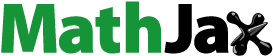
Abstract
This article evaluates the asymmetric impact of exchange rate volatility on the exports of nine ECOWAS countries to the Eurozone. By comparing Autoregressive Distributed Lag (ARDL) and Nonlinear ARDL (NARDL) models, the study concludes that the effect of volatility on ECOWAS-Eurozone exports (EEE) is asymmetric. The study also investigates the impact of foreign income and prices on the EEE and categorises the goods and services that make up the EEE for each country based on their coefficients. The results show that exchange rate volatility has an asymmetric effect on the EEE, which comprise both substitute and inferior goods. The study recommends that ECOWAS authorities avoid using proportional policies to address increased and decreased volatility, as their impact on trade is asymmetric. The long-run coefficients of income for Nigeria, Togo, and Benin are -1.29, -4.67, and -2.64 respectively, indicating that their exports are dominated by inferior goods. The long-run coefficients of foreign price for Nigeria, Niger, and Burkina Faso are 5.32, 7.87, and 1.91 respectively, suggesting that their exports are mainly substitute goods. The authors confirm long-run asymmetry for three out of nine countries and short-run asymmetry for five countries. Only three countries have an asymmetric trade-volatility relationship in both the short and long run. The study suggests that Nigeria, Togo, and Benin diversify their economies, as their exports to the Eurozone are dominated by inferior goods and services. Additionally, the study recommends that the governments of Nigeria, Niger, and Burkina Faso provide support, as their goods and services are substitutes.
1. Introduction
The collapse of the Bretton Wood exchange rate system in 1973 did not only lead to the establishment of a flexible exchange rate system, but also brought uncertainty in international trade due to high volatility in the exchange rate (Adom et al., Citation2012; Akalpler et al., Citation2017; Hooy et al., Citation2015). Exchange rate volatility refers to the unexpected and sudden swing or movement in the rate of currency exchange (Akalpler et al., Citation2017). The Economic Community of West African States (ECOWAS) is a regional economic bloc of fifteen (15) member countriesFootnote1. The establishment of the Economic Partnership Agreement (EPA) has provided a free trade area between Europe and West Africa (ECOWAS + Mauritania) in accordance with Article XXIV of GATT (ECOWAS, Citation2017; Mati et al., Citation2019; Citation2023). Although the EPA may lead to the removal of some trade barriers, uncertainty surrounding sudden exchange rate changes may impede the smooth flow of exports from ECOWAS to the Eurozone. Towards this end, this study aims to model the relationship between the ECOWAS-Eurozone exports (EEE) and exchange rate volatility to determine whether the established relationship is symmetric or asymmetric.
According to the International Monetary Fund, a 10% increase in exchange rate volatility can lead to a 1% decrease in trade flows for a typical country (Clark et al., Citation2004). A study by Rose and Yellen (Citation1989) found that exchange rate volatility had a significant negative impact on bilateral trade flows, and estimated that a 1% increase in exchange rate volatility led to a 0.5% decrease in trade. Devereux and Engel (Citation2002) estimated that exchange rate volatility can account for up to 50% of the volatility of trade flows between countries. A study by the European Central Bank found that exchange rate volatility had a larger negative impact on exports than imports, and estimated that a 1% increase in exchange rate volatility led to a 0.3% decrease in exports for a typical euro area country. (Fidora et al., Citation2020). Baier and Bergstrand (Citation2007) found that exchange rate volatility had a more negative impact on trade in intermediate goods and capital goods than on trade in final goods.
Trade-volatility relationship is symmetric (asymmetric) if the effect of increased volatility on trade is the same as (different from) the effect of decreased volatility. The common theme in some previous studies that employ export equation as in the current study is that trade-volatility relationship is symmetric (Akalpler et al., Citation2017; Asteriou et al., Citation2016). This does not capture the realities of the asymmetric relationship, which exists between the EEE and exchange rate volatility.
Bahmani-Oskooee and Aftab (Citation2017) pioneered the literature on the asymmetric effect of exchange rate volatility on trade. Despite the economic importance of the ECOWAS region, only few studies focus on the trade-volatility relationships of some of the ECOWAS members (Asteriou et al., Citation2016; Bahmani-Oskooee & Arize, Citation2020, Citation2022). The first two focus on the asymmetric effect of exchange rate volatility on the trade of selected African countries, while the last one focuses on the symmetric effect of exchange rate volatility on the trade of Mexico, Indonesia, Nigeria and Turkey. None of these studies examine the trade-volatility relationship of the ECOWAS countries or the region’s exports to the Eurozone. Akalpler et al. (Citation2017) focuses on the ECOWAS-Eurozone exports but assumes symmetry in the trade-volatility relationship.
Hooper and Kohlhagen (Citation1978) developed a basic trade-volatility model in which prices, income and uncertainty are part of the determinants of trade. The model mainly argues that trade has a negative relationship with uncertainty, and a positive correlation with prices and income. To reflect this model, the variables employed in this study are national income, prices, exports and exchange rate volatility, which represents the uncertainty. Thus, the following theoretical relationships are expected: foreign income has a positive effect on the EEE; foreign prices have a positive effect on the EEE; and exchange rate volatility has a negative effect on the EEE.
This empirical work attempts to answer some questions regarding modelling the relationship between exchange rate volatility and trade. Can the trade-volatility relationship for the EEE be modelled as asymmetric? What factors determine the EEE and how does EEE respond to them? What nature of goods or services constitute the EEE; are they substitute or complementary, inferior, necessity or luxury? How can the outcome of this study influence the decision of policy makers and businessmen in the ECOWAS? The answers to these questions can help policy makers, analysts and businessmen determine the best model that relates the EEE and exchange rate volatility, thereby guiding their economic decisions.
This study contributes to the current literature as follows. Previous studies, which used the same trade model assume symmetry of the trade-volatility relationship (Akalpler et al., Citation2017; Asteriou et al., Citation2016), while this study establishes the existence of asymmetry in the export-volatility relationship. This study identifies the nature of goods and services that dominate the EEE based on the elasticity measures. This study also relies on statistical measures to determine the IGARCH as the best volatility measure in contrast to (Akalpler et al., Citation2017; Asteriou et al., Citation2016; Bahmani-Oskooee & Arize, Citation2020). This study offers policy guidelines to the ECOWAS and Eurozone authorities.
2. Overview of the ECOWAS-EUROZONE exports
We use to visualise the change of correlation between the EEE and exchange rate volatility over time. The figure shows that the correlations fluctuate between negative and positive values, implying that volatility can cause the EEE to rise or fall.
We use EviewsR package (Mati, Citation2020; Mati et al., Citation2023) to plot , which reports the line graph of the ECOWAS-Eurozone exports in million dollars over the period of 1999 to 2017. The figure shows the declining trend of the EEE around 2008 to 2010, reflecting the consequence of the financial crisis. After that, Nigeria’s exports to the Eurozone continue to rise until 2015, a year before the country went into recession.
We use the bar chart in to compare the total exports to Eurozone by each country. The figure shows that Nigeria is the major exporter to the Euzone, followed by Cote D’Ivoire, Ghana and Senegal. The figure also reveals that Benin is at the lowest rung of the export ladder, followed by Togo, Burkina Faso and Mali.
To determine the relative contribution of each country to the EEE, we use the pie chart in . Nigeria has the highest contribution 67 per cent of the total ECOWAS exports to Eurozone, followed by Cote D’Ivoire and Ghana, which have contributed to the exports by 19 per cent and 9 per cent respectively. Thus, we can safely assert that Nigeria is the major source of the ECOWAS exports to the Eurozone.
3. Literature review
Hooper and Kohlhagen (Citation1978) developed a trade-volatility model where trade has a negative relationship with uncertainty and a positive correlation with prices and income. Krugman (Citation1983) and Helpman and Krugman (Citation1989) extended the model and introduced the concept of “product differentiation” in international trade. They argued that exchange rate volatility can affect the competitiveness of firms in different ways depending on the degree of product differentiation. Bacchetta and van Wincoop (Citation2005) developed a model that captures the relationship between exchange rate volatility and trade for homogenous goods, showing that exchange rate volatility can cause a significant reduction in trade flows, especially when exporters face high fixed costs. For a detailed survey, see Broll and Eckwert (Citation1999), Bahmani-Oskooee and Hegerty (Citation2007).
The asymmetric effects of exchange rate volatility have been examined on trade between various countries and regions. Recent studies focus on the asymmetric effects of exchange rate rate on trade between the US and the EU (Lee et al., Citation2022b), on the trade between the US and China (Lee et al., Citation2022a), on the US bilateral trade with Africa (Bahmani-Oskooee & Arize, Citation2022), on US-Pakistan trade flows (Iqbal et al., Citation2022), on trade flows of the G7 (Bahmani-Oskooee et al., Citation2022), on the Pakistan’s trade flows with major trading partners (Akhtar et al., Citation2022), on the trade between UK and China (Bahmani-Oskooee & Karamelikli, Citation2022a), and on the UK-German commodity trade (Bahmani-Oskooee & Karamelikli, Citation2022b). For similar studies on other destinations, consult Bahmani-Oskooee and Baek (Citation2021), Usman et al. (Citation2021), Hashmi et al. (Citation2021), Chang et al. (Citation2021).
A number of econometric methodologies are used to estimate the trade-volatility relationship in the literature. Some studies such as Chowdhury (Citation1993), Mckenzie (Citation1998), Aqeel and Nishat (Citation2006) and Zorlubas (Citation2011) have used the Vector Error Correction Model (VECM). Some studies, on the other hand, have employed different methodologies such as Multiple Regression (Baak, Citation2004; see Hayakawa & Kimura, Citation2009). In addition to this, some authors consider the use of the ARDL model to estimate the trade-volatility relationship (Akalpler et al., Citation2017; Asteriou et al., Citation2016). Recent studies have assumed nonlinearity in the trade-volatility relationship and therefore employed NARDL for the estimation (Arize et al., Citation2017; Bahmani-Oskooee & Aftab, Citation2017; Bahmani-Oskooee & Arize, Citation2020, Citation2022).
Various measures of volatility exist in the literature (Akalpler et al., Citation2017; Bahmani-Oskooee & Arize, Citation2020), but one of the most recent measures is the Generalized Autoregressive Conditional Heteroskedastic (GARCH) model (Asteriou et al., Citation2016; see for example, Bahmani-Oskooee & Aftab, Citation2017). Bollerslev (Citation1986) pioneered the GARCH families to serve as an extension to the Autoregressive Conditional Heteroskedastic (ARCH) model proposed by Engle (Citation1982), and extended to Integrated GARCH (IGARCH) by Nelson (Citation1990).
has shown that Mohsen Bahmani-Oskooee has the most published articles in the area of asymmetric exchange rate volatility. has shown that the United States has the most published articles in the area of asymmetric exchange rate volatility. We can discern that, despite the economic importance of the ECOWAS region, few studies are conducted related to the asymmetric effects of exchange rate on the region’s trade.
The review of the above studies implies that various studies have employed several econometric models to examine the trade-volatility relationship in different countries (Asteriou et al., Citation2016; see for example Bahmani-Oskooee & Arize, Citation2020). One common feature of these studies is that they model exchange rate as GARCH(1,1) to measure the volatility (Arize et al., Citation2017; Bahmani-Oskooee & Arize, Citation2020) or assume symmetry in the trade-volatility relationship (Akalpler et al., Citation2017; Asteriou et al., Citation2016). Therefore, this study examines the asymmetry of trade-volatility relationship and considers other variants of the GARCH families such as Integrated GARCH (IGARCH), Exponential GARCH (EGARCH) and Threshold GARCH (TGARCH) to measure the volatility.
4. Data and econometric methodology
4.1. Data
This research work utilizes monthly time series data from January, 1999 to September, 2017. This sample period has been chosen as it reflects the establishment of the Euro Area. The International Financial Statistics (IFS) database is the source of all the variables in this study. Nine (9) countries are considered in this study: Nigeria, Ghana, Senegal, Niger, Burkina Faso, Cote D’Ivoire, Mali, Benin, and Togo.
The volume of exports of each ECOWAS country to the Euro Area represents the export (X) variable. The industrial production index of the Euro Area represents foreign income (), similar to Asteriou et al. (Citation2016) and Akalpler et al. (Citation2017). The producer price index of the Euro Area serves as a measure of the foreign prices (
). Following Ribeiro et al. (Citation2020), real exchange rate (R) is calculated as the product of the nominal exchange rate (E) and the ratio of foreign prices to domestic pricesFootnote2. The consumer price index (CPI) for each country represents the domestic prices, while Eurozone CPI (
) is the proxy of foreign prices. Following Enders (Citation2015) and Akalpler et al. (Citation2017), the logarithmic change of R is employed to fit the best GARCH model for each countryFootnote3. The IGARCH (1,1) is determined to be the best model for all the countries. Subsequently, the volatility measure (V) is generated from the variance series of the IGARCH model for each country in contrast to Bahmani-Oskooee and Arize (Citation2020), Akalpler et al. (Citation2017) and Asteriou et al. (Citation2016).
4.2. Volatility measure
We follow the procedure in to estimate and decompose the volatility measure.
4.3. Export equation
We follow Asteriou et al. (Citation2016) and Akalpler et al. (Citation2017) to estimate the export equation represented by EquationEquation (1)(1)
(1) :
(1)
(1)
From the viewpoint of the ECOWAS countries, their exports (Xt) are determined by foreign income (), foreign price (
) and uncertainty, which is measured by the volatility (Vt). Thus, the following theoretical relationships are expected: foreign income has a positive effect on the EEE; foreign prices have a positive effect on the EEE; and exchange rate volatility has a negative effect on the EEE.
However, this study differs from the previous studies that use the same export equation but consider symmetric volatility effect, instead of asymmetric relationship.
4.4. ARDL and NARDL approaches
Trade models guides the choice of variables, but do not specify the econometric model(s) suitable for the variables. This gives the researchers the freedom to choose various econometric models at their disposal (Arize et al., Citation2017; see for example Asteriou et al., Citation2016; Bahmani-Oskooee & Arize, Citation2020, Citation2022). The econometric models considered in this study include the Autoregressive Distributed Lag (ARDL) model that assumes symmetry (linearity) of trade-volatility relationship, and the Nonlinear Autoregressive Distributed Lag (NARDL) model that considers the trade-volatility relationship to be asymmetric (nonlinear).
The first approach, the ARDL model, was developed by Pesaran et al. (Citation2001) and it can be applied to a mixture of I(1) and I(0), or purely I(1) regressors. Asteriou et al. (Citation2016) and Akalpler et al. (Citation2017) employed the same econometric technique. In simple form, the ARDL modelling approach involves estimating the following conditional error correction models (see Akalpler et al., Citation2017; Asteriou et al., Citation2016):
(2)
(2)
In EquationEquation (2)(2)
(2) , Δ is the difference operator, Yt is the dependent variable, Xt is a set of independent variables and ut is a serially independent random error term with zero mean. The bounds test is used for investigating one or more long-run relationships among the variables in the equation. The null hypothesis of no co-integration and the alternative hypothesis of co-integration are given below:
In the case of co-integration based on the bounds test, the error correction model (ECM) can be estimated. The ECM is a re-parametrization of the ARDL (Asteriou et al., Citation2016; Pesaran et al., Citation2001). Therefore, the most informative way to write the error correction models of co-integration can be specified as follows:
(3)
(3)
In EquationEquation (3)(3)
(3) , Δ denotes the difference operator,
is a serially independent random error with zero mean, and
is the white-noise error term, π is the coefficient of adjustment derived from the long-run co-integration model. In fact, π reveals how much of the equilibrium error is corrected each period and it is expected to be negative and statistically significant. If π = 0, then there is no adjustment and therefore there is no long-run relationship (see Pesaran et al., Citation2001 for details).
The second approach is the NARDL model, which considers one or more of the regressors as nonlinear (Shin et al., Citation2011; Citation2014). Bahmani-Oskooee and Arize (Citation2020) utilizes NARDL to estimate the asymmetric trade-volatility relationship. In a similar fashion, the nonlinear ARDL (NARDL) can be represented as:
The NARDL involves decomposition of the volatility into the partial sums of positive and negative changes. The volatility can be decomposed as follows;
Where and
are the partial sums of positive and negative changes respectively.
The export equation can be transformed in the form of the ARDL and NARDL models based on EquationEquations (2)(2)
(2) . The ARDL is modelled without decomposition of the volatility as in EquationEquation (4)
(4)
(4)
(4)
(4)
After using the decomposed volatility measures and
in place of Vt in EquationEquation (4)
(4)
(4) , the NARDL can be written as in EquationEquation (5)
(5)
(5) .
(5)
(5)
5. Empirical results
This section covers the estimation of the ARIMA(p, d, q), GARCH(p, q), ARDL and NARDL models. Subsequently, the last two models are compared and the better model is explained.
5.1. ARIMA(p, d, q) for the real exchange rate
After calculating the real exchange rate for each country, formal unit root tests based on Augmented Dickey Fuller (Dickey & Fuller, Citation1979) and Phillips-Perron (Phillips & Perron, Citation1988) are conducted. It is found that the real exchange rate is integrated of order one for all the countriesFootnote4. Thus, transformation of the series is required for the ARIMA(p, d, q) (Alamrouni et al., Citation2022; Mati, Citation2021). To that end, we consider taking the natural logarithm and first difference of each of the series to make it stationary. The best fitting ARIMA(p, d, q) for each country is given in EquationEquation (6)(6)
(6) . Although ARIMA(0,1,1) has lower SIC value for all the countries except Ghana, Senegal and Burkina, it is more reasonable to work with the reported ARIMA models because their correlograms resemble the theoretical ARIMA(1,1,0) modelFootnote5.
EquationEquation (6)(6)
(6) reports the best ARIMA models of the real exchange rate for each country. The reported coefficients are statistically significant and the error terms are white noise. We use these models to estimate the respective GARCH models reported in .
(6)
(6)
Table 1. Mean and variance equations of the GARCH.
Where R stands for the real exchange rate, Δ is the difference operator and ln is the natural logarithm.
5.2. The GARCH models
In this section, the maximum likelihood (ML) technique is used to estimate the variance equation of the GARCH model for each country, with the respective best ARIMA(p, d, q) model as the mean equation. The GARCH models compared include IGARCH, GARCH, EGARCH, TGARCH and using various lag orders. The IGARCH happens to be the best-fitting model among the GARCH families for each country. The IGARCH model is chosen because the value of the Schwarz Information Criterion (SIC) is minimum, all the parameters are statistically significant, absence of autocorrelations among standardized residuals and standardized residuals squared. presents the best GARCH model for each country. The variance series of the IGARCH model represents the volatility measure for the respective country.
5.3. Bounds test
After generating the volatility measure, we then take the natural logarithm of all the variables, plot the line graphs of each variable to ensure the absence of outliers and test for the presence of a unit root. Exports, relative price and foreign income and volatility are I(1) for all the countries. However, the decomposed volatility measures are I(0) for each country. Therefore, the ARDL and NARDL are the most suitable models for the trade equations. After that, the bounds test based on EquationEquation (2)(2)
(2) is conducted to establish the existence of a long-run relationships among the variables in the export equation for each country. As shown in , the F-value obtained from this test is then compared with the lower and upper critical values for the F-statistic found in Pesaran et al. (Citation2001). There is no cointegration if the F-value is lower than I(0), cointegration exists if the F-value is greater than the I(1) critical value, and it is inconclusive if the F-value lies between these two extreme critical values. In addition to this, ARDL and NARDL are compared in order to choose a better model between them.
Table 2. F-statistic of cointegration relationship.
reveals that the F values for the symmetric model for Togo (4.342421) lies above the I(0) and I(1) critical values of 5% and 10%, but lies between the extreme critical values of 1%. This implies that cointegration exists for the Togo-Eurozone export equation at 5% and 10%, but it is inconclusive at 1%. On the other hand, the F-value for the asymmetric model (8.603874) of Togo’s export equation is greater than the I(1) critical value, implying the existence of cointegration at all the conventional significance levels. For Ghana, the F-values for the ARDL (3.024867) lie below the I(0) and I(1) critical values at 1% and 5%, but lie between the extreme critical values of 10%, but the critical value for the NARDL (2.361283) is in between the extreme values of 5% and 10%, but below that of 1%. Based on the F-test, no cointegration exists for the Ghana ARDL at 1% and 5%, and the decision is inconclusive at 10%. Similarly, cointegration cannot be established for the Ghana NARDL at 1% and it is inconclusive at 5% and 10%. However, if an alternative method of testing for cointegration based on EquationEquation (3)(3)
(3) is utilized, cointegration is confirmed for the Ghana ARDL model because the error correction term (π) is negative and statistically significant. In summary, the Eurozone-bound export equation for each country has a long-run relationship with other regressors in the ARDL and NARDL equations at the conventional significance levels. Hence, the long-run relationship for the export equation can be estimated in the form of EquationEquations (4)
(4)
(4) and Equation(5)
(5)
(5) for ARDL and NARDL, respectively. Model Selection and Residual Diagnostics
aims to compare ARDL and NARDL with a view to choosing a better model. The measures used include information criteria (SIC), residual diagnostics (SC, H, N and AR) and stability measures (CQ and CS). Smaller SIC values imply that the respective model is more parsimonious than the other. As shown in the table, NARDL has a lower SIC value than ARDL for all the countries except Ghana. Thus, SIC chooses NARDL over ARDL for all the countries other than Ghana. However, estimation of the Ghana NARDL model indicates that at least one of the volatility measures is significant in the short run, as shown in . Hence, it will be more reasonable to model Ghana exports as NARDL instead of ARDL.
Table 3. Short run estimates of the NARDL.
The CUSUM test, as suggested by Brown et al. (Citation1975), is a good tool that provides an insight into the stability of the export parameters. The summary of the CUSUM (CQ) and CUSUM of Squares (CS) tests, which show the stability of the parameter and variance estimates, is presented in . If the CUSUM plot falls within the 5 per cent significance boundary, the model is stable, otherwise it is unstable. As indicated by the CQ, both ARDL and NARDL models for all the countries are stable, but the CS indicates that all the ARDL and NARDL models are stable for all the countries except Niger, Togo and Benin. However, CS shows that ARDL is unstable but NARDL is stable. Therefore, NARDL is more suitable for modelling the EEE.
Table 4. Model selection and diagnostic tests.
6. Estimation results
The estimation results for the NARDL are presented and explained in this section, with the aim of addressing the second research question, which tries to identify the factors that determine EEE for each country. As mentioned earlier, the dependent variable is the volume of exports from each country to the Eurozone, while the independent variables are the foreign income, foreign price and exchange rate volatility. If an independent variable is statistically significant, it implies that it is an important factor in determining the EEE for that country, and vice versa. and contain the long-run and short-run estimates for the NARDL, respectively. For both models, the maximum lag order is determined by the number of lags that whiten the error term in the unrestricted Vector Autoregressive (VAR) modelFootnote6. The order of the NARDL is determined automatically by AIC. Although SIC selects a more parsimonious model, it is more reasonable to work with AIC selection as it can reveal a better insight into the dynamics of the model. For Nigeria, significance is confirmed for all the long-run regressors at conventional levels of 1%, 5% and 10%. The significant long-run coefficient(s) is/are lnXt only for Ghana, lnXt and for Mali, lnXt and lnPt for Niger, lnXt and
for Senegal, Togo and Benin,
and
for Burkina Faso,
and
for Cote D’Ivoire. Nigeria and Burkina Faso are the only countries that record significant long-run coefficients for both
and
Mali and Cote D’Ivoire have a significant coefficient for
only. The coefficient of lnYt is only significant for Nigeria and Togo at 1%, Senegal and Benin at 5%; lnXt is significant for all the countries at 1%; and lnPt is significant for Nigeria, Niger and Burkina Faso only.
Table 5. Long-run estimates of the NARDL.
Looking at the short run coefficients from , it is clear that the positive volatility coefficients affect Senegal only and negative volatility coefficients exert influence on Ghana, Mali, Niger, Togo, Benin and Burkina Faso. The next step is to determine the countries that exhibit a short-term asymmetrical relationship between trade and volatility. From , it can be seen that Ghana, Senegal, Niger, Togo, and Benin have at least one statistically significant coefficient related to volatility measures. All of these countries display negative coefficients in line with theoretical expectations, except for Senegal’s and Benin’s
This suggests that traders in these countries may have different attitudes towards volatility. They may choose to increase exports to Eurozone when they anticipate a drop in volatility, but if the decreased volatility lasts for several months, their exports may fall since the higher lags of the short-term volatility measures have negative coefficients.
7. Discussion
The foregoing analysis indicates the suitability of modelling the export-volatility relationship as asymmetric rather than symmetric. The significance of all the long-run coefficients for Nigeria could be due to the fact that it is the largest Eurozone trade partner in the ECOWAS. As mentioned earlier, the expected signs of foreign income (Y), foreign price (P) are positive and that of volatility measures (V) is negative. The long-run coefficients of foreign price and positive volatility measures for Nigeria have the expected signs, but that of foreign income does not. The foreign income in the long run could be negatively related with Nigeria-Eurozone exports because the Eurozone is likely to find alternatives to Nigeria’s imports through support or import substitution programs as its income rises. Another explanation is that crude oil is part of the Nigerian exports to the Eurozone, and the Eurozone is shifting to the use of alternative sources of energy as its income rises. In addition to this, asymmetry of volatility effect is confirmed, as the impact of the negative volatility (0.058) is higher than that of positive volatility (-0.057). This implies that increased volatility tends to decrease exports from Nigeria to the Eurozone, while reduced volatility leads to a rise in the exports. However, as shown in , the short-run asymmetry cannot be established as only logarithmic change in current price () and its lags, and some lags of logarithmic change in exports (
) affect the logarithmic change in the Nigeria-Euro exports (
). Burkina Faso is another ECOWAS country that faces asymmetry of the impact of exchange volatility on its Eurozone-bound exports. As shown in , the impact of the negative volatility on Burkina-Faso-Eurozone exports (-0.273) is higher than that of positive volatility (-0.292). However, in absolute terms, the impact of positive volatility for Burkina Faso tend to be higher than that of negative volatility. In other words, increased volatility tends to reduce the exports of Burkina Faso more than the negative volatility. Moreover, the short-run coefficient of the negative volatility measure
for Burkina Faso, as shown in , is 5.11. The implication is that Burkina-Faso-Eurozone exports rise instantly by 5.11% as the exchange rate volatility drops by 1% and that exchange rate volatility affects the exports of Burkina Faso to Eurozone asymmetrically in both the short run and long run. The long-run asymmetry of trade-volatility relationship can also be established for Mali and Cote D’Ivoire, as shows that the coefficient of
is significant for the export equation of both countries. Exports increase with a decrease in volatility for Mali as the coefficient of
is positive, but the reverse is the case for Cote D’Ivoire. Asymmetry of trade-volatility relationship in the short run for Mali is also confirmed, as indicates that the coefficient of
is significant.
The next task is to identify the countries with short-run asymmetry of trade-volatility relationship only. As shown in , Ghana, Senegal, Niger, Togo and Benin have at least one significant coefficient of the volatility measure. All the short run volatility coefficients for these countries have the theoretically expected negative sign, except for Senegal and
for Benin. This could be explained by the country’s decision to increase exports to Eurozone whenever they anticipate a drop in volatility; however, the exports fall if the decreased volatility lasts for some months as the higher lags of the short run volatility measures have negative coefficients. This implies a different attitude to volatility by traders in different ECOWAS countries. To address the first research question in depth, long-run asymmetry is confirmed for Nigeria, Mali, Burkina Faso and Cote D’Ivoire, while short-run asymmetry is established for Mali, Burkina Faso, Ghana, Senegal, Niger, Togo and Benin. This implies that the last five countries have an asymmetric trade-volatility relationship in the short run only, while Mali and Burkina Faso are the only countries with an asymmetric trade-volatility relationship in both the short run and long run.
Besides the exchange rate volatility, other factors considered in this study are foreign income (Y) and foreign price (P). This section tries to address the second research question, which identifies the factors (foreign income, foreign price and volatility) that determine the EEE for each country. All these factors are important in determining the exports from Nigeria to the Eurozone in the long run, but only the Eurozone price is important in the short run. For Ghana, foreign prices, volatility and foreign income do not explain the changes in its export in the long run, but negative volatility and foreign income cause the changes in its import in the short run. For Mali, exports to the Eurozone respond only to decreased volatility and its own lag in the short run, but the exports only change with the changes in foreign price and a drop in volatility. For Senegal, Eurozone income is the only important factor in the long run, and foreign income, increased volatility and foreign price play an important role in determining the exports in the short run. For Togo, Eurozone income is the important determinant for export in the long run, and Eurozone income and reduced volatility influence its exports in the short run. For Benin, foreign income determines the exports in the long run, and foreign income, reduced volatility and foreign price are the main factors in the short run. For Burkina Faso, foreign price and volatility measures are the main factors in both the short run and long run. For Cote D’Ivoire, reduced volatility is the main factor in the long run and foreign income and price are the main determinants in the short run. In general, Senegal, Togo and Benin are coastal countries, which means that transportation to the Eurozone is comparatively cheaper; hence, Eurozone income is more important in determining their exports than foreign price in the long run. However, foreign price is more influential factor for Niger and Burkina Faso because these countries are landlocked and transportation to the Eurozone will be very expensive. Senegal has a positive long run coefficient of foreign income (Yt) and at least one of the short run coefficients of for Cote D’Ivoire and Benin contradicts the expected relationship. A negative coefficient for Eurozone income implies that the exports are dominated by goods and services that are inferior goods to the citizens of the Eurozone, while a positive coefficient indicates that the goods and services are normal goods. A positive coefficient of foreign price means the goods or services exported are substitutes, otherwise they are complementary or unrelated. The significant long-run coefficients of Nigeria and Burkina Faso are in line with the theoretical postulation. A negative coefficient for the exchange rate volatility indicates that the ECOWAS country is a risk taker, while a positive coefficient signifies risk aversion. For the long run, both negative and positive volatility is confirmed for Burkina Faso and Cote D’Ivoire.
To provide answer to the penultimate question regarding the nature of goods and services that constitute the EEE, the concepts of income elasticity and cross elasticity are used. Income elasticity can be used to explain the type of goods or services that constitute the exports from Nigeria, Senegal, Togo and Benin to the Eurozone, while cross elasticity will be suitable for explaining the type of goods or services exported from Niger and Burkina Faso to the Eurozone. To that end, the exports of Senegal in the long run can be said to constitute goods or services or a large share of goods or services that are necessity goods to the citizens of the Eurozone because, as shown in , the estimates of its income elasticity are positive and less than unity. The exports of Nigeria, Togo and Benin consist of goods and services or large share goods and services that are inferior as the estimates of their income elasticity is negative, while changes in foreign income leave the exports of the rest of the countries unaffected. The exports of Nigeria, Niger and Burkina Faso consist of substitute goods as the estimates of their cross elasticity are positive, indicating that their exports rise in the long run as the foreign price increase. The exports of goods and services from the other countries consist of neither complimentary nor substitute goods as the estimates of their cross elasticities are insignificant. With the help of , the same explanation can be used to classify the goods and services that constitute the exports from the ECOWAS countries to the Eurozone in the short run.
The last question attempts to establish the policy implications of the findings of these study. In general, a negative coefficient of the volatility measure requires the ECOWAS country to control its exchange rate movement by implementing policies such as a managed floating exchange rate regime. A positive coefficient of volatility augurs well for a purely floating exchange rate regime. Economic diversification would the best way for an ECOWAS country to address the negative coefficient of foreign income. If the coefficient of foreign price is positive, it implies that the government in the ECOWAS country needs to provide support or incentives for exports, otherwise its exports will not be able to compete with the goods and services in the Eurozone. However, ECOWAS should avoid implementing policies that damage the Eurozone. Hence, trade agreements such as EPA are very important.
8. Conclusion
This study attempts to establish the existence of the asymmetric impact of exchange rate volatility on exports from ECOWAS countries to the Eurozone. The measure of exchange rate volatility used for all the countries is IGARCH(1,1) as it is considered to be the most parsimonious model without redundant coefficients in both the mean and variance equations. After the bounds test confirms the existence of a long-run relationship for all the countries except Ghana, the ARDL and NARDL models were estimated, and the latter model happened to be better than the former for all the countries. Long-run asymmetry is confirmed for 3 countries (Nigeria, Mali, Burkina Faso and Cote D’Ivoire), while a short-run asymmetry is established for Mali, Burkina Faso, Ghana, Senegal, Niger, Togo and Benin. This implies that the last five (5) countries only have an asymmetric trade-volatility relationship in the short run, while Mali and Burkina Faso are the only countries with asymmetric trade-volatility relationships in both the short run and long run.
In addition to the asymmetry of volatility, other factors in the export equation are used to classify the kinds of goods and services that constitute/dominate the exports according to the estimates of foreign income and foreign price. Nigeria, Togo and Benin export inferior goods as the estimates of their income elasticities are negative, and Senegal’s exports consist of necessity goods as the estimates of its income elasticity lie between 0 and 1. On the other hand, Nigeria, Niger and Burkina Faso export substitute goods as the estimates of their cross elasticities are positive.
In order to raise their exports to the Eurozone, ECOWAS countries that face a negative (positive) impact of volatility on their exports are recommended to implement the exchange rate policies that will reduce (increase) the volatility of the exchange rate. A managed floating exchange rate regime reduces the volatility while it is increased by a purely floating exchange rate regime. On the other hand, for the Eurozone to control its imports from the ECOWAS, it should be able to persuade the countries to implement exchange rate policies that are favourable to the Eurozone. The persuasion can be in the form of incentives such as foreign aid, or signing trade agreements such as the EPA. The countries that export inferior goods and services are recommended to diversify their economies so that they can establish other source(s) of exports to the Eurozone. Governments are recommended to provide support for the exports that are substitutes to the Eurozone’s goods and services so that the price of their exports will be lower than the Eurozone price.
Although no study exists that has examined the asymmetric impact of exchange rate volatility on ECOWAS-Eurozone exports, this study is not exhaustive. It employs only one measure of exchange rate volatility, and does not consider imports from the Eurozone to ECOWAS as there are no available data on the industrial production index for the ECOWAS countries. Moreover, the study analyses the asymmetric impact of volatility on the economy as a whole, not on the individual sectors that make the whole. Therefore, this study suggests that future research should consider the inadequacies of the present study. For example, future studies can compare the results of the trade-volatility relationship between the major ECOWAS’s blocks of West African Economic and Monetary Union (WAEMU) and West African Monetary Zone (WAMZ).
Data accessibility
The data that support the findings of this study is openly available in the database of International Financial Statistics at the following addresses
– Consumer/producer price index: https://data.imf.org/regular.aspx?key=61545849
– Exchange rate: https://data.imf.org/regular.aspx?key=61545850
– Trade of goods: https://data.imf.org/regular.aspx?key=61545859
List of abbreviations | ||
ARDL | = | Autoregressive Distributed Lag model |
CPI | = | consumer price index |
ECOWAS | = | Economic Community of West African States |
EEE | = | ECOWAS-Eurozone exports |
EGARCH | = | Exponential Generalized Autoregressive Conditional Heteroskedastic |
GARCH | = | Generalized Autoregressive Conditional Heteroskedastic |
IGARCH | = | Integrated Generalised Autoregressive Conditional Heteroskedasticity |
NARDL | = | Nonlinear Autoregressive Distributed Lag model |
P | = | price |
TGARCH | = | Threshold Generalized Autoregressive Conditional Heteroskedastic |
V | = | volatility |
X | = | export |
Y | = | income |
Disclosure statement
No potential conflict of interest was reported by the author(s).
Notes
1 See this site for more details: http://www.ecowas.int/member-states/
2 Specifically
3 The mean equation for the GARCH model of each country is the best ARIMA model for the natural logarithm of R.
4 The unit root tests are not reported to save space
5 To save space, the correlograms are not reported.
6 The highest lag order indicated by the lag selection criteria is 5. However, we consider using a maximum of 7 lags for all the countries to avoid losing many degrees of freedom.
References
- Adom, D. A., Morshed, A. M., & Sharma, S. C. (2012). Sources of real exchange rate volatility in Africa: The case of ECOWAS. African Development Review, 24(1), 79–92. https://doi.org/10.1111/j.1467-8268.2012.00306.x
- Akalpler, E., Ozdeser, H., & Mati, S. (2017). Trade-volatility relationship in the light of Nigeria and the euro area. Journal of Applied Economic Sciences, 12(7).
- Akhtar, P., Ullah, S., Majeed, M. T., & Usman, A. (2022). Exchange rate volatility and trade flows of Pakistan with major trading partners: Is there an asymmetric third-country effect? Journal of International Commerce, Economics and Policy, 13(3). https://doi.org/10.1142/S1793993322500168
- Alamrouni, A., Aslanova, F., Mati, S., Maccido, H. S., Jibril, A. A., Usman, A. G., & Abba, S. I. (2022). Multi-regional modeling of cumulative COVID-19 cases integrated with environmental forest knowledge estimation: A deep learning ensemble approach. International Journal of Environmental Research and Public Health, 19(2), 738. https://doi.org/10.3390/ijerph19020738
- Aqeel, A., & Nishat, M. (2006). The effect of exchange rate volatility on Pakistan’s exports. Pakistan Economic and Social Review, 44(1), 81–92.
- Arize, A. C., Malindretos, J., & Igwe, E. U. (2017). Do exchange rate changes improve the trade balance: An asymmetric nonlinear cointegration approach. International Review of Economics & Finance, 49, 313–326. https://doi.org/10.1016/j.iref.2017.02.007
- Asteriou, D., Masatci, K., & Pılbeam, K. (2016). Exchange rate volatility and international trade: International evidence from the MINT countries. Economic Modelling, 58, 133–140. https://doi.org/10.1016/j.econmod.2016.05.006
- Baak, S. (2004). Exchange rate volatility and trade among the Asia Pacific countries. East Asian Economic Review, 8(1), 93–115. https://doi.org/10.2139/ssrn.3079180
- Bacchetta, P., & van Wincoop, E. (2005). A theory of the currency denomination of international trade. Journal of International Economics, 67(2), 295–319. https://doi.org/10.1016/j.jinteco.2004.01.006
- Bahmani-Oskooee, M., & Aftab, M. (2017). On the asymmetric effects of exchange rate volatility on trade flows: New evidence from US-Malaysia trade at the industry level. Economic Modelling, 63, 86–103. https://doi.org/10.1016/j.econmod.2017.02.004
- Bahmani-Oskooee, M., & Arize, A. C. (2020). On the asymmetric effects of exchange rate volatility on trade flows: Evidence from Africa. Emerging Markets Finance and Trade, 56(4), 913–939. https://doi.org/10.1080/1540496X.2018.1543582
- Bahmani-Oskooee, M., & Arize, A. C. (2022). The effect of exchange rate volatility on U.S. bilateral trade with Africa: A symmetric and asymmetric analysis. Economic Systems, 46(1), 100879. https://doi.org/10.1016/j.ecosys.2021.100879
- Bahmani-Oskooee, M., & Baek, J. (2021). On the asymmetric effects of exchange-rate volatility on trade flows: Evidence from Korea-U.S. commodity trade. Australian Economic Papers, 60(4), 594–629. https://doi.org/10.1111/1467-8454.12221
- Bahmani-Oskooee, M., & Hegerty, S. W. (2007). Exchange rate volatility and trade flows: A review article. Journal of Economic Studies, 34(3), 211–255. https://doi.org/10.1108/01443580710772777
- Bahmani-Oskooee, M., & Karamelikli, H. (2022a). Exchange rate volatility and commodity trade between U.K. and China: An asymmetric analysis. The Chinese Economy, 55(1), 41–65. https://doi.org/10.1080/10971475.2021.1892919
- Bahmani-Oskooee, M., & Karamelikli, H. (2022b). U.K.-German commodity trade and exchange-rate volatility: An asymmetric analysis. The International Trade Journal, 36(4), 288–305. https://doi.org/10.1080/08853908.2021.1947923
- Bahmani-Oskooee, M., Karamelikli, H., & Niroomand, F. (2022). Asymmetric effects of exchange rate volatility on trade flows: Evidence from G7. Journal of Economics and Finance, 47(1), 38–62. https://doi.org/10.1007/s12197-022-09597-5
- Baier, S. L., & Bergstrand, J. H. (2007). Do free trade agreements actually increase members’ international trade? Journal of International Economics, 71(1), 72–95. https://doi.org/10.1016/j.jinteco.2006.02.005
- Bollerslev, T. (1986). Generalized autoregressive conditional heteroskedasticity. Journal of Econometrics, 31(3), 307–327. https://doi.org/10.1016/0304-4076(86)90063-1
- Broll, U., & Eckwert, B. (1999). Exchange rate volatility and international trade. Southern Economic Journal, 66(1), 178–185. http://www.jstor.org/stable/1060843 https://doi.org/10.2307/1060843
- Brown, R. L., Durbin, J., & Evans, J. M. (1975). Techniques for testing the constancy of regression relationships over time. Journal of the Royal Statistical Society: Series B (Methodological), 37(2), 149–163. https://doi.org/10.1111/j.2517-6161.1975.tb01532.x
- Chang, B. H., Bhutto, N. A., Ahmed, F., Abro, Z., & Anjum, N. (2021). The effect of the global financial crisis on the asymmetric relationship between exchange rate volatility and trade flows. Studies of Applied Economics, 39(2) https://doi.org/10.25115/eea.v39i2.4085
- Chowdhury, A. (1993). Does exchange rate variability depress trade flows? Evidence from error correction models. Review of Economics and Statistics, 75(4).
- Clark, P. B., Wei, S.-J., Tamirisa, N. T., Sadikov, A. M., & Zeng, L. (2004). A new look at exchange rate volatility and trade flows. In A new look at exchange rate volatility and trade flows. International Monetary Fund.
- Devereux, M. B., & Engel, C. (2002). Exchange rate pass-through, exchange rate volatility, and exchange rate disconnect. Journal of Monetary Economics, 49(5), 913–940. https://doi.org/10.1016/S0304-3932(02)00130-7
- Dickey, D. A., & Fuller, W. A. (1979). Distribution of the estimators for autoregressive time series with a unit root. Journal of the American Statistical Association, 74(366a), 427–431. https://doi.org/10.1080/01621459.1979.10482531
- ECOWAS. (2017). The implementation of the ECOWAS monetary cooperation programme. ECOWAS.
- Enders, W. (2015). Applied econometric time series (4th ed.). Jhon Wiley & Sons.
- Engle, R. (1982). Autoregressive conditional heteroscedasticity with estimates of the variance of united kingrom inflation. Econometrica, 50(4), 987. https://ci.nii.ac.jp/naid/10020819931/en/ https://doi.org/10.2307/1912773
- Fidora, M., Giordano, C., & Schmitz, M. (2020). Real exchange rate misalignments in the euro area. Open Economies Review, 32(1), 71–107. https://doi.org/10.1007/s11079-020-09596-1
- Hashmi, S. M., Chang, B. H., & Shahbaz, M. (2021). Asymmetric effect of exchange rate volatility on India’s cross-border trade: Evidence from global financial crisis and multiple threshold nonlinear autoregressive distributed lag model. Australian Economic Papers, 60(1), 64–97. https://doi.org/10.1111/1467-8454.12194
- Hayakawa, K., & Kimura, F. (2009). The effect of exchange rate volatility on international trade in East Asia. Journal of the Japanese and International Economies, 23(4), 395–406. https://doi.org/10.1016/j.jjie.2009.07.001
- Helpman, E., & Krugman, P. (1989). Trade policy and market structure. MIT press.
- Hooper, P., & Kohlhagen, S. W. (1978). The effect of exchange rate uncertainty on the prices and volume of international trade. Journal of International Economics, 8(4), 483–511. https://doi.org/10.1016/0022-1996(87)90001-8
- Hooy, C.-W., Siong-Hook, L., & Tze-Haw, C. (2015). The impact of the Renminbi real exchange rate on ASEAN disaggregated exports to China. Economic Modelling, 47, 253–259. https://doi.org/10.1016/j.econmod.2015.02.025
- Iqbal, J., Aziz, S., & Nosheen, M. (2022). The asymmetric effects of exchange rate volatility on US–Pakistan trade flows: New evidence from nonlinear ARDL approach. Economic Change and Restructuring, 55(1), 225–255. https://doi.org/10.1007/s10644-020-09310-8
- Krugman, P. (1983). Oil shocks and exchange rate dynamics. In Exchange rates and international macroeconomics (pp. 259–284). University of Chicago Press.
- Lee, C.-H., Li, S.-H., & Lee, J.-Y. (2022a). An asymmetric impact analysis of the exchange rate volatility on commodity trade between the U.S. and China. International Review of Economics & Finance, 82, 399–415. https://doi.org/10.1016/j.iref.2022.07.002
- Lee, C.-H., Li, S.-H., & Lee, J.-Y. (2022b). Examining the asymmetric effects of third country exchange rate volatility on trade between the US and the EU. Journal of Risk and Financial Management, 15(8), 321. https://doi.org/10.3390/jrfm15080321
- Mati, S. (2021). Do as your neighbours do? Assessing the impact of lockdown and reopening on the active COVID-19 cases in Nigeria. Social Science & Medicine (1982), 270, 113645. https://doi.org/10.1016/j.socscimed.2020.113645
- Mati, S., Civcir, I., & Abba, S. I. (2023). EviewsR: An R package for dynamic and reproducible research using EViews, R, R markdown and Quarto. The R Journal, 15(2), 169–205. https://doi.org/10.32614/RJ-2023-045
- Mati, S., Civcir, I., & Ozdeser, H. (2019). ECOWAS common currency: How prepared are its members? Investigación Económica, 78(308), 89. https://doi.org/10.22201/fe.01851667p.2019.308.69625
- Mati, S., Civcir, I., & Özdeşer, H. (2023). ECOWAS common currency, a mirage or possibility? Panoeconomicus, 70(2), 239–260. https://doi.org/10.2298/PAN191119015M
- Mati, S. (2020). EviewsR: A seamless integration of EViews and R. https://CRAN.R-project.org/package=EviewsR
- Mckenzie, M. D. (1998). The impact of exchange rate volatility on Australian trade flows. Journal of International Financial Markets, Institutions and Money, 8(1), 21–38. https://doi.org/10.1016/S1042-4431(98)00022-5
- Nelson, D. B. (1990). Stationarity and persistence in the GARCH(1,1) model. Econometric Theory, 6(3), 318–334. https://doi.org/10.1017/S0266466600005296
- Pesaran, M. H., Shin, Y., & Smith, R. J. (2001). Bounds testing approaches to the analysis of level relationships. Journal of Applied Econometrics, 16(3), 289–326. https://doi.org/10.1002/jae.616
- Phillips, P. C. B., & Perron, P. (1988). Testing for a unit root in time series regression. Biometrika, 75(2), 335–346. https://doi.org/10.1093/biomet/75.2.335
- Ribeiro, R. S. M., McCombie, J. S. L., & Lima, G. T. (2020). Does real exchange rate undervaluation really promote economic growth? Structural Change and Economic Dynamics, 52, 408–417. https://doi.org/10.1016/j.strueco.2019.02.005
- Rose, A. K., & Yellen, J. L. (1989). Is there a j-curve? Journal of Monetary Economics, 24(1), 53–68. https://doi.org/10.1016/0304-3932(89)90016-0
- Shin, Y., Yu, B., & Greenwood-Nimmo, M. (2011). Modelling asymmetric cointegration and dynamic multipliers in a nonlinear ARDL framework. Festschrift in Honor of Peter Schmidt, W.C. Horrace and R.C. Sickles. SSRN. http://dx.doi.org/10.2139/ssrn.1807745.
- Shin, Y., Yu, B., & Greenwood-Nimmo, M. (2014). Modelling asymmetric cointegration and dynamic multipliers in a nonlinear ARDL framework. In W. Horrace & R. Sickles (Eds.), The festschrift in honor of peter schmidt (pp. 281–314). Springer. https://doi.org/10.1007/978-1-4899-8008-3__9
- Usman, A., Apergis, N., & Anwar, S. (2021). Examining the asymmetric effects of third-country exchange rate volatility on Pakistan-China commodity trade. Journal of International Commerce, Economics and Policy, 12(02), 2150008. https://doi.org/10.1142/S1793993321500083
- Zorlubas, C. (2011). Exchange rate fluctuations and the trade balance of Norway: An econometric analysis. California State University.