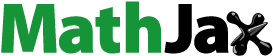
Abstract
This paper documents the different debt maturity choices of firms by allowing debt heterogeneity. We use a sample of US companies’ capital structure and employ dynamic panel regressions and Instrumental Variable approach. When taking the maturity of each type of debt into account, the researcher fails to validate the non-linear relationship between credit quality (firm size) and debt maturity predicted by Diamond in Citation1991. This study finds a non-linear relationship between revolving credit, term loan, and capital leases but does not find the same relationship with bonds and notes or trust preferred securities. Also, this research finds that different types of debt are explained differently by existing debt maturity theory such as information asymmetry, agency cost of debt, signaling, tax, matching asset maturity, and timing the market hypothesis.
Impact statement
The findings of this paper shed light on the intricate dynamics of debt maturity choices among firms, challenging existing theoretical frameworks. By meticulously examining a diverse array of debt instruments within US companies’ capital structures, the study unveils a nuanced non-linear relationship between credit quality (proxied by firm size) and debt maturity, contrary to Diamond’s seminal work. Notably, the research delineates varying patterns across different types of debt, revealing distinctive relationships for revolving credit, term loans, and capital leases compared to bonds, notes, or trust preferred securities. Moreover, the study enriches our understanding of debt maturity determinants by dissecting the influence of factors such as information asymmetry, agency costs, signaling, tax considerations, asset maturity matching, and market timing hypotheses. These findings not only contribute to the refinement of debt maturity theories but also offer valuable insights for practitioners navigating capital structure decisions in dynamic economic landscapes.
REVIEWING EDITOR:
1. Introduction
One important aspect of a firm’s financing decision is whether to take on short-term or long-term debt. A firm might opt for short-term debt if it anticipates enough earnings in the near future to pay off the debt or choose long-term debt if the long-term interest rate is low now. Previous studies have tried to find out what factors influence the debt maturity choice, but they have not accounted for the fact that different kinds of debt may behave differently. This article examines the characteristics of maturity in different types of debt for U.S. industrial firms. Using a sample of firms that have different debt structures from 2001 to 2012 and extending it till 2021, this article provides evidence about the demand-side and supply-side factors that affect the maturity of commercial paper (CP), (drawn) revolving credit (RC), term loans (TL), bonds and notes (BN), capital leases (CL), trust preferred (TP), and other debt (Other).
Our analysis produces three sets of results. First, the empirical analysis shows that firms select the maturities of RC and TL in line with Diamond’s (Citation1991) nonlinear prediction between firm size and maturity. Bigger firms are more likely to have long-term RC, TL, and BN. On the other hand, bigger firms tend to have shorter debt maturities when they use CL and TP, which is consistent with information asymmetry theory. Except for TP, firms with more R&D spending have shorter debt maturity, which is also consistent with information asymmetry. However, these types of firms are less likely to have CP. Lastly, this study shows that debt maturity is also influenced by credit-rating constraints, which is a supply-side factor. But it does not find any evidence that the choice of debt maturity was affected by the recent financial crisis.
Our article adds to several strands of literature. First, it adds to the literature on capital structure theory on debt maturity (Brick & Ravid, Citation1985; Diamond, Citation1991; Flannery, Citation1986; Myers, Citation1977) by testing the theories on debt heterogeneity. Second, it adds to the literature on capital structure empirics on debt maturity (e.g., Graham & Harvey, Citation2002; Custódio et al., Citation2013; Faulkender & Petersen, Citation2006; Rauh & Sufi, Citation2010). Lastly, it adds to the literature on debt heterogeneity (Colla et al., Citation2013; Lou & Otto, Citation2020; Rauh & Sufi, Citation2010). In this article, we test theories on debt maturity and see if the same theories apply to different types of debts. In summary, our contribution to literature is that we find that different debt maturity theories apply to different types of debt.
We structure the article in the following way: Section 2 reviews the literature; Section 3 explains the data and methodology; Section 4 reports the findings of the overall analysis of debt maturity; Section 5 shows the outcomes of debt maturities with debt heterogeneity; Section 6 displays the results of supply-side factors; Section 7 conducts sensitivity tests; and lastly, Section 8 summarizes.
2. Literature review
This section discusses the hypotheses that follow the existing literature on the factors that influence debt maturity to examine how firms choose debt maturity based on their own characteristics.
2.1. Theory
Diamond (Citation1991) suggests that there is a non-linear (i.e., an inverse U-shape) relationship between credit quality and maturity, which means that small, medium, and large firms tend to have short, long, and short-term debt, respectively. He expects that large firms will use short-term debt because they have less liquidity risk and try to show their high quality to the market. On the other hand, small firms will also use short-term debt since lenders are reluctant to lend long-term debt due to possible default risk. Another reason that small firms use short-term debt is to imitate high-quality firms. Medium firms are more likely to have long-term debt due to the liquidity risk of short-term debt.
The theory of agency cost of debt suggests that market-to-book ratio has a negative coefficient, meaning that firms with more investment opportunities use short-term debt to reduce the underinvestment problem (Myers, Citation1977).Footnote1 This is because debt maturity comes before the expiration of the growth option. A comprehensive survey by Graham and Harvey (Citation2002) shows that the main factor in choosing debt maturity is to match the maturity of debt and assets, which is consistent with Myers’s (Citation1977) theory of agency cost of debt. The agency cost theory (Myers, Citation1977) also indicates that firms with more leverage are more likely to use short-term debt because it enables frequent renegotiation. However, Diamond (Citation1991) argues that firms with high leverage tend to favor long-term debt to avoid excessive liquidation and, therefore, expects a positive coefficient on leverage.
Signaling theory suggests that a negative correlation exists between abnormal earnings and debt maturity. Specifically, firms with superior investment prospects are likely to opt for short-term debt as a means of signaling their robust quality within the market (Flannery, Citation1986). Brick and Ravid (Citation1985) posit that a widening term spread—the gap between the yields of long-term (10-year government bonds) and short-term (1-year government bonds) rates—encourages firms to increase their long-term debt holdings, largely due to the tax advantages associated with long-term debt.
2.2. Empirics
Dangl and Zechner (Citation2021) find that when the firm’s profitability is low, equityholders have no incentive to reduce debt. By contrast, short debt maturities create more flexibility to reduce leverage in bad states. Alnori (Citation2021) finds that the relationship between multinational corporations’ profitability and their leverage ratios is U-shaped and nonlinear, while this nonlinear relation does not exist for domestic firms. Ben-Nasr et al. (Citation2021) show that major board reforms lead to a decrease in bank debt, particularly in companies where bank debt is used for monitoring purposes, suggesting that bank debt and board reforms are substitutes for monitoring managers’ actions. Awartani et al. (Citation2016) find that firms in Middle East and North Africa region are less likely to use long-term debt. Belkhir et al. (Citation2016) show that firms located in countries where labor enjoys a strong protection tend to borrow more short-term. Ben-Nasr et al. (Citation2015) find evidence that firms with multiple large shareholders exhibit shorter debt maturity. Boubaker et al. (Citation2019) find that firms with better higher employee welfare prefer long-term debt over short-term debt.
3. Data
3.1. Capital structure debt database
We use data from the Capital Structure Debt Database provided by Standard & Poor’s Capital IQ. This database has debt structure information for U.S. firms that are public or private on a yearly, half-yearly, or quarterly basis. We focus on data collected yearly. The data point is a combination of a firm-debt issue–end date of financial reporting period. There may be several entries for the same debt issue–date combination for a given firm because the relevant information may be reported in several financial statements.
We clean the data in five ways. First, we only keep records with a Compustat unique identifier (gvkey) for merging and with debt maturity information (maturityyearhigh). Second, we only keep records with debt issued in US Dollar (USD). Third, we use the most recent filings for a debt issue when there are multiple filings on the same date (latestfilingforinstanceflag = 1). Fourth, we remove duplicate records as follows: We find duplicate debt items using company identifier (gvkey), report date (periodenddate), debt type (capitalStructureSubTypeId), data description (descriptiontext), priority level (leveltypeid), interest rate information (interestratetypeid, interestratehighvalue, interestratebenchmarktypeid, benchmarkspreadhighvalue), principal amount (dataitemvalue), and dollar unit type (unittypeid) and then manually delete extra items. Fifth, we merge the Capital IQ data with Compustat data for the same reporting period end date. We only keep data for the dates (PERIODENDDATE) that match the dates of Compustat Annual data set (DATADATE).
3.2. Compustat database
We use the Compustat Industrial Annual database to select our sample of U.S. firms. The sample period is from 2001 to 2021. We leave out foreign incorporated firms as well as financial firms (SIC codes 6000-6999) and utilities (SIC codes 4900-4999) because these firms are more likely to have different capital structures due to governmental regulation. We also exclude any observations with negative or missing values of total assets; missing values of total debt (dlc + dltt); and missing values of market capitalization (csho*prcc_f). We then combine the resulting sample of the Compustat firms with Capital IQ and exclude observations in Capital IQ data whose “maturity year high” is 9999 (no maturity date available in document), 8888 (perpetual), or 7777 (default – maturity date has passed, but it is not known when debt will be repaid). We also remove observations with negative debt maturity and those with zero principal due. Our sample consists of 77,411 observations at firm-debt type-year level from 5040 unique firms.
3.3. Debt structure
Capital IQ provides information on different kinds of debt: commercial paper (CP), (drawn) revolving credit (RC), term loans (TL), bonds and notes (BN), capital leases (CL), trust preferred (TP), and other debt (Other). Capital IQ gives us data about each debt component, such as security type, secured level, interest rate, maturity date, interest type, benchmark, secured flag, convertible type, issued currency, and benchmark spread. In this article, we focus on the debt type and maturity date to find out the debt maturity of each kind of debt.
3.4. Debt maturity
By using Capital IQ, we can access the maturity year of all debt types. This allows us to study the actual maturities of debt instead of using an approximation based on the percentage of debt with short- or long-term maturity, as most prior studies on debt maturity do. Stohs and Mauer (Citation1996) use actual maturity to research debt maturity, but their data only go up to 2000. Saretto and Tookes (Citation2013) also use actual maturity to explore debt maturity, but they do not report how debt maturity varies across different debt types. Therefore, we believe that our article is the first paper to employ the actual maturity year to analyze the debt maturity choice for different types of debt.
We define debt maturity as the maturity year minus filing year from Capital IQ.Footnote2 We use the definition of debt maturity from Stohs and Mauer (Citation1996) and Saretto and Tookes (Citation2013), with a small modification, to measure how long each component of debt lasts. The definition is based on the idea that firms have to report their debt on 10-K filings when they take it out. So, we use the filing year as an estimate of when the debt maturity begins. The main outcome variable we use is the principal-weighted average of maturity each year for each type of debt. The formula for calculating the principal-weighted average of maturity of type of debt for firm at time is:
(1)
(1)
where
is the number of
type of debt at time
for firm
are the maturities of
type of debt, and
where
is the principal due of each
component of debt type
in the Appendix shows a screenshot of data provided by Capital IQ indicating how we calculate the principal-weighted average of maturity.
shows the summary statistics of the debt maturity samples for different kinds of debt. We have winsorized the weighted debt maturity at the 1st and 99th percentile. Panel A displays the summary statistics. The table implies some key observations. First, the median of the weighted debt maturity of all sample data is 2 years and the mean is 3.26, which means that on average, firms borrow debts with a maturity of 3 years. The maturity also varies significantly with standard deviations of 3.90 over our sample period. Second, we observe different maturities in different debt types (debt heterogeneity). The BN and TP maturity is quite long, with a mean of 5.53 and 4.28 years. The fact that most of the zero debt maturity was found in CP means, as expected, the maturity is less than one year. Therefore, we can think of CP and BN/TP as short-term and long-term debt, respectively. Third, the most frequent debt structures are BN, RC, and TL. We will use the winsorized weighted debt maturity as our dependent variable for all the following regressions.
Table 1. Summary statistics.
3.5. The evolution of debt heterogeneity and maturity
, Panel A shows how debt maturity changed based on debt heterogeneity of the U.S. industrial firms from 2001 to 2021. The median debt maturity for all firms was 4 years in 2001 and 3.75 years in 2021. The amount of total debt outstanding increased over time and reached its highest point in 2021. The number of CP programs outstanding went down from 67 in 2001 to 18 in 2021. The number of RC programs rose from 734 in 2001 to 1014 in 2021. The biggest part of debt structure in US firms is BN, which went down from 1337 in 2001 to 974 in 2021. The order of debt outstanding in 2021 is CL, RC, BN, and TL, respectively.
Table 2. Debt maturity of different kinds of debt by year.
also shows how debt maturity changed for different debt items. Our data shows a lower debt maturity of BN. BN has the biggest fall in its maturity, going down from 4.09 years in 2001 to 3.43 years in 2021. CL has a higher maturity, going up from 0.84 years in 2001 to 1.03 years in 2021. But TL and RC stayed the same during this period.
To see if debt maturity decreased clearly during this period, we use ordinary least square (OLS) regressions of debt maturity for each kind of debt on time trend in Panel B. We test trends by year by adding time fixed effects (i.e. i.year) that will estimate a coefficient for each year. These variables will capture time-specific variation. All the coefficients for the total debt maturity are positive and significant until 2014. After that, it shows a falling trend until 2021. So, we find a statistically significant drop in recent years in all debt maturity variables consistent with previous literature (Custódio et al., Citation2013).
Mostly BN and CL are responsible for the lower maturity trend. But other types of debt do not show this trend of lower maturity. RC has gone up a lot over time. BN goes up from 2003 to 2014 and then goes down until 2021. The average maturity of CL went down lately. Others have gone down for the past ten years. So, we can say that the maturity of most corporate debt has gone down, except for RC and CL.
4. The demand-side determinants of aggregate debt maturity
4.1. Baseline results
We first run a number of panel regressions for each firm-year to study how the length of debt repayment in different debt choices depends on firm features. Our dependent variable is the same as before, our winsorized principal-weighted debt maturity in all regression models.
shows coefficients from various regressions on the overall debt maturity. The first column includes firm characteristics related to the choice of debt maturity: the size, proxied by the ln(assets),Footnote3 the size squared, market-to-book ratio, abnormal earnings, asset maturity, asset volatility, R&D and term spread. In addition, we analyze the decision of debt maturity by adding a measure of market leverage (Alnori, Citation2021) (leverage) into the regression. The coefficient of Leverage is negative and statistically significant, which implies a negative relation between market leverage and debt maturity.
Table 3. Regression in principal-weighted debt maturity.
in the Appendix explains what each variable means. The first column’s model has firm controls, and the second column has year dummies and does not have term spread because the yearly term spread is included in the year dummies. The model in column (3) adds industry dummies to the model in column (1). The model in column (4) has both year and industry dummies. The standard errors are clustered by the firm. We use firm size as a measure of credit quality, calculated by the ln(Total Assets) as in Barclay et al. (Citation2003), Johnson (Citation2003), Datta et al. (Citation2005), Billett et al. (Citation2007), and Brockman et al. (Citation2010). The coefficients in reveal some interesting results of Diamond’s prediction. First, we find the coefficient of firm size to be negative and very significant, which is consistent with our expectation that large firms have shorter debt. We also find the coefficient of size squared to be positive in our regressions. We will examine this finding in detail by using credit rating itself in the robust check.
Regarding the agency cost of debt theory, we see a positive and significant market-to-book coefficient in column (4). We do not find any support for signaling theory and agency cost of debt theory. We find consistent results of matching maturity of assets and debt, showing positive and significant coefficients of asset maturity. The negative and significant coefficient of asset volatility suggests that as asset volatility increases, indicating a higher chance of default, firms have shorter maturity debt. This is because the market observes the chance of default, and as a result, declines to offer long-term debt due to higher default risk.
The agency cost theory (Myers, Citation1977) proposes that firms with higher leverage usually have short-term debt because it enables regular renegotiation. On the other hand, Diamond (Citation1991) argues that firms with high leverage tend to choose long-term debt to avoid excessive liquidation and, therefore, expects a positive coefficient on leverage (Leverage). Our finding supports Myers’s proposal, we find that firms with higher leverage tend to have short-term debt which is significantly consistent in all our regression models.
As column (5) shows, firms that spend more on R&D usually have debt that matures faster, which fits with the idea that information asymmetry affects their financing choices, but we don’t see much evidence for this in our OLS model. Brick and Ravid (Citation1985) suggest that when the gap between long-term rate (10-year government bonds) and short-term rate (1-year government bonds) widens, firms prefer more long-term debt because they can benefit more from the tax deduction of long-term debt. Our result agrees with this suggestion.
We add year dummy variables to determine if the aggregate debt maturity increases or decreases over time, controlling firm characteristics and industry. Even though we do not tabulate the results, the OLS models in column (4) indicate that debt maturity overall increases during the sample period. We find more significance in the increasing trend after controlling for the unobservable firm heterogeneity described in the fixed effect model of (5).
4.2. Additional results
In this section we present several robustness tests on the regression results in . We add more variables to the regression specifications of .
One possible issue with our earlier results is that there may be other factors that influence debt maturity choice. We repeat the OLS regressions with several more factors: rating dummy; taxes; the return on asset (ROA) to measure profitability; cash; plant, property and equipment (PPE) to measure tangibility; dividend dummy; institutional ownership; analyst coverage; and Amihud illiquidity (Amihud, Citation2002). We define these factors in in the Appendix.
shows the results of OLS regressions that include the extra explanatory variables from Table 3's models. The credit rating coefficient is positive, implying that rated firms tend to have debt with long-term maturity. The Taxes, ROA, Cash, PPE, and dividend dummy coefficients are significant and positive, which means that firms with high taxes, profitability, cash holding, tangibility, and positive dividends and therefore, less information asymmetry, tend to use long-term debt.
Table 4. Regression with additional firm characteristics and economic variables.
also incorporates other economic factors in the benchmark model to see how debt maturity responds to different economic situations (Baker et al., Citation2003). As the real short-term rate rises, short-term debt becomes less attractive because of higher borrowing costs, in line with firms’ abilities to time the market. A rise in inflation rate is linked to a rise in debt maturity. The default spread and recession dummy both have positive and significant coefficients, suggesting that when the economy worsens firms tend to have long-term debt. The coefficient of bank market shows that when banking industry conditions improve, firms have more long-term debts. These regressions, with additional variables, address a concern about different results when using debt maturity itself as a dependent variable. Some of our findings are not consistent with prior literature, which uses the proportion of long-term debt to total debt as a dependent variable.
5. The demand-side determinants of the different types of debt maturity
In this section, we identify the determinants of debt maturity by analyzing debt heterogeneity (different debt behaves differently). We also analyze the choice between bank debt and bond debt (Ben-Nasr et al., Citation2021). Bond debt appears in Bonds and Notes (BN), and bank debt is assumed to be the rest of debts. We use OLS regressions of debt maturity weighted by principal on the explanatory variables that are identical to column (3) in .
5.1. Regression results
shows the results for CP in column (1). We use a logit regression because the commercial paper has a maturity of less than one year. This way, we can see what factors influence whether a firm issues commercial paper, or short-term debt. The dependent variable is one if the firm has CP and zero otherwise. For instance, the size coefficient is positive and significant, meaning that bigger firms have CP. Firms with higher market-to-book ratios also tend to have CP, meaning that firms with good investment opportunities have CP. Moreover, firms with more debt might not like CP. Therefore, CP is preferred by large-sized firms with less leverage.
Table 5. Regression in different debt structure.
Column (2) shows some important findings of how firm characteristics affect maturity in RC, TL, and CL. The size and size squared coefficients are positive and negative, respectively, which implies a non-linear relationship between firm size and maturity proposed by Diamond (Citation1991). Firms with RC and CL try to match the maturity of their assets and liabilities. Higher leverage makes firms have shorter RC maturity. There is a positive link between term spread and maturity of RC, BN, and CL, which is consistent with the tax hypothesis. In general, we find similar determinants between RC and CL.
For BN and TP, there is a U-shape and nonlinear relationship between size and leverage that is statistically significant, but this relationship does not exist for other kinds of debts. For RC, TL, and CL, however, the relationship between size and leverage is a reverse U-shape and nonlinear. Firms with higher default risk have shorter maturity because they cannot access the long-term debt market. The results in column (5) show that larger firms have shorter maturity CL. Also, firms with better investment opportunities have longer maturity. In economic terms, for BN, the −0.36 coefficient indicates that a 1% increase in size decreases debt maturity of Bonds and Notes by 0.36 years. While for one of bank debt, TP, the −13.39 coefficient indicates that a 1% increase in size decreases debt maturity of TP by 13 years.
The results for long-term debt, TP security, are reported in column (6). Larger firms have shorter maturity TP, which is consistent with the information asymmetry hypothesis. The coefficient of abnormal earnings is positive and significant in regression of BN, implying that firms with better quality projects tend to issue bonds with longer maturities. Similar to RC, when issuing CL, firms take asset maturity into account. When term spread is large, a debt is issued with a longer maturity.
In summary, we find clearly different debt maturity choices in different debt types. CP is a short-term debt and TP is a long-term debt; while other types of debt allow firms to choose a range of maturities from one to ten years, on average. We document similar patterns in the choices of maturity with RC, TL, and CL overall. Also, we find different evidence of a non-linear relationship predicted by Diamond (Citation1991). Thus, we can conclude that existing literature, which found a non-linear relation, could possibly be driven by the properties of RC, TL, and CL.
5.2. Additional results
We run probit regressions in place of our logit regressions for CP issuance with industry dummies; these results are reported in column (1) in Panel B of . We find similar signs of size, size squared, market-to-book, and R&D coefficients. We also run panel fixed effect to control for time invariant unobserved firm heterogeneity. In terms of size and size squared, we find an inverse U-shape relationship between RC maturity and firm size, while a U-shape relationship between BN maturity and firm size.
We also examine the determinants of debt maturity, including several additional variables in the regressions in Panel A of . For CP, we add the variables to the explanatory variables in logit regression, and the results are shown in column (1) in . Firms with ratings are more likely to use CP, which confirms that CP is only accessible for firms with excellent credit ratings. Firms that are profitable and pay dividends tend to use CP. Furthermore, firms with less cash and less institutional ownership use CP.
Table 6. Additional variables in different types of debt.
The OLS regressions results are shown in column (2) to column (7). Cash-rich firms have long-term TL, BN, and CL, and firms with more tangible assets have long-term CL. Dividend-paying firms favor long-term RC and BN. Firms with high institutional ownership lean toward long-term RC. In terms of the economic impact on debt maturity, firms have short-term RC and TL and long-term BN when inflation is high. During recession, firms choose long-term BN and CL and short-term RC and TL. We find that firms tend to have more CP and long-term BN when banking industry does well.
These results show that the maturities of different debt instruments depend on different factors. We do not find any single factor that influences the maturity choice of RC, TL, BN, and CL. However, looking only at the sign of the coefficients, we see that rated firms, firms with more cash, more profitable firms, or firms in a higher inflation rate economy, tend to have longer maturity of BN.
6. The supply-side determinants of the different types of debt maturity
In this section, we examine how different factors on the supply side influence the maturity of various kinds of debt. The choice of debt maturity depends not only on the demand side factors (firm-specific preferences), but also on the supply-side factors (credit market conditions), as suggested by Faulkender and Petersen (Citation2006) and Rauh and Sufi (Citation2010). This means that some lenders are more reluctant to offer long-term debt to certain kinds of firms (usually those with low credit quality). As a consequence, those kinds of firms realize that some kinds of debt with longer maturities are too costly, so they opt for short-term debt which is relatively cheap.
The most likely constraint to firms in supply-side is credit quality. Low quality and unrated firms can be ruled out of the long-term debt market. We define rated firms as the firms that have an S&P domestic long-term issuer credit rating. In and , we show that credit rating is a crucial determinant of firms’ choice of debt maturity. Unrated firms incur debt with shorter maturities than rated firms on average across all kinds of debt. This is consistent with our findings in Panel A of , where the rating dummy has a negative and significant coefficient. We also acknowledge that unrated firms differ from rated firms in many ways, and these differences could impact debt maturity choices.
Table 7. Credit rating on debt maturity.
shows the OLS regression results for both rated and unrated firms. Panel A of displays the estimated coefficients for rated firms. We observe a nonlinear relationship between firm size and BN maturity. That is, rated firms that are small, medium, and large, tend to have long, short, and long maturity respectively. For unrated firms, we observe a nonlinear relationship with RC, TL, and CL. That is, unrated firms that are small, medium, and large, tend to have short, long, and short maturity respectively. Based on this evidence, we can infer that different debt maturities among firms are somewhat affected by a supply effect, namely the rating effect.
Table 8. Regression results.
Another supply factor that affects debt maturity decisions is economic or financial crisis. To account for this effect, we add a No-crisis dummy that is one if the time period is not 2007/2008, and zero otherwise, to our baseline model. We expect that the recent financial crisis, when the CP and TL markets were closed, is a supply factor that influences firms’ maturity choices with different types of debt. Our regression results are in ; we see that in no crisis firms tend to use short-term RC, BN, and CL. The interaction term between rating and no-crisis dummies shows that rated firms in no crisis are more likely to use long-term BN and CL. We infer that the maturity in different types of debt seems to be affected by financial crisis. This is reasonable in the sense that during financial crises, firms are hesitant to take on short-term debt. That implies that the choice to incur debt could be affected by financial crises. Therefore, firms that choose to incur short-term debt during financial crises are more likely to be those firms that are least impacted by crises, namely high-quality rated firms.
Table 9. Supply-side factors in maturity of different debt instruments.
7. Robustness checks
We conduct several robustness checks on the results shown in . Panel A of shows the correlation matrices, and the variance inflation factors (VIFs). The high VIF of SIZE is due to the fact that both SIZE and SIZE2 were included and they are highly correlated to each other. Except for this variable, VIFs take a value between 1 and 4 indicates moderate correlation between a given explanatory variable and other explanatory variables in the model, but this is often not severe enough to require attention. Overall, the table shows the lack of high correlation among the variables applied in all regression analyses conducted. Furthermore, the VIFs confirm that multicollinearity is not a major issue.
Table 10. Robustness checks.
Third, to further verify that our results are not driven by endogeneity, we use an instrumental variable (IV) approach and a Generalized Method of Moments estimator (GMM) approach. This estimator treats the explanatory variables as endogenous. To do so, we calculate the average debt maturity for a given firm in a given year and use it as a dependent variable. Panel B of show Arellano-Bond linear dynamic panel-data estimation in column (1). We use two lags of dependent variable as covariates and maximum two lags of dependent and independent variable for use as instruments. The column (1) shows regressions with robust standard errors. Sargan test of overidentifying restrictions has a null hypothesis that overidentifying restrictions are valid, and we reject the null hypothesis. In the Arellano-Bond test for zero autocorrelation in the first and second order with a null hypothesis of no autocorrelation, we reject the null hypothesis in the first order, but we cannot reject it in the second order. The p-value of Wald chi-square test confirms the joint significance for the model. Nonlinear relationship between size and debt maturity exists.
We use two-stage least squares (2SLS) estimators with instrumental variables (IV) shown in columns (2) and (3). We instrument Leverage with the lagged leverage motivated by Awartani et al. (Citation2016) along with all control variables. The t-statistics in parentheses are based on standard errors clustered at the firm-level. All the statistics for tests report its p-value. The estimates in our first-stage regression are reported in column (2). As expected, lagged leverage is related significantly and positively to the current leverage, and the p-value of the F-statistics supports the fact that this is unlikely to be a weak instrument. We then use the predicted value of leverage from the first-stage regressions as the key explanatory variable in the second-stage regression. The leverage predicted produces an insignificant coefficient. Underidentification test using Kleibergen-Paap rank LM statistic indicates that we can reject the null hypothesis that the model is underidentified at 5% significance level. Overall, we identify that amount of leverage has little power to explain debt maturity.
8. Conclusion
We study how debt maturity is affected by both the demand and supply factors for different types of debt structures, using a sample of firms with various debt instruments. Our empirical analysis shows that firms’ size and maturity choices for RC, TL, and CL follow Diamond’s (Citation1991) non-linear relationship between size and maturity. Larger firms tend to have longer maturities for RC, TL, and CL, while larger firms have shorter maturities for BN and TP. Lastly, we show that debt maturity is also influenced by credit-rating constraints, which are supply-side factors.
Our findings in this article suggest that the choice of debt maturity for firms differs in the types of debt, namely debt heterogeneity developed by Rauh and Sufi (Citation2010). Prior literature has ignored this perspective when examining debt maturity. We hope this article shed light on identifying the differential behavior of debt maturity allowing debt heterogeneity.
Disclosure statement
No potential conflict of interest was reported by the author(s).
Data access statement
The data that support the findings of this study are available from the corresponding author, YL, upon reasonable request.
Additional information
Notes on contributors
Yuree Lim
Yuree Lim is an assistant professor of Finance at Texas Woman’s University. Her research deals with issues in Corporate, Behavioral and Household Finance. She has published papers in the Journal of Financial and Quantitative Analysis and Quarterly Journal of Finance. She received her PhD in Finance from the University of Alabama in 2016.
Notes
1 The underinvestment problem refers to a situation where a firm with existing debt may forgo or underinvest in positive net present value (NPV) projects. This occurs because the benefits of the investment accrue more to the bondholders rather than the shareholders if the firm is in financial distress or has a high level of debt.
2 We use Maturity Year High variable as the maturity year. Capital IQ indicates that Maturity Year High represents the year of the high end of the maturity range when the maturity of a debt is given as a range instead of a single value. We select Maturity Year High because (1) Capital IQ has almost all Maturity Year High values in its database; and (2) we consider it as the longest maturity that firms can achieve.
3 We run the regression with firm size proxied by the ln(Market Capitalization). The results do not change significantly.
References
- Alnori, F. (2021). Exploring nonlinear linkage between profitability and leverage: US multinational versus domesticcorporations. Journal of International Financial Management & Accounting, 32(3), 311–335. https://doi.org/10.1111/jifm.12134
- Amihud, Y. (2002). Illiquidity and stock returns: cross-section and time-series effects. Journal of Financial Markets, 5(1), 31–56. https://doi.org/10.1016/S1386-4181(01)00024-6
- Awartani, B., Belkhir, M., Boubaker, S., & Maghyereh, A. (2016). Corporate debt maturity in the MENA region: Does institutional quality matter? International Review of Financial Analysis, 46, 309–325. https://doi.org/10.1016/j.irfa.2015.10.002
- Baker, M., Greenwood, R., & Wurgler, J. (2003). The maturity of debt issues and predictable variation in bond returns. Journal of Financial Economics, 70(2), 261–291. https://doi.org/10.1016/S0304-405X(03)00147-8
- Barclay, M. J., Marx, L. M., & Smith, C. W. (2003). The joint determination of leverage and maturity. Journal of Corporate Finance, 9(2), 149–167. https://doi.org/10.1016/S0929-1199(02)00003-2
- Belkhir, M., Ben-Nasr, H., & Boubaker, S. (2016). Labor protection and corporate debt maturity: International evidence. International Review of Financial Analysis, 45, 134–149. https://doi.org/10.1016/j.irfa.2016.01.012
- Ben-Nasr, H., Boubaker, S., & Rouatbi, W. (2015). Ownership structure, control contestability, and corporate debt maturity. Journal of Corporate Finance, 35, 265–285. https://doi.org/10.1016/j.jcorpfin.2015.10.001
- Ben-Nasr, H., Boubaker, S., & Sassi, S. (2021). Board reforms and debt choice. Journal of Corporate Finance, 69, 102009. https://doi.org/10.1016/j.jcorpfin.2021.102009
- Billett, M., Dolly King, T.-H., & Mauer, D. C. (2007). Growth opportunities and the choice of leverage, debt maturity, and covenants. The Journal of Finance, 62(2), 697–730. https://doi.org/10.1111/j.1540-6261.2007.01221.x
- Boubaker, S., Chourou, L., Haddar, M., & Hamza, T. (2019). Does employee welfare affect corporate debt maturity? European Management Journal, 37(5), 674–686. https://doi.org/10.1016/j.emj.2019.08.004
- Brick, I., & Ravid, S. (1985). On the relevance of debt maturity structure. The Journal of Finance, 40(5), 1423–1437. https://doi.org/10.2307/2328122
- Colla, P., Ippolito, F., & Li, K. (2013). Debt specialization. The Journal of Finance, 68(5), 2117–2141. https://doi.org/10.1111/jofi.12052
- Custódio, C., Ferreira, M. A., & Laureano, L. (2013). Why are US firms using more short-term debt? Journal of Financial Economics, 108(1), 182–212. https://doi.org/10.1016/j.jfineco.2012.10.009
- Dangl, T., & Zechner, J. (2021). Debt maturity and the dynamics of leverage. The Review of Financial Studies, 34(12), 5796–5840. https://doi.org/10.1093/rfs/hhaa148
- Datta, S., Iskandar-Datta, M., & Raman, K. (2005). Managerial stock ownership and the maturity structure of corporate debt. The Journal of Finance, 60(5), 2333–2350. https://doi.org/10.1111/j.1540-6261.2005.00800.x
- Diamond, D. (1991). Monitoring and reputation: The choice between bank loans and directly placed debt. Journal of Political Economy, 99(4), 689–721. https://doi.org/10.1086/261775
- Faulkender, M., & Petersen, M. A. (2006). Does the source of capital affect capital structure? Review of Financial Studies, 19(1), 45–79. https://doi.org/10.1093/rfs/hhj003
- Flannery, M. (1986). Asymmetric information and risky debt maturity choice. The Journal of Finance, 41(1), 19–37. https://doi.org/10.2307/2328342
- Graham, J. R., & Harvey, C. (2002). How do CFOs make capital budgeting and capital structure decisions? Journal of Applied Corporate Finance, 15(1), 8–23. https://doi.org/10.1111/j.1745-6622.2002.tb00337.x
- Johnson, S. A. (2003). Debt maturity and the effects of growth opportunities, managerial discretion, and the security issue decision. Review of Financial Studies, 16(1), 209–236. https://doi.org/10.1093/rfs/16.1.209
- Lou, Y., & Otto, C. A. (2020). Debt heterogeneity and covenants. Management Science, 66(1), 70–92. https://doi.org/10.1287/mnsc.2018.3141
- Myers, S. C. (1977). Determinants of corporate borrowing. Journal of Financial Economics, 5(2), 147–175. https://doi.org/10.1016/0304-405X(77)90015-0
- Brockman, P., Martin, X., & Unlu, E. (2010). Executive compensation and the maturity structure of corporate debt. The Journal of Finance, 65(3), 1123–1161. https://doi.org/10.1111/j.1540-6261.2010.01563.x
- Rauh, J. D., & Sufi, A. (2010). Capital structure and debt structure. Review of Financial Studies, 23(12), 4242–4280. https://doi.org/10.1093/rfs/hhq095
- Saretto, A., & Tookes, H. E. (2013). Corporate leverage, debt maturity, and credit supply: The role of credit default swaps. Review of Financial Studies, 26(5), 1190–1247. https://doi.org/10.1093/rfs/hht007
- Stohs, M., & Mauer, D. (1996). The determinants of corporate debt maturity structure. The Journal of Business, 69(3), 279–312. https://doi.org/10.1086/209692
Appendix
Table A.1. Explanatory variable definitions.
Table A.2. An example of computing the principal-weighted debt maturity.