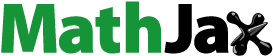
Abstract
The objective of this research is to assess the effect of holding government securities on the credit channel, taking into account the heterogeneity of WAEMU banks’ balance sheets. The methodology adopted to achieve this objective is VAR panel modelling, proposed by Abrigo and Love. The generalized moment method was applied to a sample of 95 commercial banks operating in the WAEMU covering the period 2000–2019. The main results show that a strong presence of government securities in banks’ balance sheets contributes to the reinforcement of the financial crowding-out effect in the WAEMU. This crowding-out effect differs from one category of bank to another. Indeed, the negative relationship between government securities and the credit channel is more pronounced among large banks, national banks and pan-African banks, in contrast to other bank categories in this Union. The results also point to an asymmetrical effect of monetary policy on the lending behaviour of WAEMU banks to private borrowers. However, the WAEMU monetary authorities should step up supervision of banks that hold a large proportion of sovereign debt on their balance sheets. The high proportion of sovereign debt on banks’ balance sheets creates situations of interdependence. This could have negative consequences on the solvency of banks and therefore on the stability of the banking system in the Union.
Impact statement
The aim of this research is to strengthen the existing literature on the transmission mechanisms of monetary policy. This research involves several players in the economic sphere: the Central Bank, commercial banks, WAEMU member states and the private sector (businesses and households). Indeed, it will attempt to provide a solution to the monetary authorities and regulators of the WAEMU area, by contributing to the effort to develop the public securities market and improve the financing of the economies of the Union’s countries.
Reviewing editor:
1. Introduction
The transmission channels of monetary policy are the links between the instruments used and the final objectives of the central bank, meaning the bank’s poor appreciation of these channels can lead to ineffective monetary policy. To achieve its objective, the Central Bank of West African States (BCEAO) passes through various channels (interest rates, credit, exchange rates, assets and expectations). However, not all monetary policy transmission channels are active in the West African Economic and Monetary Union (WAEMU). For Kanga (Citation2020), only the credit channel appears to be active in this union due to the size of the banking sector, the narrowness of the interbank market and the absence of a dynamic secondary market, which all limit the operation of the WAEMU's other monetary policy transmission channels (Kireyev, Citation2015). Also responsible for this limited channel use is the fixed exchange rate that the union’s monetary authorities apply.
To combat inflation more effectively, the BCEAO adopted a monetary policy that gradually eliminated direct loans to member states. Their prohibition in the BCEAO statutes since April 2010 has led the latter to favour direct market financing. As a result, the public debt market has gradually become the main source of financing for WAEMU countries. Issues of public debt securities have since increased 76-fold, from XOF 54.9 billion in 2001 to XOF 4191.6 billion in the first half of 2022. According to UMOA-TitresFootnote1 (2021), 93% of public securities issued by member states on the regional market are held by resident credit institutions, compared with 7% for other investorsFootnote2.
Moreover, government securities held by WAEMU banks are highly heterogeneous from one bank category to another. Indeed, shows a certain variability in government securities held by bank categories in the WAEMU. On average, large banks hold a higher proportion of government securities on their balance sheets than medium-sized and small banks, coming in at 15.9, 11.6 and 7.6%, respectively. Additionally, pan-African banks typically hold more government securities on their balance sheets than French banks (11.9 vs 10.4%, respectively). For domestic banks, this holding averages 10.4%, compared with 12.5% for foreign banks.
Figure 1. Average holding of public securities in the WAEMU (%). Source: Authors, bank balance sheet data 2000–2019 (BCEAO).

WAEMU member states generally turn to the regional public debt market to mobilize the resources needed to finance their budget deficits. Issues of government securities on this market vary from one country to another, depending on their financing needs. The shows the breakdown of outstanding government securities by country for the third quarter of 2023. During this period, Ivory Coast raised the most funds on the public debt market, with a total of 3,778 billion XOF, followed by Senegal with 2,958 billion XOF, Togo with 1,921 billion XOF, Mali with 1,882 billion XOF, Burkina Faso with 1,753 billion XOF, Benin with 1,324 billion XOF and Niger with 1,206 billion XOF. Guinea-Bissau, however, mobilized the lowest amount over the same period. This amounted to 322 billion XOF. There is a crowding-out effect. However, when a Member State issues securities, banks in other countries can also access them. Financial integration therefore reduces the crowding-out effect.
Figure 2. Breakdown of outstanding government securities by issuer (auctions) in Q3 2023 (in billions of XOF). Source: Authors, UMOA-Titres.

However, the increased presence of government securities on bank balance sheets could have consequences for the bank credit channel and ultimately for economic activity. The literature shows that holding government securities on bank balance sheets can lead to a contraction of credit in favour of the private sector (Bouis). Moreover, for these public securities, zero risk weighting is applied in the calculation of regulatory capital, and interest on income from these securities is tax-exempt. This could allow EU banks to concentrate public securities on their balance sheets until maturity, in the absence of a functioning secondary market for public debt (Kanga, Citation2020). According to the International Monetary Fund (IMF)Footnote3 in 2017, this high concentration of government securities could harm the development of the government securities market and the transmission of monetary policy.
Recent theoretical literature has placed the banking sector at the heart of the issues surrounding the presence of government securities on bank balance sheets. This literature highlights three possible situations. Firstly, when banks have excess liquidity, an increase in public debt on their balance sheets may not lead to a significant reduction in credit to the private sector. Secondly, if banks have access to safe public assets, this could allow them to take on more risk by increasing lending to the private sector. Thirdly, high levels of government lending may discourage banks from lending to the supposedly riskier private sector.
On an empirical level, work on the effect of banks’ holdings of public debt on the supply of credit has developed considerably in recent years. Highlighting banks’ exposure to sovereign risk as a key source of heterogeneity in the transmission of monetary policy to bank lending, Grandi (Citation2019) points out that banks with high sovereign exposure respond to monetary easing by reducing their lending, while those with low sovereign exposure tend to increase their bank lending. For a given sample of developed and developing countries, domestic public debt held by banks contributes to the reinforcement of the crowding-out effect (Haikal et al., Citation2021; Kabir & Flath, Pinardon-Touati). Authors such as Makambi et al. (Citation2017) and Laghman (Citation2022) find that, in the short term, domestic public debt stimulates credit to the private sector. However, in the long term, domestic public debt would generate a crowding-out effect on credit to the private sector. For his part, Lidiema (Citation2018) concludes that in the short and long term, domestic public debt has a negative and significant effect on capital formation.
However, the literature remains silent on the heterogeneous nature of bank balance sheets. The aim of this research is to dispel this silence in the existing literature. What’s more, we believe that this research is the first of its kind in the WAEMU. It involves several players in the economic sphere. These include the Central Bank, commercial banks, governments and the private sector (businesses and households). This article will attempt to provide a solution for monetary authorities and regulators in the WAEMU area, by contributing to the effort to develop the public securities market and improve the financing of the economies of the Union’s countries.
To alleviate this situation, this article seeks to assess the effect of holding government securities on the bank credit channel in a context of heterogeneity in the balance sheets of WAEMU banks. In this paper, we use a panel data vector autoregression (PVAR) approach based on the generalized method of moments (GMM) developed by Abrigo and Love (Citation2016) to achieve our research objective.
The remainder of this article is organized as follows: the second section gives the literature review, the third presents the method used, the fourth outlines the results and discussion, and the last section offers the conclusion.
2. Literature review
In the traditional literature on transmission channels, it is generally accepted that a monetary policy decision can affect interest rates (interest rate channel), share prices, exchange rate (other asset price channel) and the quantity of loans offered by commercial banks (credit channel). This last channel highlights the role of banks in the monetary policy transmission mechanism, as monetary policy decisions lead to a change in banks’ loan supply, insofar as these impulses affect banks’ access to loanable funds (Bernanke & Blinder, Citation1988).
The financial crisis of 2008 set the financial sector into turmoil. Low key interest rates led to banks’ excessive risk-taking. Since then, banks have had to cope with heavy exposure to public debt, which could affect their balance sheets and ability to extend credit to the private sector. According to Altavilla et al. (Citation2019), banks’ high exposure to sovereign risk can disproportionately increase their funding costs, forcing them to reduce their lending.
Recent theoretical work shows that this crowding-out effect depends on how banks behave when public debt rises, and how they modify their balance sheets. According to the literature, three situations can arise. First, when banks have excess liquidity, an increase in public debt on their balance sheets may not lead to a significant reduction in credit to the private sector. Second, if banks have access to safe public assets, this may allow them to take on more risk by increasing lending to the private sector (Chirwa & Mlachila, Citation2004; Kumhof & Tanner, Citation2005). Third, high levels of government lending may discourage banks from lending to the private sector, which is assumed to be riskier (Hauner, Citation2009).
Several empirical works have attempted to address the effect that holding public debt might have on the supply of bank credit. To this end, Albertazzi et al. (Citation2014) find that banks’ exposure to sovereign risk had a negative impact on bank lending in Italy, as it damaged banks’ balance sheets and increased their funding costs. As a result, Italian banks have reduced their lending more than their foreign competitors. Using the same data, Peydró et al. (Citation2017) reveal that during the sovereign debt crisis, undercapitalized Italian banks reacted to monetary easing by buying government securities rather than increasing credit supply.
Bofondi et al. (Citation2018) argue that the credit crunch is mainly the consequence of the increase in Italy’s country-specific sovereign risk and the generalized increase in the cost of funding for Italian banks. Similarly, Grandi (Citation2019) finds that banks with high sovereign exposure react to monetary easing by reducing their lending, while banks with low sovereign exposure tend to increase their bank lending. In a similar vein, Popov and Van Horen (Citation2015) point out that banks with higher sovereign exposure had lower participation in the syndicated loan market and higher lending rates than banks with lower exposure.
For Becker and Ivashina (Citation2018), the high holding of national public debt in the banking sectors of eurozone countries resulted in the crowding out of corporate lending during the 2010–2011 crisis period. In the same vein, De Marco (Citation2019) indicates that negative shocks to banks’ sovereign debt portfolios led to bank credit restrictions between 2010 and 2011. Analysing French government data from 2006 to 2018, Pinardon-Touati shows that increased bank- financed spending by local authorities reduced credit to the private sector; for this author, when a local authority borrowed an additional €1 from a bank, this reduced credit granted to the private sector by €0.5.
For his part, Al-majali (Citation2018) finds that in Jordan, government borrowing from domestic banks has a crowding-out effect on credit to the private sector. The author also concludes that treasury bills and government bonds on banks’ balance sheets reflect their preference to invest their surplus cash in low-risk, high-yield government securities. He refers to this type of behaviour as the ‘Lazy Bank’ approach. As for Ahmed (Citation2016), he argues that public borrowing negatively affects banks’ lending capacity, which he believes could harm Pakistan’s growth prospects.
Similarly, Fayed concludes that the Egyptian government borrowing from domestic banks crowds out private credit due to the presence of safe public assets on their balance sheets. Haikal et al. (Citation2021) went on to confirm the existence of the crowding-out effect in Egypt over the period 2009–2019. On a panel of 73 developed and developing countries covering the period 1995–2014, Kabir and Flath estimate that the rise in public debt held by banks will reduce credit to the private sector.
Hosono (Citation2006) measures the impact of the reaction of Japanese banks to monetary policy. The estimation results show that monetary policy has a greater impact on small, less liquid and less capital-intensive banks. The effects of monetary policy depend on the size of the bank, its policy stance and the evolutionary sectors of its debtors. Our research extends Hosono’s work by looking at the diversity of banks’ asset portfolios. It has been found that some WAEMU banks hold a large amount of government securities on their balance sheets. The reaction of banks to monetary policy may depend on the diversity of the bank portfolio.
In light of this review of both theoretical and empirical literature, we note that most studies have focused on the effect that banks’ public debt holding could have on credit to the private sector, albeit without emphasizing the heterogeneous nature of bank balance sheets. Some articles have focused on the balance sheet size effect. This paper attempts to fill this gap in the literature.
3. Method and data
This research uses a panel data vector autoregressive (PVAR) approach to achieve its objective. This section first presents the method and then the data itself.
3.1. Method
3.1.1. Specification of the PVAR model
To assess the effect of holding government securities on a bank’s credit channel, this article draws on the work of Abrigo and Love (Citation2016). The choice of PVAR lies in the fact that it determines no a priori restrictions on the exogeneity and endogeneity of variables. In addition, it captures both static and dynamic interdependencies. The PVAR model specification requires the assumption of specific fixed and temporal effects, taking the following form:
(1)
(1)
i = 1 …, 95 et t = 2000 …, 2019
with Yi,t the vector of endogenous stationary variables, Xi,t the vector exogenous covariates, γi,t the vector of bank- and period-specific effects, the matrices A1, A2, …, Ap−1 AP and B the parameters to be estimated and γi,t the vector of error terms.
In addition, the vector Yi,t is composed of 11 variables:
(2)
(2)
where i represents the bank, t represents the period, tmm denotes the average money market rate, credsp denotes customer credit, tpub denotes government securities, pnp denotes non- performing loans, liqb denotes bank liquidity, cap denotes bank capital, lsize denotes the logarithm of banks’ total balance sheet assets, ihh measures the Herfindahl-Hirschman banking concentration index, tpibh denotes the growth rate of gross domestic product per capita, txinf denotes the inflation rate and qreg denotes the quality of regulation.
3.1.2. Estimation method
Estimating the parameters of the PVAR model using the ordinary least squares method gives biased results when the time dimension is small, that is, ≤30, even with a large N dimension (Abrigo & Love, Citation2016). To correct this bias, Abrigo and Love (Citation2016) propose the use of the generalized method of moments (GMM) developed by Arellano and Bover (Citation1995).
Incorporating fixed effects also presents estimation problems, as they are correlated with lagged endogenous variables. Inspired by Arellano and Bover (Citation1995), Helmert’s procedure of differentiation via the mean of future observations is applied to eliminate unobservable individual effects. This method subtracts the mean of all available future observations for each bank and time to preserve the orthogonality between the transformed and lagged variables as instruments to estimate the system coefficients via the GMM (Love & Zicchino, Citation2006). This transformation preserves homoscedasticity and does not induce autocorrelation (Arellano & Bover, Citation1995). Prior to this transformation, the variables were centred by their annual averages over all the banks in our sample to eliminate temporal effects. Thus, the lagged values of the system variables represent good instruments for the model (Arellano & Bover, Citation1995).
3.2. Data presentation
The sample consisted of 95 commercial banks operating in the WAEMU from 2000 to 2019. However, the manually collected data were non-cylindrical due to entry/exit, and using an incomplete database can create bias in the quality of our results due to missing information. Faced with this risk, some researchers resort to different methods to overcome the problem of missing data (moving average, geometric average, arithmetic average, median, etc.). Even so, these methods pose considerable biases as well, as they do not take into account the uncertainty generated by the data themselves.
Faced with these limitations, Elasra (Citation2022) shows that multiple imputation based on the Markov Chain Monte Carlo method is globally better for handling missing data. According to this author, this approach consists of successively imputing several values to each missing data item. Several completed data sets are thus generated, respecting the characteristics of the distribution of observed data (variability and correlations between variables). Niass et al. report that for a dispersion of less than 20% between standard deviations on observed and imputed data, the multiple imputation method gives virtually unbiased estimates.
The data used come from several sources. The first is the BCEAO database, which provided us with statistics on credit to the private sector, government securities, non-performing loans, bank liquidity, bank capital, balance sheet size and the Herfindahl-Hirschmann index. The second is the World Development Indicators database, which provides statistics on GDP per capita growth and the inflation rate. The third is the Worldwide Governance Indicators database, which provides data on the quality of regulation in WAEMU countries ().
Table 1. Standard statistics (multiple imputation method).
4. Results and discussion
Before proceeding with the estimation of our model, it is necessary to take into account a few specificities related to panel data and the PVAR model. These are the inter-individual dependence test, the unit root test and the determination of the number of lags in the PVAR model.
Hurlin and Mignon (Citation2005) provide a very interesting discussion of unit root tests. The authors propose two generations of tests: first-generation tests (Im et al., Citation1997, Citation2003; Levin et al., Citation2002; Maddala & Wu, Citation1999) for independence between individuals, and second-generation tests (Bai & Ng, Citation2001; Choi, Citation2002; Moon & Perron, Citation2004; Pesaran, Citation2007) for dependence between individuals. To identify the best tests, we need to assess inter-individual dependence, as summarized in .
Table 2. Interindividual dependency test.
Overall, the null hypothesis of the individual dependence test is rejected. clearly shows the existence of interdependence between WAEMU banks. Consequently, the second-generation tests are the most appropriate for concluding on the stationarity of the variables. We used the second-generation unit root test proposed by Pesaran (Citation2007), as justified by the fact that among second-generation tests, Pesaran’s is the most powerful, as its results remain robust with modest sample sizes. The null hypothesis of this test is the non-stationarity of the variables.
Table 3. Pesaran (Citation2007) unit root test.
The results of Pesaran’s stationarity tests show that most variables are stationary at level except the variables credsp, tpub, lsize, pnp and qreg, which are integrated into order 1. However, using the PVAR model requires stationary variables. Consequently, these variables must be transformed into first differences, with d referring to the first-difference operator.
4.1. Estimation of the PVAR model using the GMM
We estimated our PVAR model using the PVAR package for Stata software, as proposed by Abrigo and Love (Citation2016). The first step in estimating a VAR panel is to determine the optimal number of lags to include in the model ().
Table 4. Optimal delay of the VAR panel model.
Given our large sample size, the maximum delay is limited to 3. The results indicate that the optimal delay to integrate into our PVAR model is (1). Upon determining the optimal number to include in the PVAR model, we need to estimate it using the GMM. However, given the large number of coefficients and the a-theoretical nature of the model, it would be wrong to give a theoretical interpretation to these coefficients ().
Table 5. Estimation of the PVAR model using the GMM.
As such, first we analyse the impulse response functions, then the variance decomposition of the prediction error. Finally, we test the robustness of the results.
4.2. Impulse response functions
Impulse response functions (IRFs) can be used to trace the dynamics of a variable following an impulse (shock) on another variable in the system. These simulations are carried out over a long period (10 periods). The aim is to analyse the response of the bank credit channel following a shock to the other variables in our model, for all WAEMU banks and in the context of their heterogeneous bank balance sheets.
4.2.1. IRF for all WAEMU banks
IRFs show that in the UEMOA banking system as a whole, the bank credit channel reacts negatively and significantly to a shock on government securities during the first period, then becomes slightly positive until the second period. It becomes negative again until the following period, before returning to its equilibrium level. shows that, overall, the reaction is negative and significant, which is similar to that of Hauner (Citation2006). The author shows that, faced with a high level of public debt, banks may have a preference for holding public securities on their balance sheets, which are more profitable and safer than credits granted to the private sector.
Table 6. Variance decomposition of forecast error (%).
IRFs show that a restrictive monetary policy that raises the average money market rate has a positive and significant impact on the bank credit channel. This result seems paradoxical, since in monetary theory, a rise in key interest rates has an impact on bank lending rates (cost of credit), which reduces the demand for credit from private borrowers. However, this result also suggests that the interest rate channel is not effective in the WAEMU. The response of the bank credit channel is also positive and significant following a shock to bank size in the WAEMU. Indeed, larger banks, thanks to their diversification, have access to financing on better terms. This enables them to extend more credit to their customers (Allen & Paligorova, Citation2015).
IRFs also show that the bank credit channel responds positively and significantly to a shock to the Herfindahl-Hirschman concentration index (). The response of the bank credit channel is positive and significant following a shock to the growth rate of GDP per capita. Thus, a rise in per capita income will increase demand for financial services. This will then lead to an improvement in banks’ intermediation activity. In contrast, the bank credit channel reacts negatively and significantly to a shock to bank liquidity in the WAEMU. This counterintuitive result can be explained by the fact that WAEMU banks use their excess liquidity not to finance economic activity, but rather to buy government securities, which are assumed to be safer and less risky (). The analysis also reveals that a shock to bank capital has a positive and significant effect on the bank credit channel in the WAEMU.
Figure 4. Responses of government securities and credit channel variables to a shock to bank liquidity. Source: Authors, based on BCEAO and World Bank data.

The bank credit channel reacts negatively to a shock to the inflation rate in the WAEMU. This corroborates the work of Huybens and Smith (Citation1998), who show that an increase in this variable could have negative consequences on financial development through friction on the credit market. However, a positive shock to non-performing loans has a negative yet insignificant effect on the bank credit channel in the WAEMU.
4.2.2. IRF by UEMOA bank size
show that a shock to government securities negatively affects the credit extended by large and small banks to the private sector; in turn, the credit channel response is positive for medium-sized banksFootnote4. A shock to the average money market rate also has a positive effect on the credit channel for medium-sized and small banks, while IRFs show a negative effect of the average money market rate on the credit channel for large banks. A positive shock to non-performing loans has a negative effect on the credit channel for large and medium-sized banks, while this effect is positive for small banks. This result means that, when faced with a rise in provisions for risks and charges, large and medium-sized banks tend to be cautious in their lending practices, unlike small banks.
The reaction of the credit channel to a shock to bank liquidity is positive for large and medium-sized banks. However, its effect on the credit channel is negative for small banks. A positive shock to bank capital, meanwhile, negatively affects the credit channel for large, medium-sized and small banks. This finding is contrary to the work of Allen and Paligorova (Citation2015), which indicates that the more capital a bank has, the more willing it is to absorb shocks that degrade its balance sheet, without reducing its credit volume. Conti et al. (Citation2023). These authors show that over the period 2011 and 2014, a positive shock to the capital acquired by Italian banks led to a reduction in the supply of loans and a temporary fall in Gross Domestic Product (GDP). A positive shock to the bank concentration index has a negative effect on the credit channel for large UEMOA banks. This result means that the higher this concentration, the higher the lending rates (Fungáčová et al., Citation2017). With regard to medium-sized and small banks, the results remain nuanced. The IRFs highlight a positive reaction of the bank credit channel following a positive shock to the bank concentration index during the first period, then becomes negative in subsequent periods before stabilizing.
The results also underline that an improvement in per capita income leads to an increase in demand for financial services from large and small banks. This further enables the latter to extend more credit to the private sector in the WAEMU. In contrast, a shock to the inflation rate negatively affects credit to the private sector among medium-sized and small banks. However, the results show a positive effect of the inflation rate on private sector credit among large banks.
4.2.3. IRF by type of shareholding in WAEMU banks
and show that a positive shock to government securities has a negative effect on the bank credit channel for both foreign and domestic banksFootnote5. The credit channel reacts negatively to a shock to the average money market rate at domestic banks during the first period, then the effect becomes positive in the following period. It becomes slightly negative again from the second to the third period, before stabilizing. For foreign banks, the reaction of the bank credit channel to a positive shock on the average money market rate is slightly negative in the first period, then becomes positive from the second period to the eighth period, before returning to its equilibrium level.
A positive shock to non-performing loans has a positive effect on the credit channel for both domestic and foreign banks. The reaction of the credit channel to a shock to bank liquidity is negative for foreign banks, whereas it is positive for domestic banks. Bank capital has a positive effect on the credit channel for both domestic and foreign banks.
The credit channel response to a shock to balance sheet size is positive for both domestic and foreign banks. A shock to the bank concentration index positively affects the credit channel for both domestic and foreign banks. With regards to macroeconomic variables, the results highlight that the growth rate of GDP per capita positively affects the credit channel for both domestic and foreign banks. The credit channel reacts negatively to a shock to the inflation rate for foreign banks, whereas it reacts positively for domestic banks.
4.2.4. IRF by banking group in the WAEMU
and show that a positive shock to government securities has a negative effect on the credit channel for both pan-African and French banks.Footnote6 This result shows that an increase in government securities in the portfolios of pan-African and French banks is associated with a decrease in credit to the private sector in the WAEMU.
The credit channel reacts negatively to a shock to non-performing loans for pan-African and French banks. A positive shock to bank liquidity negatively affects the credit channel for pan-African banks, whereas this effect is positive for French banks. This means that, unlike French banks, pan-African banks use their excess liquidity to invest in the WAEMU public securities market, to the detriment of the private credit market.
Positive shocks to bank capital, size, concentration index and GDP per capita growth rate have a positive effect on private sector credit for both pan-African and French banks. In addition, the response of credit to the private sector following a shock to the inflation rate is negative for both pan-African and French banks.
4.3. Variance decomposition of the forecast error
Variance decomposition allows us to determine, over a given period of time, how much of the variation in a system variable is explained by another system variable. For this purpose, we use the Cholesky decomposition method, which identifies the sensitivity of shocks based on the variance-covariance matrices of the previously estimated model. It also allows us to isolate the proportion of the variance of a given variable in the system that is explained by another variable in the system, all other things being equal. To simplify our analysis, the tenth period is used as a reference.
The decomposition analysis indicates that 79.34% of the variance in the forecast error of the private sector credit variable is explained by its own innovations, 5.61% by innovations in balance sheet size, 4.34% by innovations in government securities held by banks, 3.39% by innovations in bank liquidity, 2.25% by innovations in the average money market rate, 1.08%; 0.50% and 0.17% respectively by innovations in the Herfindahl-Hirschmann index, bank capital and non-performing loans. These results provide an important indication of the sensitivity of the bank credit channel to shocks to other variables. Indeed, balance sheet size, government securities and bank liquidity are the variables with the greatest impact on the variability of credit to the private sector in the WAEMU.
These results also show that holding government securities has a greater impact on private credit variability for large banks than for medium-sized and small banks. Monetary policy is more sensitive to the lending behaviour of small banks than large and medium-sized banks. In addition, non-performing loans have a more pronounced impact on the variability of credit to the private sector for large and medium-sized banks than for small banks. These results also show that higher concentration in the WAEMU banking system has a greater impact on the credit supply behavior of large banks than of medium-sized and small banks.
With regards to the type of bank shareholding, the results reveal that variables such as government securities, average money market rate and balance sheet size have a greater impact on the variability of credit to the private sector among domestic banks, whereas among foreign banks it is the variables balance sheet size, bank liquidity and GDP per capita growth rate that have a more pronounced impact. These results also underline that non-performing loans on the balance sheets of domestic banks are more sensitive to the variability of the bank credit channel than those present at foreign banks. This corroborates the work of Saidane et al. (Citation2021), which indicates that the average probability of domestic banks defaulting is higher than that of foreign banks. This contradicts market risk theory, which states that foreign banks bear more risk than domestic banks due to their poor knowledge of the remote management market.
This analysis also reveals that size, bank liquidity and government securities have greater impacts on private sector credit variability for pan-African banks, whereas for French banks, variables such as liquidity, size and bank capital have more pronounced impacts on private sector credit variability in the WAEMU. These results also show that risk-taking is higher among pan-African banks than among French banks. Saidane et al. (Citation2021) confirm this finding in telling that French banks have a lower default risk than pan-African banks, as French banks have been well-established since independence and have a better knowledge of the region than pan-African banks.
4.4. Testing the robustness of the results
To test the robustness of the various equations in our PVAR model, we added an institutional variable to determine whether or not the strengthening of governance in WAEMU countries changes the behaviour of banks regarding the supply of loans to private borrowers in this union. We focus on the variable quality of regulation in WAEMU countries. The results remain robust and reveal that a positive shock to the quality of regulation in the WAEMU has a positive and significant effect on the bank credit channel. Indeed, an improvement in the governance of institutions in WAEMU countries leads banks operating in the union to grant more loans to private borrowers. This result corroborates the theory of law and finance, which states that when a law protects the actors involved in the supply and demand of credit, then that law or regulation contributes significantly to financial development ().
5. Conclusion and policy implications
Table 7. Robustness of the PVAR estimation results.
The objective of this paper was to assess the effect of holding government securities on credit to the private sector in the WAEMU, taking into account the heterogeneity of banks’ balance sheets. This research used a PVAR approach based on the GMM proposed by Abrigo and Love (Citation2016), as applied to a sample of 95 commercial banks operating in the WAEMU from 2000 to 2019.
The main results show that a high presence of government securities on bank balance sheets contributes to the reinforcement of the financial crowding-out effect in the WAEMU. This crowding-out effect differs from one category of bank to another. Indeed, the negative relationship between government securities and the credit channel is more pronounced among large banks, national banks and pan-African banks, in contrast to other bank categories in the Union. The results reveal that the bank concentration index negatively affects the credit supply behavior of large banks. This means that the higher the concentration, the higher the lending rates set by the big banks.
The results also concluded that, faced with a rise in provisions for risks and charges, large banks, medium-sized banks, pan-African banks and French banks tend to be cautious in their lending practices, unlike other categories of banks in the WAEMU. The results also point to an asymmetric effect of monetary policy on the lending behaviour of WAEMU banks to private borrowers. Finally, the robustness results reveal that improved governance through good quality regulation promotes financial intermediation in the WAEMU.
These results have strong implications for economic policy. The high concentration of government securities on the balance sheets of WAEMU banks may create an interaction between fiscal stability and banking system stability. Thus, the default of one or more member states could present solvency risks for WAEMU banks, as they are not obliged to hold equity against the loans they grant to governments. In contrast, member states would find it difficult to finance their deficits in the event of instability in the national banking system. In addition, the concentration of government securities on bank balance sheets makes them less attractive to other investors such as pension funds, insurance companies, management and intermediation firms and international financial institutions. This could be detrimental to the development of the secondary market for public debt and the transmission of monetary policy in the WAEMU.
Disclosure statement
No potential conflict of interest was reported by the author(s).
Additional information
Notes on contributors
Ousmane Dieng
Ousmane Dieng holds a PhD in Economics from Cheikh Anta Diop University in Dakar. Ousmane's thesis is entitled ‘Bank Balance Sheet Heterogeneity and Monetary Policy Transmission Channels: The Case of the West African Economic and Monetary Union (WAEMU).’ Ousmane is an active member of the Laboratoire de Finances pour le Développement (LAFIDEV), whose research interests revolve around finance, monetary policy and sovereign debt.
Babacar Sene
Babacar Sene is Full Professor of Economics specializing in monetary and financial issues at Cheikh Anta Diop University in Dakar. Director of the Development Finance Laboratory (LAFIDEV) and Associate Editor of Finance Research Letters (Elsevier). He is the author of several articles published in journals such as Journal of International Financial Markets, Institutions and Money, Finance Research Letters, Discrete Dynamics in Nature and Society
Notes
1 The WAEMU public securities market.
2 Individuals, institutions, etc.
3 IMF Regional Economic Outlook Report for Sub-Saharan Africa (2017).
4 According to the classification proposed by the WAEMU Banking Commission, large banks have assets in excess of XOF 200 billion; medium-sized banks have assets between XOF 100 and 200 billion; and small banks have assets under XOF 100 billion.
5 Pasiouras and Kosmidou (Citation2007) classify a bank as foreign if foreigners hold more than 50% of its share capital, or domestic if nationals hold more than 50% of its share capital.
6 A bank is considered pan-African if the parent company is domiciled in Africa, or French if the parent company is domiciled in France.
References
- Abrigo, M. R., & Love, I. (2016). Estimation of panel vector autoregression in Stata. The Stata Journal: Promoting Communications on Statistics and Stata, 16(3), 778–804. https://doi.org/10.1177/1536867X1601600314
- Ahmed, J. (2016). Credit conditions in Pakistan: Supply constraints or demand deficiencies? The Developing Economies, 54(2), 139–161. https://doi.org/10.1111/deve.12106
- Albertazzi, U., Ropele, T., Sene, G., & Signoretti, F. M. (2014). The impact of the sovereign debt crisis on the activity of Italian banks. Journal of Banking & Finance, 46, 387–402. https://doi.org/10.1016/j.jbankfin.2014.05.005
- Allen, J., & Paligorova, T. (2015). Bank loans for private and public firms in a liquidity crunch. Journal of Financial Stability, 18, 106–116. https://doi.org/10.1016/j.jfs.2015.03.004
- Al-Majali, A. A. (2018). Crowding out effect of public borrowing: The case of Jordan. International Review of Management and Marketing, 8(1), 119–125.
- Altavilla, C., Canova, F., & Ciccarelli, M. (2019). Mending the broken link: Heterogeneous bank lending rates and monetary policy pass-through. Journal of Monetary Economics, 110, 81–98. https://doi.org/10.1016/j.jmoneco.2019.01.001
- Arellano, M., & Bover, O. (1995). Another look at the instrumental variable estimation of error- components models. Journal of Econometrics, 68(1), 29–51. https://doi.org/10.1016/0304-4076(94)01642-D
- Bai, J., & Ng, S. (2001). A consistent test for conditional symmetry in time series models. Journal of Econometrics, 103(1-2), 225–258. https://doi.org/10.1016/S0304-4076(01)00044-6
- Becker, B., & Ivashina, V. (2018). Financial repression in the European sovereign debt crisis. Review of Finance, 22(1), 83–115. https://doi.org/10.1093/rof/rfx041
- Bernanke, B. S., & Blinder, A. S. (1988). Credit, money, and aggregate demand (No. w2534). National Bureau of Economic Research.
- Bofondi, M., Carpinelli, L., & Sette, E. (2018). Credit supply during a sovereign debt crisis. Journal of the European Economic Association, 16(3), 696–729. https://doi.org/10.1093/jeea/jvx020
- Chirwa, E. W., & Mlachila, M. (2004). Financial reforms and interest rate spreads in the commercial banking system in Malawi. IMF Staff Papers, 51(1), 96–122. https://doi.org/10.2307/30035865
- Choi, I. (2002). Combination unit root tests for cross-sectionally correlated panels. Hong Kong University of Science and Technology.
- Conti, A. M., Nobili, A., & Signoretti, F. M. (2023). Bank capital requirement shocks: A narrative perspective. European Economic Review, 151, 104254. https://doi.org/10.1016/j.euroecorev.2022.104254
- De Marco, F. (2019). Bank lending and the European sovereign debt crisis. Journal of Financial and Quantitative Analysis, 54(1), 155–182. https://doi.org/10.1017/S0022109018000510
- Elasra,A. (2022). Multiple imputation of missing data in educational production functions. Computation, 10(4), 49. https://doi.org/10.3390/computation10040049
- Fungáčová, Z., Shamshur, A., & Weill, L. (2017). Does bank competition reduce cost of credit? Cross- country evidence from Europe. Journal of Banking & Finance, 83, 104–120. https://doi.org/10.1016/j.jbankfin.2017.06.014
- Grandi, P. (2019). Sovereign stress and heterogeneous monetary transmission to bank lending in the Euro area. European Economic Review, 119, 251–273. https://doi.org/10.1016/j.euroecorev.2019.07.011
- Haikal, G., Abdelbary, I., & Samir, D. (2021). ‘Lazy Banks’: The case of Egypt. Macroeconomics and Finance in Emerging Market Economies, 16(3), 447–457. https://doi.org/10.1080/17520843.2021.1998743
- Hauner, D. (2009). Public debt and financial development. Journal of Development Economics, 88(1), 171–183. https://doi.org/10.1016/j.jdeveco.2008.02.004
- Hauner, M. D. (2006). Fiscal policy and financial development (No. 6-26). International Monetary Fund.
- Hosono, K. (2006). The transmission mechanism of monetary policy in Japan: Evidence from banks’ balance sheets. Journal of the Japanese and International Economies, 20(3), 380–405. https://doi.org/10.1016/j.jjie.2005.07.003
- Hurlin, C., & Mignon, V. (2005). Une synthèse des tests de racine unitaire sur données de panel. Économie & prévision, n° 169–171(3), 253–294. https://doi.org/10.3917/ecop.169.0253
- Huybens, E., & Smith, B. D. (1998). Financial market frictions, monetary policy, and capital accumulation in a small open economy. Journal of Economic Theory, 81(2), 353–400. https://doi.org/10.1006/jeth.1997.2372
- Im, K. S., Pesaran, M. H., & Shin, Y. (1997). Testing for unit roots in heterogeneous panels. Indiana University and University of Cambridge.
- Im, K. S., Pesaran, M. H., & Shin, Y. (2003). Testing for unit roots in heterogeneous panels. Journal of Econometrics, 115(1), 53–74. https://doi.org/10.1016/S0304-4076(03)00092-7
- Kanga, D. (2020). Les Banques et la Transmission de la Politique Monétaire dans l‘Union Économique et Monétaire Ouest-Africaine. Document de politique générale (No. 677). AERC.
- Kireyev, M. A. (2015). How to improve the effectiveness of monetary policy in the West African Economic and Monetary Union (No. 15-99). International Monetary Fund. https://doi.org/10.5089/9781484366646.001
- Kumhof, M. M., & Tanner, M. E. (2005). Government debt: A key role in financial intermediation (No. 5-57). International Monetary Fund.
- Laghmam, F. Z. (2022). Assessing the impact of domestic public debt on bank credit to the private sector. International Journal of Accounting, Finance, Auditing, Management and Economics, 3(1-2), 458–470. https://doi.org/10.5281/zenodo.5910291
- Levin, A., Lin, C. F., & Chu, C. S. J. (2002). Unit root tests in panel data: asymptotic and finite-sample properties. Journal of Econometrics, 108(1), 1–24. 7 https://doi.org/10.1016/S0304-4076(01)00098-
- Lidiema, C. (2018). Intra-market linkages in the financial sector and their effects on financial inclusion (No. 28). KBA Centre for Research on Financial Markets and Policy Working Paper Series.
- Love, I., & Zicchino, L. (2006). Financial development and dynamic investment behavior: Evidence from panel VAR. The Quarterly Review of Economics and Finance, 46(2), 190–210.https://doi.org/10.1016/j.qref.2005.11.007
- Maddala, G. S., & Wu, S. (1999). A comparative study of unit root tests with panel data and a new simple test. Oxford Bulletin of Economics and Statistics, 61(S1), 631–652. https://doi.org/10.1111/1468-0084.0610s1631
- Makambi, S., Muhindi, R., & Nduku, G. (2017). Influence of bank lending to the government on private sector credit in Kenya: A fiscal deficit specification (No. 18). KBA Centre for Research on Financial Markets and Policy Working Paper Series.
- Moon, H. R., & Perron, B. (2004). Testing for a unit root in panels with dynamic factors. Journal of Econometrics, 122(1), 81–126. https://doi.org/10.1016/j.jeconom.2003.10.020
- Pasiouras, F., & Kosmidou, K. (2007). Factors influencing the profitability of domestic and foreign commercial banks in the European Union. Research in International Business and Finance, 21(2), 222–237. https://doi.org/10.1016/j.ribaf.2006.03.007
- Pesaran, M. H. (2007). A pair-wise approach to testing for output and growth convergence. Journal of Econometrics, 138, 312–355. https://doi.org/10.1016/j.jeconom.2006.05.024
- Peydró, J. L., Polo, A., & Sette, E. (2017). Monetary policy at work: Security and credit application registers evidence. Journal of Financial Economics, 140(3), 789–814. https://doi.org/10.1016/j.jfineco.2021.01.008
- Popov, A., & Van Horen, N. (2015). Exporting sovereign stress: Evidence from syndicated bank lending during the euro area sovereign debt crisis. Review of Finance, 19(5), 1825–1866. https://doi.org/10.1093/rof/rfu046
- Saidane, D., Sène, B., & Kanga, K. D. (2021). Pan-African banks, banking interconnectivity: A new systemic risk measure in the WAEMU. Journal of International Financial Markets, Institutions and Money, 74, 101405. https://doi.org/10.1016/j.intfin.2021.101405