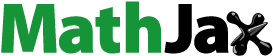
Abstract
The dynamics of international trade play a pivotal role in shaping economic growth and development for nations worldwide. This significance is particularly pronounced in the context of India’s agrarian economy. With a substantial portion of its population dependent on agriculture, engaging in global trade presents a myriad of advantages. As a member of the World Trade Organization (WTO), India benefits from a framework that fosters transparent and fair-trade relations, enabling dispute resolution and favourable negotiations. India’s agro-climatic diversity grants it a competitive edge in cultivating various agricultural products, predominantly rice. Enabling policies, like Minimum Support Price and subsidies, incentivize farmers, while adherence to international quality standards enhances the acceptance of Indian rice abroad. The gravity model employed in this study to analyze India’s rice trade with major importing countries, offers valuable insights into trade dynamics. When delving into specific determinants of trade, the Heckman selection equation proves useful. Factors like Gross Domestic Product (GDP), per capita income, trade history, and exchange rates consistently impact partner selection. The likelihood of choosing a trading partner is influenced by economic compatibility, historical trade relations and WTO membership. Additionally, shared borders and regional affiliations play a role, while economic recessions tend to decrease partner selection due to reduced demand. Examining trade quantity reveals nuanced dynamics. Historical trade interactions, economic indicators and WTO membership consistently influence trade volumes. Larger GDPs, per capita incomes, and populations of trading partners enhance trade prospects, while disparities in income and exchange rate fluctuations impact trade negatively. Importantly, distance remains a key factor affecting trade volume, as logistical complexities and transportation costs influence trade decisions. These findings shed light on trade dynamics, enabling evidence-based policy decisions to enhance trade relationships, boost competitiveness and propel India’s rice exports to new heights.
REVIEWING EDITOR:
1. Introduction
International trade serves as a crucial driver of economic growth and development, extending a nation’s reach beyond its domestic borders. This engagement with other countries enables access to a wider customer base, resulting in expanded production and economies of scale. This, in turn, fosters economic advancement. Moreover, international trade’s encouragement of specialization allows countries to focus on areas where they hold a comparative advantage, leading to efficient resource allocation, heightened productivity, and global economic benefits. One prominent advantage lies in the enhanced consumer choices made possible through importing goods and services that might be absent or more affordable elsewhere. This diversity of offerings elevates living standards and consumer satisfaction. Furthermore, international trade’s role in job creation cannot be understated. Export-driven industries often experience employment growth as they cater to international markets, thereby reducing unemployment rates and elevating incomes. Beyond economic ramifications, international trade facilitates the exchange of knowledge, technology, and best practices between nations. This interchange nurtures innovation and progress, propelling global development through the adoption of novel methods and technologies. This dynamic exchange of ideas accelerates overall societal advancement (Saxena et al., Citation2023). The significance of international trade extends to diplomatic and political arenas. It fosters robust relationships between countries, fostering peaceful cooperation and creating avenues for future collaboration. This dialogue-driven interaction cultivates mutual understanding and decreases the likelihood of conflicts. Additionally, the diversification of goods and services sources mitigates dependency on a single market or supplier, offering resilience against supply chain disruptions and domestic demand fluctuations. This attribute is particularly valuable during economic uncertainties (Anh Lan Thi et al., Citation2022).
In the context of India’s substantial agrarian economy, international trade of agricultural commodities holds immense significance. With a significant portion of population (58%) relying on agriculture for livelihood, engaging in global trade offers numerous advantages (Agricultural Statistics at a Glance, Citation2021). It grants access to larger markets, facilitates diversification, and introduces modern technology and techniques. This, in turn, stimulates economic growth, reduces poverty, and elevates living standards in rural areas. By participating in international trade as a member of the World Trade Organization (WTO), India benefits from a framework of rules that promote fair, transparent, and predictable trade relations. This involvement strengthens India’s ability to resolve disputes, negotiate favorable agreements and safeguard its interests in the global agricultural market (Khalid et al., Citation2020). Engaging in international trade empowers India’s agricultural sector by providing access to global markets, extending opportunities for farmers and businesses beyond national borders, increase foreign exchange earnings, facilitate exchange of knowledge, technology, and best practices etc. So, the international trade of agricultural commodities, especially rice, provides India with a pathway to economic prosperity, technological advancement, and cooperative global engagement, while simultaneously benefiting the global agricultural landscape (Kumar, Citation2019; Nguyen, Citation2022; Nikolche & Usama, Citation2022; Sreevidhya & Elango, Citation2019).
India’s diverse agro-climatic zones provide a fertile ground for cultivating a wide range of agricultural products. The availability of vast land resources enables the country to produce staple crops like rice and wheat, meeting the substantial domestic demand, while also having surplus quantities available for export. Additionally, India can cultivate cash crops such as cotton, sugarcane, spices, and others, further contributing to its competitive edge in international market. India’s rich biodiversity and traditional knowledge of agricultural practices further bolster its comparative advantage. Low production cost is another key factor that contributes for India’s competitiveness in global agricultural market. Labor costs in India is comparatively lower than in many other countries, enabling cost-effective agricultural production. Access to affordable agricultural inputs also plays a crucial role in keeping production costs competitive. India’s farming community is large and skilled, with extensive experience in agriculture. This skilled workforce contributes to efficient farm management, timely harvesting, and post-harvest handling, ensuring a steady supply of high-quality agricultural produce for export. The Government of India’s favourable policies and initiatives further support the agricultural sector and boost agricultural exports. Schemes like the Minimum Support Price (MSP) and agricultural subsidies incentivize farmers, encouraging them to increase productivity and enhance their competitiveness in global markets. In addition to traditional strengths, India has made significant strides in adhering to international quality and safety standards for agricultural produce. This compliance enhances acceptance of Indian agricultural commodities in international markets, instilling confidence in buyers regarding the safety and quality of the products. The government actively promotes agricultural exports through various export promotion schemes, incentives, and diplomatic efforts. This support facilitates market access with other countries, fostering India’s position as a reliable supplier of agricultural commodities (Gupta & Kumar, Citation2020; Nguyen et al., Citation2020; Nugroho et al., Citation2021).
The application of the gravity model to analyze India’s international trade of rice with its major importing countries serves as a robust analytical tool to unravel the intricate dynamics that govern these trade relationships. This model, widely used in international economics, considers factors such as economic sizes of countries and distance between them, providing a systematic framework to comprehend trade patterns and devise policies accordingly. The strategic selection of Bangladesh, Benin, China, Ivory Coast, Iran, Iraq, Nepal, Saudi Arabia, Senegal, and Vietnam () as the focal points of this analysis stems from their collective contribution of 54% to India’s total rice exports (www.fao.org). This subset’s substantial representation emphasizes its significance in India’s trade portfolio and signals its influential role in shaping country’s rice export landscape. This specific group of countries mirrors a diverse set of market conditions, economic statuses, and geographical locations, amplifying the complexity of India’s trade interactions and making it an ideal cohort for analysis. This focused analysis offers insights into the core drivers of India’s rice exports, their economic impact, and market trends. It enables policymakers to tailor strategies that align with specific demands, enhance market penetration, and diversify offerings. Moreover, the examination identifies potential risks arising from concentrated dependence, aiding in the development of risk mitigation measures and market diversification strategies. The gravity model’s insights guide evidence-based trade policy formulation, foster sustainable trade relations, and bolster India’s global competitiveness, positioning it to seize emerging trade opportunities (Adhikari et al., Citation2016; Nazir et al., Citation2021; Suman et al., Citation2022). Ultimately, this study is crucial for shaping effective trade strategies, optimizing market access, and sustaining India’s role as a reliable and substantial participant in the global rice trade landscape, with potential to reshape policies, drive economic growth and foster lasting trade partnerships.
2. Review of literature
Moustafa Ismaiel et al. (Citation2023) undertook an examination of the determinants shaping Egypt’s overall trade dynamics and, more specifically, its rice trade with 11 rice-importing partner nations: Libya, Lebanon, Syria, Saudi Arabia, Sudan, Jordan, Turkey, the United Kingdom, Ukraine, Belgium and Romania. Delving into the 2001 to 2016 rice crop data with the aforementioned trading partners, the research yielded noteworthy insights through employing Gravity model. Among its key findings, the study identified that Egypt’s Gross Domestic Product (GDP) variable negatively impacted the total value of imports while positively contributing to total exports, agricultural exports, and rice exports. Egyptian imports, exports, and population growth are found adversely affected by Egyptian population. The study also recognized that higher transportation costs led to diminished trade volumes for both imports and exports, in line with economic theory. Additionally, the variable representing the distance between capitals exhibited a negative effect on Egyptian exports. This study advocated for the enhancement of economic relations between Egypt and its trading partners, urging a shift from mere import-export collaboration toward strategic cooperation aimed at bolstering food security.
Amjad et al. (Citation2021) in their study analyzed the influence of the China-Pakistan Regional Trade Agreement on Pakistan’s export performance through employing gravity trade model utilizing Poisson Pseudo Maximum Likelihood (PPML) estimator for export data spanning a 16-year period from 2003 to 2018. The analysis focuses on ten specific products categorized at the HS-4 level, ensuring a comprehensive evaluation of the trade dynamics. The findings showed that the traditional variables associated with the gravity equation, such as GDP, bilateral distance, colonial relationship, language commonality, and landlocked importing countries demonstrate a statistically significant and positive impact of the China-Pakistan Regional Trade Agreement on Pakistan’s exports. This finding underscores the substantive value of the trade agreement in bolstering the country’s export activities and highlights its effectiveness in promoting international trade interactions.
Nazir et al. (Citation2021) uncovered the primary determinants influencing Nigerian cocoa exports, utilizing the gravity model as its analytical framework. Employing three distinct approaches—Heckman selection models, Generalized Least Squares (GLS), and PPML—the research addresses challenges including multi-collinearity, serial correlation, heteroscedasticity, and zero trade. Drawing on panel data spanning 24 years (1995 to 2018) encompassing 36 significant cocoa importing partners, this study inferred that the EU countries, including the Netherlands, Germany, and France, along with the USA, Canada and Malaysia emerge as pivotal markets for Nigerian cocoa exports. Furthermore, GDP, WTO membership, EU involvement and shared colonial history exhibit a positive correlation with Nigerian cocoa export flows. The study also highlights the favorable impact of exchange rate policies. Conversely, per capita GDP, distance, landlocked status, African Union, ECOWAS and Asian markets are identified as negative factors affecting Nigerian cocoa export flows. So, to bolster cocoa export revenue, a gradual devaluation of the Naira, coupled with an export policy that encourages cocoa production, is advised.
In their study, Sokvibol et al. (Citation2019) utilized a dynamic gravity framework and employed GLS, PPML and Heckman Sample Selection models to analyze the essential determinants influencing the export of Cambodian rice using a comprehensive 22-year panel data set spanning 1995 to 2016 and encompassing 40 selected importing partners. The findings revealed that historical ties, exchange rate policies, and agricultural land reforms significantly stimulate rice exports. Notably, economic recession poses a challenge, constraining export flows and demanding targeted attention. Exchange rate policies and agricultural land expansion are identified as core driving forces behind the promotion of rice exports.
Irshad et al. (Citation2018a) employed PPML estimator to study determinants for rice exports from Pakistan across 144 importing countries spanning 2003 to 2016. The findings established that GDP of Pakistan and its trading partners, per capita differences, distance, bilateral exchange rates, contiguity, WTO membership, and trade agreements showed positive association with rice exports. However, bilateral exchange rates were discovered to have a negative impact, implying that appreciation of the Pakistani currency could decrease rice exports. Trade agreements had no significant influence on rice exports. The study cautioned that Pakistan should focus on addressing challenges such as high taxation, production costs, insufficient infrastructure, and electricity shortages hindered Pakistani rice producers and exporters.
Shaiara and Shanjida (Citation2015) conducted a comprehensive analysis of the gravity model for rice exports, revealing noteworthy findings. The coefficients associated with the distance variable were found to be statistically significant exhibiting the anticipated negative sign. Notably, the random effects estimations, both one-way and two-way, yielded larger coefficients (−2.19 and −2.18%), emphasizing the amplification of trade distance elasticity when accounting for country-specific heterogeneity in comparison to the pooled Ordinary Least Squares (OLS) estimate. The economic mass, represented by the product of the GDP of countries, displayed a positive correlation with trade, aligning with expectations. However, the two-way fixed effects estimate of the GDP variable presented an unexpected negative sign. Per capita GDP variables consistently exhibited significance at the one percent level with positive signs across both one-way and two-way fixed effects estimation methods. This consistency indicates that an escalation in the economic development of the combined entity defined by the two countries results in a more than proportional increase in trade volume. The R2 values for both one-way and two-way fixed effects estimations stood at 0.84, surpassing the R2 values derived from pooled OLS and random effects estimations. This underscores the importance of considering country-specific heterogeneity when estimating trade flows and highlights the robustness of fixed effects models in effectively capturing the determinants of rice exports in the gravity model.
Numerous other studies also harnessed Gravity model approach viz., Thorbecke (Citation2015) for China’s exports; Irshad and Xin (Citation2017) for South Korea’s international trade dynamics; Irshad et al. (Citation2018b) on China’s trade interactions with OPEC member countries, etc., and they underscored both versatility and applicability of this model in analyzing diverse trade relationships and uncovering the multifaceted dynamics that govern international trade patterns. Building upon the insights from previous reviews, this study aimed to meticulously examine the pivotal determinants that exert a substantial influence on performance of India’s rice agricultural exports within the global market. This investigation will be conducted through the utilization of a dynamic panel gravity model. The central focus is to pinpoint and comprehend fundamental factors that play a significant role in shaping export performance of Indian rice. By achieving this objective, the study seeks to make a noteworthy contribution to the existing body of literature concerning India’s agricultural trade, with a specific emphasis on its rice exports. The significance of this research is underscored by a multitude of compelling factors. Firstly, it enriches the existing literature by extending application of commodity-specific gravity model to agricultural trade. Several studies explored export determinants of individual products, such as rice (Cosslett & Cosslett, Citation2018; Thuong, Citation2018), soybean (Boerema et al., Citation2016; Wang, Citation2016), tea (Hwang & Lim, Citation2017), poultry (Zhou et al., Citation2019), egg (Tamini et al., Citation2016), sea-food (Natale et al., Citation2015), beef (Ghazalian et al., Citation2012; Schierhorn et al., Citation2016), sheep meat (Lee & Tcha, Citation2005), wine (Castillo et al., Citation2016; Dal Bianco et al., Citation2016; Dascal et al., Citation2002) and honey (Wei et al., Citation2012), this research contributes by broadening the scope to encompass multiple determinants within a single study. Secondly, this research stands out by integrating various analytical approaches—namely GLS, PPML, Heckman selection models and one-way and two-way Fixed effects model—in a singular investigation (Anderson & Wincoop, Citation2004; Baier & Bergstrand, Citation2007; Baldwin & Taglioni, Citation2006; Jemal & Tekilu, Citation2021; Rose & Van Wincoop, Citation2001). While these methods have been previously employed individually in trade literature, their concurrent application in this study enhances the analysis by providing increased depth and precision. The study’s innovative approach aligns with the diverse nature of trade dynamics, ensuring a more comprehensive assessment of the determinants affecting Indian rice exports. Thirdly, the research addresses a critical gap in the literature by examining the determinants of Indian rice exports across a 27-year period (1995–2023), involving a comprehensive dataset encompassing a total of 10 countries. By expanding the coverage of both time and countries, the study enhances the robustness and applicability of its findings. This temporal and geographical breadth enables a nuanced understanding of the evolving trade dynamics and the factors that have shaped India’s rice export performance.
3. Methodology
3.1. Sample size and data sources
In examining the determinants of Indian rice exports, we propose adoption of a dynamic panel commodity-specific gravity model. This methodological choice is underpinned by the recognized benefits of panel data in addressing biases inherent in cross-sectional and time-series estimations, as underscored in the works of notable economists (Baltagi et al., Citation2018; Matyas, Citation2017; Shepherd, Citation2019). Encompassing the period from 1995 to 2021, India’s rice trade network extended its reach to engage with the top 10 rice-importing nations globally, namely Bangladesh, Benin, China, Ivory Coast, Iran, Iraq, Nepal, Saudi Arabia, Senegal, and Vietnam () are purposively selected for this study. The geographic distribution of these interactions is visually represented on the global stage in . Notably, the cumulative rice exports from India to these 10 nations contribute significantly, comprising 54% of India’s overall rice exports (www.fao.org). The intentional inclusion of this select subset accentuates its paramount significance within India’s expansive trade portfolio, indicating a pivotal role in sculpting the nation’s rice export landscape. Consequently, this study meticulously canters on India and its prominent 10 rice-importing partners over a comprehensive 27-year span. The choice of these 10 nations stems from their mean annual rice import volumes, ensuring a robust and meaningful dataset. Critically, the rice exports to these key destinations during the specified timeframe constituted approximately 55 percent of India’s total rice export quantity, resulting in a thorough dataset comprising a total of 270 observations (N = 10 * T = 27). Elaborate information regarding the data sources for the employed variables is provided in . The estimation of parameters was carried out using Stata version 17.0 software package, ensuring a rigorous analytical framework.
Table 1. Description and expected sign of empirical models’ independent variables.
3.2. Techniques employed
3.2.1. Panel cross-section dependence (CD) test
Cross-section dependence in macro panel data has gained attention in panel time series literature. This correlation result from global shocks impacting countries differently (say, 2008 global economic recession), or local spillover effects (Eberhardt & Teal, Citation2010; Moscone & Tosetti, Citation2009). Prior to estimating the gravity equation, a CD test is crucial to detect cross-sectional dependence. Neglecting this test, following Breusch and Pagan (Citation1980) and Pesaran (Citation2004) may bias gravity results. We employ three tests—Pesaran’s residual CD test (EquationEq. 1(1)
(1) ), Friedman test (EquationEq. 2
(2)
(2) ), and Frees test (EquationEq. 3
(3)
(3) )—aligned with temporal aspects (Rafael & Vasilis, Citation2006). CD tests apply to temporal variables, excluding time-invariant ones (Rasoulinezhad, Citation2017; Rasoulinezhad & Kang, Citation2016). Notably, zero values and dummies are excluded from CD tests, considered across the sample.
(1)
(1)
(2)
(2)
(3)
(3)
The null hypothesis (Ho) in the above tests states that there is no cross-sectional dependence present in the panel data model. In other words, the residuals (errors) of the model are independent across different entities, and any observed correlations or dependencies are due to random chance. So, if the p-value associated with the above test statistics is sufficiently smaller than 0.05, then there is evidence to reject the null hypothesis and accept alternative hypothesis indicating the presence of cross-sectional dependence in the panel data model.
3.2.2. Trade-related Indices
The utilized trade-related indices in this study are outlined below (Alka & Harpreet, Citation2023).
i. Trade Intensity index (TII): This index serves as a metric to elucidate a country’s global trade importance. Mathematically derived, this index quantifies a nation’s significance in the world trade arena by the following formula:
(4)
(4)
where,
and
are the India’s exports and world’s exports of rice to trading partners ‘j’ (trade partners);
and
are total exports of rice from India and world respectively. TII is notably influenced by a combination of factors including politico-historical ties, economic complementarity, and geographical proximity.
ii. Export Intensity index (EII): This index for India is given by:
(5)
(5)
where,
= India’s export of rice to trading partners;
= Total India exports of rice;
= Total trading partners import of rice;
= World’s imports of rice;
= Total India imports of rice.
iii. Import Intensity index: This index for India with trading partners is given by:
(6)
(6)
where,
= India’s import of rice from trading partners;
= Total India imports of rice;
= Total export of rice from trading partners;
= World’s exports of rice;
= Total India exports of rice.
3.2.3. Gravity model
This model posits that, bilateral trade between countries is directly correlated with product of their economic sizes (GDPs) and inversely correlated with the distance between them. The origin of this model can be traced back to the pioneering research of Tinbergen (Citation1963), Poyhonen, (1963) and Chaney (Citation2008, Citation2018). This economic relationship is encapsulated in the following mathematical expression:
where,
is the flow of exports into country ‘j’ from country ‘
’,
and
are country ‘
’s and country ‘j’s GDPs and
is the geographical distance between the countries’ capitals.
Building upon this foundational concept, the dynamic gravity framework was applied through a combination of analytical models, namely the GLS, PPML and Heckman Sample Selection models, as elucidated below.
i. GLS approach: This approach to analyze dynamic gravity model of Indian rice exports takes the following form:
(7)
(7)
where, the log(.) is logarithm form. The detail description and expected sign of variables are given in . ‘α’ denotes the intercept term, while
s are estimated coefficients, and
is the statistical error term.
ii. “zeros” trade problem: The issue of "Zeros" in trade values poses a challenge when dealing with the logarithmic form of the gravity equation, as the logarithm of ‘0’ is undefined (Sokvibol et al., Citation2019). This is particularly relevant when some country pairs do not engage in trade during specific time periods. To address this concern, we adopted the PPML method (Santos Silva & Tenreyro, Citation2006), along with the Heckman Sample Selection model (Heckman, Citation1979). These two approaches are widely used in gravity model to effectively handle the "Zeros" trade problem.
a. PPML approach: As outlined by Santos Silva and Tenreyro (Citation2006), in this approach, the dependent variable considered is ‘’ and not ‘log(
)’ to effectively address the "Zeros" trade problem. This model is formulated as below.
(8)
(8)
b. Heckman Sample Selection model: This consists of two different equations, namely, sample selection equation and outcome equation. The sample selection equation take form as follows:
(9)
(9)
In the above equation, the latent variable ‘’ is not directly observable; however, the trade occurrence between India (exporter) and trade partner pairs is observed, indicating whether trade takes place (
= 1) or not (
= 0). This binary outcome reflects the presence or absence of bilateral trade between India and importing countries. Additionally,
represents a vector of variables influencing
The error term,
, introduces randomness in the model. The detailed expression for EquationEq. (9)
(9)
(9) is presented below:
(10)
(10)
However, there are some more variables that might affect the outcome equation. So, the study has also added some dummies to find out impact of other dummy variables like
and
on rice exports (Nazir et al., Citation2021). So, the outcome equation of Heckman selection model takes the same form as the gravity model in EquationEq. (7)
(7)
(7) , as shown below:
(11)
(11)
c. Fixed effects model: Further, in this study, fixed effect models, including both one-way and two-way fixed effects, are employed to provide a robust solution for controlling unobserved factors that could lead to biased estimates in GLS or PPML or Heckman selection models. In the realm of rice exports, factors such as trade policies, infrastructure, and governance that vary across countries can be adequately addressed by these fixed effects, ensuring a more accurate estimation of gravity model parameters. Moreover, the two-way fixed effect model allows for the control of both time-invariant and time-variant unobserved heterogeneity. This added flexibility is beneficial when dealing with dynamic changes in factors affecting rice exports over time. In contrast, GLS might not effectively handle unobserved heterogeneity, and Heckman selection models, designed to address sample selection biases, might not be the primary concern in the context of rice exports.
In a one-way fixed effects model, variation along one dimension (countries) is captured by introducing dummy variables, assuming that unobserved factors are constant across time. This is suitable when there are entity-specific effects that remain consistent over the observation period. On the other hand, a two-way fixed effects model extends this concept to account for variations along two dimensions – countries and time (Jemal & Tekilu, Citation2021; Shaiara & Shanjida, Citation2015). It includes both country-specific and time-specific dummy variables, accommodating changes in unobserved factors over time. Both models offer a robust approach to control for unobserved effects and enhance the accuracy of regression analyses in panel datasets.
For the one-way fixed effects model:
(12)
(12)
Here, represents country-specific fixed effects, β is the vector of coefficients, and
is the error term.
For the two-way fixed effects model:
(13)
(13)
In this equation, captures country-specific fixed effects,
represents time-specific fixed effects, β is the vector of coefficients, and
is the error term.
These models allow for the inclusion of fixed effects to control for unobserved heterogeneity across entities and/or time, providing a more accurate representation of the relationships within panel data.
As the selection of appropriate explanatory variables poses a challenging task, this study has adhered to trade theories and precedent empirical investigations to guide the identification of pertinent variables for shaping the empirical gravity model’s specification. This approach underscores the significance of incorporating both economic reasoning and empirical insights to construct a robust and comprehensive model that accurately captures the complexities of trade dynamics.
4. Results and discussion
4.1. Descriptive statistics
furnishes a comprehensive overview of various logarithmically transformed variables, each accompanied by crucial statistical measures encompassing mean, Standard Deviation (SD), minimum (Min), and maximum (Max) values. Regarding 'log ' variable, its mean of 4.25 serves as a beacon of the broad spectrum of trade volumes encapsulated within dataset. The substantial SD of 1.86 attests to the significant variability in trade levels exhibited across the different cases. This variability is a testament to the multifaceted nature of trade, influenced by factors such as economic conditions, trade agreements, and the ebb and flow of market dynamics. It is this intricate interplay that gives rise to the pronounced range between the minimum (0.00) and maximum (6.39) values, as the diverse economic scenarios of individual cases impact their trade performance. For 'log(
)', the mean value of 10.93 stands as a signpost indicating a substantial average magnitude. The relatively modest SD of 1.03 hints at a distribution that clusters closely around this mean. However, the span from 8.94 to 13.75 is where the richness of economic disparities becomes evident. This range underscores existence of diverse economies within the dataset, each characterized by varying levels of GDP. These variations are due to natural resource endowments, economic strengths, and the intricate web of trade relationships that foster interdependencies among economies (Sokvibol et al., Citation2019). Similarly, the mean of 6.18 for ‘log(
)' provides a snapshot of the logarithmic product of PCI levels. The relatively constrained SD of 0.69 suggests a distribution that clusters around the mean. However, the range from 4.89 to 7.73 unveils a moderate variance that underscores the nuanced economic capacities of the countries involved. These distinctions are due to industry specialization, technological advancement, and differing access to resources and opportunities. Further delving into 'log(
)', the mean of 2.75 denotes the average magnitude of change in PCI levels. This statistical marker is accompanied by a notable SD of 0.79, which indicates substantial variability around this mean. The range from 0.47 to 4.36 bears testimony to the considerable extent of change in PCI across cases. This divergence could be attributed to varying economic growth rates, shifts in industrial composition, and changes in policy frameworks that contribute to the evolving economic landscape.
Table 2. Descriptive statistics of selected variables.
As we examine 'logGFCFj’, its mean of 4.22 signifies the average magnitude of gross fixed capital formation. The considerable SD of 0.99 indicates significant variation in investment levels, underscoring the diverse investment patterns within the dataset. The range spanning from 1.98 to 6.87 mirrors the economic cycles and infrastructural initiatives that influence investment decisions. The dynamic nature of economic growth and infrastructure development contributes to this discernible variation in investment. In the realm of 'logTIRj’, the mean of 5.57 underscores an average magnitude of total imports of rice by trade partners. The SD of 0.94 hints at relatively clustered values around the mean, while the range from 0.30 to 6.69 reveals a spectrum of total imports. This spectrum is shaped by trade agreements, economic specialization, and market access that contribute to observed variability. The higher mean value of 5.45 for 'logAREAj’ is complemented by a relatively higher SD of 1.79, indicating substantial variability in land areas. The range spanning from 0.04 to 7.50 reflects the broad spectrum of land sizes across cases, serving as a reflection of geographical diversity and the varied land utilization patterns that influence economic activities. Similarly, the 'logPRODj’ variable, with a mean of 5.96 and a notable SD of 1.93, unveils the significant variability in production quantities. The range spanning from 0.32 to 8.33 underscores the wide variation in production levels, which is attributed to the diverse economic activities and sectors encompassed within the dataset. Moving on to 'logEXCRTj’, the mean of 2.47 and higher variability (SD = 1.15) is underscored by the range from 0.57 to 4.62, which serves as a testament to dynamic nature of international currency markets. This dynamism is fuelled by various factors viz., economic indicators, geopolitical events, and market sentiments that contribute to observed variability. The range of 'logPOPj’ from 3.78 to 6.15 encapsulates diverse population sizes, reflecting the demographic landscape of the observed cases, and is characterized by a mean of 4.67. Similarly, the mean of 3.45 for 'logDISTj’ spanning from 2.18 to 4.08, reflects the diverse trade relationships influenced by factors like geographic proximity, transportation infrastructure, and trade agreements (Yadav, Citation2021).
The tabulated data also comprises binary indicator variables. Regarding 'COBj’, denoting common border between India and trade partners, the mean value of 0.3 indicates that approximately 30 per cent of trade partners have a common border with India (Bangladesh, China and Nepal). This historical aspect could potentially exert influence on trade dynamics through shared historical ties and economic legacies. The 'WTOmemj’ variable assumes a mean of 0.6, signifying that 60% of trade partners are WTO members. This condition applies to key trade partners like Bangladesh, Benin, China, Côte d‘Ivoire, Senegal, and Vietnam. Membership in the WTO implies adherence to standardized trade regulations, potentially fostering smoother trade transactions and diminished trade barriers. Regarding 'COLONYj’, reflecting colonial relationships, the mean of 0.1 signifies that 10% of trade relationships involve colonial links (Bangladesh). Conversely, the lower values for other nations indicate the absence of this historical aspect in their trade relationships. The 'LLj’ variable holds a mean of 0.1, implying Nepal is only landlocked status and can introduce distinct trade challenges due to reliance on neighboring countries for transportation routes, potentially shaping trade patterns and intensity. Similarly, with a mean of 0.1, 'ASEANj’ shows Vietnam is the sole ASEAN country and this membership can confer preferential trade agreements and regional economic cooperation, thereby influencing trade interactions. The 'AFRICAj’ variable carries a mean value of 0.2, implying that approximately 20% of trade partners are African nations. This aspect holds significance for Benin and Senegal. This membership can impart distinct trade dynamics due to regional economic agreements and policies that shape trading relationships. Lastly, the binary indicator 'REC2008j’ assumes a mean value of 0.7, indicating that around 70% of trade partners experienced a recession between 2008 and 2016. This information proves pivotal in understanding trade patterns during economic downturns. Notably, the absence of recession within Ivory Coast, Nepal, and Senegal during this period could potentially contribute to their trade resilience. These indicators offer a nuanced understanding of India’s trade relationships with top partners in terms of rice exports. The interplay of historical, economic, and regional factors shapes these dynamics, highlighting the multifaceted nature of international trade.
4.2. Panel CD test
presents the outcomes of the Panel CD test using different tests. These results collectively suggest strong evidence of CD among residuals (Ho: No CD among residuals). The confirmed CD guarantees that the forthcoming outcomes of the gravity model will rest upon a thorough understanding of the complex interrelationships and interactions present among the variables. This enhances the reliability and accuracy of the ensuing analysis, leading to a more nuanced and insightful exploration of the underlying dynamics governing the data.
Table 3. Results of Panel CD test.
4.3. Trade-related Indices for India in relation to leading importing partners
The Export, Import, and Trade Intensity Indices (TE 2021) provide insights into the trade relationship between India and its top 10 leading trade partners in terms of rice (). These indices help us understand the dynamics of rice trade between India and these countries.
Table 4. India’s Export, Import and Trade Intensity Indices of rice among top 10 leading trade partners (TE 2021).
i. EII: This index serves as a pivotal metric, illuminating the importance of India’s rice exports to its top 10 leading trade partners. This index quantifies the magnitude of India’s rice exports to a specific partner concerning its overall rice export portfolio. In this context, the EII values spanning from 1.490 (quantity) to 2.008 (value) underscore India’s active participation in rice trade with these nations. Several key factors synergistically contribute to this dynamic trade landscape. Geographical proximity and geopolitics emerge as influential forces, especially in the cases of countries like Bangladesh, Nepal, and Iran. The geographical closeness shared with India plays a pivotal role in facilitating streamlined trade logistics, consequently lowering transportation costs for commodities such as rice. Moreover, historical and geopolitical connections could be nurturing more robust trade relationships, thereby amplifying the observed EII values. Even the sizable populations of trading partners along with distinctive rice consumption patterns fuelled heightened demand for rice imports. As a trusted and reliable rice supplier, India is strategically positioned to meet this surging demand, consequently boosting the observed EII values.
ii. III: This index unveils the intricate tapestry of factors that underscore the critical role of Indian rice in the import strategies of these partner nations. It gauges the degree of dependence these countries have on India as a primary source of rice imports, in comparison to other potential trade partners. The III values, spanning from 0.185 (value) to 0.205 (quantity), vividly underscore the pivotal status of India as a key rice supplier to these nations. Notably, the quality and variety of Indian rice significantly contribute to its prominence within these countries’ import portfolios. Renowned for its diverse array of rice varieties that align adeptly with varying culinary preferences, Indian rice stands out as an enticing choice for these partner nations. This culinary compatibility is a compelling factor that heightens the prominence of Indian rice within their import baskets. The price competitiveness of Indian rice emerges as another compelling driver. Striking the right balance between quality and pricing, Indian rice is notably attractive to these partner nations. The fusion of affordability and acceptable quality presents a compelling proposition, potentially spearheading the robust III values. Moreover, supply assurance plays an instrumental role in solidifying the reliance on Indian rice imports.
iii. TII: This index, a comprehensive metric factoring in both EII and III, provides a holistic panorama of the bilateral trade dynamics. With TII values spanning from 1.375 (quantity) to 1.891 (value), these indices serve as a barometer of the depth and vigour characterizing these trade relationships. These values underscore the reciprocal advantages yielded through trade of rice. The spirit of mutual cooperation and existence of favourable diplomatic ties can catalyze the expansion of trade, thereby reflecting in higher TII values. The enduring trade partnerships between India and its counterparts have significantly propelled Indian rice exports, amplifying the TII values.
These three indices collectively provide a comprehensive framework through which to comprehend the intricate dynamics of India’s rice trade with its top 10 leading trade partners. These indices serve as insightful tools that unveil the multifaceted tapestry of factors underpinning the significant trade relationships in the global rice market. This intricate interplay of various elements sheds light on the key drivers that contribute to India’s remarkable rice exports to these partner nations.
4.4. Determinants for bilateral trade between India and trading partners:
The utilization of Heckman selection equation () serves the purpose of delving into the complex dynamics that underlie the decision-making process behind the selection of trading partners. This analytical model effectively addresses potential biases that could arise when studying a subset of potential trading partners. By doing so, it provides valuable insights into the intricate interplay of factors that influence the probability of opting for a particular partner for bilateral trade with India. The coefficients in this equation are indicative of direction of influence each factor holds in this selection process.
Table 5. Determinants for bilateral trade between India and trading partners (Selection equation from Heckman model).
The positive coefficient of +12.518 associated with the variable log() indicates that the interaction between GDPs of India and its trading partners has a notable and favorable influence on the likelihood of selecting a particular partner for bilateral trade (Anh Thu et al., Citation2019; Liu et al., Citation2020). This suggests that when the combined GDPs of India and a trading partner are higher, it signifies economic compatibility and trade potential. Such partnerships offer larger consumer markets, increased demand for goods and services, and diversified trade opportunities across sectors. The elevated GDPs also reflect economic stability and resilience, fostering confidence in trade relationships. Similarly, regarding interaction variable viz., log(
), the positive coefficient of 1.375 signifies that countries with higher per capita incomes are more attractive as trading partners due to their enhanced purchasing power. Conversely, the log(
) coefficient of −1.010 carries a negative sign, indicating that an increase in difference between per capita incomes between India and a trading partner, the probability of selecting that partner for bilateral trade diminishes. So, when substantial income disparities exist, the appeal of engaging in trade with such partners wanes due to differing levels of economic capacity and consumer demand. The coefficient of logTIRj (5.476*) suggests that, as total imports of rice by a trading partner increase, the likelihood of India selecting that partner for bilateral trade also increases (Sokvibol et al., Citation2019). Similarly, coefficient of logGFCFj at −0.972 unveils an intriguing pattern: an increase in GFCF in a trading partner’s economy is linked with a decreased likelihood of being selected for bilateral trade. This alignment implies that partners with greater investment focus might prioritize domestic production over imports, thereby mitigating their reliance on trade. In the realm of exchange rates, the coefficient of logEXCRTj (7.567) demonstrates that depreciation of Indian currency results in increase in probability of rice exports to trading partners (Igue & Ogunleye, Citation2014; Irshad et al., Citation2018b; Jordaan & Eita, Citation2012). The negative coefficient for logAREAj (−0.196) indicates that an increase in area under rice of a trading partner reduces the likelihood of selecting that partner for bilateral trade. Furthermore, the negative coefficient of logPRODj (−1.663) suggests that an elevation in the level of production within a trading partner’s economy corresponds to a lower likelihood of being chosen for bilateral trade. This trend could reflect a preference for trading with partners specializing in distinct goods to promote diversification.
The influence of distance on partner selection is evident through coefficient of logDISTj (−1.378). As distance between India and a trading partner increases, the probability of choosing that partner for bilateral trade declines. This outcome underscores the higher costs and logistical complexities associated with greater geographical separation (Braha et al., Citation2017; Nasrullah et al., Citation2020; Yadav, Citation2021). The positive coefficient of logPOPj (1.018) implies that a larger population in a trading partner’s country heightens the likelihood of being selected for bilateral trade. Larger populations equate to expanded consumer bases and more substantial trade prospects.
4.5. Determinants for quantity of rice trade
provides details about the estimates of gravity model from three distinct models—GLS (Sokvibol et al., Citation2019), PPML (Amjad et al., Citation2021; Martin & Pham, Citation2020; Santos Silva & Tenreyro, Citation2011); Heckman (Haq et al., Citation2013; Hasiner & Yu, Citation2019; Semykina & Wooldridge, Citation2013; Sokvibol et al., Citation2019; Xiong & Chen, Citation2014). The signs of estimated coefficients consistently align with expectations and attain statistical significance at conventional levels. Notably, the outcomes of the PPML and Heckman approaches exhibit greater significance when compared to GLS model. This emphasizes the robustness and enhanced statistical validity of the PPML and Heckman methodologies, offering more substantial insights into the relationships being explored. The findings showed that ‘log()’ consistently displays positive coefficients in all models, indicating that historical trade interactions positively influence selection of partners for current bilateral trade. This suggests that trade relationships are built upon past engagements, fostering a sense of familiarity and trust among partners. Previous studies like Martínez-Zarzoso et al. (Citation2009), Olivero and Yotov (Citation2012), Gashi et al. (Citation2016) and Kahouli (Citation2016), also provided empirical evidences to support the importance of history in performance of trade. The interactions of economic indicators, such as log(
) and log(
), consistently show strong and positive coefficients across models (Filippini & Molini, Citation2003; Shahriar et al., Citation2018). This signifies that partners with larger combined GDPs and PCIs are more preferred for bilateral trade. The enhanced market potential, purchasing power, and economic compatibility associated with larger GDPs and incomes contribute to this positive relationship. Notably, the variable log(
) consistently demonstrates negative coefficients across models highlighting that larger disparities in per capita incomes among trading partners affect rice trade from India. This consistently negative and statistically significant effect underscores adherence of Indian rice exports to the principle of Linder hypothesis (Linder, Citation1961). According to this hypothesis, countries with comparable income levels tend to share similar characteristics, supply capacities, and demand profiles, consequently fostering higher levels of trade between them. This could also be attributed to trade challenges arising from significant income differences, affecting market preferences and trade capacities. A body of existing research further reinforces this key tenet of the Linder hypothesis. Studies such as those by Arnon and Weinblatt (Citation1998), Choi (Citation2002), Chow et al. (Citation1999), and McPherson et al. (Citation2000) lend support to this hypothesis by substantiating the idea that nations with akin income levels are inclined to engage in more extensive trade interactions with one another. The coefficients associated with logTIRj also have consistent patterns across the models. In the PPML and Heckman models, the coefficients are positive and statistically significant implying positive correlation between trading partner’s rice imports and India’s rice exports. That is, as total imports of rice by a trading partner increase, there is corresponding rise in demand for rice exports from India. However, it is important that this relationship is also influenced by various market factors, supply-demand dynamics, and the competitive position of Indian rice in trading partner’s market.
Table 6. Estimation of Gravity model through GLS, PPML and Heckman approaches.
Both ‘logAREAj’ and ‘logPRODj’ exhibit negative association with quantum of trade between India and trading partners. That is, an increase in area and production of rice in trading partners, the trade quantity between India and that partner tends to decrease. This finding underscores the role of distance-related factors, including transportation costs and market accessibility, which can deter trade and consequently influence trade volumes. It also indicates the inclination for India to engage in trade with partners specializing in different goods, avoiding excessive competition that could impact trade volumes. Similarly, the uniform positive coefficients for logEXCRTj underscore a pivotal aspect: the depreciation of Indian currency between India and its trading partners can make Indian rice more attractive and affordable to international markets. As a result, trading partners increase their demand for
Indian rice, leading to an upsurge in its exports. The preceding research conducted by Bui and Chen (Citation2017) and Irshad et al. (Citation2018b), similarly underscores the significant influence exerted by exchange rate policies on the realm of rice exports.
The positive coefficients for logPOPj prevalent across models accentuate a compelling trend: trading partners with larger populations typically engage in larger trade volumes with India, though non-significant. This trend can be explained by factors such as heightened demand, specialization, and geographic factors. The coefficient of logDISTj consistently displayed negative sign across all the models, indicating that as distance between India and its trading partners increases, trade volumes tend to decrease. This outcome reflects the various cost-related, logistical, and administrative challenges ultimately influencing trade decisions and resulting in reduced trade volumes. The coefficient of COBj is consistently positive and found significant both in PPML and Heckmen models. This implies, trade between countries sharing a border tends to increase due to factors like reduced transportation costs, quicker delivery times, improved infrastructure, trade agreements, cultural affinities fostering cooperation, and spillover effects benefiting both economies. Proximity enables efficient supply chains and access to neighboring markets, encouraging trade growth. The positive coefficients for ‘WTOmemj’ across all models indicate that member countries of WTO tend to engage in larger quantities of rice trade with India. This is due to facilitation of trade agreements, reduced trade barriers, and increased market access that often accompany WTO membership, encouraging more substantial bilateral trade volumes (Rahman et al., Citation2019; Soeng & Cuyvers, 2018). Additionally, streamlined customs procedures and harmonized trade facilitation efforts reduce transaction complexities and costs. Alignment with international trade standards and the enhanced reputation of being a responsible trading partner further enhance the appeal of WTO members. Altogether, these factors create a synergy that encourages bilateral trade selection, offering partners the benefits of reduced barriers, a secure legal framework, and increased trade opportunities.
However, the influence of certain factors viz., COLONYj, LLj, ASEANj and AFRICAj appears negative and non-significant influence on quantum of rice trade from India (Paudel & Cooray, Citation2018). These findings disclose that if the importer is landlocked, transaction costs are too high (Milner & Mcgowan, Citation2013). The variable REC2008j consistently shows negative coefficients across the models, indicating a certain trend in its impact on quantity of rice trade between India and its trading partners. However, this negative effect is found statistically significant only in PPML model. This indicates decreased demand for rice imports among trading partners from India (Huot & Kakinaka, Citation2007; Kahouli, Citation2016).
presents estimates from both the one-way and two-way fixed effects models for the determinants of rice exports from India in the gravity model context. Notably, the coefficient for () is statistically significant in both models, with a substantial increase in magnitude in the two-way fixed effects model. This suggests a strong positive correlation between the economic sizes of the trading partners, indicating that larger economies tend to engage in more agricultural trade. Similarly, the coefficient for log(
) is negative in both models, implying that as the income disparity between the trading partners decreases, agricultural trade increases. The two-way fixed effects model shows a more pronounced negative impact, suggesting that income parity is a crucial factor influencing agricultural exports. The log(
) has a positive and significant effect on agricultural exports in both models, indicating that as the income levels of both countries change, agricultural trade is positively affected. The log(
) of the importing country’s area and log(
) has a negative impact, but it is statistically insignificant in the one-way fixed effects model. However, in the two-way fixed effects model, the coefficient for log(
) becomes significant and negative, suggesting that larger agricultural areas and production in the importing country could hinder agricultural exports from India. Population size of the importing country does not show a significant impact on agricultural exports in either model. The log(
) has a positive impact in both models is a result of a combination of factors ranging from India’s production capacity, competitive pricing, adherence to quality standards, diplomatic relations, cultural preferences, and efficient trade logistics. The log(
) has a positive impact in both models, suggesting that a depreciation of the importing country’s currency may stimulate agricultural exports from India. The F-test for overall model significance is statistically significant in both models, indicating that the models are collectively meaningful. The Hausman test suggests a preference for the fixed effects model over random effects model. The R2 values for both models are reasonably high indicating that the models explain a substantial portion of the variability in agricultural exports.
Table 7. Gravity model estimates: one-way and two-way fixed effects model (n = 270).
5. Summary and conclusions
In summary, international trade stands as a linchpin of economic growth and development, enabling nations to expand their economic horizons beyond domestic borders. This engagement with global economy brings forth a myriad of advantages, from increased consumer choices and job creation to the exchange of knowledge and technology. International trade fosters diplomatic relationships, mitigates supply chain vulnerabilities, and provides avenues for stability during economic uncertainties. For India, a country with a substantial agrarian economy, the international trade of agricultural commodities, particularly rice, holds immense significance. This engagement opens doors to larger markets, stimulates economic growth, reduces poverty, and elevates living standards.
India’s participation in international trade is reinforced by its membership in WTO, which offers a framework for fair and transparent trade relations. This membership empowers India to resolve disputes, negotiate favourable agreements, and protect its interests in global agricultural market. Leveraging its diverse agro-climatic zones, India has emerged as a leading exporter of rice in international market. The country’s expansive land resources, ranging from the fertile plains of Punjab and Haryana to the deltas of the eastern and southern coasts, provide ideal conditions for cultivating a wide variety of rice. India’s ability to produce both traditional and aromatic rice varieties give it a competitive edge, catering to diverse preferences in global markets.
The EII values, ranging from 1.375 to 2.008, highlight active rice trade driven by factors like geographical proximity, reducing transportation costs, and geopolitics. The III values, spanning 0.185 to 0.205, underscore India’s pivotal status as a primary rice supplier. Indian rice’s diverse varieties align with culinary preferences, enhancing its prominence. The TII, combining EII and III, with values, 1.375 to 1.891, showcased the depth and vigour of trade relationships. Political stability, diplomacy, and long-term partnerships catalyze trade intensity, reflecting in higher TII values, fostering mutual cooperation and robust interactions.
The Gravity model employed in analyzing India’s international rice trade provides a robust analytical framework to unravel intricate dynamics governing these trade relationships. By focusing on major importing countries like Bangladesh, Benin, China, Ivory Coast, Iran, Iraq, Nepal, Saudi Arabia, Senegal, and Vietnam, the study offers insights into the core drivers of India’s rice exports, their economic impact, and market trends. The model underscores the influence of historical trade interactions, economic indicators, geographical proximity, WTO membership, and other factors on partner selection and trade quantity.
In comparing the outcomes of PPML and Heckman models with the one-way and two-way fixed effects models for determining rice exports from India in the context of the gravity model, several key insights emerge. Notably, both PPML and Heckman approaches exhibit greater significance compared to GLS model, highlighting their robustness and enhanced statistical validity. The findings emphasize the substantial influence of historical trade interactions, as indicated by the consistently positive coefficients of ‘log()’ across all models. This underscores the notion that past engagements foster familiarity and trust among trading partners, influencing current bilateral trade decisions. Economic indicators, such as log(
) and log(
) consistently display strong and positive coefficients, emphasizing that partners with larger combined GDPs and PCIs are more preferred for bilateral trade. The consistently negative coefficient of 'log(
)' underscores adherence to the Linder hypothesis, suggesting that disparities in per capita incomes among trading partners negatively affect rice trade from India. Moreover, variables like ' logTIRj’ exhibit positive correlations with India’s rice exports, implying that as total rice imports by a trading partner increase, there is a corresponding rise in demand for rice exports from India. Conversely, ‘logAREAj’ and ‘logPRODj’ show negative associations, indicating that an increase in the area and production of rice in trading partners tends to decrease trade quantity between India and that partner. This reflects the role of distance-related factors, including transportation costs and market accessibility. The positive coefficients for 'logEXCRTj’ underscore the significant influence of currency depreciation in the importing country, making Indian rice more attractive and affordable to international markets. Population size of the importing country (logPOPj) and the distance between India and its trading partners also demonstrate expected trends, with larger populations and shorter distances positively impacting trade volumes. The one-way and two-way fixed effects models provide additional insights into the determinants of rice exports. The coefficients for ‘log(
)’ and ‘log(
)' remain statistically significant, with the two-way fixed effects model exhibiting a more pronounced impact. Income parity and changes in income levels continue to influence agricultural trade positively. Importantly, the two-way fixed effects model introduces the significance of ‘logAREAj’ and ‘logPRODj’, suggesting that larger agricultural areas and production in the importing country could hinder agricultural exports from India. PPML, Heckman and one-way and two-way fixed effects models provide a nuanced understanding of the determinants of rice exports from India. Each model contributes unique perspectives, emphasizing the importance of considering historical interactions, economic indicators, and geographical factors in comprehensively analyzing the complex dynamics of agricultural trade.
This study offers valuable insights to strategically enhance international trade relationships. India’s active involvement in global trade, particularly in rice, reflects its commitment to utilizing strengths and contributing to the global market. The policy implications range from diversifying markets and improving quality to facilitating trade, diplomatic engagement, sustainability, and capacity building. Diversifying markets is crucial, urging policymakers to explore new regions through proactive trade agreements. Elevating the quality of Indian rice requires investing in research and development to keep its appeal high. Streamlined customs procedures and efficient logistics networks are essential for smooth cross-border trade. Encouraging sustainable agricultural practices can enhance competitiveness. Empowering farmers through training, technology, and financial support is vital. By strategically addressing these areas, India can solidify its role as a major player in global rice trade, promoting economic growth, agricultural development, and international cooperation. These policies align with Sustainable Development Goals, enabling India to fortify its position in the global rice trade and contribute positively to a sustainable and prosperous future.
Authors’ Contributions
K.N.R.K.: conceptualization, methodology, data collection, data curation, data analysis, writing—initial draft; K.G.R: expert comments and suggestions; M.J.M.: data analysis, interpretation, expert suggestions; A.B.S.: expert comments and suggestions, revisions. All authors have read and agreed to the published version of the manuscript.
Disclosure statement
The authors declare no conflict of interest.
Data availability statement
The data presented in this study are available on request from the authors.
Additional information
Notes on contributors
K. Nirmal Ravi Kumar
Dr. K. Nirmal Ravi Kumar holding Master’s and Ph.D. degrees in Agricultural Economics, has studied at Acharya N.G. Ranga Agricultural University (ANGRAU). He has a brilliant academic career with specialization in ‘Agricultural Marketing’ both in his post-graduate and doctoral programmes. Dr. Kumar is currently Professor & Head (Agril. Economics) in Agricultural College, Bapatla. He is actively involved both in agricultural research and teaching activities during the past twenty-two years in the ANGRAU. He also worked as Director (Agricultural Marketing) in National Institute of Agricultural Extension Management (MANAGE), Ministry of Agriculture & Farmers’ Welfare, Government of India. He was the recipient of ‘Sri Mocherla Dattatreyulu Gold Medal’ (2013) and ‘State Best Teacher Award’ (2016). Dr. Kumar has written extensively and has to his credit 10 books, and six (6) published articles in reputable journal of higher impact.
K. Gurava Reddy
Dr. K. Gurava Reddy has played a pivotal role in advancing agricultural research and development, particularly in the domain of enhancing smallholder livelihoods through innovative institutional arrangements. Serving as the Technical Secretary to the Vice Chancellor of ANGRAU and a Visiting Scientist at ICRISAT, his contributions span various areas. Noteworthy achievements include training watershed teams in Participatory Rural Appraisal techniques, conducting research on critical issues such as cotton farmers’ suicides and the productivity of scientists, and promoting Dry Direct Seeded Rice cultivation, significantly expanding its acreage in Guntur district. Dr. K. Gurava Reddy’s multifaceted contributions underscore his dedication to advancing agricultural practices and improving rural livelihoods.
Adinan Bahahudeen Shafiwu
Adinan B. Shafiwu, Ph.D. Adinan B. Shafiwu, is currently a J-Pal African Scholar who has just finished his project on Digitalization of Cocoa Value Chain in Ghana under the DigiFi initiatives. He has worked and participated in J-pal initiatives that involves Randomized Control Trial. As part of his training under J-Pal he participated in both DIWA and Development Methodologies workshops here in Ghana and Morocco-Rabat respectively. He holds a PhD and MPhil in (Agricultural Economics) and also a chartered Health Economics certificates and currently works with the University for Development Studies in the capacity as a lecturer. He is an expert in Adoption Studies, efficiency, welfare and consumption studies. He is into modeling and has deep seated knowledge in econometrics and its models. A good data manager. He has also consulted for Plan International Ghana and Oxfarm on both midline and Baseline survey. He has published over twenty (20) research articles in reputable journals with higher impact. He is Highly regarded for his good leadership, communication, proposal writing, research, and analytical skills. Able to establish, implement and monitor systems for effectiveness and efficiency at organizations.
M. Jagan Mohan Reddy
Dr. M. Jagan Mohan Reddy has 23 years of experience in the areas of teaching, extension, communication and administration in the university. He started his career as Assistant Extension Specialist in Agriculture Research Station, Tandur in 1998, from there he continued his journey has a good teacher and administrator till date. He worked has a teacher at College of Agriculture for 10 years, Extension specialist and Programme Coordinator at KVK, Palem, Mahabubnagar dist and DAAT T Centre, Ranga Reddy district for 6 years, 1 year at Agril. Information & Communication Centre, Rajendranagar, Hyderabad. He published nearly 105 research papers; Guided 12 students : M.Sc (Ag)-10-major; 5 minor and Ph.D-02 scholars were completed as major; 2 are under guidance as major, and organized 3 webinars.
References
- Adhikari, A., Sekhon, M. K., & Kaur, M. (2016). Export of rice from India: Performance and determinants. Agricultural Economics Research Review, 29(1), 135–150. https://doi.org/10.5958/0974-0279.2016.00026.4
- Agricultural Statistics at a Glance. (2021). Ministry of agriculture and farmers welfare, department of agriculture, cooperation & farmers welfare. Government of India.
- Alka, S., & Harpreet, K. (2023). India’s trade potential with China: A gravity model approach. European Chemical Bulletin, 12(10), 1339–1358.
- Amjad, M., Sami, U., Yasmin, T., & Aslam, M. (2021). Impact of China-Pakistan Regional Trade Agreement on Pakistan’s Exports: An Analysis Using Gravity Trade Model. Pakistan Journal of Applied Economics, 31(1), 99–112. https://www.aerc.edu.pk/wp-content/uploads/2021/08/Paper-939-AMJAD-MASOOD-V-1.pdf
- Anderson, J. E., & Wincoop, E. (2004). Trade costs. Journal of Economic Literature, 42(3), 691–751. https://doi.org/10.1257/0022051042177649
- Anh Lan Thi, N., Le Pham, T., & Xuyen, V. K. T. (2022). Determinants of the world’s rice trade: The role of trade costs. Journal of Asian Finance, Economics and Business, 9(5), 313–324. https://doi.org/10.13106/jafeb.2022.vol9.no5.0313
- Anh Thu, L., Fang, S., & Kessani, S. S. (2019). Factors influencing Vietnam’s handicraft export with the gravity model. Journal of Economics and Development, 21(2), 156–171. https://doi.org/10.1108/JED-08-2019-0021
- Arnon, A., & Weinblatt, J. (1998). Linder’s hypothesis revisited: income similarity effects for low income countries. Applied Economics Letters, 5(10), 607–611. https://doi.org/10.1080/135048598354267
- Baier, S. L., & Bergstrand, J. H. (2007). Do free trade agreements actually increase members international trade? Journal of International Economics, 71(1), 72–95. https://doi.org/10.1016/j.jinteco.2006.02.005
- Baldwin, R., & Taglioni, D. (2006). Gravity for dummies and dummies for gravity equations. CEPR. In Discussion papers. National Bureau of Economic Research, Working Paper 12516. http://www.nber.org/papers/w12516.
- Baltagi, B. H., Egger, P. H., & Kesina, M. (2018). Generalized spatial autocorrelation in a panel-probit model with an application to exporting in China. Empirical Economics, 55(1), 193–211. https://doi.org/10.1007/s00181-017-1409-0
- Boerema, A., Peeters, A., Swolfs, S., Vandevenne, F., Jacobs, S., Staes, J., & Meire, P. (2016). Soybean trade: balancing environmental and socio-economic impacts of an intercontinental market. PloS One, 11(5), e0155222. https://doi.org/10.1371/journal.pone.0155222
- Braha, K., Qineti, A., Cupák, A., & Lazorčáková, E. (2017). Determinants of Albanian agricultural export: The gravity model approach. AGRIS on-Line Papers in Economics and Informatics, 9(665-2017-1574), 3–21. https://doi.org/10.22004/ag.econ.262457
- Breusch, T. S., & Pagan, A. R. (1980). The Lagrange multiplier test and its applications to model specification in econometrics. The Review of Economic Studies, 47(1), 239–253. https://doi.org/10.2307/2297111
- Bui, T. H. H., & Chen, Q. (2017). An analysis of factors influencing rice export in Vietnam based on gravity model. Journal of the Knowledge Economy, 8(3), 830–844. https://doi.org/10.1007/s13132-015-0279-y
- Carrère, C. (2006). Revisiting the effects of regional trade agreements on trade flows with proper specification of the gravity model. European Economic Review, 50(2), 223–247. https://doi.org/10.1016/j.euroecorev.2004.06.001
- Castillo, J. S., Villanueva, E. C., & García‐Cortijo, M. C. (2016). The international wine trade and its new export dynamics (1988–2012): A gravity model approach. Agribusiness, 32(4), 466–481. https://doi.org/10.1002/agr.21463
- Chaney, T. (2008). Distorted gravity: The intensive and extensive margins of international trade. American Economic Review, 98(4), 1707–1721. https://doi.org/10.1257/aer.98.4.1707
- Chaney, T. (2018). The gravity equation in international trade: An explanation. Journal of Political Economy, 126(1), 150–177. https://doi.org/10.1086/694292
- Choi, C. (2002). Linder hypothesis revisited. Applied Economics Letters, 9(9), 601–605. https://doi.org/10.1080/13504850110111234
- Chow, P., Kellman, M., & Shachmurove, Y. (1999). A test of the Linder hypothesis in pacific newly industrialized countries trade. Applied Economics, 31(2), 175–182. https://doi.org/10.1080/000368499324408
- Cosslett, T. L., & Cosslett, P. D. (2018). Sustainable development of rice and water resources in Mainland Southeast Asia and Mekong River Basin. Singapore: Springer. https://doi.org/10.1007/978-981-10-5613-0
- Dal Bianco, A., Boatto, V. L., Caracciolo, F., & Santeramo, F. G. (2016). Tariffs and non-tariff frictions in the world wine trade. European Review of Agricultural Economics, 43(1), 31–57. https://doi.org/10.1093/erae/jbv008
- Dascal, D., Mattas, K., & Tzouvelekas, V. (2002). An analysis of EU wine trade: A gravity model approach. International Advances in Economic Research, 8(2), 135–147. https://doi.org/10.1007/BF02295344
- Eberhardt, M., & Teal, F. (2010). Aggregation versus Heterogeneity in cross-country growth empirics. CSAE working paper series 2010-32, Centre for the study of African economies. University of Oxford. https://ora.ox.ac.uk/objects/uuid:adc43db1-daab-434a-9aea-ac149861d6a9
- Fan, Z., Zhang, R., Liu, X., & Pan, L. (2016). China’s outward FDI efficiency along the Belt and Road: An application of stochastic frontier gravity model. China Agricultural Economic Review, 8(3), 455–479. https://doi.org/10.1108/CAER-11-2015-0158
- Filippini, C., & Molini, V. (2003). The determinants of East Asian trade flows: A gravity equation approach. Journal of Asian Economics, 14(5), 695–711. https://doi.org/10.1016/j.asieco.2003.10.001
- Gashi, P., Hisarciklilar, M., & Pugh, G. (2016). Kosovo–EU trade relations: A dynamic panel poisson approach. Applied Economics, 49(27), 2642–2654. https://doi.org/10.1080/00036846.2016.1245836
- Ghazalian, P. L., Tamini, L. D., Larue, B., & Gervais, J. P. (2012). A gravity model to account for vertical linkages between markets with an application to the cattle/beef sector. The Journal of International Trade & Economic Development, 21(4), 579–601. https://doi.org/10.1080/09638199.2010.505297
- Gupta, S., & Kumar, N. (2020). Time varying dynamics of globalization effect in India. Portuguese Economic Journal, 22(1), 81–97. https://doi.org/10.1007/s10258-020-00190-4
- Haq, Z. U., Meilke, K., & Cranfield, J. (2013). Selection bias in a gravity model of agrifood trade. European Review of Agricultural Economics, 40(2), 331–360. https://doi.org/10.1093/erae/jbs028
- Hasiner, E., & Yu, X. (2019). When institutions matter: A gravity model for Chinese meat imports. International Journal of Emerging Markets, 14(1), 231–253. https://doi.org/10.1108/IJoEM-11-2016-0290
- Heckman, J. J. (1979). Sample selection bias as a specification error. Econometrica, 47(1), 153–161. https://doi.org/10.2307/1912352
- Huot, N., & Kakinaka, M. (2007). Trade structure and trade flows in Cambodia: A gravity model. Asean Economic Bulletin, 24(3), 305–319. https://doi.org/10.1353/ase.2008.0008/10.1355/ae24-3b
- Hwang, C. W., & Lim, S. S. (2017). Effect of non-tariff measures on international tea trades. Journal of Korea Trade, 21(4), 309–323. https://doi.org/10.1108/JKT-05-2017-0054
- Igue, N. N., & Ogunleye, T. S. (2014). Impact of real exchange rate on trade balance in Nigeria. African Development Review, 26(2), 347–358. https://doi.org/10.1111/1467-8268.12086
- Irshad, M. S., & Xin, Q. (2017). The gravity behind South Korea’s international trade: A panel data approach. The Myanmar Journal, 4(2), 76–89. http://www.komyra.com/doc/submission.php
- Irshad, M. S., Xin, Q., & Arshad, H. (2018a). Competitiveness of Pakistani rice in international market and export potential with global world: A panel gravity approach. Cogent Economics & Finance, 6(1), 1486690. https://doi.org/10.1080/23322039.2018.1486690
- Irshad, M. S., Xin, Q., Shahriar, S., & Arshad, H. (2018b). A panel data analysis of China’s trade pattern with OPEC members: Gravity model approach. Asian Economic and Financial Review, 8(1), 103–116. https://doi.org/10.18488/journal.aefr.2018.81.103.116
- Jemal, Abafita, & Tekilu, Tadesse. (2021). Determinants of global coffee trade: Do RTAs matter? Gravity model analysis. Cogent Economics & Finance, 9(1), 1892925. https://doi.org/10.1080/23322039.2021.1892925
- Jordaan, A. C., & Eita, J. H. (2012). Determinants of South Africa’s exports of leather products. Agrekon, 51(2), 38–52. https://doi.org/10.1080/03031853.2012.695137
- Kahouli, B. (2016). Regional integration agreements, trade flows and economic crisis: A static and dynamic gravity model. International Economic Journal, 30(4), 450–475. https://doi.org/10.1080/10168737.2016.1204338
- Khalid, M. W., Kayani, A. S., Alotaibi, J. M., Muddassir, M., Alotaibi, B. A., & Kassem, H. (2020). Regional trade and food security challenges: The case of SAARC countries. Agricultural Economics (Zemědělská Ekonomika), 66(7), 335–344. https://doi.org/10.17221/3/2020-AGRICECON
- Kumar, M. (2019). India’s rice export: What is in it for farmers? Agrarian South: Journal of Political Economy, 8(1–2), 136–171. https://doi.org/10.1177/2277976019851930
- Lee, J. W., & Pyun, J. H. (2018). North Korea’s economic integration and growth potential. Asian Economic Journal, 32(3), 301–325. https://doi.org/10.1111/asej.12157
- Lee, M., & Tcha, M. (2005). Pass-through elasticity, substitution and market share: The case for sheep meat exports. The Journal of International Trade & Economic Development, 14(2), 209–228. https://doi.org/10.1080/09638190500093380
- Li, L., Sun, Z., & Long, X. (2019). An empirical analysis of night-time light data based on the gravity model. Applied Economics, 51(8), 797–814. https://doi.org/10.1080/00036846.2018.1523612
- Lien, D., & Lo, M. (2017). Economic impacts of cultural institutes. The Quarterly Review of Economics and Finance, 64, 12–21. https://doi.org/10.1016/j.qref.2017.02.002
- Linder, S. B. (1961). An essay on trade and transformation (pp. 82–109). Almqvist & Wiksell. https://ex.hhs.se/dissertations/221624-FULLTEXT01.pdf
- Liu, A., Lu, C., & Wang, Z. (2020). The roles of cultural and institutional distance in international trade: Evidence from China’s trade with the Belt and Road countries. China Economic Review, 61, 101234. https://doi.org/10.1016/j.chieco.2018.10.001
- Marti, L., Puertas, R., & García, L. (2014). Relevance of trade facilitation in emerging countries’ exports. The Journal of International Trade & Economic Development, 23(2), 202–222. https://doi.org/10.1080/09638199.2012.698639
- Martin, W., & Pham, C. S. (2020). Estimating the gravity model when zero trade flows are frequent and economically determined. Applied Economics, 52(26), 2766–2779. https://doi.org/10.1080/00036846.2019.1687838
- Martínez-Zarzoso, I., Felicitas, N. L. D., & Horsewood, N. (2009). Are regional trading agreements beneficial? Static and dynamic panel gravity models. The North American Journal of Economics and Finance, 20(1), 46–65. https://doi.org/10.1016/j.najef.2008.10.001
- Martínez-Zarzoso, I., & Johannsen, F. (2017). The gravity of arms. Defence and Peace Economics, 30(1), 2–26. https://doi.org/10.1080/10242694.2017.1324722
- Matyas, L. (Ed.). (2017). The econometrics of multi-dimensional panels: Theory and applications. Series Vol. 50. Springer. https://doi.org/10.1007/978-3-319-60783-2
- McPherson, M. A., Redfearn, M. R., & Tieslau, M. A. (2000). A re-examination of the Linder hypothesis: A random-effects Tobit approach. International Economic Journal, 14(3), 123–136. https://doi.org/10.1080/10168730000000032
- Milner, C., & Mcgowan, D. (2013). Trade costs and trade composition. Economic Inquiry, 51(3), 1886–1902. https://doi.org/10.1111/j.1465-7295.2012.00496.x
- Moscone, F., & Tosetti, E. (2009). “GMM estimation of spatial panels,” MPRA Paper 16327. University Library of Munich. https://mpra.ub.uni-muenchen.de/id/eprint/16327
- Moustafa Ismaiel, A. I., Zhou, D., Shawky Eladawy, R., El-Rasoul, A. A. E. Y. A., Tawfik Yousef Alkhateeb, T., Ali Ahmed Abdullah, T., & Mahmood, H. (2023). Determinants and potential of trade using the gravity model approach: Empirical evidence of Egyptian Rice Crop. Complexity, 2023, 1–16. https://doi.org/10.1155/2023/4791707
- Narayan, S., & Nguyen, T. T. (2016). Does the trade gravity model depend on trading partners? Some evidence from Vietnam and her 54 trading partners. International Review of Economics & Finance, 41, 220–237. https://doi.org/10.1016/j.iref.2015.08.010
- Nasrullah, M., Chang, L., Khan, K., Rizwanullah, M., Zulfiqar, F., & Ishfaq, M. (2020). Determinants of forest product group trade by gravity model approach: A case study of China. Forest Policy and Economics, 113, 102117. https://doi.org/10.1016/j.forpol.2020.102117
- Natale, F., Borrello, A., & Motova, A. (2015). Analysis of the determinants of international seafood trade using a gravity model. Marine Policy, 60, 98–106. https://doi.org/10.1016/j.marpol.2015.05.016
- Nazir, M. A., Shahriar, S., Kea, S., Abdullahi, A. M., Zhang, Q., & Huo, X. (2021). Nigeria’s cocoa exports: a gravity model approach. Ciência Rural, 51(11), e20201043. https://doi.org/10.1590/0103-8478cr20201043
- Nguyen, B. X. (2010). The determinants of Vietnamese export flows: Static and dynamic panel gravity approaches. International Journal of Economics and Finance, 2(4), 122–129. https://doi.org/10.5539/ijef.v2n4p122
- Nguyen, D. D. (2022). Determinants of Vietnam’s rice and coffee exports: Using stochastic frontier gravity model. Journal of Asian Business and Economic Studies, 29(1), 19–34. https://doi.org/10.1108/JABES-05-2020-0054
- Nguyen, H. T. M., Do, H., Kay, A., & Kompas, T. (2020). Rice policy in a transitional economy: Balancing the social and political objectives. Food Security, 12(3), 549–566. https://doi.org/10.1007/s12571-019-01005-x
- Nikolche, Jankulovski, & Usama, Ehsan Khan. (2022). Asian Rice Exports and the European Demand: An Application of the Modified Panel Gravity Model. Journal of Agricultural Science, 14(8). https://doi.org/10.5539/jas.v14n8p18
- Nugroho, A. D., Bhagat, P. R., Magda, R., & Lakner, Z. (2021). The impacts of economic globalization on agricultural value added in developing countries. PloS One, 16(11), e0260043. https://doi.org/10.1371/journal.pone.0260043
- Olivero, M. P., & Yotov, Y. V. (2012). Dynamic gravity: Endogenous country size and asset accumulation. Canadian Journal of Economics/Revue Canadienne D'économique, 45(1), 64–92. https://doi.org/10.1111/j.1540-5982.2011.01687.x
- Paudel, R. C., & Cooray, A. (2018). Export performance of developing countries: Does landlockedness matter? Review of Development Economics, 22(3), e36–e62. https://doi.org/10.1111/rode.12389
- Pesaran, M. H. (2004). General diagnostic tests for cross section dependence in panels (IZA Discussion Paper No. 1240). http://repec.iza.org/dp1240.pdf
- Popova, L., & Rasoulinezhad, E. (2016). Have sanctions modified Iran’s trade policy? An evidence of Asianization and De-Europeanization through the gravity model. Economies, 4(4), 24. https://doi.org/10.3390/economies4040024
- Poyhonen, P. (1963). A tentative model for the volume of trade between countries. Weltwirtschaftliches Archiv, 90, 93–100. https://www.jstor.org/stable/40436776
- Rafael, E. De Hoyos, & Vasilis, Sarafidis. (2006). Testing for cross-sectional dependence in panel-data models. The Stata Journal, 6(4), 482–496. https://doi.org/10.1177/1536867X0600600403
- Rahman, R., Shahriar, S., & Kea, S. (2019). Determinants of exports: A gravity model analysis of the Bangladeshi textile and clothing industries. FIIB Business Review, 8(3), 229–244. https://doi.org/10.1177/2319714519872643
- Rasoulinezhad, E. (2017). China’s foreign trade policy with OPEC member countries. Journal of Chinese Economic and Foreign Trade Studies, 10(1), 61–81. https://doi.org/10.1108/JCEFTS-09-2016-0027
- Rasoulinezhad, E., & Kang, G. S. (2016). A panel data analysis of South Korea’s trade with OPEC member countries: The gravity model approach. Iranian Economic Review, 20(2), 203–224. https://ier.ut.ac.ir/article_58799_cdd6d2fcb5efb7932f0ce589c2a4f4f5.pdf
- Rasoulinezhad, E., & Popova, L. (2017). An estimation of the impact of economic sanctions and oil price shocks on Iran-Russian trade: Evidence from a gravity-VEC approach. Iranian Economic Review, 21(3), 469–497. https://ier.ut.ac.ir/article_62926.
- Rasoulinezhad, E., & Wei, W. (2017). China’s trade with OPEC member countries: A panel-gravity model approach. The Chinese Economy, 50(5), 339–355. https://doi.org/10.1080/10599231.2022.2065565
- Rose, A. K., & Van Wincoop, E. (2001). National money as a barrier to international trade: The real case for currency union. American Economic Review, 91(2), 386–390. https://doi.org/10.1257/aer.91.2.386
- Santos Silva, J. M. C., & Tenreyro, S. (2006). The log of gravity. The Review of Economics and Statistics, 88(4), 641–658. https://doi.org/10.1162/rest.88.4.641
- Santos Silva, J. M. C., & Tenreyro, S. (2011). Further simulation evidence on the performance of the poisson pseudo-maximum likelihood estimator. Economics Letters, 112(2), 220–222. https://doi.org/10.1016/j.econlet.2011.05.008
- Saxena, R., Raman, M. S., Srivastava, S. K., Khan, A., & Kumar, R. (2023). Does India need a different rice ecosystem to harness the export advantages and manage the virtual water exports? Current Science, 124(4), 407–414. https://www.i-scholar.in/index.php/CURS/article/view/220935
- Schierhorn, F., Meyfroidt, P., Kastner, T., Kuemmerle, T., Prishchepov, A. V., & Müller, D. (2016). The dynamics of beef trade between Brazil and Russia and their environmental implications. Global Food Security, 11, 84–92. https://doi.org/10.1016/j.gfs.2016.08.001
- Semykina, A., & Wooldridge, J. M. (2013). Estimation of dynamic panel data models with sample selection. Journal of Applied Econometrics, 28(1), 47–61. https://econpapers.repec.org/RePEc:wly:japmet:v:28:y:2013:i:1:p:47-61 https://doi.org/10.1002/jae.1266
- Shahriar, S., Qian, L., & Kea, S. (2018). China’s economic integration with the Greater Mekong sub-region: An empirical analysis by a panel dynamic gravity model. Economics Discussion Paper, 44, 1–45. http://www.economics-ejournal.org/economics/discussionpapers/2018-44/file
- Shaiara, H., & Shanjida, Y. (2015). Does the gravity model explain Bangladesh’s Direction of Trade? A panel data approach. IOSR Journal of Economics and Finance (IOSR-JEF), 6(3), 01–14. https://doi.org/10.9790/5933-06320114
- Shepherd, B. (2019). Mega-regional trade agreements and Asia: An application of structural gravity to goods, services, and value chains. Journal of the Japanese and International Economies, 51, 32–42. https://doi.org/10.1016/j.jjie.2018.10.004
- Soeng, R., & Cuyvers, L. (2018). Domestic institutions and export performance: Evidence for Cambodia. The Journal of International Trade & Economic Development, 27(4), 389–408. https://repository.uantwerpen.be/docman/irua/91af78/146048_2019_04_14.pdf https://doi.org/10.1080/09638199.2017.1386230
- Sokvibol, Kea, Hua, Li, Saleh, Shahriar, Nazir, Muhammad Abdullahi, Samnang, Phoak, & Tharo, Touch. (2019). Factors Influencing Cambodian Rice Exports: An Application of the Dynamic Panel Gravity Model Emerging Markets Finance and Trade, 1–22. 10.1080/1540496X.2019.1673724
- Sreevidhya, K. S., & Elango, L. (2019). Temporal variation in export and import of virtual water through popular crop and livestock products by India. Groundwater for Sustainable Development, 8, 468–473. https://doi.org/10.1016/j.gsd.2019.01.002
- Suman, J., Shekhawat, P. S., Kharkwal, S., & Verma, D. K. (2022). Growth instability and comparative advantage in export of Indian Rice. International Journal of Social Sciences, 11(4), 213–218. https://doi.org/10.46852/2249-6637.04.2022.3
- Tamini, L. D., Doyon, M., & Simon, R. (2016). Analyzing trade liberalization effects in the egg sector using a dynamic gravity model. Canadian Journal of Agricultural Economics/Revue Canadienne D'agroeconomie, 64(2), 383–411. https://doi.org/10.1111/cjag.12084
- Thorbecke, W. (2015). China–US trade: A global outlier. Journal of Asian Economics, 40, 47–58. https://doi.org/10.1016/j.asieco.2015.09.001
- Thuong, N. T. T. (2018). The effect of sanitary and phytosanitary measures on Vietnam’s rice exports. EconomiA, 19(2), 251–265. https://doi.org/10.1016/j.econ.2017.12.001
- Tinbergen, J. (1963). Shaping the world economy. The International Executive, 5(1), 27–30. https://doi.org/10.1002/tie.5060050113
- Wang, J. (2016). Analysis of the factors influencing Japan’s soybean import trade: Based on gravity model. American Journal of Industrial and Business Management, 06(02), 109–116. https://doi.org/10.4236/ajibm.2016.62011
- Wei, G. X., Huang, J. K., & Jun, Y. (2012). Honey safety standards and its impacts on China’s honey export. Journal of Integrative Agriculture, 11(4), 684–693. https://doi.org/10.1016/S2095-3119(12)60056-2
- Xiong, B., & Chen, S. (2014). Estimating gravity equation models in the presence of sample selection and heteroscedasticity. Applied Economics, 46(24), 2993–3003. https://doi.org/10.1080/00036846.2014.920481
- Yadav, P. (2021). Globalization and India’s international trade: Does distance still matter? GeoJournal, 86(4), 1927–1941. https://doi.org/10.1007/s10708-020-10152-0
- Zhou, L., Li, L., & Lei, L. (2019). Avian influenza, non-tariff measures and the poultry exports of China. Australian Journal of Agricultural and Resource Economics, 63(1), 72–94. https://doi.org/10.1111/1467-8489.12285