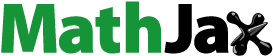
Abstract
The objective of this paper is to investigate the differences in the agricultural productivity of male and female cereal growers in rural Ghana. This study uses data from the Ghana Socioeconomic Panel Survey (GSPS) conducted in 2017/2018. The paper employs the Oaxaca–Blinder decomposition method to decompose the unconditional gender productivity gap into endowment effects and structural effects. The findings show that the total cereal production of female plot managers is 46% lower than that of their male counterparts. The results from the study reveal that the gender productivity gap varies across productivity distributions. Access to agricultural lands and inputs was found to be the principal factor driving the gender productivity gap. The paper contribute to literature by using actual harvest per acre of land for the yield function instead of using sales revenue which has been adopted by most earlier. Unlike several earlier studies that rely on dataset from small localized communities that cannot provide much information on external validity of their findings, the study uses a national representative dataset for its analysis. The study is the first study in Ghana that tries to investigate the dynamics of the endowment effect and structural effect at different quintiles of the yield function of farm produce.
Impact statement
Even though it is widely acknowledged that productivity gap exists between female and male farmers, the available evidence has often relied on samples from small localized communities. Unlike earlier studies this paper uses data from Ghana that covers a wide geographical area and therefore findings and policy recommendations are nationalistic in nature. Thus, any policy to improve access to agricultural land and inputs by women will greatly contribute to the reduction of the gender productivity gaps among cereal growers in rural Ghana and other similar rural communities in sub-Sahara Africa that share similar cultural, religious and socio-economic characteristics like that of rural Ghana.
1. Introduction
Agriculture is the predominant source of employment and is central to the livelihoods of most people living in sub-Saharan Africa (AfDB (African Development Bank Group), Citation2019; World Bank, FAO & IFAD, Citation2009). For many developing countries, especially those in sub-Saharan Africa, to realize sustainable development goals (SDGs), there is a need to pay attention to the transformational dynamics in the agricultural sector (AfDB (African Development Bank Group), Citation2016). One of the key transformational dynamics in the sector that ought to be addressed to ensure improved productivity and food security is to resolve the gender imbalance in acquiring agricultural lands and inputs. Although women account for more than 40 percent of the labor force in agriculture (FAO, Citation2011; Palacios-Lopez et al., Citation2017), several constraints prevent them from achieving their highest potential in the sector (FAO, Citation2011; World Bank, Citation2012). Several studies have outlined a lack of access to land, weaker tenure security on land, and limited access to technology, information, fertilizers, labor, and agricultural extension services as the main constraints that affect the productivity of women in the agricultural sector (see, inter alia, Lambrecht, Citation2016; Lambrecht et al., Citation2018; Oseni et al., Citation2015; Quaye et al., Citation2019; Tufa et al., Citation2022; Yokying & Lambrecht, Citation2020).
Maize, rice, sorghum, and millet are the main cereal crops grown in Ghana, and they remain the primary source of energy for all Ghanaians (Effraim, Citation2013). Thus, for Ghana to achieve the sustainable development goal of attainable food security and gender equality there is a need to look at the productivity of both male and female farmers producing these crops. According to FAO (Citation2011), women’s farm yield can improve by 20–30 percent if they are given the same productivity resources as their male counterparts. In this regard, directing policies to areas that may improve women’s agricultural productivity would greatly benefit the country towards food sustainability, women’s empowerment, and closing of the gender productivity gap. Therefore, there is a need to understand the actual problems causing the gender inequalities and the dynamics of this problem along the yield function of the cultivation of cereals in the country. Despite the policy relevance of understanding the gender inequalities in the agricultural productivity of cereal growers in Ghana, there is still relatively little evidence in this area of study in the country.
A comprehensive review of literature in this field suggests that gender productivity gap may differ across geographical locations and the type of crop cultivated. Oseni et al. (Citation2015) argued that because of differences in socioeconomic needs and agro-economic and environmental challenges, it would be inappropriate to generalize the gender productivity gap in agriculture. It is therefore important to do country specific and crop specific analysis in gender productivity gap in cultivation. However, there has been no such study in Ghana that has examined the gender productivity gap in the cultivation of cereals. Several of the studies in the country examine the gender gap in accessing a specific input in the country. Against these considerations, this paper provides new evidence of gender inequalities in the productivity of cereal growers in the country. To address this primary objective, the following research questions would be explored: (1) Do the characteristics of female farmers growing cereal crops differ from those of their male counterparts? (2) What are the characteristics that predict the productivity gap of cereal growers? (3) What proportion of the productivity gap, if any, can be attributed to the endowment effect? (4) Does the gender productivity gap change across the distribution of the yield function?
To answer all the research questions, the study adopts the Oaxaca decomposition to estimate the total productivity gap and then classifies the productivity gap into two parts: the part of the total productivity gap referred to as the endowment effect in the development economics literature (gender gap in productivity resulting from differential individual characteristics and access to factors of agricultural production) and the part that is a result of the structural effect (gender gap in productivity resulting from the difference in returns to individual characteristics and factors of production). We take advantage of the rich information on the demographic characteristics of the farmer, yield from farmland, labour inputs on farmlands, fertilizers and chemicals applied on farmlands, and plot characteristics in the Ghana Socio-Economic Panel Survey (GSPS) conducted in 2018 to decompose the productivity gap into endowment effects and structural effects.
The lack of consensus on the differences in gender productivity gap by agriculture has been attributed to the methods that are often used to collect and analyzed data (see, inter alia, Karamba & Winters, Citation2015; Peterman et al., Citation2014). Several studies often rely on data from small localized communities and therefore findings from such studies may not be able address external validity. This study on the contrary uses a national representative dataset for its analysis. This is one of the ways the study contributes to literature. Second, while there are studies on the gender productivity gap in agriculture (see, inter alia, Aguilar et al., Citation2015; Ali et al., Citation2016; Bello et al., Citation2021; Kinkingninhoun-Mêdagbé et al., Citation2010), several of these studies used revenues from selling farm produce as the yield function. This article uses the actual harvest measured in minibags of cereals harvested from the plot of land to represent the yield function. The advantage of using the actual harvest from the plot of land is that the harvested crops that were consumed by the household will be captured, and more so, price variations across communities may not affect the yield function. Third, despite the policy relevance of understanding the gender productivity gap among farmers in Ghana, there is little evidence in the country. Particularly for cereal crops, no study in Ghana has tried to evaluate the gender productivity gap. This study attempts to contribute to closing these literature gaps by investigating the potential factors that drive the gender productivity gap among cereal growers in Ghana. Finally, to the best of our knowledge, no study in Ghana has investigated the dynamics of the endowment effect and structural effect at different quintiles of the yield function of cereal growers. Knowing these dynamics would help direct policies to farmers in a particular quintile where the gender productivity gap is wide.
Our results indicate that the total cereals that women produce are 46 percent lower than their male counterparts, which is significant. However, in terms of productivity per acre of land, the results reveal that women have a 5 percent higher harvest per acre of land than their male counterparts, but it is not significant. The endowment effect explains over 90 percent of the total productivity gap in maize cultivation and cereals in general. The estimated results reveal that plot size, household size, distance from plot to house, irrigation, and application of chemical fertilizers on the plot are important variables contributing to the productivity gap. Further analysis of whether the gender productivity gap exists at different percentiles reveals that there is a production gap at the 10th, 25th, 50th, 75th, and 90thpercentiles, and it is in favor of men. However, in terms of productivity per acre of land, our results show that women do better in the 10th and 25thpercentiles, but such productivity performance is eroded at the higher percentile levels.
The rest of the paper unfolds as follows. Section 2 presents the estimation strategy for the study. Section 3 describes the sources of data, defines key variables, and shows some relevant descriptive statistics. Section 4 discusses the empirical results and robustness checks of our estimation, while section 5 provides the conclusion and policy implications from the findings.
2. Literature review
2.1. Alternative conceptual definitions in literature
Understanding of factors that generate gender gaps has become one of the topical issues in both academia and political cycles. However, an important concern is the use of stylized but erroneous facts about the term gender (Doss et al., Citation2015). Some well established institutions such as the European Commission (European Commission Directorate-General for Employment Social Affairs & Inclusion, Citation1998) define gender gap as the differences that exist between men and women in terms of rights, participation, access and benefits. The unequal access to information, rights, participation and benefits between men and women often affect productivity of women. Productivity is generally measured in terms of output per a specific input or output per an aggregate of input (Kaliski, Citation2001). Thus, productivity is a term used to define the efficiency of production of goods or services expressed by some measure. Dissimilarities in various measures used as indicator for gender has often resulted in differences in research findings by studies trying to ascertain gender differentials between males and females (de la et al., Citation2016). Sickles and Zelenyuk (Citation2019) has also demonstrated that the differences in productivity measures often explain the differences in research outcomes of studies that concentrate on productivity.
After a substantial review of 27 studies on gender differentials in agricultural productivity, it was revealed that different gender indicators were used to measure the gender gap in agricultural productivity (FAO, Citation2011). It was revealed in their study that whiles some studies used sex of household as a measure of gender, other studies used indicators such as sex of plot manager or sex of plot owner as a measure of gender. Peterman et al. (Citation2010) argues that the different measures of gender makes comparisons across studies very difficult. Using any of the measures has its own advantages and disadvantages. For example, using sex of household head as a gender indicator measure both vulnerabilities of female headship and market imperfections and conditions that the entire household face (de la et al., Citation2016). Even though many studies have used sex of household as gender indicator due to lack of data, it is very problematic to use this indicator as it does not provide enough information on the decision maker of the plot of land under consideration thereby creating confusion for policymaking and targeting (de la et al., Citation2016; Doss & Morris, Citation2001).
The type of indicator use as gender is very relevant as decision-making in agricultural production such as the type of crops to be planted, the type of inputs to be used and whether to apply fertilizer on farmland are partly based on bargaining power, rights and access to entitlements (Goldstein & Udry, Citation2008). However, most traditional and farming communities tend to deny women equal access to such vital rights that would enable them to compete favourably with their male counterparts. In West Africa settings where female and male household members work and manage different family plots of land, it is better to use the plot manager indicator as a measure of gender (Udry, Citation1996). Besides using the indicator sex of plot manager as gender, plot holder and plot owner has also been used, just that the latter are not often been used (Peterman et al., Citation2010). The less likelihood of using plot owner as gender is most likely due to the lack of data on sex-disaggregated land ownership (de la et al., Citation2016). In this study, the indicator sex of plot manager is used as gender since it can capture the gender of the individual who makes decisions on the plot of land under consideration. Our study adds up to literature by using an indicator that may correctly capture the gender productivity gap for the entire country instead of a small community.
2.2. Agriculture in Ghana
Like most developing countries, agriculture is still the main source of income for Ghanaian rural households (World Bank, Citation2017). Land rights in rural Ghana are principally governed by customary laws where kinship and inheritance remain the key determinants of land access (Yokying & Lambrecht, Citation2020). Unlike countries in East Africa, joint holdings of farmland by couples are rare in Ghana (Lambrecht et al., Citation2018). Once an individual is allocated to farmland, that person has control over what is planted, harvested, and sold (Lambrecht & Asare, Citation2016). It is estimated that women hold approximately 30% of all agricultural plots in Ghana (De Brauw, Citation2015), but the distribution of land holdings over gender significantly varies across regions. Compared to men, several studies have found women’s access to agricultural inputs to lag behind their male counterparts, and this lag is seen to be greatest in northern Ghana (Ahmed et al., Citation2016; Ankrah et al., Citation2020; Ragsdale et al., Citation2018).
For studies in Ghana, it is established that women in the country have relatively fewer rights to use household labor on their plots, have less access to credit, and give less priority to their plots in terms of resource allocation than their male counterparts (Hill et al., Citation2014; Lambrecht, Citation2016; Quaye et al., Citation2014). A study by Doss and Morris (Citation2001) attributed the gender productivity gap in the country to differences in technology adoption and differences in access to complementary inputs, such as land, labor, and extension services. A recent study by Lambrecht et al. (Citation2018) reveals that women in Ghana are as likely as their male counterparts to sell crops when one controls for community, household, and plot characteristics. Their study further reveals that Ghanaian men tend to use more input, but there is no difference between men and women in terms of input use per acre of land. The same study also concludes that women spend more on labour than men. The above review suggests that there is no study in Ghana that has investigated the dynamics of the endowment effect and structural effect at different quintiles of the yield function of cereal growers and this study sought to fill this gap.
2.3. Empirical evidence on gender productivity gap
Several studies in SSA demonstrate that plots managed by women are less productive than their male counterparts. For example, a study in Nigeria by Oseni et al. (Citation2015) found the productivity of female-managed plots to be 28% lower than their male counterparts. Similar findings are reported by studies conducted in countries such as Malawi, Niger, Ethiopia, Tanzania and Uganda (Abdisa et al., Citation2024; Aguilar et al., Citation2015; Ali et al., Citation2016; Backiny-Yetna & McGee, Citation2015; Julien et al., Citation2023; Kilic et al., Citation2015). Some studies attribute this productivity gap to gaps in accessing nonland agricultural input, technology, and extension services (Peterman et al., Citation2014) and gaps in accessing labour input (World Bank, Citation2014).
In the case of studies that analyse the productivity gap by splitting the gap into an endowment and structural effect, they report mixed findings. Findings from countries such as Malawi, Southern Nigeria, Kenya, and Uganda reveal that the endowment effect explains a significant proportion of the gender productivity gap (Ali et al., Citation2016; Fisher & Kandiwa, Citation2014; Kilic et al., Citation2015; Oseni et al., Citation2015; Slavchevska, Citation2015; Tufa et al., Citation2022). On the other hand, evidence from countries such as Ethiopia and Northern Nigeria demonstrates that the Structural effect explains a considerable proportion of the gender productivity gap. (Abdisa et al., Citation2024; Aguilar et al., Citation2015; Bello et al., Citation2021). The various studies across countries in SSA further demonstrate significant differences in factors that contribute to the productivity gap. For example, land, herbicide use, age of farmer, child dependency ratio, and the number of adult females are significant factors that affect the endowment effect in Nigeria (Backiny-Yetna & McGee, Citation2015; Oseni et al., Citation2015); in Uganda, pesticides, type of crop, improved seeds, and total assets explain the endowment gap (Ali et al., Citation2016); and in Malawi, type of crop, fertilizer, soil type and access to agricultural equipment explain the endowment effect (Kilic et al., Citation2015; Tufa et al., Citation2022).
3. Estimation strategy
3.1. Decomposition of the gender productivity gap
The most widely used method to examine differences in agricultural productivity between men and women is to estimate a yield function that models output per acre of land as a function of a set of variables that affect production as well as the dummy for the gender of the plot manager (see inter alia, Aguilar et al., Citation2015; Kilic et al., Citation2015; Oseni et al., Citation2015. The essence of using this approach is to examine the contributing factors that explain what determines the differences between male- and female-managed plots. Plot manager as used in this article refers to the person who has full access, use, and control rights over the plot of land and the power to consume or sell the produce that is harvested on the plot for the period under consideration. To estimate the average productivity gap between women and men cultivating cereals, we examine the yield function for plot i under the management of plot manager j as follows:
(1)
(1)
Where is the total cereals harvested in plot i, which is managed by individual j living in district k.
is the variable gender, where in this article, gender is simply one’s biological sex. Thus,
is measured dichotomously, indicating 1 if farm manager j is a female who lives in district k,
is a vector of characteristics of plot manager j living in district k,
is a vector of plot characteristics in district k,
is a vector of labor input of farm manager j applied on plot i in district k,
is a vector of farming practices by farm manager j on plot i in district k, and
is a district fixed effect that captures the time-invariant district characteristics.
is the idiosyncratic error term. The explanatory variables
and
are chosen based on earlier empirical studies (Aguilar et al., Citation2015; Ali et al., Citation2016; Bello et al., Citation2021; Kilic et al., Citation2015; Oseni et al., Citation2015).
Using OLS to estimate EquationEquation (1)(1)
(1) is useful to identify the factors that explain the productivity of male- and female-managed plots but does not isolate the relative importance of the different factors. To gain insights into the importance of these factors and the proportion of the gap that can be attributed to these factors, we adopt the Oaxaca–Blinder decomposition method. The model allows us to quantify the contribution of each variable to the productivity differential for male- and female-managed plots (Oseni et al., Citation2015). This method helps us to first identify whether there exists a productivity gap between men and women in agriculture and, if any productivity gap exists, what proportion of the gap can be attributable to differences in observable characteristics. The remaining model provides a detailed explanation of the model for the study.
To compute the productivity gap, the study employs the decomposition framework developed by Oaxaca (Citation1973) and Blinder (Citation1973). To ensure that the decomposition is not sensitive to the choice of the reference group, the paper follows the approach presented in Fortin (Citation2008). In his approach, the reference structure in EquationEquation (1)(1)
(1) is obtained from a pooled regression for both male and female production so that the productivity of male advantage would be equivalent to the productivity of female disadvantage concerning the reference structure in EquationEquation (1)
(1)
(1) that would be obtained from the pooled regression. Before estimating the Oaxaca–Blinder decomposition, the study first uses OLS to estimate EquationEquation (1)
(1)
(1) for each gender-managed plot, which is given as:N
(2a)
(2a)
(2b)
(2b)
where the subscripts f and m represent female and male, respectively.
We assume that and
have a zero conditional mean, and therefore, the ordinary least square estimates are unbiased. This assumption suggests that the total productivity gap can be decomposed into terms based on observables and their returns (Yahmed, Citation2018). With the assumption that the distributions of
and
are the same, we can perform the decomposition since the same distribution of the error terms suggests an identical selection bias for both females and males. Under this assumption, the mean productivity gap between males and females can be represented as follows:
(3)
(3)
where W is the vector of covariates that include the gender of the plot manager, characteristics of the plot manager, plot characteristics, characteristics of labor, and the type of farming practices, as explained in EquationEquation (1)
(1)
(1) . The subscript p shows that the parameters are from the pooled productivity regression. The first term on the right-hand side of the equation captures the endowment effect, and the last two terms represent the structural effect.
3.2. Recentered influence function (RIF)
The Oaxaca–Blinder decomposition explained in Section 2.1 can only be used to estimate the gender productivity gap at the mean, and therefore, the results obtained from such estimation provide the mean outcome difference between male and female managers. However, the productivity gap may differ across the yield distribution. A similar study in Nigeria by Oseni et al. (Citation2015) and in Malawi by Kilic et al. (Citation2015) found that the gender productivity gap at the mean is not necessarily the same as that found across other points in the distribution. To this end, this study also aims to test whether similar findings can be observed using the Ghanaian dataset.
Thus, the study further adopts quintile regression as the methodology is useful in determining relationship among variables outside the mean of the data. The methodology is more useful in determining what accounts for productivity gap in agriculture at different percentile for the yield function. The nonlinear relationship which often characterise the productivity function in the agriculture sector makes the use of quintile regression a good methodology to be used to analyse the factors contributing to the productivity gap at various quintiles. Quintile regression is useful because it drops the assumption that variables that determine productivity gap at the lower tails or upper tails of the distribution is the same as that of the mean. Thus, the methodology helps us to identify the factors that are important determinants of productivity gap for different subgroups of farmers. By adopting a regression technique proposed by Firpo et al. (Citation2009), we can evaluate the gender productivity gap across the distribution in a way that is similar to the Oaxaca decomposition except that the dependent variable would now be replaced with the RIF of the outcome of interest. The RIF is defined as:
(4)
(4)
Where Y is the outcome variable of interest, which is to be replaced by its estimated IF(Y;U). The last term in Equationequation (4)(4)
(4) is the influence function that corresponds to the observed value of Y for the distributional statistic U(FY) and is equivalent to:
where τ is the τth quantile of
is the density of the marginal distribution of the dependent variable (Y), and
is an indicator function equal to 1 if the term inside the brackets is satisfied and 0 if not. For more details on how to construct RIF of the variable of interest and the procedure involved in computing regressions from RIF, see Firpo et al. (Citation2009). The RIF is estimated separately for each gender, and the outcome variables are replaced with their respective RIF, after which the Oaxaca decomposition procedure discussed in Section 2.1 is applied.
4. Data and descriptive statistics
The data used for the study are from the Ghana Socioeconomic Panel Survey (GSPS). The survey was conducted in 2017/2018 across all ten regions (now sixteen regions) in the country. A two-stage stratified sample design was used for the survey, and the stratification was based on the 10 regions of Ghana. In the first stage, geographical clusters from an updated master sampling frame constructed from the 2000 Ghana Population and Housing Census are selected. A total of 334 clusters were randomly selected from the master sampling frame. In the second stage of the selection, a simple random sampling of 15 listed households is selected from each selected cluster. In all, 5010 households from 334 Enumeration Areas (EAs) were sampled. The survey provides regionally representative data for the 10 regions of Ghana. The number of EAs for each region was proportionately allocated based on the estimated 2009 population share for each region. EAs for the Upper East and Upper West regions, which have relatively smaller population sizes, were oversampled to allow for a reasonable number of households to be interviewed in these regions.
Information on plot-level characteristics of cereal farmers and characteristics of plot managers are retrieved from the dataset. In the case of characteristics of plot managers, information on gender, age, years of schooling, literacy, a dummy indicating whether the business owner is head of the household, ethnicity, religion, marital status, size of household, number of children in the household and total number of plots held by plot manager were retrieved from the dataset. In the case of plot-level characteristics, information on yield, plot size, distance from house to the plot, soil type, type of labor that works on the plot, types of cereals considered, if the plot is intercropped, irrigation is done on the plot, improved seeds being used, weedicide, organic fertilizer, and chemical fertilizer were retrieved from the dataset. Plot sizes that were less than an acre of land were excluded from the analysis, and we used a similar definition for smallholder farms by IFPRIFootnote1 (2014), which considered farm sizes that are less than 12 acres as smallholder farms. Restricting the plot size to a maximum of 12 acres and excluding plot sizes that are less than one acre of land causes the total observation used in the analysis to be 2,995, and out of this figure, 2,553 are male plot managers, while the remaining are female plot managers. The low proportion of female plot managers demonstrates how difficult it is for women to obtain access to agricultural lands in the country.
provides descriptive statistics of the plot manager. Column (1) presents the characteristics of business managers for the pooled sample, column (2) for only male managers, column (3) for only female managers, and column (4) is the result of the test of the The mean difference between the characteristics of male plot managers and the characteristics of female plot managers. Evidence from suggests a very small difference in the ages of female plot managers and male plot managers. Furthermore, male managers have more experience in farming than their female counterparts. The data show that men have more years of education than women, although the levels are generally low. Female plot managers are less likely to be married but more likely to be single compared to male plot managers. In terms of ethnicity and religion, there are some slight differences between female plot managers and male plot managers. The data reveal that compared to men, female plot managers are less likely to be head of the household, live in a larger household, and live in a household with more children. For example, while 95 percent of male plot managers are household heads, only 60 percent of female plot managers are heads of household. On average, female plot managers are most likely to live in a household that has a nonfarm enterprise. On average, female plot managers own fewer plots than their male counterparts. Apart from the age of the plot manager, for which the test of mean difference is not significant, the rest of the variables are all significant, and most of them favor male plot managers.
Table 1. Demographic characteristics and results of a test of mean differences by gender of plot manager.
provides summary statistics on yield, labor input, sales, and farming practices. On average, men produce more cereals than women. It can be seen from the table that out of the three main cereal crops grown in Ghana, it is only rice that the total product of females is not different from their male counterparts. The table further reveals that female productivity per acre of land is not different from that of men. For example, whereas the total average harvest of a male (female) plot manager is 2.26(1.89) minibag and the test of mean difference is significant, the average harvest per acre of land is 1.19(1.25) minibag and the test of mean difference is not significant. This suggests that there may be some constraints that make the total production of women less than that of men, which will be investigated later in this study. and show kernel densities for the two plot managers for cereals in general and then for maize, millet, and rice. Both density functions in and suggest differences in the total production of maize, millet, and cereals in general but no difference in the total harvest per acre of land (i.e. productivity) in the same crops, including rice.
Figure 1. Distribution of total cereals harvested and total cereals harvested by an acre of land by gender. Source: 3rd wave of Ghana Socioeconomic Panel Survey.

Figure 2. Distribution of total maize, millet, and rice harvested and total maize, millet, and rice harvested by an acre of land by gender. Source: 3rd wave of Ghana Socioeconomic Panel Survey.

Table 2. Plot-level characteristics and results of a test of mean differences by gender of plot manager.
Men are more likely to farm on sandy and gravel soil than women, but they are equally likely to farm on loamy and clay-filled soils. It can be seen from the table that male plot managers are more likely to be directly involved in working on their plots and have household members working on their plots than female plot managers. However, female plot managers are more likely to employ hired laborers in their plots than male plot managers. Whereas female plot managers are more likely to cultivate maize than their male counterparts, male plot managers are also more likely to cultivate millet than their female counterparts. In the case of the cultivation of rice, the mean test indicates no significant difference between male plot managers and female plot managers. shows that compared to men, women tend to sell their produce directly to consumers within the community but have a lesser likelihood of selling it to traders. However, comparing sales between the two plot managers, there is no significant difference in sales of products to consumers in a nearby community or a trade organization. Compared to male plot managers, female plot managers are more likely to do irrigation, use improved seeds, and apply weedicide and pesticides but less likely to apply chemical fertilizers, although the overall levels of application of these important farming practices are generally low. For example, whereas 1% (7%) of male (female) plot managers do irrigation, 35% (28%) applied chemical fertilizers on their plots of land. The table further reveals that female plot managers tend to practice intercropping on their plots of land more than their male counterparts.
5. Results
5.1. Presentations and discussion of results
5.1.1. Determinants of agricultural productivity of cereal farmers
To understand the factors that affect the productivity of female-managed plots and male-managed plots, the study uses OLS to estimate EquationEquations (1)(1)
(1) , Equation(2a)
(2a)
(2a) , and Equation(2b)
(2b)
(2b) in Section 2. presents the results obtained from the OLS estimate. Columns (1) – (4) provide results for total harvest as a dependent variable, and columns (5) – (8) provide results for total minibags per acre of land harvested as a dependent variable. In column (1) of the table, we present the results of the regression with the gender dummy as the only explanatory variable. Column (2) presents the results for the pooled regression, and columns (3) and (4) present the results for female-managed plots and male-managed plots, respectively. Columns (5) – (8) follow a similar pattern as in columns (1) – (4).
Table 3. OLS results on gender productivity of cereal growers in rural Ghana.
From the table, the naïve regression shows that the total production of cereals by female plot managers is 39 percent lower than their male counterparts, but once we controlled for plot-level characteristics and demographic characteristics, the total gap is significantly reduced, and the statistical test becomes insignificant. In the case of total harvest per acre, the result shows no significant difference in the productivity of male plot managers and female plot managers for both the naïve regression and the full model. The table reveals that the status of married men does not have any effect on productivity, but married women and those who have ever married are most likely to be more productive than their counterparts who are not married. This is likely to be possible because husbands of married female plot managers may facilitate access to farm inputs for their wives, which may help them to be more productive than their counterparts who are unmarried. While household size affects the productivity of men, there is no such effect for women plot managers. Surprisingly, while having children in the household does not have a significant effect on the productivity of female plot managers, it is somehow significant for male plot managers at the 10 percent confidence level.
The table reveals that plot size is an important factor that determines productivity for both male and female plot managers. An important revelation from the table shows that, whereas an increase in plot size increases the total cereals cultivated, an increase in plot size also has a negative effect on productivity per acre for both men and women, and this finding is consistent with Oseni et al. (Citation2015) and Carletto et al. (Citation2013), who find a negative relationship between land and productivity. further reveals that there is a differential impact of soil type on the productivity of male plot managers and female plot managers. While all sales outlets affect the productivity of male plot managers, only sales-to-market traders affect the productivity of women. We see from the table that intercropping and application of chemical fertilizers, weedicides, and pesticides have a positive effect on the productivity of male plot managers, but only pesticides and weedicides have an effect on female plot managers.
5.1.2. The gender productivity gap
The results presented in indicate that the factors that shape the productivity of female-managed plots are systematically different from those of male-managed plots. To better understand how the factors of production shape the gender productivity gap, we apply Oaxaca decomposition to decompose the productivity gap into endowment effects and structural effects. Since it is much easier to interpret the contribution of each variable in the endowment effect, the study will only report the coefficient estimates of all the variables in the part that describe the endowment effect.
Columns (1) – (4) of show the total gap in cereal production, and the last four columns present the total productivity gap per acre of land. The top six rows present results from the significance test of the gender productivity gap. The first two rows provide estimated results of the coefficient and the robust standard errors for the total productivity gap between male-managed plots and female-managed plots. Similarly, the third and fourth rows show the results for the endowment part, and the remaining two rows show the results of the structural effect.
Table 4. Decomposition of the gender differential in the productivity of cereal farmers.
As displayed in the top six rows of , the regression results reveal a gender gap in the total production of cereals harvested but not yield per acre of land. By disaggregating the total production of cereals into the three most highly cultivated cereal crops in the country, the result indicates that men are more likely to produce maize and millet than women, but there is no significant difference between men and women in terms of the total yield of rice harvested. However, the results for yield per acre of land reveal no significant difference between men and women in the cultivation of maize and millet, but women seem to do better in yield per acre of land for rice production. The results indicate that the endowment effect explains approximately 94%Footnote2 of the total gap in cereals produced, which is consistent with some earlier studies done in Malawi, Uganda, and Southern Nigeria (Ali et al., Citation2016; Fisher & Kandiwa, Citation2014; Kilic et al., Citation2015; Oseni et al., Citation2015; Slavchevska, Citation2015; Tufa et al., Citation2022). Similarly, the proportion of the endowment effect on the overall productivity gap for maize, millet, and rice can be estimated. Apart from millet, where the endowment effect for total produce is not statistically significant, the rest of the estimates are significant. In the case of yield per acre, the overall productivity gap by gender is insignificant, and the proportion of the endowment effect to total cultivation is also smaller in magnitude and statistically insignificant. For example, while the overall gap for yield per acre of land of cereals cultivated is -0.053 and is statistically insignificant, the endowment effect is 43% of the overall gap. Although the overall gap in yield per acre of rice cultivated favours women, that of the endowment effect is statistically insignificant. The findings suggest that women in cereal crop production in rural Ghana are equally productive as men in terms of utilization of agricultural lands. However, due to the small acreages of land that they harvest, their total cultivations fall short of that of men. In the case of Millet, the results reveal that the structural effect is stronger in determining the gender gap, which is also consistent with studies by Aguilar et al. (Citation2015) and Bello et al. (Citation2021). The specific variables that influence the gender productivity gap and to what degree they contribute to explaining the endowment effect are reported in the lower portions of . Here, much of the concentration will be on the variables with positive coefficient estimates that are significant, since it provides evidence of the contribution of observable characteristics to the total gender productivity gap.
The size of the household, plot size, distance to the plot, farmland soil type, chemical fertilizer applied to the land, harvest sold to traders, Gurma, Mole-Dagbani ethnic group, and irrigation are the most consistent predictors of the gender productivity gap. Plot size is the only variable that is statistically significant across all five outcome variables. The results on the effect of plot size on the gender productivity gap in yield per acre demonstrate a female advantage in the productivity gap for yield per acre. This female advantage is observed because women often manage smaller plot sizes compared to their male counterparts, and the results in reveal a negative relationship between plot size and yield per acre, which is consistent with Oseni et al. (Citation2015). A further examination of the table reveals that the size of household, application of chemical fertilizers, and irrigation have a positive effect on the productivity gap for yield per acre, and it is statistically significant.
In summary, the findings from the second part of suggest that the size of the farmland is the most significant determinant of the gender gap in cereal production and that if women are equally given the same opportunity to access farmlands, the gender productivity gap in the cultivation of cereals would decrease. The estimations in the table reveal that at least 48% of the endowment effect in the productivity gap comes from the size of the plot. For example, the contribution of plot size to the endowment effect in the total production of cereals is approximately 50% Footnote3 of the gap. Moreover, equitable distribution of agricultural inputs such as farm laborersFootnote4, chemical fertilizers and irrigation will minimize the gender gap in production. Even though the effect of chemical fertilizers and irrigation on the productivity gap seems to be small, the impact on the gender productivity gap would have been much more significant if the adaptation of irrigation and application of chemical fertilizers by Ghanaian farmers were to be high. Thus, nongendered access to these inputs would likely minimize the gender productivity gap. The table shows that irrigation and application of chemical fertilizers have a higher combined effect on the gender gap for maize growers than on the other cereal crops. Findings from this paper corroborate the argument by FAO. (Citation2011) that reducing the gender inequalities in access to agricultural land and other essential inputs is likely to increase women’s productivity by more than 20%.
5.1.3. Decomposition across total yield and yield per acre distribution
We now turn our attention to investigating our final research question. The Oaxaca decomposition explained above provides results for the productivity gap at the mean of the outcome variable. While analysing the gender productivity gap at the mean is very informative, analysing the gap across the distribution also provides additional information that may be useful to policymakers. To be able to estimate the gender productivity gap at various quantiles, the outcome variables need to be replaced with their respective RIF, as discussed in Section 2.1 of the manuscript. presents the RIF results for total production and yield per acre of land for maize, millet, rice, and all three cereal crops combined.
Table 5. Decomposition of the gender differential in productivity at various percentiles.
The results presented in columns (1) – (4) of the table indicate a significant gender gap in overall production across all quantiles apart from the results of the fifth decile for the estimation for rice plot managers, which is not significant. The observed gap across all quantiles is in favour of male plot managers. Interestingly, it can be further observed that the gender gap in the overall production of cereals increases from the first decile to the ninth decile. The results for the gender gap in production for maize farmers are consistent with the estimates obtained for the whole cereal crop. In the case of millet and rice, even though the result shows a clear gender gap in the total production of these two crops, there is no clear trend across the distribution. For example, the gender gap in production for rice farmers decreases from 0.66 log points to 0.30 log points and then rises to 0.66 log points at the 50th, 75th, and 90th percentiles, respectively. Apart from the first decile, in which we find the structural effect dominating the endowment effect for maize and cereals in general, the results for the other higher quantiles reveal that the endowment effect is more important in explaining the gender productivity gap. Similarly, apart from the 50th percentile of millet, in which the structural effect is seen to be more important than the endowment effect, the rest of the results for millet and rice reveal the endowment effect to be more important in explaining the gap than the structural effect. These results suggest that agricultural inputs matter more in closing the gender productivity gap than the returns to these inputs.
This section shifts attention to the explanation of the last four columns of . In these columns, the outcome variable yield per acre is replaced with its RIF. The results for the first decile show women to be more productive in terms of yield per acre than men for all three cereal crops. However, such a productivity advantage seems to decline and largely turns out to be insignificant at higher levels of the distributions for maize, rice, and cereals in general. For example, the gender productivity gap in yield per acre of land of cereals (maize) rice consistently declines from −0.2432(−0.1795)−0.3309 to −0.0773(0.0834) −0.1415 at the 10th, 25th 50th, and 75th percentiles. The test of significance of the gap shows insignificance for the 50th and 75thpercentiles. Both the endowment effect and structural effect tend to explain the gap in yield per acre. The endowment effect for the lower part of the distribution favors women, and the returns to this endowment favor men. In contrast, the endowment effect at the higher part of the distribution largely favors men. Our finding is similar to that of Oseni et al. (Citation2015), who found the endowment effect to favor women at the lower quartile but reverse to favour men at the upper quartiles in Northern Nigeria. In summary, the size of our gap and the pattern of the endowment and structural effect obtained in our study are very close to those of northern Nigeria (Oseni et al., Citation2015) but somewhat different from those of southern Nigeria (Oseni et al., Citation2015).
6. Conclusion
The study set out to investigate the gender productivity gap between female cereal growers and their male counterparts. The study findings reveal a gender productivity gap attributable to both endowment and structural effects of both genders. The gender productivity gap recorded is generally positive and significant. The findings show that the total cereal production of female plot managers is 46% lower than that of their male counterparts. In terms of productivity per acre of land, the results reveal that the productivity of female plot managers is not significantly different from that of male plot managers. This finding is consistent with the result obtained for each of the cereal crops (maize, millet, and rice) analysed. Apart from millet, the result from the decomposition shows that the endowment effect is much more important than the structural effect in explaining the gender performance gap. The results from the RIF suggest that the gender productivity gap is not the same across productivity distributions. This finding is consistent with a similar study in Nigeria by Oseni et al. (Citation2015) and in Malawi by Kilic et al. (Citation2015).
Plot size is found to be the variable that has the highest positive effect on the gender gap in productivity. In contrast, the results show that the larger the farmland is, the lower the yield per acre of cereals harvested. Given that men cultivate bigger farmlands than their female counterparts, the effect of plot size on the gender gap in productivity of yield per acre of land tends to significantly reduce—this is because the female advantage of plot size on the gender gap in yield per acre of cereals is high. This finding is consistent with Oseni et al. (Citation2015) and Carlleto et al. (2013), who found a negative relationship between land size and productivity. The adoption of chemical fertilizer and irrigation is seen to have a positive effect on the gender productivity gap. The effect is seen to be larger for maize growers. The size of the household has a significant effect on the gender productivity gap, and it is in favor of men. In several rural communities in Ghana, members of households often serve as laborers to work in plots considered to belong to the entire household, and such household plots are often managed by men. This possibly explains why the size of households has a significant effect on the gender productivity gap. Other variables, such as the type of soil of the farmland and distance to the farmland, all contribute to the gender productivity gap.
This study presents some key policy implications. It makes an important contribution to the overall effort by Ghana to achieve three important sustainable development goals. First, a greater proportion of rural women are in agriculture, and therefore, policies that seek to reduce the productivity disparity would help Ghana achieve SDG 1 (poverty eradication), SDG 2 (zero hunger), and SDG 5 (gender equality). Policies aimed at improving female endowments such as access to farmlands, access to chemical fertilizer, irrigation, or market access will likely reduce the gender productivity gap in agriculture. An important policy dimension to promote access to chemical fertilizer and the market would be to form women groups through which the distribution of chemical fertilizers and provision of a produce market would be promoted. In terms of access to farmlands, there should be a deliberate effort to educate opinion leaders on the need to change customary practices that discriminate against women.
Generally, the use of chemical fertilizers and irrigation is not widespread enough in the country. The low adoption of chemical fertilizers and irrigation may be one reason why these technologies seem not to contribute substantially to the aggregate gap. Although the adoption rate is still small, evidence suggests that these technologies are important in contributing to output yield; therefore, farmers need to be sensitized to use these technologies in their farming activities. The differences in the ratio of male to female adoption of these technologies and the statistically significant contribution of these technologies to the gap suggest that programs to encourage the adoption of these technologies should incorporate elements to ensure that adoption of these technologies is gender-neutral. For example, the use of chemical fertilizers can be subsidized to improve their adoption, and vouchers can be introduced to avoid any gender discrimination that is likely to occur during distribution.
Like most studies, this study also has some limitations. First, the dataset analysed in this study does not capture who decides on the time allocations and labor hours that must be spent on the plot of farmland analysed. In some traditional settings, heads of households decide the time allocation and labour hours that need to be spent on a plot of land owned by a household member but not the plot manager since the farmland belonging to the household head is considered to be the farm for all household members. Given this and the fact that most household heads in a majority of rural households tend to be men, data on who makes decisions on the plot of land if available and controlled for is likely to cause the gender productivity gap to be smaller than our regression estimate.
Second, the study uses self-reported variables (outcome variables), which may cause some potential bias if the errors in reporting the productivity of yields from the plot of land are not randomly distributed across individual observations or if the reports from those females are systematically different from their male counterparts. In the case of any systematic bias in reporting the productivity of men and women, the productivity gap estimated in the current study may be different from the actual productivity gap in the country.
Finally, some studies have shown how the definition of the gender variable may affect the gender productivity gap. For example, de la O Campos et al. (2016) used three definitions for the gender variable, namely, a female is the head of the household, a female is the plot owner and a female is the plot manager, to measure the gender productivity gap in Uganda. Evidence from their study showed that the gender productivity gap in Uganda depends on the choice of the type of gender definition used. It is possible to have similar situations in the Ghanaian context; nonetheless, this study limited itself to only one definition of gender. However, this definition used in our study allows us to match the characteristics of the individual in the household owning and managing the plot to the input and activities employed on the plot and the productivity of the plot.
Notwithstanding the limitations of this study, it presents some avenues for future research. First, the study establishes and provides evidence of the existence of a productivity gap between female and male cereal growers; however, future studies with larger observations may explore analysing the gender productivity gap only for cereal farmers that do monocropping on the farm. Moreover, the study opens attractive avenues for future studies to explore the gender gaps in other important plant crops, such as cocoa, cashew, and some other relevant cash crops.
Additionally, tracing the trends in the productivity gap for cereal growers to ascertain whether the productivity gap for cereal growers is increasing or reducing would be useful.In this regard, future studies that have long-term longitudinal data may explore this by analysing the trend of the productivity gap in Ghana and the factors that contribute to the closing or widening of the gender productivity gap among these farmers.
Future studies, for instance, may consider examining the gender productivity gap for several crops and analyse the productivity gap according to geographical locations to ascertain the locations where female farmers perform better. Such findings may provide empirical evidence to policymakers on the type of crops or locations within the agricultural sector where women could be encouraged to concentrate in attempting to address the productivity gap. Finally, future studies may consider following de la O Campos et al. (2016) to ascertain whether the gender productivity gap in Ghana also depends on the definition of the gender variable considered.
Ethical approval
This article does not contain any studies with human participants performed by any of the authors.
Acknowledgment
There is no funding for this research.
Disclosure statement
No potential conflict of interest was reported by the author(s).
Data availability statement
The datasets analysed during the current study are available in the Yale Economic Growth Center, and the web link for the dataset is https://www2.statsghana.gov.gh/nada/index.php/catalog/97.
Additional information
Notes on contributors
Emmanuel Adu Boahen
Emmanuel Adu Boahen is a development economist with special research interest in education economics, labour market and population economics. Dr. Boahen has more than 10 years university teaching experience and has several publications in high impact journals.
Justice Boateng Dankwah
Justice Boateng Dankwah is a marketing specialist with roughly six years teaching experience at the tertiary level. His research interest is in consumer behaviour and international marketing.
Daniel Berko
Daniel Berko is finance and accounting expert with special interest in green accounting, international finance and micro finance. He has a rich Banking experience and has roughly 10 years university teaching experience. Daniel Berko has published several articles in high impact journals.
Notes
1 International Food Policy institute.
2 The percentage difference for the endowment part is obtained by:
3 This is obtained as.
4 Household size can serve as a proxy for farm laborers as most rural farmers tend to rely on family laborers for cultivation.
References
- Abdisa, T., Mehare, A., & Wakeyo, M. B. (2024). Analyzing gender gap in agricultural productivity: Evidence from Ethiopia. Journal of Agriculture and Food Research., 15, 100960. https://doi.org/10.1016/j.jafr.2023.100960
- AfDB (African Development Bank Group). (2016). Feed Africa – strategy for agricultural transformation in Africa, 2016–2025.
- AfDB (African Development Bank Group). (2019). Creating decent jobs; strategies, policies and instruments. https://www.afdb.org/en/documents/creating-decent-jobs-strategies-policies-and-instruments (Accessed November 2019).
- Aguilar, A., Carranza, E., Goldstein, M., Kilic, T., & Oseni, G. (2015). Decomposition of gender differentials in agricultural productivity in Ethiopia. Agricultural Economics, 46(3), 311–334. https://doi.org/10.1111/agec.12167
- Ahmed, A., Lawson, E. T., Mensah, A., Gordon, C., & Padgham, J. (2016). Adaptation to climate change or non climatic stressors in semi-arid regions? Evidence of gender differentiation in three agrarian districts of Ghana. Environmental Development, 20(2016), 45–58. https://doi.org/10.1016/j.envdev.2016.08.002
- Ali, D., Bowen, D., Deininger, K., & Duponchel, M. (2016). Investigating the gender gap in agricultural productivity: Evidence from Uganda. World Development, 87(2016), 152–170. https://doi.org/10.1016/j.worlddev.2016.06.006
- Ankrah, A. D., Freeman, Y. C., & Afful, A. (2020). Gendered access to productive resources – evidence from smallholder farmers in Awutu Senya West District of Ghana. Scientific African, 10(2020), e00604. https://doi.org/10.1016/j.sciaf.2020.e00604
- Backiny-Yetna, P., & McGee, K. (2015). Gender differentials and agricultural productivity in Niger. Policy Research Working Paper, 7199.
- Bello, O. L., Lloyd, J. S., Baiyegunhi, J. S. L., Danso-Abbeam, G., & Ogundeji, A. A. (2021). Gender decomposition in smallholder agricultural performance in rural Nigeria. Scientific African, 13(2021), e00875. https://doi.org/10.1016/j.sciaf.2021.e00875
- Blinder, A. S. (1973). Wage discrimination: Reduced form and structural estimates. The Journal of Human Resources, 8(4), 436–455. https://doi.org/10.2307/144855
- Carletto, C., Savastano, S., & Zezza, A. (2013). Fact or artefact: The impact of measurement errors on the farm size-productivity relationship. Journal of Development Economics, 103, 254–261.
- De Brauw, A. (2015). Gender, control, and crop choice in northern Mozambique. Agricultural Economics, 46, 1–14.
- de la, O., Campos, A. P., Covarrubias, K. A., & Patron, A. P. (2016). How does the choice of the gender indicator affect the analysis of gender differences in agricultural productivity? Evidence from Uganda. World Development, 77(C), 17–33. https://doi.org/10.1016/j.worlddev.2015.08.008
- Doss, C. R., & Morris, M. L. (2001). How does gender affect the adoption of agricultural innovations? Agricultural Economics, 25(1), 27–39. https://doi.org/10.1111/j.1574-0862.2001.tb00233.x
- Doss, C., Kovarik, C., Peterman, A., Quisumbing, A., & van den Bold, M. (2015). Gender inequalities in ownership and control of land in Africa: Myth and reality. Agricultural Economics, 46(3), 403–434. https://doi.org/10.1111/agec.12171
- Effraim, K. P. (2013). Help tools and investment potentials of a wheat business in Ghana: A case study of Olam-Ghana. theseus.fi/handle/10024/70276 (Accessed November 2020)
- European Commission, Directorate-General for Employment, Social Affairs and Inclusion. (1998). One hundred words for equality: A glossary of terms on equality between women and men. Publications Office.
- FAO. (2011). The state of food and agriculture 2010–11: Women in agriculture. FAO.
- Firpo, S., Fortin, N. M., & Lemieux, T. (2009). Unconditional quantile regressions. Econometrica, 77(3), 953–973.
- Fisher, M., & Kandiwa, V. (2014). Can agricultural input subsidies reduce the gender gap in modern maize adoption? Evidence from Malawi. Food Policy. 45, 101–111. https://doi.org/10.1016/j.foodpol.2014.01.007
- Fortin, N. M. (2008). The gender wage gap among young adults in the United States. The importance of money versus people. Journal of Human Resources, 43(4), 884–918. https://doi.org/10.1353/jhr.2008.0006
- Goldstein, M., & Udry, C. (2008). The profits of power: Land rights and agricultural investment in Ghana. Journal of Political Economy, 116(6), 981–1022. https://doi.org/10.1086/595561
- Hill, R. V., Vigneri, M., Quisumbing, A. R., Meinzen-Dick, R., Raney, T. L., Croppenstedt, A., & Behrman, J. A. (2014). Mainstreaming gender sensitivity in cash crop market supply chains. In A. Peterman (Eds.), Gender in agriculture: Closing the knowledge gap (pp. 315–342). Springer.
- Julien, C. J., Boris, E. B., & Rada, E. N. (2023). Gender and agricultural productivity: Econometric evidence from Malawi, Tanzania, and Uganda. World Development, 171(2023), 106365. https://doi.org/10.1016/j.worlddev.2023.106365
- Karamba, R. W., & Winters, P. C. (2015). Gender and agricultural productivity: Implications of the farm input subsidy program in Malawi. Agricultural Economics, 46(3), 357–374. https://doi.org/10.1111/agec.12169
- Kaliski, B. S. (2001). Encyclopedia of business and finance. Macmillan Reference USA (ISBN 0028650654. OCLC 45403115).
- Kilic, T., Palacios-López, A., & Goldstein, M. (2015). Caught in a productivity trap: A distributional perspective on gender differences in Malawian agriculture. World Development, 70(2015), 416–463. https://doi.org/10.1016/j.worlddev.2014.06.017
- Kinkingninhoun-Mêdagbé, F. M., Diagne, A., Simtowe, F., Agboh-Noameshie, A. R., & Adégbola, P. Y. (2010). Gender discrimination and its impact on income, productivity, and technical efficiency: Evidence from Benin. Agriculture and Human Values, 27(1), 57–69. https://doi.org/10.1007/s10460-008-9170-9
- Lambrecht, I. B. (2016). As a husband I will love, lead, and provide. Gendered access to land in Ghana. World Dev., 88(20150), 188–200. https://doi.org/10.1016/j.worlddev.2016.07.018
- Lambrecht, I., & Asare, S. (2016). The complexity of local tenure systems: A smallholders’ perspective on tenure in Ghana. Land Use Policy, 58, 251–263. https://doi.org/10.1016/j.landusepol.2016.07.029
- Lambrecht, I., Schuster, M., Asare Samwini, S., & Pelleriaux, L. (2018). Changing gender roles in agriculture? Evidence from 20 years of data in Ghana. Agricultural Economics, 49(6), 691–710. https://doi.org/10.1111/agec.12453
- Moore, D. P., & Buttner, E. H. (1997). Women entrepreneurs: Moving beyond the glass ceiling. Academy of Management Review, 24(2). https://doi.org/10.5465/amr.1999.2202142
- Oaxaca, R. L. (1973). Male–female wage differentials in urban labor markets. International Economic Review, 14(3), 693–709. https://doi.org/10.2307/2525981
- Oseni, G., Corral, P., Goldstein, M., & Winters, P. (2015). Explaining gender differentials in agricultural production in Nigeria. Agricultural Economics, 46(3), 285–310. https://doi.org/10.1111/agec.12166
- Palacios-Lopez, A., Christiaensen, L., & Kilic, T. (2017). How much of the labor in African agriculture is provided by women? Food Policy. 67, 52–63. https://doi.org/10.1016/j.foodpol.2016.09.017
- Peterman, A., Quisumbing, A., Behrman, J., & Nkonya, E. (2010). Understanding gender differences in agricultural productivity in Uganda and Nigeria (IFPRI discussion paper 01003), International Food Policy Research Institute (IFPRI).
- Peterman, A., Behrman, J. A., & Quisumbing, A. R. (2014). A Review of Empirical Evidence on Gender Differences in Nonland Agricultural Inputs, Technology, and Services in Developing Countries. In: Quisumbing, A., Meinzen-Dick, R., Raney, T., Croppenstedt, A., Behrman, J., Peterman, A. (eds), Gender in Agriculture. Springer, Dordrecht. https://doi.org/10.1007/978-94-017-8616-4_7
- Quaye, W., Fuseini, M., Boadu, P, & Asafu-Adjaye, N. Y. (2019). Bridging the gender gap in agricultural development through gender responsive extension and rural advisory services delivery in Ghana. Journal of Gender Studies, 28(2), 185–203. https://doi.org/10.1080/09589236.2017.1419941
- Quaye, W., Okai, M., Dowuona, S. N., & Dziedzoave, N. (2014). Gender dimensions of decision making on production assets and challenges facing women in making among men and women smallholder farmers in Ghana’s Northern Region. Journal of Rural Studies, 64(2018), 123–134.
- Ragsdale, K., Read-Wahidi, M. R., Wei, T., Martey, E., & Goldsmith, P. (2018). Using the WEAI + to explore gender equity and agricultural empowerment: Baseline evidence among men and women smallholder farmers in Ghana’s Northern Region. J. Rural Stud., 64(2018), 123–134.
- Sickles, R., & Zelenyuk, V. (2019). Measurement of productivity and efficiency: Theory and practice. Cambridge University Press.
- Slavchevska, V. (2015). Gender differences in agricultural productivity: The case of Tanzania. Agricultural Economics, 46(3), 335–355. https://doi.org/10.1111/agec.12168
- Udry, C. (1996). Gender, agricultural production, and the theory of the household. Journal of Political Economy, 104(5), 1010–1046. https://doi.org/10.1086/262050
- Tufa, A. H., Alene, D. A., Cole, M. S., Manda, J., Feleke, S., Abdoulaye, T., Chikoye, D., & Manyong, V. (2022). Gender differences in technology adoption and agricultural productivity: Evidence from Malawi. World Development, 159(2022), 1–15. https://doi.org/10.1016/j.worlddev.2022.106027
- World Bank, FAO & IFAD. (2009). Gender in agriculture sourcebook. Washington, DC. https://openknowledge.worldbank.org/bitstream/handle/10986/6603/461620PUB0Box3101OFFICIAL0USE0ONLY1.pdf?sequence=1&isAllowed=y.
- World Bank. (2012). World development report 2012: Gender equality and development. The World Bank.
- World Bank. (2014). Levelling the field: Improving opportunities for women farmers in Africa. The World Bank.
- World Bank. (2017). Ghana-agriculture sector policy note: Transforming agriculture for economic growth, job creation, and food security. http://documents.worldbank.org/curated/en/336541505459269020/Ghana-Agriculture sector-policy note-transforming agriculture for economic growth job creation and food security.
- Yahmed, S. B. (2018). Formal but less equal. Gender wage gaps in formal and informal jobs in urban Brazil. World Development, 101(2018), 73–87. https://doi.org/10.1016/j.worlddev.2017.08.012
- Yokying, P., & Lambrecht, I. (2020). Landownership and the gender gap in agriculture: Insights from northern Ghana. Land Use Policy, 99(2020), 105012. https://doi.org/10.1016/j.landusepol.2020.105012