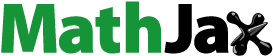
Abstract
The aim of this study is to explain the output growth across countries as an effect of intellectual capital growth. Defining intellectual capital as an integral of all the various intangibles, it argued to resolve the conceptual complexities and empirical inconsistencies. For this, the study defined the flow of new ideas as a function of change in intellectual capital level. Thus, it developed a model in which broad ideas predetermine the quality of capital and labor necessary for final goods production. The model was estimated using dynamic common correlated effect estimators for a panel of 29 countries from all income levels and geographic regions from 1990 to 2020. The results show a positive and significant contribution of national intellectual capital to economic growth. Hence, policymakers must enhance innovation in all spaces and phases of learning.
Impact statement
The introduction of intellectual capital (IC) to explain economic growth, amidst unceasing complexities in conceptions and modeling intangibles, offers unprecedented significance for academics and policy issues. The concept of IC helps to encompass both technological and social innovation capabilities. Hence, for scholars interested in this field, the approach provides a novel model that explain the rate of flow of new technological and non-technological ideas of a given nation as a function of change in national IC level. This new idea generation function is also safe from ‘scale effect’, an inevitable problem facing all various generations of endogenous models. For economic policy makers the approach could simplify the conceptual complexities and also helps to look for domestic technological and non-technological capabilities before embarking on absorption of frontier technologies.
REVIEWING EDITOR:
1. Introduction
As human intellect shines overtime the assertions about causes of difference in economic growth across nations went from simple geographical advantages in the pre-classical period to complex and intangible technological progress in 1950s. For the last seven decades, economists have endeavored to understand the essence of technological progress and its linkage to output growth but, it remained an elusive and factious factor. Those insisted on neoclassical accumulation mechanics labeled technological progress as efficiency, a residual, or any exogenous shift factor. Others unsatisfied with neoclassical mechanics labeled it human capital or research and development (R&D). Following the latter, the new endogenous growth theorists further specified it as an effect of rival human knowledge and non-rival ideas.
The view of differences in knowledge of doing with objects, rather than the mere possession of objects, as a source of economic dissimilarities was apparent. However, the essence and formation of such knowledge and its linkage to output growth remained baffling. Technological progress, as defined in all these models, fails to explain the promised catch-up hypothesis. Romer (Citation1993) repeated Nelson’s (Citation1956) promise that the poorest countries could easily break the poverty trap and leapfrog to catch up through openness to potential knowledge in the advanced countries. As a result, many studies have included foreign R&D effort, import and Foreign direct investment (FDI) to GDP ratio to account for external knowledge. However, the promise was no longer synchronous with the data trends. Besides, the issue of scale effect was the other inevitable challenge for the varieties of endogenous growth models. These models are also facing unbounded criticisms in relation to the scope and evolution of knowledge.
Given that, this study was aimed to define intellectual capital(IC) as a fundamental factor behind all the varieties of intangibles to provide a consistent explanation of output growth across countries. It states economic growth as a function of intellectual capital. It argues that the notion of ideas in endogenous growth models emphasize on technological ideas confined to frontier countries and thus, far from recognition non-technological ideas important for economic growth. It followed a novel approach of integrating IC index into Romer’s final goods sector model to generate a new empirical model.
The estimation result confirms IC as significant and consistent driver of output growth for panel of countries across various income categories. This implies, technological knowledge and its spillovers, measured in whatsoever way, were too narrow to explain the short-run and long-run growth path.
In this regard, this study could bring some cherries to the branches of giants. First, the concept of IC resolve terminological complexities and brings all the scattered intangibles to a single and meaningful factor. Second, it develops a new model of idea generation that defines the flow of new broad ideas as a function of changes in IC level.
The article proceeds with a substantive theoretical reflections on literature, then provide and discuss empirical evidences based on proposed methods. In the end, puts concludes and policy recommendations.
2. Literature review
This section provides a brief review of historical perspectives, theories and recent empirical evidences associated to sources of differences in economic growth. Following the various theoretical views and models certain reflections and the concept of intellectual was made integrated into Romer (Citation1990) innovation sector.
2.1. Theoretical framework
The history of inquires to know the causes of differences in progress across nations goes back to Petty. Following Petty’s geographical advantage and personal characteristics; the people’s attitude towards luxury and slothfulness were the often considered sources differences in the pre-classical period (Arndt, Citation1981; Spiegel, Citation1955). In the classical period Adam Smith shifted the focus to the division of labor. For Smith the division of labor was a key to improve productivity: it increases dexterity, saves time, and directs the mind to new inventions. This division of labor is not an inherent gift of nature but a gradual consequence of habits, customs, and education, acquired on the basis of necessity (Smith, Citation1776). Smith confirms what Petty said before a century, but get disposed to proximate analysis, the thrift and industry guided by the invisible hand, a liberal mechanics often called classical framework.
Others doubt the practicality of this mechanics and argued intellect, the degree of foresight, as a fundamental differential factor for its capability of orienting the effort of a man towards innovations that abridge labor (Laudardale, Citation1819; Marshall, Citation1890; Mill, Citation1848). For Marshall ‘the residuum’ may fail to progress because the toil gives no chance of making the best of their mental faculties. He realized education and technological progress as means of progress for they relieve the working class.
From Petty to Marshall (Citation1890) economists emphasized on differences in natural advantages and personal qualities as fundamental causes of differences in progress across nations. The different characteristics of people are not taken as innate but learned. However, the neo-classicals, after Bohm-Bawerk (Citation1891) theory of capital, replaced thrift and industry by saving and capital accumulation and redefined saving as the ordinary act of behavior. The theory assumes that the only productive force capable to generate surplus, a source of growth, was naturally embodied in objects (capital goods). Bohm-Bawerk and his subsequent Neo-classical theorists employed casuistry rules to justify individual decisions as constant, rational, and consistent based on perfect knowledge. The variations in individual knowledge, habits, and manners and the assumed underlying force of differences from Petty to Marshall were dodged from economic analysis. As a result, the approach was marked as mechanics that had no place for human intellect (Clark, Citation1886) or lifeless treaties (Veblen, Citation1908) or fiction a (Hayek, Citation1935) that dealt too mechanically with the human element (Haavelom, Citation1954).
Against this neoclassical approach, Schumpeter was first to consider entrepreneurial innovation as prominent cause of growth (Schumpeter, Citation1911). Later, it was more apparent for Haavelom (Citation1954) and Kaldor (Citation1954) that economic dissimilarities could be driven by ‘something intangible’, the habits and manners of individuals, which orient individuals to innovation. It was also accompanied by the series of evidences, where the traditional factors were failed to explain 7/8 of output growth (Abramovitz, Citation1956; Fabricant, Citation1954; Solow, Citation1957). The mysterious factor was variously labeled as: technical or technological progress, change, innovation and knowledge. However, its essence, formation and linkage to output growth was more elusive and indeed a source of division for exogenous, endogenous, and evolutionary growth theories.
Exogenous growth theorists were satisfied with neoclassical mechanics and hence, insisted to explain such an intangible source of growth as something exogenously evolving as a function of time (Domar, Citation1961; Solow, Citation1956). They measured the unexplained output growth as a residual. The phrase ‘technological or technical change was used to refer to any shift factor (Solow, Citation1956, Citation1957). It can be an effect of R&D, economies of scale, external economies, labor improvements (health, education and skills), better management, and improved product mix (Johnston, Citation1966).
On the other, those unsatisfied with neoclassical mechanics specified the unexplained output growth as effect of human talent or improved techniques adapted and diffused (Griliches, Citation1957; Citation1973; Schultz, Citation1956). Following these, the new endogenous growth models regarded the elusive factor as an effect of intentional investment in education or R&D. Though, they commonly consider technological progress as endogenous factor, there are varieties of understandings within new endogenous growth models.
The first-generation models, Lucas (Citation1988), Romer (Citation1990) and Aghion and Howitt (Citation1992) defined the factor as rival skills and non-rival ideas springing from R&D. Specifically, for variety of goods model a new idea was a linear function of stock of previous knowledge and number of researchers. However, for quality ladder models, new ideas flash from researcher randomly in the type of Poisson arrival rate of innovations. In both models ideas affect final output production once embodied in intermediate goods.
Nonetheless, Jones (Citation1996) found that all first-generation models share a prediction of scale effect, which puts them at odds with empirical evidence. He argued that, an increase in stock of previous ideas and number of researchers accompany a fishing out and duplication problem, respectively. Kortum (Citation1997) and Segerstrom (Citation1998) followed the same trick to avoid the scale effect.
In the third Young (Citation1998), Dinopoulos and Thompson (Citation1998), Peretto (Citation1998), Howitt and Aghion (Citation1998), and Howitt (Citation1999), developed another variety of proposals to rebut the charges issued by second-generation models. Despite the differences in assumptions and interpretations, all third-generation models argue that they retain fully endogenous long-run growth determined by research effort. They used to avoid the scale effect by distinguishing the roles of new variety innovations to offset the scale effect and quality innovation as an independent engine of long-term growth.
Different from exogenous and endogenous theories, Nelson (Citation1956) argued that given a state of knowledge, favorable sociopolitical environment could stimulate economies to fully utilize the available inputs or better techniques and hence bring economic growth. He scratched a way, the later evolutionary growth models, which considers the role of social innovation. As in new growth theories, technological progress was an endogenous source of growth in all varieties of evolutionary growth models. However, in evolutionary growth rationality is bounded and thus, the process of knowing, searching, is local or routine leading to uneven growth of knowledge and, hence, uneven economic growth. Moreover, the choice of technology (recipes) for production is not only a product of technological knowledge, but also organizational, managerial, and scientific knowledge (Nelson & Winter, Citation1982, Citation2002). Since, uneven knowledge is a necessary condition for advancing knowledge, there is no need for production possibilities to be sharply defined (Metcalfe, Citation2009).
Overall, there is no common understanding about the nature of the intangible, supposed to be a source of growth. The exogenous growth models crudely comprises everything unaccounted for, including measurement errors. It does not differentiate whether the intangible is rival, excludable, scientific, technical, or technological. Endogenous growth models based on Romer (Citation1990) emphasize the rivality and excludability properties, and hence exclusively consider the effects of R&D-based ideas. Meanwhile, evolutionary growth models approach through broader concept of innovation and hence consider organizational and social innovations. They emphasize technological practices rather than theoretical knowledge or ideas. This notes the absence of common understanding about the essence, formation, and linkage of the fundamental factor responsible for economic growth.
2.2. Reflection and contributions
In the preceding section, it was common to observe the interchangeable use of technological and technical combined with change, progress, innovation, knowledge and practice. However, in knowledge economies, the system of generating value, from planning for production to marketing, is critically determined by various categories of intangibles. This means, intangibles are of various categories and, hence, possess various direct and indirect effects on the production system. In this sense, indiscriminate use of terms could lead to conceptual complexities and understate the value of other intangibles.
Thus, to overcome the complexities, it first differentiates between technology and innovation and then provide a fundamental factor behind all the various concepts. For this, the literature on the history and philosophy of science, technology, and innovation were referred. In these literature, technology is understood as the application of rational knowledge, science (theoria), and techniques combined for useful purposes (Salomon, Citation1984). The tangible and intangible goods, arts, and techniques they create to satisfy human fantasies are differentiated as technological products, rather than technologies.
Accordingly, technology is defined here as a rational and replicable practice of striving to know the means of pursuing self-empowerment. This definition implies that technology is a means to self-empowerment. Empowerment refers to an individual’s ability to overcome economic, social, and natural inefficiencies. It distinguishes technology as inquisitive in creating new products that are replicable and separable within a given environment. It is a practice that follows neither existing procedures (routine or technical) nor behavioral conditioning (wisdom). A practice can be technical which mean an action that has no separate end product. A wisdom can be a practice but implies an act of conditioning to develop a new or existing model or habit by frequently sending volitional orders. From this, a practice that refers to technology is an act that create or improve knowledge in the art of production through a replicable process.
On the other hand, innovation reflects a person’s deviation from ordinary rules or understanding (Godin, Citation2015). It is intentional and is not an effect of biological or physiological evolutionary changes. This does not mean that all kinds of deviations give rise to innovation for some bad ideas, inventions, and costly designs that may have no social or market value (Fagerberg & Verspagen, Citation2009). Innovation can be technological (R&D driven ideas or inventions), behavioral (deviation from ordinary rites, thinking, habits, etc.), organizational, and institutional setups. Innovation starts with the generation of ideas and ends with the realization of its value in the market. It is not essential to innovate by following scientific theory or laboratory experiments, as one can follow a new marketing strategy without any controlled experiment in advance. Thus, this study considers innovation, in its general sense, as a value-based departure from past practice or thought.
In the end, the use of various terminologies are summarized in depicting the complexity of the human learning process. Innovation is understood as the general process of learning in human intellect. The categories technology, technical, scientific, and non-scientific are spaces of intellectual learning. The various conjugations are the input, process, output, and outcome indicators in each learning space. For instance, technological change is used to imply the immediate output in the technological space, like a new car design, while in the same space technological progress is used to refer the outcome, a degree of advancement in car due to a new design. In this sense, the integral effect or outcome of all these types of learning defines intellectual capital progress or growth.
The knowledge management literature defines IC as a possession of knowledge, experience, organizational technology, customer relationships, and professional skills that provide a competitive edge in the market (Edvinsson & Malone, Citation1997). It divides national IC into: renewal capital (RC), human capital (HC), relational or marketing capital (MC), and process capital (PC) (Michalczuk & Fiedorczuk, Citation2017). This notes IC as an integral of R&D, skills and education, social and marketing, organizational and institutional capabilities which facilitate the creation of valuable products and services. In this sense, IC can effectively resolve the various conceptual complexities.
The second source of difference among growth models is rooted in the modes of idea generation. For exogenous growth models, technological progress was not exclusively knowledge and assumed to have evolved spontaneously. Contrarily, both evolutionary and endogenous growths recognize technological change as knowledge. However, the evolutionary models remained intact on Nelson and Phleps’s assumption of exogenous theoretical knowledge. Thus, they rely overly on individuals’ bounded rationality to strengthen the view that knowledge of the best technological practice comes through market selection among blindly acquired heterogeneous routines. They also critically challenge new endogenous growth models assuming non-linearity between basic, theoretical, and technological knowledge.
By contrast, endogenous growth models assume a linear relationship between theoretical and technological knowledge. Hence, they sharply defined knowledge production as a function of intentional effort put on research and a stock of ideas implicit in previous designs or coded in books. However, this R&D function faces criticism from two perspectives. The first is the consideration of labor as an input leads to scale effect known after Jones (Citation1996). Second the R&D function faces criticism for assumption of linearity between new ideas and stocks of previous knowledge. Besides, Weitzman (Citation1998) argues the relation to be combinatoric.
With regard to the issue of scale effect, this study argues that the blemish was fundamentally entrenched with the assumption of ‘research effort’ as an input in the idea production function. All endogenous growth models, including Jones (Citation1996), hailed the assumption as a micro-foundation, individuals drive new ideas. To counter this argument this study goes back to Griliches (Citation1973) to realize the philosophical reasoning behind defining knowledge (K) = w(R). It asks to know why did Griliches had chose to measure research effort as spending rather than labor units and treat it as an indicator of knowledge rather than an input factor creating knowledge? It was because there is no way to measure the amount of knowledge employed by a firm or an individual engaged in innovation. This implies that knowledge is the sole input for new knowledge production. The research effort measured as R&D spending (R) or numbers of researchers (LA) are the indicators of current knowledge. Researchers are considered as input in knowledge production only for they carry a potential indicator of the knowledge embodied in them. In both research lab and field experiments, physical capital or labor in absolute terms is not an input for new-idea production. Although there is physical labor, equipment, infrastructure, and energy, including the laboratory room, the role of dexterity of an engineer and services of all these assets is the same as the manual labor or materials in the final goods production, and thus, their absolute value should be accounted once in no way different from the general labor or capital account. Thus, the ratio of specialists and equipment specific to idea-generation activities can be indexed as a potential indicator of the current technological knowledge.
Weitzman (Citation1998) argument is to provide hybrid corn as an analogy for new idea production. But, one could note that before the process of hybridizing corn, there is a design or idea of recombining varieties of cultivars derived from the basic knowledge of genetic engineering. In this sense, the hybrid corn, the product of recombination of various genes, but the design or idea of hybridizing a corn is not a recombination of various previous ideas. Products can be recombined and reconfigured but ideas are cumulative and path-dependent. Thus, the variety of ideas either external or internal feeds each other not in a complementary fashion, but supplementarily enhances an innovator for new insights.
Differently, this study approaches as an intermediary between the endogenous and evolutionary growth models. It basis on Romer’s idea generation but, Romer’s concept still faces certain limitations. Despite, Romer’s (Citation2010) great pitch to combine rules with technological ideas, he remained focused on technological ideas and far from recognizing non-R&D innovations. Besides, Romer’s scope of ideas is not only limited to technological ideas but also confined to R&D sectors of advanced countries.
Thus, a primary modification pertains to the generalization of ideas. Technological ideas are key to the advancement of technological goods and services, but are not the sole essential factor in the process of creating more value. The new technological product must be absorbed and effectively employed by many, and a new production system must be reinstalled and managed effectively. Marketing chains and channels of distribution and pricing mechanisms must be developed so that new production processes can be more productive and generate more value and national GDP. This suggests that the scope of ideas or knowledge must be extended to include all the ideas necessary for the creation of value or output. As summarized in , humans learn or generate ideas not only through technological innovation but also through technical, scientific, and social innovations. In other words, the creation of more value or output growth requires change in all varieties of ideas, summed as IC change or growth. However, the change or growth of each of these ideas requires the base knowledge (existing stock of knowledge) or IC level as the input for learning.
Accordingly, the process of generating new ideas must be redefined as a function of IC level. As shown in , IC level is an integral capability or broad indicator of the existing stock of knowledge (At), which determines the flow of new ideas. In other words, the flow of new ideas () is, by definition, an effect of the change in the IC level (ΔIC):
in discrete terms. From these,
(1)
(1)
EquationEquation (1)(1)
(1) implies that both the input (At) and output (
) of the innovation sector are broader in scope, including technological, technical, and socialFootnote1 knowledge or ideas linked to production inputs, outputs, processes, management, and marketing. This new function is expected to have two advantages. First, the generation of new ideas as a function of changes in IC level, an index of various factors, effectively dilutes the scale effect. Second, the growth of the IC index is supposed to be directly proportional to the growth of new ideas and, hence, could avoid empirical puzzles and accompanying unnecessary explanations. The question is how are the various categories of broader knowledge engaged in the value creation process or final goods sector? Romer (Citation1990) differentiates technological ideas or knowledge (A) embodied in intermediate goods (x) as non-rivals and knowledge embodied in humans (H) as rivals. In the final goods production of,
(2)
(2)
Technological knowledge is assumed to affect the final goods sector as it is instantly transformed into intermediate goods. Human capital enters the final goods sector as an independent factor in addition to ordinary labor (L). However, Jones (Citation1996) defines human capital as skill and experience related to new technological products, and thus modified Romer’s final goods production as:
(3)
(3)
In this specification, human capital (h) is skill or experience related to advanced technological goods. Consequently, the maximum range of innovative ideas is proportional to the current level of human capital. For Comin (Citation2004), all intangibles other than technological ideas are assumed to be Hicks-neutral. Others considered human capital as labor augmenting and the rest of the non-technological ideas as Hick-neutral.
As argued above, however, the generation of various ideas or knowledge needs to be recognized as a function of the national IC of agents (R&D or non-R&D firms) placed in the global North or South. In other words, the level of the existing stock of all these varieties of knowledge does not directly determine the final output production process. Then, the acquired design of the product, process, and skill affects the final output production as embodied either in the form of capital goods or augment labor as disembodied knowledge. Thus, final goods production takes the form:
(4)
(4)
The only difference from Romer (Citation1990) is that all categories of knowledge, skills and experiences, technological knowledge, and other non-technological knowledge are integrated as ideas. This approach is appealing for two reasons: First, the acquired basic skills and experiences of individuals are initially ideas that are taken up, learned, or absorbed by ordinary labor in the same way as intermediate goods. Second, the basic skills and experiences of individuals are the effects of technological or non-technological knowledge, such as social, organizational, and institutional innovations, and all affect output production through labor (inventors, managers, and workers) or capital goods. Given these, this study includes human capital not as an independent factor directly affecting output production, but as part of IC.
As in Romer (Citation1990), given an infinite possible new ideas the maximum valuable ideas could be equal to Thus, the relation in Equation(4)
(4)
(4) takes the following form:
(5)
(5)
with the assumption of symmetry and unit intra & inter elasticity of substitution. Holding
as in Romer the final output (Y) takes:
(6)
(6)
Taking the natural log after (Equation6(6)
(6) ) and then its derivative with respect to time, while considering (LA) as the interaction term, gives:
(7)
(7)
Alternatively, Equationequation (6)(6)
(6) can be transformed to per worker terms as:
(8)
(8)
and after taking derivative with respect to time:
(9)
(9)
where A refers to the maximum number of new broader ideas generated proportional to the existing level of IC, that is,
and
2.3. Empirical literature
The concept of IC goes back to J. Kenneth Galbraith in 1969, but it is still scarce to find studies with IC heading in the growth literature (Sokół, Citation2017). The literature on IC is mostly at firm level and is based on accounting and management concepts (Pedro et al., Citation2018). It realized IC as a key driver of innovation and, hence, a core factor in the value creation process and competitiveness through innovation of efficient products, processes, and marketing ideas (Kim & Kumar, Citation2009). However, the focus of this study is on the effect of national IC; therefore, studies with too narrow definitions of IC and at micro-level are not part of this review.
At the macro level, a few studies have analyzed the correlation between the national IC index and economic growth (Alfaro et al., Citation2014; Jednak et al., Citation2017; Kuzkin et al., Citation2019; Marcin, Citation2013; Ståhle et al., Citation2015; Uziene, Citation2014). They found that countries with higher national IC levels had higher GDP per capita. Mačerinskiene and Aleknaviciute (Citation2017) carried out a regression analysis and found a significant impact of the national IC index on economic growth. However, they were too eclectic in their model specification; their model omitted all the conventional factors without referring to any theoretical models.
Meanwhile, there is a vast amount of literature relating only some components of IC to economic growth without explicitly referring to the term IC. These are mostly based on new endogenous growth models that emphasize the contributions of human capital, R&D, knowledge spillovers (such as FDI and openness), and institutions (property rights, financial, and marketing regulations).
Theoretically, human capital can affect economic growth both directly as an input in the production process (Lucas, Citation1988) and indirectly through TFP (Nelson & Phelps, Citation1966). This indirect effect implies that human capital determines the effective management and absorption of tangible and intangible resources.
However, surveys of empirical studies relating human capital to economic growth have documented an array of time series and panel analyses with mixed results. The report shows the positive, weak, or even negative impact of human capital on economic growth (Osiobe, Citation2019; Rossi, Citation2020). They attribute the observed non-linearity of evidence to variations in measurement and other complementary factors, such as institution and cultural setups. Although educational attainment is fairly high, the advantage of new ideas can only be derived if entrepreneurs are free to earn profits (Jones, Citation1996). Rossi (Citation2020) concluded that the average educational attainment gap is not the primary driver of cross-country gaps in economic performance.
From endogenous theory, current R&D effort play a key role in adding a new knowledge pool and improving the final output production process. In this sense, R&D effort could be a source of productivity directly by improving intermediate goods and processes and indirectly by adding an idea to the future potential for research (Coe & Helpman, Citation1995). Due to the non-excludability feature, domestic R&D builds the capacity to absorb externally provided knowledge (Cohen & Levinthal, Citation1989). Lichtenberg and De La Potterie (Citation1998) found that the more open a country is to trade with research-intensive countries, the more likely it is to benefit from foreign R&D. In contrast, Kao et al. (Citation1999) found no effect for trade-related foreign R&D. Others, shifted from the direct measurement of spillovers to the control of knowledge transfer channels, institutions, and infrastructures that facilitate foreign knowledge flow. For instance, many used FDI to account for foreign knowledge, but the results have been inconclusive. Importantly, for Coe et al. (Citation2009) and Pietrucha et al. (Citation2018) the effect of knowledge spillover depends on the host country’s market orientation, financial markets, human capital, and the way of doing business or institutions.
Overall, the empirical results indicate that human capital, R&D, and its spillover channels explain little of the cross-country differences in output or productivity growth. This might be because these lists are only part of the integral behind the general process of innovation that affects economic growth.
3. Methods
To estimate Equationequation (7)(7)
(7) , a panel data of 29 countries drawn from a Groningen growth and development centre for a period of 1990–2020 were used (see ). The sample frame was chosen for its sound classification and for data consistency and availability. However, the time dimension was limited due to prevalence of missing data for most developing countries for time prior to 1990. Moreover, some countries like China (mainland, Hong Kong, and Taiwan), India and Costa Rica, were dropped for population size and administrative factors. Some missing of data within the period were linearly interpolated and total missing of labor share for some SSA countries were filled by taking regional average.
Details of the variables, parameter definitions, and sources are provided in . For construction of IC index various indicators were adapted from Ståhle et al. (Citation2015) as summarized in (in the appendix). All the necessary data were sourced from World Development Indicators (WDI) database. As shown on at least five various quantitative and qualitative indicators are selected to form a single component of national IC. To integrate these indicators as national IC index of a given nation the simple additive or weighted linear combination method was adapted from Uziene (Citation2014) and Thakkar (Citation2021). Accordingly, each individual indicator noted as positive contributor of national IC was first normalized by dividing each indicator’s series of values by its respective overall maximum value across all nations and overtime (j max). That is: For indicators assumed to possess a dragging-out effect or whose higher value implies conditions of poor IC, the overall minimum(j min) was dividend for the series of entries. That is
Where, i = 1, 2,3 & 4 _referring to the sub-components of IC; j = 1, 2,…, 5 or 6 refers to the various indicators and t = period from 1990 to 2020.
Table 1. Variable definitions and data sources.
In this way each indicator was normalized to a ratio between 0 and 1 to avoid the unnecessary effects of variations coming from differences in measurement units across indicators. Second the simple average of the normalized indicators of a given component is taken as an index of that particular component of a nation at that particular year. Finally, the simple summation of the four indices makes up a national IC index in a given year. While, taking average and summation each indicator or sub-indices was given an equal weight, to allow Solow’s (Citation1963) argument for equal role of embodied technological and disembodied technical progress or this time social innovation and technological innovation capabilities.
The choice of an appropriate estimation method depends on the cross-sectional and time-series properties of the data. The data were diagnosed for cross-sectional dependence (CSD), slope homogeneity, structural breaks, panel unit root, and co-integration properties prior to estimation. The result in shows rejection of null hypothesis of weak CSD for both variables at 1%, and the estimated exponent of dependence (α) was greater than 0.5. This implies the existence of strong CSD among cross-sectional units, suggesting the need to look for estimation and test methods that address CSD. Accordingly, the Blomquist and Westerlund (Citation2013) test of slope heterogeneity was used, as it accounts for the CSD. Following Bersvendsen and Ditzen (Citation2021) argument, the test was allowed to be a heteroskedastic autocorrelation (HAC) robust estimator. As shown in , the delta values are significant at the 1% level, implying that the slopes are heterogeneous.
Table 2. The CD and slope homogeneity test.
Given the evidence of CSD and slope heterogeneity, a second-generation panel unit root test was used to investigate the integration levels of the variables. In , the results of the CIPS test show that all variables are stationary in level, both with and without a trend. Moreover, expecting the possibility of unknown structural breaks, we used the Karavias and Tzavalis (Citation2014) panel unit root test recently developed by Chen et al. (Citation2022), which accounts for unknown structural breaks. The result from the new command once again confirms that the variables are stationary at level, both with and without a linear trend, while considering single and double unknown structural breaks.
Table 3. Stationarity test.
Finally, all four statistics from Persyn and Westerlund (Citation2008) error-correction-based co-integration test were significant at the 1% level for all independent variables. Thus, the rejection of the null hypothesis of no co-integration implies that all regressors are strongly co-integrated with output growth.
Following the dimensional specification of the data and the nature of the variables, Equationequation (7)(7)
(7) can be better stated as a dynamic macro panel as follows:
(10)
(10)
In the pre-estimation tests, the rejection of cross-sectional independence and slope homogeneity was a practical challenge in obtaining an estimator that could reconcile these with stationarity properties in dynamic macro panels. The pooled mean group and panel dynamic OLS approaches are useful for slope heterogeneity, but do not allow for error CSD. A strong CSD implies the possible correlation between common factors and regressors could cause bias and inconsistency.
This study followed Chudik and Pesaran (Citation2015) more recent and profound common correlated effect approach to CSD in the context of dynamic macro panels. The approach treats CSD as part of an error term Accordingly, Equationequation (10)
(10)
(10) becomes
(11)
(11)
where
is the unobserved common factor and p is the maximum number of lags. Following Pesaran (Citation2006) and Chudik and Pesaran (Citation2015) in their successive works, they approximated the common factors by taking cross-sectional averages of the contemporaneous dependent and independent variables (
) with a floor of
= PT lags. That is,
(12)
(12)
where T refers to the time dimension of the panel data and vi is a unit-specific fixed effect.
β, and γ are the heterogeneous slopes and factor loadings across units, respectively, and ei is the white noise. Chudik and Pesaran (Citation2015) proposed a mean group estimation using a cross-sectional augmented autoregressive distributed lag (CS-ARDL) for a dynamic macro panel. Thus, Equationequation (12)
(12)
(12) was estimated as dynamic common correlated mean group (DCC-MG) and CS-ARDL, following Ditzen’s (Citation2021) xtdcce2 version 3.01 stata command. The command directly estimates long-run relationships and provides comparable results for both estimators. The CCE approach is preferred because it adds the averages of the independent and dependent variables to approximate common factors without prior information.
4. Result and discussion
provides the summary of descriptive statistics of the data across income categories. The mean IC growth shows direct proportionality with output growth, both decreasing across income levels from the low income countries (LIC) to high income countries (HIC). However, the mean IC level increased across income levels from the LIC to the HIC. The 2.4 percent mean IC growth recorded for LIC is plausible given their previous lower IC levels. The three emerging Asian countries (AEC) possessed the highest mean IC and output growth. Previous studies have linked miracle growth with human capital; however, the data show a direct proportionality with IC growth.
Table 4. Mean growth of IC and output.
Moreover, the trend of the mean IC index for HIC countries and the USA shows a continued but sluggish rise. The mean IC index moderately improved in the other groups. As a result, the mean IC index for AEC exceeded the mean of HIC, excluding the USA (see ).
However, as shown in , the mean IC growth first rises and then decreases for all economies, despite differences in the time period. The high rate of IC growth for LIC reflects that they are improving their quality of human capital, and social and technological innovations relative to the previous lower status reached a maximum by 2015. The USA and other advanced countries attained their maximum IC growth sometime earlier in this sample period, and hence experienced declining progress throughout the sample period. However, the huge fall for advanced countries could be partly attributed to deteriorating relational, marketing, and social capital indicators. In particular, this implies that the knowledge inflow from the rest world to advanced economies is weakening relative to the outflow, as indicated by FDI. For instance, the USA is still at the frontier of technological knowledge, but its innovation level may fall because of incumbent rivalry and restrictive policies.
presents the estimation result for the specified model where the output growth (y_g) is a dependent variable. The first 3 columns to the right of variables column report a DCC-MG and CS-ARDL estimates for full sample of which the third is regressed without lags for an interaction factor (lic_g). The next 3 columns shows specific results for groups and economic regions: high income (HIC), African and Asian countries. For all, the post-estimation CD-test statistic is very small and insignificant, implying the effectiveness of the common correlated effect approach used. As Chudik et al. (Citation2016) and Ditzen (Citation2018, Citation2021) suggested, the MG estimator provided an equivalent short-run estimates to CS-ARDL. Except for the lag of dependent variable, the slope coefficients for all the important variables, including the adjustment term, carry the expected sign and are statistically significant. Moreover, for all estimators the standard errors are lower. All these imply, the specified model fits well with data and the chosen estimators.
Table 5. Estimation result for output growth (y_g) as dependent variable.
The coefficients for the adjustment term are almost equal to those from CS-ARDL, implying that the distortions from the equilibrium can be fully recovered within a period. The mean group estimate coefficients from DCC-MG and CS-ARDL show that a 10 percent rise in capital contributes to an output growth of about 1.8 percent. Meanwhile, a 10 percent growth of IC augmented labor brings about 1.4 percent to 2.4 percent of the nation’s output growth in the short and long run, respectively. However, the slope coefficients of IC augmented labor are higher for the subgroups. Particularly, for Asian countries, the effect increases to 3.5 percent for every 10 percent, implying that output growth in Asian countries is relatively more fueled by IC.
The results reflect that IC has a consistent and significant positive contribution to national output growth across various income levels. As shown in the descriptive statistics, the mean level of IC and IC augmented labor proportionally increases across income levels, but their mean growth rate falls. Given this, the positive coefficient of growth in IC-augmented labor implies that the high rate of output growth observed for developing countries relative to advanced countries is not due to mere growth in labor force, capital accumulation, or R&D effort. Particularly, the AEC countries possesses both higher IC levels next to HIC and higher IC growth next to LIC, and hence experienced the highest output growth of 5.4 percent. The observed proportionality between IC and economic growth is also consistent with the findings of Alfaro et al. (Citation2014), Ståhle et al. (Citation2015) and Kuzkin et al. (Citation2019). On the other hand, the result contrasts with studies which attribute the constant lower rate of output growth in advanced countries to difficulty of obtaining new ideas. The argument here is the cause of lower output growth in advanced countries goes beyond a usual narrow concept of technological progress. To a great extent, it could be an integral effect technological and non-technological innovations.
Moreover, the result is also robust to changes in the estimator and dependent variable, and hence, changes in estimable models and variation in sample size. In the first case, the results from the DCC-MG and CS-ARDL estimators are almost the same for the full-sample case, as shown in . Second, the dependent variable, measured as the output growth of a given nation in , is replaced by the growth in output per worker. presents the estimation results per specification model (9). The result is fundamentally the same, but the coefficients of IC growth are less significant in the short run. Third, the specified models (7) and (9) are now estimated for 13 high-income countries, 8 OECD countries, 8 SSA countries plus Morocco, and 7 Asian countries, as presented in . Nonetheless, the results are generally robust to changes in estimation method, model, and sample size.
Table 6. CS-ARDL result of output per worker growth (yl_g) as dependent variable.
5. Conclusion
This study analyzes the effects of intellectual capital on economic growth. It provides intellectual capital as an integral of all intangible capabilities: human capital, R&D knowledge and its spillovers, and all other knowledge from non-R&D innovations. Based on this, it argues to resolve conceptual complexities and provide a consistent empirical explanation for differences in national output growth. To do so, the flow of new ideas was defined as a function of change in IC level. By integrating a new idea production function into Romer’s (Citation1990) final goods production it derive a new model with IC augment labor.
The model was estimated using dynamic common correlated effect estimators for a panel of 29 countries from all income levels and geographic regions from 1990 to 2020. The results show the existence of significant and positive short- and long-run links between output growth and IC growth. This result confirms the hypothesis that economic growth is a function of national IC growth, an integral factor behind the broader innovation process. Hence, to improve economic growth, policymakers need to enhance innovation in all spaces (technological, technical, basic science, and arts) and phases (product, process, and marketing). However, lack of adequate and rigorous data for some key indicators was a challenge for the construction of national intellectual capital index in developing countries. Thus, future studies should take into account additional tests of robustness and various scenario analyses to ensure rigorous measurement of IC indicators.
Authors contribution
Kalalto Gashe, Zerayehu Sime and Melkamu Mada participated in conducting the study. Kalalto Gashe a PhD candidate in development economics had made a substantial contributions in the conception and design; acquisition, analysis and interpretation of data and drafting. Both Zerayehu Sime (PhD) is an associate professor of economics, and an editor-in-chief of EJBE in Addis Ababa University and Melkamu Mada (PhD) is an associate professor of economics from Arba Minch University. Zerayehu Sime and Melkamu Mada had participated in designing and critical revision of the work for important intellectual content. Finally, all the three authors approved the final version to be published and agreed to be responsible for all aspects of the work published.
Data availability statement
The data supporting the findings of this study are openly available in figshare at 10.6084/m9.figshare.22692550 or https://figshare.com/s/645ce6d7d0a15981ad67.
Disclosure statement
No potential conflict of interest was reported by the author(s).
Notes
1 Social comprises ideas or knowledge of innovation in: R&D social sciences, behvioral arts and wisdom, organizational and public management and institutions.
References
- Abramovitz, M. (1956). Resource and output trends in the United States since 1870. The American Economic Review, 46(2), 5–23.
- Aghion, P., & Howitt, P. (1992). A model of growth through creative destruction. Econometrica, 60(2), 323–351. https://doi.org/10.2307/2951599
- Alfaro, J. L., Lopez, V. R., & Nevado, D. (2014). Economic growth and intangible capitals: Europe versus Asia. Panoeconomicus, 61, 261–274.
- Arndt, H. W. (1981). Economic development: A semantic history. Economic Development and Cultural Change, 29(3), 457–466. https://doi.org/10.1086/451266
- Bersvendsen, T., & Ditzen, J. (2021). Testing for slope heterogeneity in Stata. The Stata Journal: Promoting Communications on Statistics and Stata, 21(1), 51–80. https://doi.org/10.1177/1536867X211000004
- Blomquist, J., & Westerlund, J. (2013). Testing slope homogeneity in large panels with serial correlation. Economics Letters, 121(3), 374–378. https://doi.org/10.1016/j.econlet.2013.09.012
- Bohm-Bawerk, E. (1891). The positive theory of capital (W. Smart, Trans.), Macmillan.
- Chen, P., Karavias, Y., & Tzavalis, E. (2022). Panel unit-root tests with structural breaks. The Stata Journal: Promoting Communications on Statistics and Stata, 22(3), 664–678. https://doi.org/10.1177/1536867X221124541
- Chudik, A., & Pesaran, M. H. (2015). Common correlated effects estimation of heterogeneous dynamic panel data models with weakly exogenous regressors. Journal of Econometrics, 188(2), 393–420. https://doi.org/10.1016/j.jeconom.2015.03.007
- Chudik, A., Mohaddes, K., Pesaran, M. H., & Raissi, M. (2016). Long-run effects in large heterogeneous panel data models with cross-sectionally correlated errors. In R. C. Hill, G. Gonzalez-Rivera, & T.-H. Lee (Eds.), Essays in honor of Aman Ullah (Advances in econometrics) (vol. 36, no. 135). Emerald Group Publishing Limited.
- Clark, J. B. (1886). The philosophy of wealth: Economic principles newly formulated. GINN & Company.
- Coe, D. T., & Helpman, E. (1995). International R&D spillovers. European Economic Review, 39(5), 859–887. https://doi.org/10.1016/0014-2921(94)00100-E
- Coe, D. T., Helpman, E., & Hoffmaister, A. (2009). International R&D spillovers and institutions. European Economic Review, 53(7), 723–741. https://doi.org/10.1016/j.euroecorev.2009.02.005
- Cohen, W. M., & Levinthal, D. A. (1989). Innovation and learning: The two faces of R&D. The Economic Journal, 99(397), 569–596. https://doi.org/10.2307/2233763
- Comin, D. (2004). R&D: A small contribution to productivity growth. Zootaxa, 5420(1), 1–121. https://doi.org/10.1007/s10887-004-4541-6
- Dinopoulos, E., & Thompson, P. (1998). Schumpeterian growth without scale effects. Journal of Economic Growth, 3(4), 313–335. https://doi.org/10.1023/A:1009711822294
- Ditzen, J. (2018). Estimating dynamic common-correlated effects in Stata. The Stata Journal: Promoting Communications on Statistics and Stata, 18(3), 585–617. https://doi.org/10.1177/1536867X1801800306
- Ditzen, J. (2021). Estimating long-run effects and the exponent of cross-sectional dependence: An update to xtdcce2. The Stata Journal: Promoting Communications on Statistics and Stata, 21(3), 687–707. https://doi.org/10.1177/1536867X211045560
- Ditzen, J., Karavias, Y., & Westerlund, J. (2021). Testing and estimating structural breaks in time series and panel data in Stata. arXiv:2110.14550v2.
- Domar, E. D. (1961). On the measurement of technological change. The Economic Journal, 71(284), 709–729. https://doi.org/10.2307/2228246
- Edvinsson, L., & Malone, M. S. (1997). Intellectual capital: Realizing your company’s true value by finding its hidden brainpower. Harper Business.
- Fabricant, S. (1954). Economic progress and economic change. In 34th Annual Report of NBER.
- Fagerberg, J., & Verspagen, B. (2009). Innovation studies-The emerging structure of a new scientific field. Research Policy, 38(2), 218–233. https://doi.org/10.1016/j.respol.2008.12.006
- Fan, J., Liao, Y., & Yao, J. (2015). Power enhancement in high-dimensional cross-sectional tests. Econometrica, 83(4), 1497–1541.http://www.jstor.org/stable/43616976. https://doi.org/10.3982/ECTA12749
- Feenstra, R. C., Inklaar, R., & Timmer, M. P. (2015). The next generation of the Penn World Table. American Economic Review, 105(10), 3150–3182. https://doi.org/10.1257/aer.20130954
- Godin, B. (2015). Innovation: A conceptual history of an anonymous concept.
- Gordon, R. J. (2018). Why has economic growth slowed when innovation appears to be accelerating? NBER WP24554. National Bureau of Economic Research.
- Griliches, Z. (1957). Hybrid corn: An exploration in the economics of technological change. Econometrica, 25(4), 501–522. https://doi.org/10.2307/1905380
- Griliches, Z. (1973). Research expenditures and growth accounting. In B. R. Williams (Ed.), Science and Technology in Economic Growth. International Economic Association Conference Volumes, Numbers 1–50. London: Palgrave Macmillan. https://doi.org/10.1007/978-1-349-01731-7_3
- Haavelom, T. (1954). A study in the theory of economic evolution. North-Holland Publishing Company.
- Hayek, F. A. (1935). The maintenance of capital. Economica, 2(7), 241–276.
- Howitt, P. (1999). Steady endogenous growth with population and R&D inputs growing. Journal of Political Economy, 107(4), 715–730. https://doi.org/10.1086/250076
- Howitt, P., & Aghion, P. (1998). Capital accumulation and innovation as complementary factors in long-run growth. Journal of Economic Growth, 3(2), 111–130. https://doi.org/10.1023/A:1009769717601
- Jednak, S., Dmitrovic, V., & Damnjanovic, V. (2017). Intellectual capital as a driver of economic development. Journal of Economics and Business, 15(2), 77–84.
- Johnston, R. E. (1966). Technical progress and innovation. Oxford Economic Papers, 18(2), 158–176. https://doi.org/10.1093/oxfordjournals.oep.a041016
- Jones, C. I. (1995). R&D-based models of economic growth. Journal of Political Economy, 103(4), 759–784.http://www.jstor.org/stable/2138581
- Jones, C. I. (1996). Human capital, ideas, and economic growth. Palgrave Macmillan UK.
- Kaldor, N. (1954). The relation of economic growth and cyclical fluctuations. The Economic Journal, 64(253), 53–71. https://doi.org/10.2307/2227090
- Kao, C., Chiang, M., & Chen, B. (1999). International R&D spillovers: An application of estimation and inference in panel cointegration. SSRN Electronic Journal, 61(51), 691–709. https://doi.org/10.2139/ssrn.1807962
- Karavias, Y., & Tzavalis, E. (2014). Testing for unit roots in short panels allowing for a structural break. Computational Statistics & Data Analysis, 76(c), 391–407. https://doi.org/10.1016/j.csda.2012.10.014
- Kim, D.-Y., & Kumar, V. (2009). A framework for prioritization of intellectual capital indicators in R&D. Journal of Intellectual Capital, 10(2), 277–293. https://doi.org/10.1108/14691930910952669
- Kortum, S. S. (1997). Research, patenting, and technological change. Econometrica, 65(6), 1389–1420. https://doi.org/10.2307/2171741
- Kuzkin, Y., Cherkashyna, T., Nebaba, N., & Kuchmacz, B. (2019). Economic growth of the country and NIC. Problems and Perspectives in Management, 17(1), 348–359. https://doi.org/10.21511/ppm.17(1).2019.30
- Laudardale, E. (1819). An inquiry into the nature and origin of public wealth and in to the means and cause of its increase (1804).
- Lichtenberg, F. R., & De La Potterie, B. V. P. (1998). International R&D spillovers: A comment. European Economic Review, 42(8), 1483–1491. https://doi.org/10.1016/S0014-2921(97)00089-5
- Lucas, R. E. (1988). On the mechanics of economic development. Journal of Monetary Economics, 22(1), 3–42. https://doi.org/10.1016/0304-3932(88)90168-7
- Mačerinskiene, I., & Aleknaviciute, R. (2017). National intellectual capital influence on economic growth in the EU. Quarterly Journal of Economics and Economic Policy, 12(4), 573–592. https://doi.org/10.24136/eq.v12i4.30
- Marcin, K. (2013). Intellectual capital as a key factor of socio-economic development of regions and countries. Procedia Economics and Finance, 6, 288–295. https://doi.org/10.1016/S2212-5671(13)00142-1
- Marshall, A. (1890). Principles of economics (8th ed.). Palgrave Classics in Economics.
- Metcalfe, S. (2009). Technology and economic theory, Papers on economics and evolution, No. 0909, Max-Planck-Inst.
- Michalczuk, G., & Fiedorczuk, J. (2017). Macroeconomic perspective of intellectual capital. Optimum. Studia Ekonomiczne, 5(89), 117–133. https://doi.org/10.15290/ose.2017.05.89.08
- Mill, J. S. (1848). Principles of political economy with some of their application to social philosophy (7th ed.). Longmans.
- Nelson, R. R. (1956). A theory of the low-level equilibrium trap in underdeveloped economies. The American Economic Review, 46(5), 894–908.
- Nelson, R. R., & Phelps, E. (1966). Investment in humans, technological diffusion, and economic growth. The American Economic Review, 56, 69–75.
- Nelson, R. R., & Winter, S. (1982). An evolutionary theory of economic change. Harvard University Press.
- Nelson, R. R., & Winter, S. (2002). Evolutionary theorizing in economics. Journal of Economic Perspectives, 16(2), 23–46. https://doi.org/10.1257/0895330027247
- Osiobe, E. U. (2019). A literature review of human capital and economic growth. Business and Economic Research, 9(4), 179. https://doi.org/10.5296/ber.v9i4.15624
- Pedro, E., Leitão, J., & Alves, H. (2018). Back to the future of intellectual capital research: A systematic literature review. Management Decision, 56(11), 2502–2583. https://doi.org/10.1108/MD-08-2017-0807
- Peretto, P. (1998). Technological change and population growth. Journal of Economic Growth, 3(4), 283–311. https://doi.org/10.1023/A:1009799405456
- Persyn, D., & Westerlund, J. (2008). Error-correction–based cointegration tests for panel data. The Stata Journal: Promoting Communications on Statistics and Stata, 8(2), 232–241. https://doi.org/10.1177/1536867X0800800205
- Pesaran, M. H. (2006). Estimation and inference in large heterogeneous panels with a multifactor error structure. Econometrica, 74(4), 967–1012. https://doi.org/10.1111/j.1468-0262.2006.00692.x
- Pesaran, M. H. (2007). A simple panel unit root test in the presence of CSD. Journal of Applied Econometrics, 22(2), 265–312. https://doi.org/10.1002/jae.951
- Pesaran, M. H. (2015). Time series and panel data econometrics. Oxford University Press.
- Pesaran, M. H. (2021). General diagnostic tests for CSD in panels. Empirical Economics, 60(1), 13–50. https://doi.org/10.1007/s00181-020-01875-7
- Pietrucha, J., Żelazny, R., Kozłowska, M., & Sojka, O. (2018). Import and FDI as channels of international TFP spillovers. Equilibrium. Quarterly Journal of Economics and Economic Policy, 13(1), 55–72. https://doi.org/10.24136/eq.2018.003
- Romer, P. (1990). Endogenous technological change. Journal of Political Economy, 98(5), S71–S102. https://doi.org/10.1086/261725
- Romer, P. (1993). Idea gaps and object gaps in economic development. Journal of Monetary Economics, 32(3), 543–573. https://doi.org/10.1016/0304-3932(93)90029-F
- Romer, P. (2010). Technologies, rules, and progress: The case for charter cities. Center for Global Development Essay No.1423916.
- Rossi, F. (2020). Human capital and macroeconomic development: A review of the evidence. The World Bank Research Observer, 35(2), 227–262. https://doi.org/10.1093/wbro/lkaa002.
- Salomon, J. J. (1984). What is technology? The issue of its origins and definitions, history and technology. History and Technology, 1(2), 113–156. https://doi.org/10.1080/07341518408581618
- Schultz, T. W. (1956). Reflections on agricultural production, output and supply. Journal of Farm Economics, 38(3), 748. https://doi.org/10.2307/1234459
- Schumpeter, J. A. (1911). [1949]). The theory of economic development: An inquiry into profits, capital, credit, interest, and the business cycle. HU Press.
- Segerstrom, P. S. (1998). Endogenous growth without scale effects. American Economic Review, 88(5), 1290–1310.
- Smith, A. (1776). An inquiry into the nature and causes of the wealth of nations. In E. Cannan (Ed.), Elec book classics.
- Sokół, A. (2017). Diagnosis of IC in macroeconomic terms on the example of Szczecin and opportunities for development of creative sector. HRM& Ergonomics, 11(2), 59–75.
- Solow, R. M. (1956). A contribution to the theory of economic growth. The Quarterly Journal of Economics, 70(1), 65–94. https://doi.org/10.2307/1884513
- Solow, R. M. (1957). Technical change and the aggregate production function. The Review of Economics and Statistics, 39(3), 312–320. https://doi.org/10.2307/1926047
- Solow, R. M. (1963). Capital theory and the rate of return. North-Holland.
- Spiegel, H. W. (1955). Theories of economic development: History and classification. Journal of the History of Ideas, 16(4), 518–539. https://doi.org/10.2307/2707508
- Ståhle, P., Ståhle, S., & Lin, C. (2015). Intangibles and national economic wealth – a new perspective on how they are linked. Journal of Intellectual Capital, 16(1), 20–57. https://doi.org/10.1108/JIC-02-2014-0017
- Thakkar, J. J. (2021). Multi-criteria decision making. Studies in systems, decision and control. Springer. https://doi.org/10.1007/978-981-33-4745-8
- Uziene, L. (2014). National intellectual capital as an indicator of the wealth of nations: The case of the Baltic States. Social and Behavioral Sciences, 156, 376–381.
- Veblen, T. (1908). On the nature of capital. The Quarterly Journal of Economics, 22(4), 517–542. https://doi.org/10.2307/1884915
- Weitzman, M. L. (1998). Recombinant growth. The Quarterly Journal of Economics, 113(2), 331–360. https://doi.org/10.1162/003355398555595
- Young, A. (1998). Growth without scale effects. Journal of Political Economy, 106(1), 41–63. https://doi.org/10.1086/250002
Appendix
Table A1. Sample countries by income group and region.
Table A2. Compositions of intellectual capital index.
Table A3. Conjugated terminologies.