citation2020) support this finding, arguing that a high dependency ratio means that households can borrow more to meet the needs of economic growth.
The years of education of a respondent significantly determined the amount of credit received by a borrower, and this is significant at the 5% level. The result indicates that as the number of educational years attained increases, the likelihood of receiving higher amounts of credit also increases. Specifically, a 1% increase in the years of education will lead to an increase in the credit received by about 0.153%. Ma-Azu (Citation2015), in his study, also found a similar result, indicating that increasing formal education increases credit amounts. Furthermore, this finding is consistent with the findings of Sekyi (Citation2017), which showed that families with higher education are more likely to borrow compared to their less educated counterparts. Asiamah et al. (Citation2021) also found that highly educated people are not restricted in their borrowing.
The amount of loan one receives is greatly influenced by one’s access to microfinance information. The findings of the study indicate that the availability of microfinance information is of great statistical significance at the 1% level of significance. Access to microfinance information greatly improved one’s chances of increasing the amount of credit they received. Thus, people with relatively low amounts of microfinance information are likely not to receive a higher amount of credit. This result resonates with the notion that information asymmetry is a crucial factor in many individuals being excluded from the loan market. The results indicate that having access to information increases the amount of a loan by about 0.932%. This outcome is confirmed by the research of Osano and Languitone (Citation2016), who reveal that access to microfinance information increases credit accessibility in Mozambique.
The length of the repayment period for loans has a considerable effect on the credit amount received. It is significant at the 1% level. The findings reveal that a 1% increase in the duration of loan repayment would lead to a rise of ∼0.897% in the loan amount. Therefore, it can be deduced that a longer repayment period would result in a higher loan amount for the beneficiaries from the lending institutions. This can be explained by the fact that a longer loan repayment period provides more time for the beneficiaries to work with the loan, and the smaller the installment payments, the lower the repayment burden. This discovery aligns with the research conducted by Tura et al. (Citation2017), which also found the payback duration to be statistically significant in assessing access to credit from formal and informal sources. The authors argue that longer repayment periods minimise the possibility of default and thus increase the likelihood of credit access.
The negative effect of the interest rate on the loan amount is significant at 10%. Specifically, a percentage point rise in the interest rate corresponds to a decrease in the loan amount by 0.198%. These results are consistent with the research conducted by Balogun and Yusuf (Citation2011), which reported the negative impact of interest rates on loan demand, as well as the findings of Nwaru et al. (Citation2011), which indicate a similar trend in the demand for informal loans. Moreover, the results validate the observations made by (Owusu-Antwi and Antwi Citation2010), who identified the rate of interest on credit as the primary bottleneck to loan demand, thereby suggesting a negative relationship. Furthermore, the study corroborates the conclusions of Osano and Languitone (Citation2016), who found that interest rates have a detrimental effect on credit access. Consequently, higher interest rates tend to reduce access to credit.
In general, the results suggest that various factors, including but not limited to household income, gender of the recipient, dependency ratio, education level, distance, availability of information, and loan repayment period, exhibit a positive impact on the credit amount acquired by beneficiaries. Conversely, the age of the recipient, the interest rate, household size, number of members in the credit group, and experience with borrowing have a negative impact on the credit amount received by participants, as demonstrated by the findings presented in .
Determinants of loan amount received by microfinance institutions
Further, an estimation of the determinants of amount of credit received by borrowers of the respective microfinance institutions has been made. gives an overview of the determinants of loan amounts among borrowers of the three microfinance institutions (informal, semi-formal, and formal microfinance institutions).
Table 6. Determinants of loan amount by microfinance institutions.
The results in indicate that distance to microfinance institutions plays a role in influencing the amount of credit received only with informal microfinance institutions. Distance is, however, not a significant determinant between semi-formal and formal institutions. A percentage point increase in kilometres reduces the amount of loan received by borrowers of informal microfinance institutions by about 0.375%. This could result from the fact that disaggregating the data reveals underlying differences in borrower characteristics across the three sample types. It is possible that informal borrowers have different attributes compared to semiformal and formal borrowers, which may influence the relationship between distance and access to credit. Also, the disaggregation might reveal market segmentation, where different types of lenders cater to different borrower segments. It is possible that informal lenders are more prevalent in remote areas, leading to a negative effect of distance on access to credit for the informal sample. In contrast, semiformal and formal lenders might have a more widespread presence, making distance less influential for borrowers in those categories.
Belonging to a group is a significant determinant of the credit amount received by informal microfinance institutions. Being a member of a loan group increases the amount of credit received relative to people who are not members of such groups. Being a member of a group increases the percentage of loan amounts received by about 0.824%. The group lending system is a proven system against moral hazards and adverse selection, especially for informal microfinance institutions that do not have sophisticated logistics to recover loans. Thus, group lending could increase loan amounts more than individual lending. The result is consistent with the findings of (Behr et al., Citation2011), who also find that group lending increases the probability of accessing a loan. It further corroborates with Mersland and Strøm (Citation2009), who find that the outreach of microfinance institutions is lower in the case of lending to individuals than in the case of lending to solidarity groups.
The results indicate that household income has a significant effect on credit amounts across the three microfinance institutions, consistent with the result in . The results indicate that a 1% increase in household income could lead to a 0.445% increase in loans received in informal microfinance institutions, a 0.349% increase in semi-formal institutions, and a 0.435% increase in formal microfinance institutions. Lotto (Citation2019) also finds this in his study, concluding that access to credit is influenced by the incomes of households, and the relationship is significant.
Consistent with the findings presented in , the dependency ratio exerts a positive impact on the credit amount obtained from formal microfinance institutions. Specifically, a 1% increase in the dependency ratio is associated with a 0.372% rise in the loan amount disbursed by formal microfinance institutions. This outcome could potentially be explained by the fact that households with a high number of dependents may experience a surge in consumption expenditure, thereby necessitating additional borrowing to supplement their income and smooth household consumption. These results are in line with the research of Tura et al. (Citation2017), who similarly found a significant and positive relationship between the dependency ratio and loan amount. They posited that a higher dependency ratio may result in households taking out more loans to meet the needs of a growing household.
reveals that microfinance information access is a crucial determinant of the credit amount obtained from microfinance institutions. This finding is consistent with the results reported in . However, access to microfinance information is not a significant factor for formal financial institutions. Access to microfinance information leads to a 1.146 and 1.535% increase in the credit amount received from informal and semi-formal institutions, respectively. Both results are statistically significant at the 1% level.
Regarding interest rates, this factor significantly determines the loan amount for borrowers of semi-formal microfinance institutions but not for informal and formal microfinance institutions. Specifically, a 1% increase in interest rate leads to a 2.815% rise in the loan amount disbursed by semi-formal financial institutions. Consistent with the findings in , shows that longer repayment periods increase the loan amount obtained across microfinance institutions, except for formal microfinance institutions. Specifically, a one-year increase in the repayment period leads to a 1.027% rise in the loan amount disbursed by informal financial institutions and a 0.905% increase for semi-formal institutions.
The results generally indicate that regardless of whether the data is aggregated or not, the determinants of the amount of loan accessed by borrowers are consistent across the results.
Diagnostics test
To ensure the validity of the results, various tests including the test for homoscedasticity, the test for multicollinearity, specification test have been performed. The tests are indicated in . The results of the various tests indicate that the models are free from all the econometrics problems tested.
Table 7. Model diagnostic tests.
To test whether the residual from the model follows a normal distribution, the Jarque Bera test for normality has been used. As indicated in , we fail to reject the null hypothesis of normally distributed residual terms. To correct for the presence of heteroskedasticity among the residuals, the models were estimated by taking robust standard errors.
The results in also indicate that the model does not suffer from the problem of omitted variables bias and that the model is correctly specified since the test statistic is not significant.
Conclusions
Access to finance is indicated in the finance literature as a significant tool for alleviating poverty, smoothing consumption, and accumulating savings among Ghanaian households. The study sought to identify the factors affecting the credit amount received by borrowers of microfinance institutions in the upper west. Primary data was collected on respondents with the aid of a questionnaire.
The OLS estimation technique was employed to determine the factors that influenced the amount of credit received by applicants to microfinance institutions. To ensure that the results of the estimation were robust, the model initially ran an aggregated model with data from respondents from all the microfinance institutions. Further estimations were conducted using data for each of the three microfinance institutions. Diagnostic tests were conducted to determine whether the models were free from econometric issues. To cater to the problem of heteroskedasticity, robust standard errors were estimated in all models.
For all models, it is realised that there is consistency in the variables that affect the amount of loans received by borrowers. In the aggregated model, the factors that determine the credit amount accessed from the three microfinance institutions included the distance from the institution, the income of the respondent, the respondent’s gender, microfinance information access, dependency ratio, the number of years of schooling, and the period used for repayment, all of which have a significant positive significant relationship to the credit amount received. Factors, such as the age of the respondent, the size of the household, the number of persons in a group requesting a loan, and the interest rate charged by the various microfinance institutions have a negative relationship with the credit received. Other variables that affect the amount of loan received are whether the respondent belongs to a group and the experience the respondent has in borrowing. These factors are, however, relevant for only the segregated models.
Based on the results and the conclusions, the following recommendations are made:
The government should encourage microfinance institutions to offer flexible repayment periods to increase loan accessibility. This can be done by offering tax breaks or subsidies to institutions that offer flexible repayment options or by setting guidelines for institutions to follow when structuring their loan products. This would make it easier for individuals with irregular income or other financial constraints to access credit.
Further, gender-specific policies should be implemented to address the negative impact of gender on loan accessibility. For example, microfinance institutions could be encouraged to offer special loan products or services for women or to provide training and support to help women overcome any cultural or societal barriers that may be preventing them from accessing credit.
Again, group lending serves as a form of monitoring of borrowers and thus prevents the issues of moral hazards and adverse selections. However, when groups become larger, the ability to monitor reduces. It is thus recommended that groups be set to moderate sizes so that the potential for monitoring is possible. Smaller groups with possible monitoring stand a higher chance of receiving bigger loans from microfinance institutions. This can be done by providing financial incentives for institutions to offer group loans or by setting guidelines for institutions to follow when structuring group loan products. This would make it easier for individuals to access credit as a group.
Finally, the current study, being cross-sectional in nature, is subject to the limitations of cross-sectional studies in that individual heterogeneities cannot be accounted for. It is thus recommended that future studies on this subject could consider building a panel and also going beyond the Upper West Region.
Disclosure statement
No potential conflict of interest was reported by the author(s).
Additional information
Notes on contributors
Paul Bata Domanban
Paul Bata Domanban is a Senior Lecturer and the Dean of Students at the Simon Diedong Dombo University of Business and Integrated Development Studies (SDD-UBIDS), Ghana. He holds a PhD in Endogenous Development (University for Development Studies, Ghana). This current study ties in with his research interests in microfinance, poverty and development studies.
References
- Agyire-Tettey, F.,Ackah, C. G., &Asuman, D. (2018). An unconditional quantile regression based decomposition of spatial welfare inequalities in Ghana. The Journal of Development Studies, 54(3), 537–556. https://doi.org/10.1080/00220388.2017.1296571
- Akudugu, M. A. (2012). Estimation of the determinants of credit demand by farmers and supply by rural banks in Ghana’s Upper East Region. Asian Journal of Agriculture and Rural Development, 2(2), 189–200.
- Amankwah-Amoah, J. (2016). An integrative process model of organisational failure. Journal of Business Research, 69(9), 3388–3397. https://doi.org/10.1016/j.jbusres.2016.02.005
- Amidu, M. (2006). The link between monetary policy and banks lending behaviour: The Ghanaian case. Banks and Bank Systems, 1(4), 38–48.
- Amidu, M., Sissy, A. M., Abor, J. Y., & Wolfe, S. (2022). Cross-border banking, bank pricing and financial inclusion in Africa. In The economics of banking and finance in Africa: Developments in Africa’s financial systems (pp. 515–554). Springer International Publishing.
- Amonoo, E., Acquah, P. K., & Asmah, E. E. (2003). The impact of interest rates on demand for credit and loan repayment by the poor and SMEs in Ghana. IFLIP Research Paper, 92(4), 2–11.
- Anang, B. T., & Asante, B. O. (2020). Farm household access to agricultural services in northern Ghana. Heliyon, 6(11), e05517. https://doi.org/10.1016/j.heliyon.2020.e05517
- Aristei, D., & Gallo, M. (2016). Does gender matter for firms’ access to credit? Evidence from international data. Finance Research Letters, 18, 67–75. https://doi.org/10.1016/j.frl.2016.04.002
- Arun, T., & Kamath, R. (2015). Financial inclusion: Policies and practices. IIMB Management Review, 27(4), 267–287. https://doi.org/10.1016/j.iimb.2015.09.004
- Asiamah, T. A., Steel, W. F., & Ackah, C. (2021). Determinants of credit demand and credit constraints among households in Ghana. Heliyon, 7(10), e08162. https://doi.org/10.1016/j.heliyon.2021.e08162
- Awunyo-Vitor, D., & Abankwah, D. V. (2012). Substitutes or complements? Formal and informal credit demand by maize farmers in Ashanti and Brong Ahafo Regions of Ghana. International Journal of Agriculture and Forestry, 2(3), 105–112. https://doi.org/10.5923/j.ijaf.20120203.05
- Baiyegunhi, L. J. S., Fraser, G. C. G., & Darroch, M. A. G. (2014). Credit constraints and household welfare in the Eastern Cape Province, South Africa. African Journal of Agricultural Research, 5(16), 2243–2252.
- Balogun, O. L., & Yusuf, S. A. (2011). Determinants of demand for microcredit among the rural households in South-Western States, Nigeria. Journal of Agriculture & Social Sciences, 7(2), 41–48.
- Bank of Ghana (2020). Annual report and financial statements.
- Batuo, M. E. (2015). The role of telecommunications infrastructure in the regional economic growth of Africa. The Journal of Developing Areas, 49(1), 313–330. https://doi.org/10.1353/jda.2015.0005
- Behr, P.,Entzian, A., &Güttler, A. (2011). How do lending relationships affect access to credit and loan conditions in microlending? Journal of Banking & Finance, 35(8), 2169–2178. https://doi.org/10.1016/j.jbankfin.2011.01.005
- Bendig, M., Giesbert, L., & Steiner, S. (2009). Savings, credit and insurance: Household demand for formal financial services in rural Ghana. German Institute of Global and Area Studies, Research Programme: GIGA working paper No. 94.
- Boateng, F. G., Nortey, S., Asamanin Barnie, J., Dwumah, P., Acheampong, M., & Ackom-Sampene, E. (2016). Collapsing microfinance institutions in Ghana: An account of how four expanded and imploded in the Ashanti region. International Journal of African Development, 3(2), 37–62.
- Boussetta, A. (2021). Microfinance, poverty and education. Comparative Economic Studies, 64(1), 86–108. https://doi.org/10.1057/s41294-021-00145-4
- Buera, F. J.,Kaboski, J. P.,Rogerson, R., &Vizcaino, J. I. (2018). Skill Biased Structural Change. Working Paper 21165, National Bureau of Economic Research.
- Chauke, P., Motlhatlhana, M., Pfumayaramba, T., & Anim, F. (2013). Factors influencing access to credit: A case study of smallholder farmers in the Capricorn district of South Africa. African Journal of Agricultural Research, 8, 582–585. https://doi.org/10.5897/AJAR2013.6700
- Chikalipah, S. (2017). What determines financial inclusion in sub-Saharan Africa? African Journal of Economic and Management Studies, 8(1), 8–18. https://doi.org/10.1108/AJEMS-01-2016-0007
- Demirgüç-Kunt, A., Klapper, L., Singer, D., Ansar, S., & Hess, J. (2020). The Global Findex Database 2017: Measuring financial inclusion and opportunities to expand access to and use of financial services. The World Bank Economic Review, 34(Supplement_1), S2–S8. https://doi.org/10.1093/wber/lhz013
- D’Espallier, B., Hudon, M., & Szafarz, A. (2017). Aid volatility and social performance in microfinance. Nonprofit and Voluntary Sector Quarterly, 46(1), 116–140. https://doi.org/10.1177/0899764016639670
- De Andrés, P.,Gimeno, R., &De, Cabo, R. M. (2021). The gender gap in bank credit access. Journal of Corporate Finance, 71, 101782 https://doi.org/10.1016/j.jcorpfin.2020.101782
- Dorfleitner, G., Forcella, D., & Nguyen, Q. A. (2022). The digital transformation of microfinance institutions: An empirical analysis. Journal of Applied Accounting Research, 23(2), 454–479. https://doi.org/10.1108/JAAR-02-2021-0041
- Dzadze, P., Osei, M. J., Aidoo, R., & Nurah, G. K. (2012). Factors determining access to formal credit in Ghana: A case study of smallholder farmers in the Abura-Asebu Kwamankese district of central region of Ghana. Journal of Development and Agricultural Economics, 4(14), 416–423.
- Egyir, I. S. (2010, September 19–23). Rural women and microfinance in Ghana: Challenges and prospects [Paper presentation]. Contributed Paper Presented at the Joint 3rd African Association of Agricultural Economists (AAAE) and 48th Agricultural Economists Association of South Africa (AEASA) Conference, Cape Town, South Africa.
- Finch, G., & Kocieniewski, D. (2022). Tiny loans were going to solve global poverty. Then big money got involved. Bloomberg.com, 3.
- Gozgor, G., Demir, E., Belas, J., & Yesilyurt, S. (2019). Does economic uncertainty affect domestic credits? An empirical investigation. Journal of International Financial Markets, Institutions and Money, 63, 101147. https://doi.org/10.1016/j.intfin.2019.101147
- GSS (2022). 2020 Population and housing census. Ghana Statistical Service.
- Henri-Ukoha, A., Orebiyi, J. S., Obasi, P. C., Oguoma, N. N., Ohajianya, D. O., Ibekwe, U. C., & Ukoha, I. I. (2011). determinants of loan acquisition from the financial institutions by small-scale farmers in Ohafia agricultural zone of Abia state, South East Nigeria. Journal of Development and Agricultural Economics, 3(2), 69–74.
- John Ewoi, L., A Ali, B., & Stephen, M. (2023). Effect of education on microfinance institutions’ credit accessibility by persons with disabilities in Mombasa county, Kenya. Journal of Economics, Management and Trade, 29(2), 44–54. https://doi.org/10.9734/jemt/2023/v29i21078
- Kebede, J., Tawiah, V., & Gyapong, E. (2023). The effect of corruption on microfinance loan portfolio: A semiparametric analysis. Economics of Transition and Institutional Change, 31(1), 241–268. https://doi.org/10.1111/ecot.12332
- Kiplimo, J. C., Ngenoh, E., Koech, W., & Bett, J. K. (2015). Determinants of access to credit financial services by smallholder farmers in Kenya. Journal of Development and Agricultural Economics, 7(9), 303–313.
- Kotir, J. H., & Obeng-Odoom, F. (2009). Microfinance and rural development: A Ghanaian perspective. Journal of Developing Societies, 25(1), 85–105. https://doi.org/10.1177/0169796X0902500104
- Krejcie, R. V., & Morgan, D. W. (1970). Determining sample size for research activities. Educational and Psychological Measurement, 30(3), 607–610. https://doi.org/10.1177/001316447003000308
- Kuwornu, J. K., Ohene-Ntow, I. D., & Asuming-Brempong, S. (2012). Agricultural credit allocation and constraint analyses of selected maize farmers in Ghana. British Journal of Economics, Management & Trade, 2(4), 353–374.
- Leland, H. E., &Pyle, D. H. (1977). Informational asymmetries, financial structure, and financial intermediation. The Journal of Finance, 32(2), 371–387. https://doi.org/10.1111/j.1540-6261.1977.tb03277.x
- Lotto, J. (2019). Access to credit and households’ borrowing behavior in East Africa. Journal of Economics and Business, 2(2), 237–246. https://doi.org/10.31014/aior.1992.02.02.83
- Ma-Azu, A. J. (2015). Determinants of access to credit and its impact on household food security in Karaga district of the Northern region of Ghana [MPhil thesis]. Kwame Nkrumah University of Science and Technology.
- Mduduzi, B., & Bianca, F. (2017). Determinants of access to formal credit by the poor households, Studia Universitatis Babeș-Bolyai Oeconomica. Science, 62(1), 50–60.
- Mersland, R., & Strøm, R. Ø. (2009). Performance and governance in microfinance institutions. Journal of Banking & Finance, 33(4), 662–669. https://doi.org/10.1016/j.jbankfin.2008.11.009
- Mishra, A. K., & Bhardwaj, V. (2022). The determinants of access to informal credits in India: An application of quantiles via moments method. Journal of Quantitative Economics, 20(1), 1–22. https://doi.org/10.1007/s40953-021-00255-x
- Moro, A., Wisniewski, T. P., & Mantovani, G. M. (2017). Does a manager’s gender matter when accessing credit? Evidence from European data. Journal of Banking & Finance, 80, 119–134. https://doi.org/10.1016/j.jbankfin.2017.04.009
- Mpuga, P. (2010). Constraints in access to and demand for rural credit: Evidence from Uganda. African Development Review, 22(1), 115–148. https://doi.org/10.1111/j.1467-8268.2009.00230.x
- Nwaru, J. C., Essien, U. A., & Onuoha, R. E. (2011). Determinants of informal credit demand and supply among food crop farmers in Akwa Ibom State, Nigeria. Journal of Rural and Community Development, 6(1), 129–139.
- Obeng-Odoom, F. (2019). The intellectual marginalisation of Africa. African Identities, 17(3–4), 211–224. https://doi.org/10.1080/14725843.2019.1667223
- Okpukpara, B. (2010). Credit constraints and adoption of modern cassava production technologies in rural farming communities of Anambra State, Nigeria. African Journal of Agricultural Research, 5(24), 3379–3386.
- Okurut, F. N., Schoombee, A., & Berg, S. (2005). Credit demand and credit rationing in the informal financial sector in Uganda. The South African Journal of Economics, 73(3), 482–497. https://doi.org/10.1111/j.1813-6982.2005.00033.x
- Onyeneke, R. U., & Iruo, F. A. (2012). Socioeconomic analysis of the effect of microfinance on small-scale poultry production in Imo State, Nigeria. Agricultural Science Research Journals, 2(2), 84–91.
- Osano, H. M., & Languitone, H. (2016). Factors influencing access to finance by SMEs in Mozambique: Case of SMEs in Maputo central business district. Journal of Innovation and Entrepreneurship, 5(1), 1–16. https://doi.org/10.1186/s13731-016-0041-0
- Osei-Assibey, K., & Dikgang, O. (2020). International trade and economic growth: The Nexus, the evidence, and the policy implications for South Africa. The International Trade Journal, 34(6), 572–598. https://doi.org/10.1080/08853908.2020.1737598
- Owusu-Antwi, G., & Antwi, J. (2010). The analysis of the rural credit market in Ghana. International Business and Economics Research Journal, 9(8), 45–56.
- Quoc, D. V. (2012). Determinants of household access to formal credit in the rural areas of the Mekong Delta, Vietnam. African and Asian Studies, 11, 261–287.
- Sekyi, S. (2017). Rural households’ credit access and loan amount in Wa municipality, Ghana. International Journal of Economics and Financial Issues, 7(1), 506–514.
- Stiglitz, J. E. (1990). Peer Monitoring and Credit Markets. The World Bank Economic Review, 4(3), 351–366. https://doi.org/10.1093/wber/4.3.351
- Stijn, C. (2005). Access to financial services: A review of the isss and public policy objectives. World Bank Policy Research Working Paper No. 3589, Washington DC: World Bank.
- Tang, S., & Guo, S. (2017, July). Formal and informal credit markets and rural credit demand in China. In 2017 4th International Conference on Industrial Economics System and Industrial Security Engineering (IEIS) (pp. 1–7). IEEE. https://doi.org/10.1109/IEIS.2017.8078663
- Tura, E. G., Kenea, T., & Kaso, T. (2017). Determinants of demand for credit among wheat and teff smallholder farmers in central of Ethiopia (Arsi and South West Shewa). American Research Journal of Business and Management, 3(1), 17.
- Waje, S. S. (2020). Determinants of access to formal credit in rural areas of Ethiopia: Case study of smallholder households in Boloso Bombbe district, Wolaita zone, Ethiopia. Economics, 9(2), 40–48.
- World Bank (2018). Poverty and shared prosperity 2018: Piecing together the poverty puzzle. The World Bank.