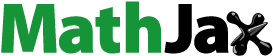
Abstract
The emergence of digital financial systems, especially mobile money, has significantly changed the financial services space in sub-Saharan Africa in ways that promote financial inclusion among the poor, including those in rural communities. Focusing on mobile money, which is the main avenue for digital financial services for rural households in Ghana, this study investigates whether digital finance affects rural households’ livelihood diversification, using a nationally representative data on Ghana. Employing several econometric methods, including instrumental variable techniques to address potential endogeneity bias, the study finds mobile money to be positively associated with households’ choice of non-farm business as a livelihood option as well as their engagement in livestock production. However, we find a negative relationship between mobile money and diversification in crop production including the extent to which households engaged in the crop sector are able to diversify crop production. The implication is that when rural households have access to mobile money services, they produce fewer number of different crops while exploring non-farm activities and livestock production as additional livelihood options. The results underscore the need to address constraints that limit rural households’ access to digital financial tools, especially mobile money, in Ghana and in similar developing country contexts.
Impact Statement
This study employs a nationally representative micro-data from rural Ghana to investigate the effect of digital finance on livelihood diversification using several econometric methods including instrumental variables to address potential endogeneity bias. The study represents a significant attempt at addressing an important gap in the existing literature which is about whether digital finance brings any value addition to livelihood diversification efforts by rural households. The key finding is that when rural agricultural households have access to digital financial services through mobile money, they produce fewer different crops, while exploring non-farm activities and livestock production as additional livelihood options. The finding implies that access to digital finance may influence rural households’ ability to reallocate productive resources to areas that yield higher returns to their livelihoods and/or mitigate the risk of income loss. Expanding upon this finding with an exploration of the key correlates of diversification and access to digital finance, the study shows that addressing constraints to access to digital finance or mobile money (particularly, the limited mobile phone connectivity, electricity access or supply challenges, and low education or digital illiteracy in rural areas) is important for deepening livelihood diversification in rural Ghana.
Reviewing Editor:
1. Introduction
The advent of digital finance (DF), such as mobile money, has significantly changed the financial services space in sub-Saharan Africa (SSA), especially for the poor and the historically unbanked, allowing countries to use innovative technologies to leapfrog the financial development stages that developed countries went through (Aron, Citation2018; Bara, Citation2013). Mobile money has become an important innovative tool for facilitating financial inclusion and enhancing financial market participation (Ahmad et al., Citation2020; Amoah et al., Citation2020; Bongomin et al., Citation2018; N’dri & Kakinaka, Citation2020; Ouma et al., Citation2017) especially in SSA. According to the 2021 Global Findex report, SSA is leading the mobile money revolution, with the share of adults owning mobile money accounts increasing from 12% in 2014 to 33% in 2021, compared with the global share of 2% and 10% in 2014 and 2021, respectively (Asli et al., Citation2022). In addition, differences in financial account ownership in SSA across wealth quintiles have been halved over the past decade, driven mainly by mobile money (Asli et al., Citation2022).
Aside from facilitating financial inclusion, there is growing empirical evidence linking digital finance to various livelihood and welfare outcomes (Atta-Ankomah, Citation2022; Lee et al., Citation2021; Ouma et al., Citation2017; Peprah et al., Citation2020). For instance, in Bangladesh, Lee et al. (Citation2021) found that mobile money increases household consumption, reduces extreme poverty and borrowing, and shore-up savings. Similarly, Peprah et al. (Citation2020) found a positive relationship between mobile money adoption and household outcomes, including farm output, consumption expenditure, and asset value, using data from three districts in Ghana. Ouma et al. (Citation2017) observed that access to digital finance enhances savings behavior and the extent of saving among households in Kenya, Malawi, Uganda, and Zambia. Recent studies using a nationally representative data on Ghana also observed a positive correlation between mobile money account ownership and access to credit, health insurance and remittances (Atta-Ankomah, Citation2022; Atta-Ankomah et al., Citation2024). In addition, recent advances in digital financial technology have allowed poor households with mobile money accounts to receive international remittances (Ahmad et al., Citation2020; Amoah et al., Citation2020). In a more recent study, Okyere et al. (Citation2024a) show that adoption of digital financial services has positive effect on the resilience capacity of farm households in Northern Ghana.
Other existing evidence also suggests a positive effect of digital finance on household investments and production activities. Specifically, for agriculture, which is the main means of livelihood of the rural poor (Davis et al., Citation2017), Miller (Citation2019) documents four channels through which digital finance could impact the sector’s development: (1) savings to help smoothen consumption over time; (2) insurance against possible crop loss; (3) payment and transactions, including cash transfers to rural households; and (4) credit to farmers. A recent study by Okyere et al. (Citation2024b) found that mobile money adoption increases farm performance (including productivity) and welfare of households in Northern Ghana. A. Islam et al. (Citation2018) found that mobile money increased small- and medium-scale enterprise purchases of fixed assets in Uganda, Tanzania, and Kenya, with reduced transaction costs, increased liquidity, and creditworthiness being the main enablers. Ahmad et al. (Citation2020) and Aron (Citation2018) provide more detailed reviews of the extant literature on the micro-economic implications of digital finance.
Digital finance has the potential to revolutionize agri-food systems and livelihood diversification of rural households (Finger, Citation2023), but studies in this area are lacking. In particular, the role of digital finance (mobile money) in influencing rural households’ livelihood diversification, and the extent or form of diversification has remained largely unexplored in the literature especially those on Ghana, although many empirical studies have established a linkage between mobile money and household welfare (Amoah et al., Citation2020; Atta-Ankomah & Okyere, Citation2022; Jack & Suri, Citation2014; Lee et al., Citation2021; Mahama & Maharjan, Citation2017; N’dri & Kakinaka, Citation2020; Peprah et al., Citation2020), as noted above. Also, this evidence gap exists in spite of a growing literature on the drivers of rural livelihood diversifications (Dagunga et al., Citation2022; Loison, Citation2015, Citation2019; Martin & Lorenzen, Citation2016) and the importance of diversification to household economic wellbeing including resilience to various shocks (see Akaakohol & Aye, Citation2014; Asfaw et al., Citation2019; Asmah, Citation2011; Dagunga et al., Citation2020; Mohammed et al., Citation2021). On the drivers of livelihood diversification, Dagunga et al. (Citation2022), for example, found a positive linkage between savings and youth income diversification in five cocoa growing regions of Ghana. Also, access to finance has been highlighted as an important driver of diversification in several other studies (see Batool & Jamil, Citation2019; Khatun & Roy, Citation2012; Loison, Citation2015, Citation2019). Using panel data, Loison (Citation2019) delved into the regional and gender differences as drivers of livelihood diversification in two agricultural regions of rural Kenya. While these studies are insightful, none explored the link between digital finance and rural livelihood diversification.
Against this background, this study examines the linkage between digital finance (mobile money) and rural household livelihood diversification by focusing on three important issues in Ghana. First, we examine the drivers of mobile money adoption among rural households, including the gender dimensions of these determinants. Second, we examine the effect of mobile money on different livelihood diversification options (crop production, livestock production, and non-farm business operations) while exploring whether access to digital finance is associated with a de-emphasis on more traditional (agricultural) production and emphasis on less traditional alternatives in the non-farm sector. Third, we disaggregate the sample by gender of the household head to explore whether mobile money adoption has a gendered-differential impact on livelihood diversification.
This study is vital from both policy and literature perspectives. As noted above, while studies on the micro impact of mobile money have expanded recently, none of the existing studies explore the linkage between mobile money and rural livelihood diversification in Ghana. Thus, the current study will contribute uniquely to the growing literature on digital finance and rural livelihood diversification in Africa. This study’s policy relevance emanates from the gender dimension it explores, the different livelihood options considered, and the fact that rural households in Ghana engage in a portfolio of livelihood activities (Davis et al., Citation2017). In addition, the government of Ghana is implementing a number of livelihood improvement programs and policies to strengthen mobile money service delivery in the country, making the current study relevant in that regard. The policy implications of this study are relevant to other SSA countries with a context similar to that of Ghana.
This study uses nationally representative micro-data from rural Ghana and employs econometric methods (including instrumental variables) to control for the potential endogeneity between mobile money and the livelihood diversification variables. Specifically, we used the penetration of mobile phones at the household level as an instrument. We find that when rural agricultural households have access to digital financial services through mobile money, they produce fewer different crops, while exploring non-farm activities and livestock production as additional livelihood options. In other words, they can diversify into less traditional sources of livelihood. The implication is that access to digital finance may influence rural households’ ability to reallocate productive resources to areas that yield higher returns to their livelihoods and/or mitigate the risk of income loss. These effects pertain to both male-headed and female-headed households, except in the case of the de-emphasis on crop production, which mainly pertains to male-headed households. These findings, together with the key results on other important correlates of diversification and access to digital finance, suggest that constraints to access to digital finance or mobile money, such as limited mobile phone connectivity, access to electricity, and low education or digital illiteracy in rural areas, need policy attention.
The rest of the paper is organized as follows. Section 2 provides a brief contextual background of mobile money and livelihood diversification in Ghana. Section 3 presents the data and empirical strategy used for the study, and Section 4 discusses the results. Finally, in Section 5, we present the conclusions and policy implications of the study.
2. Mobile money and livelihood outcomes in Ghana
Ghana is an interesting country for this study for several reasons. First, mobile phone subscriptions have increased from 13 in 2005 to approximately 123 per 100 people by 2021 (World Bank Microdata Catalog, Citation2023). Similarly, the number of individuals with mobile money accounts soared from about 350,000 in 2012, three years after the launch of mobile money in Ghana to approximately 17.1 million in 2020 (Dabalen & Mensah, Citation2023; Mattern, Citation2018). In fact, Ghana is one of the countries in SSA that has seen a surge in mobile money usage in recent years, and the share of adults aged 15+ years with mobile money accounts increases by 47 percentage points between 2014 and 2021 (13% to 60%), while the share of accounts at financial institutions increased from 35% to 39% over the same period (Asli et al., Citation2022; Mattern, Citation2018). In addition, the joint ownership of bank accounts and mobile money accounts has seen a substantial increase from 23% in 2014 to about 30% in 2021, driven mainly by mobile money (Asli et al., Citation2022).
This surge in mobile money usage in the country could be explained by the regulatory policies of the Bank of Ghana and the financial sector interoperability system introduced jointly by the government of Ghana, central and commercial banks, and the Ghana Chamber of Telecommunications (Oxford Business Group, Citation2018). In addition, the government of Ghana, in collaboration with other development partners, has introduced a number of policies, including the National Financial Inclusion and Development Strategy, the Digital Financial Services Policy, and the Cash-Lite Roadmap, in an effort to improve mobile money services delivery and expand the geographic reach (Ministry of Finance, n.d.).
In 2022, the government of Ghana, through an act of parliament, introduced an electronic levy (E-Levy) to generate revenue, adopting models that appear to have worked in other countries in the region. This policy was not popular among Ghanaians (Amoah et al., Citation2023), probably because of its less pro-poor design. Currently, there are three main telecommunication companies in Ghana (MTN Ghana, Airtel/Tigo, and Vodafone Ghana) providing mobile money services, with MTN Ghana having the largest share.
Agriculture tends to be the major source of income, and hence, the livelihood for the rural poor in SSA and Ghana in particular. Davis et al. (Citation2017) show that about 44% of households in Ghana have farming as their main source of income. In addition, diversification into non-farm income-generating activities is common among the rural poor in the country. More than 23.5% of rural households in Ghana have diverse income portfolios (Davis et al., Citation2017). Despite serving as the main means of livelihood for the rural poor and contributing substantially to the country’s GDP, agriculture in Ghana is dominated by smallholder farmers, and is beset with a myriad of challenges, including low adoption of sustainable agricultural practices, low level of commercialization, and climate change (Asravor et al., Citation2022). Resorting to and diversifying into alternative sources of livelihood, such as non-farm businesses and livestock production, is very common in rural Ghana (Mahama & Maharjan, Citation2017; Mahama & Nkegbe, Citation2021).
3. Methodology
3.1. Data
This study uses secondary micro data from the seventh and most recent round of the Ghana Living Standards Surveys (GLSS 7), a nationally representative survey conducted by the Ghana Statistical Service (GSS) in 2016/2017.Footnote1 The survey was designed to cover 15,000 households, selected through a two-stage probability sampling approach. In the first stage, 1000 enumeration areas (EAs) were randomly selected from all regions of Ghana based on the probability proportional to size (PPS) of each region. Following the household listing of the selected EAs, the second stage involved random selection of 15 households from each EA. Of the 15,000 selected households, 14,009 were successfully interviewed. Of the households interviewed, 7991 (which accounts for about 57%) were located in rural areas. This study focuses on the rural subsample to understand the potential effect of access to digital finance on different forms of livelihood diversification in the rural economy.
GLSS 7 is a multi-topic household survey that provides comprehensive data on household socioeconomic characteristics and engagement in many forms of economic activities. We rely on information on households’ ownership of mobile money accounts, which are the dominant means of digital financial services available to rural households in Ghana, as well as information on their involvement in crop production, livestock production, and non-farm business activities.
3.2. Regression model, measurement of variables and estimation techniques
To investigate the relationship between access to digital finance and livelihood diversification, we first explored the key correlates of each of these two variables in separate regression models. However, in the model for access to digital finance, measures of livelihood diversification are included as independent variables; similarly, access to digital finance enters the models for livelihood diversification as an independent variable. We specify two main models as follows:
(1)
(1)
(2)
(2)
EquationEquation (1)(1)
(1) is the model for access to digital finance, which is measured by the ownership of mobile money accounts by the household, while EquationEquation (2)
(2)
(2) models the correlates of livelihood diversification. X in both equations represents a vector of control variables or other correlates of access to digital finance and livelihood diversification. Momo is a dichotomous measure that takes a value of 1 if at least one member of the household has a mobile money account, but zero otherwise. Div captures household livelihood diversification. Section 3.2.1 explains how livelihood diversification and other variables are measured.
3.2.1. Measures of livelihood diversification and other variables
The measures of diversification used in the study capture diversification within a given livelihood source in the rural economy, of which three sources are considered – crop production, livestock production and non-farm enterprises. The diversification within crop production, which we refer to as crop diversification, is measured in two ways. First, we measure crop diversification as the total number of different types of crops cultivated by households, which means that for rural households that are not engaged in crop production, the score for crop diversification will be zero. Second, we used the entropy measure of diversification to create an index of crop diversification, which provides a composite measure of diversification across and within six different groups of crops (i.e. cash crops, cereals, fruits, legumes, roots, and vegetables). The entropy measure of diversification has mainly been applied to measure the distribution of firms’ activities across different products or market segments (see Palepu, Citation1985; Raghunathan, Citation1995; Theil, Citation1967). We follow this approach to generate a diversification index for households’ engagement in different types of crop production. The index is formally derived as follows:
(3)
(3)
Where CDi is the diversification score for household i. Pi is the share of a particular crop group, say legumes, in the total number of crops grown by the household. In other words, the number of different legume crops grown by a household is expressed as a proportion of the total number of crops grown by the household. n is the number of crop groups, and there are six crop groups considered in this study, as mentioned earlier. According to Raghunathan (Citation1995), the numerator is referred to as the entropy value, whereas the denominator provides the maximum entropy value. The values of CD range between zero and one, where zero corresponds to no diversification (that is, the household grows only a single crop from only one of the six groups), and one means that the distribution is even across the six different groups of crops (that is, the number of crops grown within each of the six groups are all equal). By definition, the entropy score exists only for households that grow at least one crop; hence, CD actually measures the extent of crop diversification among households that are into crop production.
For diversification within livestock production, we use the total number of different types of livestock raised by the household as a measure. Additionally, we examine livestock diversification using a measure that explores the extent of diversification among households that reported raising livestock. However, we used principal component analysis (PCA) to generate this measure instead of the entropy method for two reasons: (1) the number of different types of livestock that the households were asked to report on was much smaller (24 different livestock) compared to the 42 different crops; (2) for 10 of these different livestock, the data showed that the number of households raising each of the 10 constituted less than a percentage of all rural households, leaving only 14 for classification into subgroups. As a result, we performed PCA on the remaining 14 different livestock types to generate a score (based on the first principal component) that measures the extent of livestock diversification among households that raise livestock. Each of the 14 livestock types entered our PCA as a binary variable, where one means the household raised a particular livestock, and zero if otherwise.
With regards to diversification involving non-farm activities, we used only a dichotomous measure which takes a value of 1 if the household owns at least a non-farm enterprise and zero if otherwise. We rely only this dichotomous measure because while the survey asked households to report on up to four non-farm enterprises, the data showed that only six percent of the households had two or more non-farm enterprises. Those with no non-farm enterprises and those with only one non-farm enterprise constituted 67% and 27% of the sample, respectively.
provides further details regarding the variable labels and descriptions of livelihood diversification variables. Similarly, provides information on how all the other variables including the control variables are defined as well as with their summary statistics.
Table 1. Descriptive statistics on variables used.
3.2.2. Estimation techniques and strategy for addressing potential endogeneity
provides the details of the specific estimation techniques applied to EquationEquations (1)(1)
(1) and Equation(2)
(2)
(2) . Each estimation technique was selected based on its suitability for the nature of the dependent variable being modeled. For dichotomous variables, logit regression method was applied while Poison regression method was applied to count dependent variables and the Ordinary Least Squares method was applied to models with continuous dependent variables. Thus, the models for access to digital finance and ownership of non-farm enterprises were estimated using logit regressions, whereas those for the count measures of diversification (ie Crop_total and n_livestock) were estimated using Poisson regressions and those for continuous measures of diversification (ie Total_diverse and livestock_div) were estimated using OLS.
Table 2. Estimation strategy.
By definition, EquationEquations (1)(1)
(1) and Equation(2)
(2)
(2) indicate a potential simultaneity problem, which could be a major source of endogeneity bias, between each of the diversification variables and the mobile money variable. Without addressing this problem, EquationEquations (1)
(1)
(1) and Equation(2)
(2)
(2) only help us learn about the association between access to digital finance and our measures of livelihood diversification. We therefore address this potential endogeneity bias using instrumental variable models. Given our interest in the effect of access to digital finance (mobile money) on livelihood diversification, we sought for an instrument for mobile money, and not for livelihood diversification. Following recent studies (such as Aker & Mbiti, Citation2010; Atta-Ankomah, Citation2024; Bukari & Koomson, Citation2020), we considered two candidates as potential instruments for mobile money: the proportion of households in a community/enumeration area that owns mobile phones (labelled in as m_penetration) and the proportion of household members that own mobile phone (labelled in as prop_mm). While these two variables are measures of mobile phone penetration, m_penetration provides a measure at the level of the community/enumeration area level, whereas prop_mm measures mobile phone penetration at the household level. In our preliminary analysis, both variables passed the under-identification test; however, only prop_mm passed the weak identification test. Hence, the results reported in this study used prop_mm as the instrument for mobile money.
Our instrumental variable models were estimated using the limited-information maximum likelihood (LIML) estimator. Our choice of LIML was informed by the argument that the LIML estimator tends to produce less biased estimates, is more robust to weak instruments, and produces confidence intervals with better coverage rates than two-stage least squares (Poi, Citation2006; Stock et al., Citation2002; Stock & Yogo, Citation2005). In addition, the LIML estimator performs better than both two-stage least squares and generalized methods of moments in finite samples but is equivalent to a two-stage least squares method in large samples (Cameron & Trivedi, Citation2010).
4. Results and discussion
This section presents and discusses the findings of the study. We first present brief descriptive results on the relationship between our diversification variables and access to digital finance, after which we present the results of the econometric analysis. Under the econometric results, the correlates of access to digital finance in rural Ghana are first presented and discussed, followed by the correlates of livelihood diversification. The section then discusses the results of the effect of access to digital finance on livelihood diversification in models that account for potential endogeneity.
4.1. Descriptive analysis
The average proportion of rural households owning mobile phones across the various communities/enumeration areas was 49%, while only 17% of the households owned at least one mobile money account (see ). This suggests that there were many households in rural Ghana with mobile phones that did not own or operate a mobile money account as of 2016/2017, the survey year. The chart in the bottom right of shows that the mobile money account ownership rate differs slightly between male-headed and female-headed households, by two percentage points in favor of male-headed households. also shows the distribution of households based on the number of different crops they cultivate (upper left), the number of different livestock (upper right), and the number of non-farm enterprises owned or operated by households (bottom left). About 31% of rural households were not involved in crop production, while a typical crop-growing household tends to grow two to five different crops. Livestock production is less common among households than crop production, with more than half of households (58%) having no livestock. The proportion of households with livestock declines continuously at higher and higher with a higher number of different livestock reared by the household.
Figure 1. Distribution of dependent variables and mobile money (momo).
Source: From authors’ analysis of data.

explores how the mean of the dependent variables varies by ownership of mobile money accounts and by the gender of the household head. also shows the entropy measures of crop diversification for crop farming households, as well as the PCA scores for livestock diversification for households that are in livestock production. We can observe from that female-headed households (both owners and non-owners of mobile money accounts) have higher proportions of ownership of non-farm enterprises than their respective counterparts in male-headed households (second chart in the bottom panel of ). By contrast, for all the remaining diversification variables, we observe higher values for male-headed households than for female-headed households. Thus, similar to the ownership of mobile money accounts, we observe a gendered pattern in terms of livelihood diversification, with male-headed rural households focusing more on agricultural production, while their female-headed counterparts diversify into non-farm enterprises. More interestingly, we can observe generally from that, irrespective of the gender of the household head, having mobile money accounts is associated with higher values for the various diversification measures. However, an exception is the entropy measure of crop diversification among households in crop production, where we observe that having a mobile money account is associated with a lower diversification score among male-headed households and no difference between female-headed households with mobile money accounts and those with no mobile money accounts.
Figure 2. Variation in dependent variables by mobile money and gender of household head.
Note: ‘Av.’ stands for ‘average’. Source: From authors’ analysis of data.

In the next section, we investigate these observed patterns or relationships in multiple regression frameworks, including models that help deal with potential endogeneity issues between the ownership of mobile money and measures of diversification among rural households in Ghana.
4.2. Regression results
4.2.1. Key correlates of access to digital finance
displays correlates of access to digital finance services in rural Ghana. Columns (1) and (2) presents results for the full sample of rural households; (3) and (4) show results for the sub-sample of male-headed households (MHHs), while (5) and (6) contain results for the sub-sample of female-headed households (FHHs). The results show that education is important for access to mobile money services, particularly among households headed by males. The higher the level of education of the head of the household the more likely the household owns mobile money accounts. This may largely due to the fact that educated people tend to have greater knowledge about new technology and appreciate its benefits better than the uneducated. The gender heterogeneity with regards to education observed in the results aligns with those of Amoah et al. (Citation2020), N’dri and Kakinaka (Citation2020), and Myeni et al. (Citation2020). Furthermore, the highly-educated household heads are likely to be those with more stable jobs and better incomes, which (all things being equal) come with higher demand for financial transactions and services that are offered in a relatively more efficient manner such as mobile money services.
Table 3. Regression results on correlates of access to digital finance (dependent variable = momo).
Similarly, we find that owning a non-farm enterprise (NFE) has a strong association with access to digital finance, a result that is consistent among both male- and female-headed households. Households that own and operate an NFE generally tend to have numerous financial transactions to undertake, especially in relation to running the enterprise, and would likely take advantage of the efficiency and convenience of digital financial services. Relatedly, we find that households that spend more on agricultural inputs, irrespective of the gender of the head, are likely to have access to digital finance.
The proportion of household members owning a mobile phone (prop_mm) has a positive and significant association with access to digital finance. Similarly, the higher the mobile phone penetration rate at the community level (m_penetration), the higher is the likelihood of a household owning mobile money account(s), although this association is not as strong as that observed for household-level mobile phone penetration (prop_mm). These findings suggest that, as more people become owners of and familiar with the use of mobile phones within their households or communities, the greater the likelihood that they or some members of their households will have access to digital financial services through mobile money. This can be attributed to network effects and externalities. That is, the adoption and diffusion of mobile money services is greater if people within one’s network use mobile phone technologies. This agrees with the findings of Mothobi and Grzybowski (Citation2017) and Grzybowski (Citation2015) who found a positive relationship between social networks and mobile phone adoption and related services, including mobile money.
The results show that households headed by females are more likely to adopt digital finance than those with male heads, which is consistent with Myeni et al. (Citation2020), Mothobi and Grzybowski (Citation2017), but contrasts those of N’dri and Kakinaka (Citation2020). Other household-level factors found to have a positive correlation with access to digital finance were household size (Asravor et al., Citation2022; Baffour et al., Citation2021) and connection of the household to the national electricity grid. On the other hand, total farm size owned or operated by households was found to have a negative association with access to digital finance. This appears counterintuitive, but may suggest that rural households that depend more on subsistence farming have less access to digital finance, especially given that our model controls for the effect of agricultural input costs and ownership of NFEs. The results also show that the age of the household head is negatively associated with the ownership of mobile money accounts, consistent with Asravor et al.. (2022) and Amoah et al. (Citation2020). However, the squared term of age is positive and significant, suggesting that beyond a certain age threshold, there is a greater likelihood of access to digital finance in the household. This is plausible, as older people may have younger household members, such as grandchildren, in their households. These younger household members, who are youthful and potentially technology savvy, are likely to patronize digital financial services.
4.2.2. Key correlates of livelihood diversification
This sub section presents the results on the key correlates of livelihood diversification in rural Ghana. presents the results for crop diversification in columns (1) and (2), where column (1) considers the total number of different crops cultivated by households, and (2) has the entropy measure of the diversity of crops, which is a more robust measure of the extent of crop diversification by households. Columns (3) and (4) represent livestock diversification. Column (3) reports the results of the model with the total number of different animals the household raises as the dependent variable, while the results in Column (4) are for the model in which the PCA score for ownership of different livestock is used as the dependent variable. The results in Column (4) restrict the sample to households that raised at least one type of livestock. Column (5) examines the correlates of owning a non-farm enterprise (NFE).
Table 4. Regression results on correlates of livelihood diversification in rural Ghana.
The results show that having a mobile money account has a strong positive association with NFE ownership (see Column 5), as found in the previous section, but not with crop and livestock diversification (see Columns 1 – 4). With regards to the education of the household head, the results show that relative to household heads with no formal education, those with educational levels of primary and junior high/middle school are more likely to own or operate NFEs. This finding is consistent with Kassegn and Abdinasir (Citation2023). However, having secondary/vocational and higher levels of education show no significant relationship with NFE ownership and livestock diversification and such households are also less likely to diversify their crop production. This is potentially the case because people with higher levels of education may have better job opportunities in the paid (formal) employment sector and may be less likely to engage in self-employment in the non-farm sector (which mainly consists of informal micro and small enterprises). Thus, some level of education is needed to diversify into NFEs rather than no education at all, but very highly educated people are likely not to engage in NFE activities, which are largely informal.
Households that experienced a shock, specifically injury or illness of a household member, in the year immediately preceding the survey were associated with higher livelihood diversification. These households diversify in crop production, animal production, and NFE ownership. The lessons from such a shock, in terms of the likely negative effect on welfare, may have spurred such households to have a more diversified livelihood portfolio. This confirms the findings of Nguyen et al. (Citation2022), who found that households in Thailand and Vietnam use diversification as a coping strategy against shocks and that such diversification helps households maintain per capita consumption in the face of shocks.
The results show that households with larger sizes tend to diversify their livelihood portfolios to include livestock production and the operation of NFEs compared to smaller-sized households. This finding corroborates those of Dirribsa and Tassew (Citation2015) but contradicts that of Gebru et al. (Citation2018), who found that household size negatively impacts livelihood diversification in the Eastern Tigray region of Ethiopia. Larger households have a relatively larger financial burden. This is especially the case if the dependency ratio in the household is high or if only a few household members are economically active. Thus, such households tend to diversify to increase their income base. Furthermore, these households, by virtue of their large size, have enough personnel to handle the various activities they diversify into. Similarly, households whose heads are married or in consensual unions diversify their livestock production and engage in NFE activities. We also find that ecological zones are important drivers of livelihood diversification. Relative to coastal households, households in forest and savannah zones diversify into crop production. This is expected because farming is practiced more in the forest and savannah zones of Ghana, compared to coastal areas where households tend to engage more in fishing, largely because of their proximity to the sea. Households with larger farm sizes have positive and significant relationship with crop and animal diversification but negative relationship with ownership of NFEs. Similarly, high expenditures on agricultural inputs are positively associated with diversification in crop and livestock production.
The results show, however, that having a female as the household head has a negative association with livestock diversification but a positive association with owning NFEs, which agrees with the findings of Loison (Citation2019) and Lay et al. (Citation2008), but contrasts those of Ahmed et al. (Citation2018). Ahmed et al. (Citation2018) argued that a greater degree of diversification for males in rural Bangladesh stems from the fact that males have better work opportunities outside the home than their female counterparts. The age of the household head is positively associated with crop diversification and ownership of NFEs, whereas age squared is negatively correlated. This suggests that households generally diversify crop production and livelihoods as the head gets older, but beyond a certain threshold of age, these households tend to reduce diversification. Households with higher adult equivalent consumption expenditures tend to have a negative association with crop diversification but a positive association with owning NFEs and the extent of livestock diversification.
4.2.3. The effect of digital finance on livelihood diversification – addressing potential endogeneity problem
A key objective of this study is to investigate the effect of access to digital finance on livelihood diversification among rural households in Ghana. However, access to digital finance, proxied by the ownership of a mobile money account, is potentially endogenous, thus rendering the analysis from the previous section insufficient to conclude on the causal effect of digital finance on livelihood diversification. For example, it is possible that households that have access to digital finance also have some unobservables that may be related to livelihood diversification measures, thus confounding the true effect of digital finance on livelihood diversification. In this section, we address the potential endogeneity problem by employing an instrumental variable approach.
Footnote3 displays the results from the instrumental variable estimation, in which the number of household members owning mobile phones as a proportion of the total number of household members is used as an instrument for mobile money account ownership. It is observed from that the endogeneity test produces a p value of less than .01 for the two crop diversification measures (Columns 1 and 2), as well as livestock diversification measured using the total number of animals (Column 3), leading to a rejection of the null hypothesis of exogeneity in favor of the alternative hypothesis of endogeneity. Thus, we conclude that access to digital finance is endogenous in models (1), (2), and (3). This also implies that instrumental variable models are more appropriate for determining the effect of digital finance on crop diversification and livestock diversification. In the case of the model for ownership of an NFE and the extent of livestock diversification (using the PCA score), we do not reject the null hypothesis of exogeneity. The exogeneity of digital finance in non-farm enterprises and the intensity of livestock diversification models imply that the results in Columns (4) and (5) of hold. The tests for under-identification, with the null hypothesis that the model is under-identified, yield a p value of less than .01 for all the models, and hence, we reject the null hypothesis and conclude that the models are not under-identified. In other words, there is a high correlation between the instrument and endogenous variable, confirming that our instrument is relevant. Similarly, the weak identification test, with the null hypothesis that the model is weakly identified, is rejected for all the models because the F-statistic for each of the models is far greater than 10, the conventional threshold for determining weak identification.
Table 5. IV_LIML regression results on effect of mobile money on livelihood diversification.
The IV LIML results in show that access to digital finance tends to increase the number of different types of livestock reared. The results in Column 4 indicates that the effect disappears with respect to the extent of livestock diversification measured using PCA scores, which restricts the sample to households that own at least one type of livestock. This means that the significant effect in the model for the number of different livestock (see Column 3 of ) may be largely driven by the variation between households with no livestock and those with any number of livestock. In contrast to the results on diversification toward livestock, we find that access to digital finance reduces diversification between crops and within crop groups. In other words, households with access to digital finance are less likely to have diversified crop production. Thus, access to digital financial services is associated with diversification into non-crop production avenues and this may be driven by allocative efficiency that may be linked to access to digital financial services, including access to credit, spreading risk, or both. In particular, it is reasonable to believe that rural agricultural households specialize in the production of particular crops if, through access to digital finance, they are able to engage in NFEs that generate more income than when they grow only crops. Indeed, evidence suggests that financial inclusion, especially access to agricultural credit, leads farmers to intensify the cultivation of specific crops and lower diversification within crop production (Gebreselassie, Citation2006; Loison, Citation2019; Xie et al., Citation2019).
4.2.4. Digital finance and NFEs - sub-sample results for only rural agricultural households
To reinforce our findings on the effect of digital finance on diversification through NFEs, we restrict the sample to rural agricultural households only and re-examine this relationship. The results are presented in .Footnote4 These results serve as further robustness check. Column (1) is for households that are in at least one type of agricultural activity (ie crop production, or livestock production), while column (2) is for households that are in both types of agricultural activities. The findings show that having access to digital finance has a positive and significant effect on diversifying livelihood sources among rural agricultural households (that is, when non-agricultural households are excluded from the analysis), and the effect is stronger for households that engage in both types of agricultural activities. It is possible that those households that are involved in both crop and livestock production have garnered the experience of diversifying even though this diversification was limited to the agricultural sector. Therefore, they find it easier to diversify into NFEs when they have access to digital finance and opportunities for inclusion in the financial system. These results show that our overall finding of the strong effect of digital finance in promoting livelihood diversification among rural households is consistent for all rural households and rural agricultural households.
Table 6. Logit regression results on effect of digital finance on ownership of NFEs (sub-sample results for only rural agricultural households).
4.2.5. Gender heterogeneity in the effect of digital finance on livelihood diversification
summarizes the heterogeneity in the effect of digital finance on livelihood diversification by the gender of the household head.Footnote5 Where the endogeneity test yields an endogenous result for access to digital finance, the IV results are interpreted, whereas the OLS/logit/Poison results are interpreted for cases where the endogeneity test showed ‘not endogenous’ results. The results show a significant effect on ownership of NFE for both male- and female-headed households, although the effect appears stronger among female-headed households, which are consistent with the findings of Loison (Citation2019) and Lay et al. (Citation2008).
Table 7. Heterogeneous effects of momo on livelihood diversification by gender of household head.
While the results demonstrate no significant effect of digital finance on crop diversification among female-headed households, we observe a negative relationship among male-headed households, implying that the negative effect of digital finance on crop diversification observed in the previous section is mainly driven by male-headed households. Regarding livestock production, the results show significant diversification in the number of different livestock raised among male-headed households while digital finance has no significant effect on livestock diversification among households headed by females. An implication of these findings is that increased access to digital finance among females in rural Ghana will lead to greater degree of livelihood diversification away from agriculture. Previous research has shown that FHHs in rural areas tend to be more vulnerable in terms of access to resources than other households (Adjei-Mantey et al., Citation2022). Thus, support to such households through improved access to mobile money and other digital financial services can help expand opportunities for economic participation by females, and lead to increased female economic empowerment and improvement in living standards. This is in line with a recent study by M. S. Islam et al. (Citation2022) that found that participation in NFEs has significant and positive effects on rural women’s income. NFE as an important avenue for women’s economic participation is further underpinned by existing structural challenges (such as the patriarchal nature of land ownership) in Ghana that prevent women from earning maximum returns from farm work.
5. Conclusion
The emergence of digital financial systems, especially mobile money, has significantly changed the financial services space in SSA in ways that provide opportunities to promote financial inclusion among the poor, especially those in rural areas. Focusing on mobile money, which is the main avenue for digital financial services for rural households in Ghana, this study investigates whether digital finance influences rural households’ livelihood diversification, using nationally representative data from Ghana. This study also delves into the effect on different forms of livelihood diversification among rural households. Using several econometric methods, including instrumental variable techniques, the study found mobile money to be positively associated with households’ choice of non-farm enterprises as a livelihood option, as well as engagement in livestock production. However, we find a negative relationship between mobile money and diversification in crop production, as well as the extent of crop diversification by households within the crop sector. In other words, when agricultural households have access to mobile money services, they tend to produce fewer number of different crops while exploring non-farm activities and livestock production as additional livelihood options. Generally, the results show that when rural households become more included in the financial system through mobile money, they tend to diversify into less traditional sources of livelihood. This effect pertains to both male-headed and female-headed households, except in the case of diversification in crop production, where we found the negative effect to be mainly driven by male-headed households. In addition to mobile money, other important correlates of diversification worth highlighting are the education of the household head, the experience of a health shock by the household, expenditure on agricultural inputs, and the geographic location of the household. Education was also found to be an important correlate of access to digital finance in addition to other factors such as agricultural input cost, access to the national electricity grid, and the proportion of household/community members owning a mobile phone.
These findings have several important policy implications. The diversification away from crop production and the diversification into non-farm business activities and livestock production suggests that rural households may be reallocating productive resources into areas that yield higher returns to their livelihoods and/or mitigate the risk of income losses, and that access to digital finance facilitates this process. While this shift may enhance rural livelihoods, it may also free land resources for more commercialized agricultural production beyond subsistence agriculture practiced by many rural households in developing countries such as Ghana. This means that the Government of Ghana should implement policies to support the adoption and use of digital financial services by rural households as part of broader strategies to revolutionize agriculture and the rural economy rather imposing an E-levy on mobile money transactions. Such supportive policies could also lead to increases in women’s economic participation and empowerment by facilitating their engagement in NFE activities. The results also highlight the need to address constraints that limit rural households’ access to digital financial tools, especially mobile money, for which ‘non-formal’ education of the less educated on how to use these digital tools is crucial. To do this, we recommend a collaborative effort between government and major telcos operating mobile money services in Ghana. In addition, government should enhance investment in the provision of complementary infrastructure required for broadening access to mobile money services in rural communities, such as making electricity more accessible as well as incentivizing telcos to make mobile phones and networks more accessible in rural communities.
While the data used in this study came from the latest GLSS (a nationally representative household survey in Ghana), it is important to acknowledge that the data was collected in 2016/2017, hence, may not capture recent developments within the mobile money space. Therefore, while this study offers significant insights into the socioeconomic impact of mobile money in the rural economy, it may be worthwhile to replicate the study when a new nationally representative data becomes available in order to learn about the impact of recent developments in the mobile money space. Also, the measures of diversification used in this study largely focused on diversification within broad livelihood activities areas and not across these areas, which is an area further studies can explore. Additionally, further studies may directly explore impact of mobile money on income diversification, which can encapsulate other sources of income such as remittances and assets.
Author contributions
Richmond Atta-Ankomah: Conception, methodology, data management and analysis, writing draft and discussion; revising and editing the intellectual
Kwame Adjei-Mantey: Literature review, writing draft and discussion, revising and editing the intellectual contents
Akuffo Amankwah: Literature review, writing draft and discussion, revising and editing the intellectual contents
Supplemental Material
Download MS Word (94.8 KB)Data availability statement
The main data underlying this study are available upon request from the Ghana Statistical Service (https://microdata.statsghana.gov.gh/index.php/catalog/97/study-description).
Disclosure statement
No potential conflict of interest was reported by the author(s).
Additional information
Funding
Notes on contributors
Richmond Atta-Ankomah
Richmond Atta-Ankomah is a development economist and Senior Research Fellow at the Institute of Statistical, Social and Economic Research, University of Ghana. His research interest is diverse but largely converges around development issues Concerning households and firms in sub Saharan Africa.
Kwame Adjei-Mantey
Kwame Adjei-Mantey is researcher at the Department of Applied Economics, University of Environment and Sustainable Development, Somanya, Ghana. His research interests are in the areas of energy and environmental economics, development economics and behavioral economics.
Akuffo Amankwah
Akuffo Amankwah is an Economist for the Living Standards Measurement Study (LSMS), the World Bank’s flagship household survey program housed at the Development Data Group. His primary areas of research are poverty, labor, aquaculture, agriculture and rural development, and methodological studies to improve household surveys.
Notes
1 The authors obtained an anonymized version of the data from GSS and did not directly engage any of the participants of the survey. Hence, the research activities conducted by the authors of this study are very less likely to lead to a violation of any research ethics.
2 This study uses the ten regions that existed at the time of the GLSS 7 survey. The regional boundaries have since been re-demarcated to create additional six regions in the country.
3 The full regression results are displayed in Table A2 in the Online Appendix.
4 The full results are presented in Table A3 in the online appendix.
References
- Adjei-Mantey, K., Kwakwa, P. A., & Adusah-Poku, F. (2022). Unraveling the effect of gender dimensions and wood fuel usage on household food security: Evidence from Ghana. Heliyon, 8(11), e11268. https://doi.org/10.1016/j.heliyon.2022.e11268
- Ahmad, H. A., Green, C., & Jiang, F. (2020). Mobile money, financial inclusion and development: A review with reference to African experience. Journal of Economic Surveys, 34(4), 753–792. https://doi.org/10.1111/joes.12372
- Ahmed, M. T., Bhandari, H., Gordoncillo, P. U., Quicoy, C. B., & Carnaje, G. P. (2018). Factors affecting extent of rural livelihood diversification in selected areas of Bangladesh. SAARC Journal of Agriculture, 16(1), 7–21. https://doi.org/10.3329/sja.v16i1.37419
- Akaakohol, M. A., & Aye, G. C. (2014). Diversification and farm household welfare in Makurdi, Benue State, Nigeria. Development Studies Research. An Open Access Journal, 1(1), 168–175. https://doi.org/10.1080/21665095.2014.919232
- Aker, J. C., & Mbiti, I. M. (2010). Mobile phones and economic development in Africa. Journal of Economic Perspectives, 24(3), 207–232. https://doi.org/10.1257/jep.24.3.207
- Amoah, A., Korle, K., & Asiama, R. K. (2020). Mobile money as a financial inclusion instrument: What are the determinants? International Journal of Social Economics, 47(10), 1283–1297. https://doi.org/10.1108/IJSE-05-2020-0271
- Amoah, A., Kwablah, E., Amoah, B., & Adjei-Mantey, K. (2023). Willingness to pay for electronic transaction levy: Empirical evidence from Ghana. African Journal of Economic and Management Studies, 14(4), 663–679. https://doi.org/10.1108/AJEMS-09-2022-0359
- Aron, J. (2018). Mobile money and the economy: A review of the evidence. The World Bank Research Observer, 33(2), 135–188. https://doi.org/10.1093/wbro/lky001
- Asfaw, S., Scognamillo, A., Di Caprera, G., Sitko, N., & Ignaciuk, A. (2019). Heterogeneous impact of livelihood diversification on household welfare: Cross-country evidence from Sub-Saharan Africa. World Development, 117, 278–295. https://doi.org/10.1016/j.worlddev.2019.01.017
- Asli, D., Klapper, L., Singer, D., & Ansar, S. (2022). The Global Findex Database 2021: Financial inclusion, digital payments, and resilience in the age of COVID-19. World Bank. https://doi.org/10.1596/978-1-4648-1897-4
- Asmah, E. E. (2011). Rural livelihood diversification and agricultural household welfare in Ghana. Journal of Development and Agricultural Economics, 3(7), 325–334.
- Asravor, R. K., Boakye, A. N., & Essuman, J. (2022). Adoption and intensity of use of mobile money among smallholder farmers in rural Ghana. Information Development, 38(2), 204–217. https://doi.org/10.1177/0266666921999089
- Atta-Ankomah, R. (2022). Credit for households in Ghana: Has mobile money (momo) improved inclusive access? Scientific African, 16, e01230. https://doi.org/10.1016/j.sciaf.2022.e01230
- Atta-Ankomah, R. (2024). Mobile money and intra-household employment diversification in Ghana. African Journal of Science, Technology, Innovation and Development, 1–17. https://doi.org/10.1080/20421338.2024.2317657
- Atta-Ankomah, R., Asante-Addo, C., Okyere, C. Y., & Asante-Poku, N. A. (2024). Does digital financial technology improve access to finance among households experiencing idiosyncratic health shocks in Ghana? Unpublished Manuscript, Last modified, January 9, 2024.
- Atta-Ankomah, R., & Okyere, Y. C. (2022). Welfare effects of financial inclusion services in Ghana: A comparative analysis of mobile money and other financial services. Global Social Welfare, 10(1), 83–92. https://doi.org/10.1007/s40609-022-00234-x
- Baffour, P. T., Rahaman, W. A., & Mohammed, I. (2021). Impact of mobile money access on interna remittances, consumption expenditure and household welfare in Ghana. Journal of Economic and Administrative Sciences, 37(3), 337–354. https://doi.org/10.1108/JEAS-04-2020-0045
- Bara, A. (2013). Mobile money for financial inclusion: Policy and regulatory perspective in Zimbabwe. African Journal of Science, Technology, Innovation and Development, 5(5), 345–354. https://doi.org/10.1080/20421338.2013.829287
- Batool, S., & Jamil, F. (2019). Rural employment and income diversification in Pakistan. Pakistan Journal of Agricultural Sciences, 56(02), 503–510. https://doi.org/10.21162/PAKJAS/19.7484
- Bongomin, O. C. G., Ntayi, M. J., Munene, C. J., & Malinga, A. C. (2018). Mobile money and financial inclusion in Sub-Saharan Africa: The moderating role of social networks. Journal of African Business, 19(3), 361–384. https://doi.org/10.1080/15228916.2017.1416214
- Bukari, C., & Koomson, I. (2020). Adoption of mobile money for healthcare utilization and spending in rural Ghana. In S. A. Churchill (Ed.), Moving from the millennium to the sustainable development goals (pp. 37–60). Palgrave Macmillan.
- Cameron, A. C., & Trivedi, P. K. (2010). Microeconometrics using Stata (rev. ed.). Stata Press.
- Dabalen, A., & Mensah, J. T. (2023). Ten facts about digital technology adoption in Ghana. Africa Can End Poverty.
- Dagunga, G., Ayamga, M., & Danso-Abbeam, G. (2020). To what extent should farm households diversify? Implications on multidimensional poverty in Ghana. World Development Perspectives, 20, 100264. https://doi.org/10.1016/j.wdp.2020.100264
- Dagunga, G., Azumah, S. B., Zakaria, A., Boateng, A. N., Mensah, B. K., Boateng, S. E., Tsekpo, M. E., & Kankam, P. (2022). Savings and economic diversification among youth in Ghana: Implications for policy and practice. Cogent Economics & Finance, 10(1), 2123887. https://doi.org/10.1080/23322039.2022.2123887
- Davis, B., Di Giuseppe, S., & Zezza, A. (2017). Are African households (not) leaving agriculture? Patterns of households’ income sources in rural Sub-Saharan Africa. Food Policy, 67, 153–174. https://doi.org/10.1016/j.foodpol.2016.09.018
- Dirribsa, S., & Tassew, B. (2015). Analysis if livelihood diversification strategy of rural households: A case study of Ambo District, Oomiya Regional State, Ethiopia. International Journal of Current Research and Academic Review, 3(8), 406–426.
- Finger, R. (2023). Digital innovations for sustainable and resilient agricultural systems. European Review of Agricultural Economics, 50(4), 1277–1309. https://doi.org/10.1093/erae/jbad021
- Gebreselassie, S. (2006). Intensification of smallholder agriculture in Ethiopia: Options and scenarios (Future Agricultures Consortium Discussion Paper 007). Future Agricultures.
- Gebru, G. W., Ichoku, H. E., & Phil-Eze, P. O. (2018). Determinants of livelihood diversification strategies in Eastern Tigray Region of Ethiopia. Agriculture & Food Security, 7(1), 62. https://doi.org/10.1186/s40066-018-0214-0
- Grzybowski, L. (2015). The role of network effects and consumer heterogeneity in the adoption of mobile phones: Evidence from South Africa. Telecommunications Policy, 39(11), 933–943. https://doi.org/10.1016/j.telpol.2015.08.010
- Islam, A., Muzi, S., & Meza, J. L. R. (2018). Does mobile money use increase firms’ investment? Evidence from enterprise surveys in Kenya, Uganda, and Tanzania. Small Business Economics, 51(3), 687–708. https://doi.org/10.1007/s11187-017-9951-x
- Islam, M. S., Islam, S., Fatema, K., & Khanum, R. (2022). Rural women participation in farm and off-farm activities and household income in Bangladesh. Heliyon, 8(9), e10618. https://doi.org/10.1016/j.heliyon.2022.e10618
- Jack, W., & Suri, T. (2014). Risk sharing and transactions costs: Evidence from Kenya’s mobile money revolution. American Economic Review, 104(1), 183–223. https://doi.org/10.1257/aer.104.1.183
- Kassegn, A., & Abdinasir, U. (2023). Determinants of rural households’ livelihood diversification strategies: In the case of north Wollo zone, Amhara National Regional State, Ethiopia. Cogent Economics and Finance, 11(1), 2185347. https://doi.org/10.1080/23322039.2023.2185347
- Khatun, D., & Roy, B. C. (2012). Rural livelihood diversification in West Bengal: Determinants and constraints. Agricultural Economics Research Review, 25(1), 115–124.
- Lay, J., Mahmoud, T. O., & M’Mukaria, G. M. (2008). Few opportunities, much desperation: The dichotomy of non-agricultural activities and inequality in western Kenya. World Development, 36(12), 2713–2732. https://doi.org/10.1016/j.worlddev.2007.12.003
- Lee, J. N., Morduch, J., Ravindran, S., Shonchoy, A., & Zaman, H. (2021). Poverty and migration in the digital age: Experimental evidence on mobile banking in Bangladesh. American Economic Journal: Applied Economics, 13(1), 38–71. https://doi.org/10.1257/app.20190067
- Loison, S. A. (2015). Rural livelihood diversification in sub-Saharan Africa: A literature review. The Journal of Development Studies, 51(9), 1125–1138.
- Loison, S. A. (2019). Household livelihood diversification and gender: Panel evidence from rural Kenya. Journal of Rural Studies, 69, 156–172.
- Mahama, A. T., & Maharjan, K. L. (2017). Determinants of livelihood diversification in Ghana from the national livelihood strategies and spatial perspective. Journal of International Development and Cooperation, 23(1), 75–90.
- Mahama, A. T., & Nkegbe, K. P. (2021). Impact of household livelihood diversification on welfare in Ghana. Scientific African, 13, e00858. https://doi.org/10.1016/j.sciaf.2021.e00858
- Mattern, M. (2018). How Ghana became one of Africa’s top mobile money markets. CGAP (Consultative Group to Assist the Poor) Blogpost. What You Need to Know.
- Martin, S. M., & Lorenzen, K. (2016). Livelihood diversification in rural Laos. World Development, 83, 231–243. https://doi.org/10.1016/j.worlddev.2016.01.018
- Miller, H. (2019). What works for digital financial services in agricultural development? USAID Feed the Future Initiative.
- Ministry of Finance. (n.d). Toward a cash-lite Ghana – Building an inclusive digital payments ecosystem: Digital payments roadmap. https://mofep.gov.gh/sites/default/files/acts/Ghana_Cashlite_Roadmap.pdf
- Mohammed, K., Batung, E., Kansanga, M., Nyantakyi-Frimpong, H., & Luginaah, I. (2021). Livelihood diversification strategies and resilience to climate change in semi-arid northern Ghana. Climatic Change, 164(3-4), 1–23. https://doi.org/10.1007/s10584-021-03034-y
- Mothobi, O., & Grzybowski, L. (2017). Infrastructure deficiencies and adoption of mobile money in Sub-Saharan Africa. Information Economics and Policy, 40, 71–79. https://doi.org/10.1016/j.infoecopol.2017.05.003
- Myeni, S., Makate, M., & Mahonye, N. (2020). Does mobile money promote financial inclusion in Eswatini? International Journal of Social Economics, 47(6), 693–709. https://doi.org/10.1108/IJSE-05-2019-0310
- N’dri, L. M., & Kakinaka, M. (2020). Financial inclusion, mobile money, and individual welfare: The case of Burkina Faso. Telecommunications Policy, 44(3), 101926. https://doi.org/10.1016/j.telpol.2020.101926
- Nguyen, D. L., Nguyen, T. T., & Grote, U. (2022). Shocks, household consumption, and livelihood diversification: A comparative evidence from panel data in rural Thailand and Vietnam. Economic Change and Restructuring, 56(5), 3223–3255. https://doi.org/10.1007/s10644-022-09400-9
- Okyere, C. Y., Atta-Ankomah, R., & Asante-Addo, C. (2024a). Does Digital Financial Inclusion Improve Food Security and Household Resilience? Evidence from Northern Ghana. Unpublished Manuscript, Last Modified, January 2, 2024.
- Okyere, C. Y., Atta-Ankomah, R., & Asante-Addo, C. (2024b). Mobile money adoption, farm performance and household welfare in Northern Ghana. Unpublished Manuscript, Last modified, January 10, 2024.
- Ouma, S. A., Odongo, T. M., & Were, M. (2017). Mobile financial services and financial inclusion: Is it a boon for savings mobilization? Review of Development Finance, 7(1), 29–35. https://doi.org/10.1016/j.rdf.2017.01.001
- Oxford Business Group. (2018). Ghana rolls out mobile money interoperability platform. https://oxfordbusinessgroup.com/articles-interviews/ghana-rolls-out-mobile-money-interoperability-platform.
- Palepu, K. (1985). Diversification strategy, profit performance and the entropy measure. Strategic Management Journal, 6(3), 239–255. 10.1002/smj.4250060305
- Peprah, J. A., Oteng, C., & Sebu, J. (2020). Mobile money, output and welfare among smallholder farmers in Ghana. SAGE Open, 10(2), 215824402093111. https://doi.org/10.1177/2158244020931114
- Poi, B. P. (2006). Jackknife instrumental variables estimation in Stata. The Stata Journal: Promoting Communications on Statistics and Stata, 6(3), 364–376. https://doi.org/10.1177/1536867X0600600305
- Raghunathan, S. P. (1995). A refinement of the entropy measure of firm diversification: Toward definitional and computational accuracy. Journal of Management, 21(5), 989–1002. https://doi.org/10.1177/014920639502100510
- Stock, J. H., Wright, J. H., & Yogo, M. (2002). A survey of weak instruments and weak identification in generalized method of moments. Journal of Business and Economic Statistics, 20(4), 518–529. https://doi.org/10.1198/073500102288618658
- Stock, J. H., & Yogo, M. (2005). Testing for weak instruments in linear IV regression. In D. W. K. Andrews (Ed.), Identification and inference for econometric models (pp. 80–108). Cambridge University Press.
- Theil, H. (1967). Economics and Information Theory. North-Holland.
- World Bank Microdata Catalog. (2023). Mobile cellular subscriptions (per 100 people) – Ghana. https://data.worldbank.org/indicator/IT.CEL.SETS.P2?locations=GH
- Xie, H., Huang, Y., Chen, Q., Zhang, Y., and Wu Q. (2019). Prospects for agricultural sustainable intensification: A review of research. Land 8(11), 157. https://doi.org/10.3390/land8110157