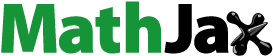
Abstract
The paper investigates aggregation bias by comparing the risk and returns characteristics of stock exchange-traded shares and Treasury bills (T’bills) in Ghana. The study uses end of period annualized data on T’bills and stocks returns, and inflation from 1990 to 2020. We mainly consider four separate investment periods: 1990–2000, 2001–2010, 2011–2020, and 1990–2020 (i.e. the aggregated period) in order to determine possible aggregation bias occurring from lumping the years together. We measure average annual returns, standard deviations, co-efficient of variations, Sharpe ratio, ANOVA, Jarque-Bera test, maximum drawdowns (MDD), and correlation analysis to determine risk and return characteristics of the two instruments. The study finds that T'bills compared to stocks shows higher returns yet lower risk, thereby indicating an inverted yield curve. Levene’s Test for Equality of Variances indicates stocks significantly outperformed T’bills over the 31-year aggregated period. The study reveals the presence of aggregation bias as stock and T’bill risk and return characteristics of two segregated periods (i.e. 1990–2000 and 2011–2020) contradict the general expectation of risk-return trade-off theory contrary to that of the aggregated period. The MDD, ANOVA results, Anova F-test and Welch F-test reveal aggregation bias for T’bills but not for stocks. We recommend future studies to ensure that analysis and conclusions made do not suffer aggregation bias by disaggregating aggregated units.
Impact statement
The problem of aggregation bias is one of the neglected issues in most research in finance and economics. There is perceived reliability and generalizations provided by studies with larger sample sizes without recourse to changes in political and macroeconomic conditions that potentially impact investment performance. Hence, investment decisions and policies could be affected by misleading conclusions drawn from aggregated data. By segregating the aggregated periods into three separate decades, we show that risk and return characteristics (as measured by risk-return trade-off theory, co-efficient of variation, Sharpe ratio, maximum drawdowns, and likelihood of loss) of stocks and treasury bills differ among the segregated periods suggesting the presence of aggregation bias. The study potentially enhances the effectiveness of investment and policy decisions among investors. As investor value increases through prudent investment decisions, it would attract more investors, thereby improving the efficiency of the capital market and ensuring efficient allocation of (economic) resources through increased market participation and enhanced liquidity leading to economic activities and GDP growth.
REVIEWING EDITOR:
Introduction
The financial market of Ghana is still at its developmental state even though there has been significant improvement over the past years. The risk and return characteristics of a capital market determines the volume of transactions, instruments traded and the participants. There is ample evidence to believe that risk and returns in the stocks and money markets co-vary (Gusni et al., Citation2018; Markowitz, Citation1952; Panigrahi et al., Citation2020; Qureshi et al., Citation2019) and this can alter the inventory risk borne by both markets (Bellelah et al., Citation2017). Changes in the macroeconomic environment over a period of time causes a change in risk and return characteristics of the various classes of assets. For this reason, analysis and conclusion made on investment returns using aggregated periods may result in aggregation bias (Bessembinder, Citation2018; Di Maggio et al., Citation2020; Kolapo et al., Citation2018). We argue that previous empirical studies (such as Aboagye et al., Citation2008; Antwi et al., Citation2012; Bessembinder, Citation2018; Di Maggio et al., Citation2020) that use aggregated data might have suffered aggregation bias. This may consequently give misleading information to investors and policy makers in their investment and policy decisions.
The objective of this study is to investigate aggregation bias by comparing the risk and return characteristics of stocks and treasury bills (T’bills forthwith) in Ghana, a country that has gone through various macroeconomic and socio-political transitions (Gyimah et al., Citation2021) over the past three decades. We use annual returns of stocks and treasury bills covering a period of 31 years to assess and compare the risk and return characteristics of stocks and treasury bills. For the purpose of ascertaining whether or not there is aggregation bias, we segregate the 31-year period into three separate periods based on defined timelines. We assess the extent to which the risk and return characteristics of each of the disaggregated periods support the risk and return trade-off theory of investments. Our analysis explores the heterogeneities in risk and return characteristics of stocks and T’bills using the risk-return trade-off theory to shape policy, monetary and investment decisions. Our study provides evidence of aggregation bias in risk and return characteristics of stocks and treasury bills in Ghana. In real terms, T’bill have outperformed stocks except during the 2001–2010 segregated period, and in most cases risk-adjusted return (i.e. covariance) was low for T’bill compared with stocks. When risk alone is analysed, robustness check revealed no aggregation bias with stocks as no significant difference was observed among the mean returns of the segregated periods. T’bill however suffered aggregation bias. Real maximum drawdowns for stocks were high and almost the same (i.e. 71.09%, and 68.48%, 69.68%, 67.35%) among the aggregated and segregated periods. In nominal terms, no maximum drawdown was observed for T’bill.
This paper differs from Antwi et al. (Citation2012) and make significant contributions to existing literature on investment performance in Ghana. Firstly, this study provides evidence that the risk and return characteristics of financial instruments differs when the same data is disaggregated. Investment returns respond to economic conditions (Bessembinder, Citation2018; Di Maggio et al., Citation2020; Kolapo et al., Citation2018). Using aggregated data from 1990–2010, Antwi et al. (Citation2012) in their study compared risk and returns characteristics of T’bill and stocks in Ghana to find out which of the two instruments gives better rewards to investors. Their result confirms the risk and returns characteristics associated with investment-the higher the risk, the higher the returns. By disaggregating the 31-year investment holding period (i.e. 1990–2020), we prove that two out of the three of the segregated periods (i.e. 10-year investment holding period each) violate the risk-return relationship which is an evidence of aggregation bias. This result is new to existing literature. Secondly, this paper made use of data spanning a period of 31 years covering All Share Index and the Composite Index. This contrast from 21-year period (1990–2010) and All Share Index employed by Antwi et al. (Citation2012). All things being equal, the use of larger sample size should provide better appreciation of the risk and return characteristics of stocks and T’bill in Ghana. Thirdly, the novelty of this study is seen from the perspective of continuous growth of the GSE. Although the GSE is yet to attain market efficiency (Frimpong & Oteng-Abayie, Citation2006), there have been significant improvement in key indicators such as market capitalization, trading volume, liquidity, number of listed stocks, regulation etc. over the past decades (Ghana Stock Exchange, Citation2023; Gyimah et al., Citation2021). Hence, the use of data covering 1990–2020 in contrast with 1990–2010 data employed by Antwi et al. (Citation2012) ought to offer novel insight into the risk and return characteristics of stocks and T’bill in Ghana. Fourthly, previous studies by Antwi et al. (Citation2012) relied only on average returns, standard deviation, and coefficient of variation in determining the risk and returns characteristics of the two instruments. The present study adds to the existing literature by extending the parameters used in assessing the risk and return characteristics of stocks and T’bill to include Sharpe ratio, ANOVA, kurtosis, skewness, Jacque Bera test, maximum drawdown and likelihood of loss to further test aggregation bias and offer better insight and comprehensive perspective on the risk and returns characteristics of stocks and T’bills in Ghana. More so, the saliency of information on investment performance significantly influenced investor’s appreciation and investment decision (Riley & Yan, Citation2022; Barber et al., 2005). Considering the crucial effect of inflation on the cumulative real value of investment in developing economies, the cumulative real value of investment at the of end each of the four investment periods and the computed real maximum drawdowns provide salient information that should aid investors in their investment decisions without the need for sophisticated investment performance analytical tools or analyst. Finally, contrary to ‘conventional wisdom that active management does not necessarily create value for investors’ (Cremers et al., Citation2019), our result suggests that, holding all other variables equal, stocks and T’bill returns is dynamic. This requires that investor and managers of investment need to be active in order to maximise investment returns.
The remaining sections are organised as follows: literature review, methodology, results, and conclusion of the study.
Literature review
Hypothesis relating to aggregation bias states that individual units from which the aggregated data is drawn may be units with heterogeneous characters (Abhishek et al., Citation2015; Bessembinder, Citation2020). Computing the financial or economic relations suppresses these heterogenous behaviours (differences hidden in the disturbances within the model constructed from the aggregation of the data) resulting in bias estimates (Nasser et al., Citation2018). In the case of stock market and T’bills returns, significant differences in risk and return characteristics of the instruments provide evidence of aggregation bias (Beshears et al., Citation2017; Kolapo et al., Citation2018; Qureshi et al., Citation2019).
Di Maggio et al. (Citation2020), Bessembinder (Citation2018), Antwi et al. (Citation2012), and Aboagye et al. (Citation2008), compare the risk and return characteristics of stocks and money market instruments such as T’bills to determine which of the instruments reward investors better. The authors lump the years together without recourse to changes in political and macroeconomic conditions that may have impacted investment performance over the years (Nasser et al., Citation2018; Ndlovu et al., Citation2018) and consequently expose the findings to possible aggregation bias (Hilliard & Zhang, Citation2015; Liu et al., Citation2019), with the assumption that what is true for the aggregated period could be true for all time. Bogoev and Sergi (Citation2012) suggest that segregating the periods or timelines or investment horizons caters for pragmatic investment and policy decisions.
Risk-return trade-off theory and the relationship between risk and return characteristics
The relationship between risk and return is often represented by a trade-off (Di Maggio et al., Citation2020; Kolapo et al., Citation2018). The risk-return trade-off theory states that the expected return of an asset increases as the level of risk increases (Mohamed & Ahmed, Citation2018; Qureshi et al., Citation2019). This connotes a positive correlation between risk and returns, meaning low levels of risk (uncertainty) are connected with lower expected returns and vice versa. A fundamental assumption of the risk-return trade-off theory is that investors are risk averse, and hence require to be compensated for risk bearing. The more investors take risk, the higher the return expected as compensation for the higher risk taken; implying a trade-off (Bricker et al., Citation2019). Thus, investors assess the various classes of asset and their risk and return characteristics before making investment decisions (Beshears et al., Citation2017; Kolapo et al., Citation2018). It can be seen from that; various asset classes have their own risk-return characteristics. An investment with high risk exposure is expected to yield high returns (Qureshi et al., Citation2019).
Table 1. Classes of assets, risk characteristics and risk measure.
Risk and return differ among different classes of investments such as cash and cash equivalents, fixed income, and equities, and therefore over time behave differently in response to macroeconomic and socio-political conditions (Ndlovu et al., Citation2018; Ndubuaku et al., Citation2017). Shares or equities are known to potentially have higher returns as well as higher risk (Jordà et al., Citation2019; Kolapo et al., Citation2018) compared with treasury bills (Bessembinder et al., Citation2019; Gyimah et al., Citation2021). Thus, investing in risky asset such as shares would require adequate risk premium in order to hold onto their assets.
Maximum drawdowns
Most studies on investment performance primarily concentrate on risk-adjusted returns rather than on risk alone. When assessing risk directly, volatility or beta are commonly used as metrics (Riley & Yan, Citation2022). It’s critical to track drawdowns over time in order to evaluate the magnitude of losses an investment is exposed to. As an additional indicator of market performance, maximum drawdown (MDD) measures the difference between the value of the highest peak before the trough and the lowest trough or maximum decline in an investment’s value (Riley & Yan, Citation2022). MDD is easily understood by an investor with no background in finance or mathematics. Investors can enhance their comprehension of investment risk and make well-informed assessments by scrutinising the highest losses encountered in various market scenarios. According to earlier studies, investors’ consideration of information while making judgements about investments is significantly influenced by its saliency (Barber et al., 2005). The computation of MDD relies on changes in cumulative returns and the investment’s volatility is determined by calculating the difference between its highest peak and lowest trough values. MDD gives investors a nearly precise indicator of how much its value will fluctuate in the future. A low MDD number, denotes a smaller level of risk due to the investment’s value swings. Higher MDD increases the possibility that investors may lose capital. When weighing two investment alternatives, the option with the lower MDD value is usually preferred by an investor who wants to be assured of steady returns. However, another investor would select assets with higher MDD values if they were ready to assume a greater level of risk in exchange for a larger return (Riley & Yan, Citation2022).
The Ghana Stock Exchange
The Ghana Stock Exchange (GSE) is the official stock exchange of Ghana. The GSE was incorporated on the 10th of July 1989 and began operation in 1990 (Ghana Stock Exchange, Citation2023). The GSE was adjudged the world’s best performing stock market in 2003. As at Friday 14th January, 2022, the market capitalization of GSE was GHS64, 213.36 million or GHS64billion (an equivalent of $10billion). The GSE currently has 37 companies with 42 listed equities and 2 corporate bonds. Since its inception, the GSE's the GSE All-Share Index listings have been part of its main index. The GSE composite index was introduced in 2011. The exchange has a pre-market session from 9:30 am to 10:00 am and normal trading sessions start from 10:00am to 15:00pm on all days of the week except Saturdays, Sundays and holidays declared by the exchange in advance (Coffie, Citation2019; Ghana Stock Exchange, Citation2023).
Empirical literature
Aggregation bias has received a lot of attention in statistical analyses especially from econometricians who are interested in precisely modelling the relationship between aggregate (macro) behaviour and individual (micro) statistics so that estimations and inferences made regarding economic parameters using data from both the macro and micro level are accurate and inform effective policy decision. Abhishek et al. (Citation2015) define aggregation bias from the perspective of ecology as the expected difference between the effects for the group and the effects for the individual, if there is no confounding. Luloff and Greenwood (Citation1980) defines aggregation bias as deviation of macro parameters from the mean of their corresponding micro parameters. It is the incorrect assumption that ‘what is true about the group, is true about the individual, leading to ‘ecological fallacy’– the conclusion that what is true for the group must be true for the sub-group or the individuals (Abhishek et al., Citation2015). Aggregation bias is therefore the difference in results emanating from lumping the data as compared to their disaggregated components. The aggregation bias hypothesis argues that macroeconomic, political and technological changes affect investment returns over time, and for that matter there is the need to segregate long-run investment periods in order to provide fair, valid and reliable analysis of investment returns. Greenwood and Luloff (Citation1979) noted aggregation bias can have an uneven impact on a regression equation’s overall fitness and can affect how the conventional t-test is applied to aggregated coefficients. The authors report coefficients with varying signs and magnitudes, with the sign varying staying statistically significant. Foroni and Marcellino (Citation2014) and Brockmeier and Bektasoglu (Citation2014) report various forms of aggregation bias in separate studies.
Rising prices are inevitable especially in developing and emerging economics. Generally, inflation reduces the real income and value of an investment (Mohamed & Ahmed, Citation2018). In theory, fixed income earners such as salary workers, interest bearing asset owners such as fixed deposits and T’bill holders suffer most (Kolapo et al., Citation2018) while equity investors such as shareholders and owners of businesses appear to prosper. Thus, stocks perform well in a period of rising prices as the effect of inflation is absorbed as revenue and earnings increases almost at the same magnitude with the rate of inflation (El Abed & Zardoub, Citation2019; Macharia, Citation2018). For this reason, stocks are regarded as effective hedge against both unexpected and expected inflation as nominal interest rate co-vary with inflation. On the contrary, empirical studies (Fama & Gibbons, Citation1981; Katz et al., Citation2017; Zhang, Citation2021) have conflicting results. In the United States of America and other industrialised nations, it is documented that nominal stock return is negatively impacted by inflation (Fama, Citation2014; Katz et al., Citation2017; Zhang, Citation2021). According to Zhang (Citation2021), the negative relationship between stock and inflation are as result of inverse relationship between real activity and inflation.
A number of studies attempted to compare the risk and returns of investment instruments such as stocks, treasury bill and mutual funds to find out which of the instruments performed better against the other using various methodologies. Notable among these researches are Gyimah et al. (Citation2021), Di Maggio et al. (Citation2020), Bessembinder (Citation2018), Antwi et al. (Citation2012) and Aboagye et al. (Citation2008). Other studies (Coffie, Citation2019; El Abed & Zardoub, Citation2019; Gupta & Sinha, Citation2016; Mohamed & Ahmed, Citation2018; Ndubuaku et al., Citation2017; Panigrahi et al., Citation2020) have examined the extent to which macroeconomic factors influence investment returns and have found a significant relationship between macroeconomic variables and investment returns.
Comparing the performance of treasury and stocks using time series data covering a period of eleven (11) years (1991–2001), Aboagye et al. (Citation2008) found that investors in stock over the period realised an average return of 54% annually while treasury bill investors retained an average of 36.3%. Investors in stock retained a positive risk premium of 18%, meaning investors have been rewarded for bearing risk. Their finding again revealed that average returns of both stocks and treasury bill were over and above the average rate of inflation. In similar study Antwi et al. (Citation2012) using annual return covering a period of 21 years (1990–2010) confirm the general expectation of relationship between risk and returns connected with investment–the higher the risk, the higher the return. The findings further indicate that means returns of both stocks and treasury bills are over and above the general increase in price levels (inflation) over the period 1990–2010. From the deliberations, we hypothesize that;
H1: Investments in shares (stocks) have higher returns compared with T’bills
H2: Risk and return characteristics differ among segregated periods.
Methodology
Study design and data
Data used for the empirical analysis comprises stocks returns, T’bill returns, and inflation. prices and returns of stocks on the GSE are reported daily for all working days within the 365 days. The 91-days T’bill used are produced quarterly or four (4) times within the year. The study made use of annualised returns of the two instruments. Hence, data on stock returns, T’bill returns, and inflation from 1990 to 2020 comprising 31 years was obtained from the WDI, the Bank of Ghana website, and the World Bank. The 31-year period was segregated into three investment holding periods (decades): 1990–2000 and 2001–2010, and 2011–2020 and also for the aggregated period. Additionally, eight (8) years investment holding period was also considered based on the number of years the two main political parties (PartyA & PartyB) in Ghana have been in power (i.e. 1992–2000PA, 2001–2008PB, 2009–2016PA, and 2017–2020PB) under the fourth republic. We recognise that there are macroeconomic and political factors specific to the various time periods from 1990 to 2020 that may influence investment returns. For instance, the 1990s was a period of military regime until 1993 when democracy was restored. The year 2000 marks the beginning of the new millennium with all its expectations and also an end to the first regime of the Party A and transition into another regime which is the first regime of the Party B under the fourth republic of Ghana. The first Party B regime ended in 2008, ushering in the second Part A regime, also ending in 2016 and then the second Party B regime from 2017 to 2020. Kempf et al. (Citation2023) opine that ideological alignment is an important, yet an omitted factor in models of (international) capital flows, and that ideological alignment on both social and economic issues affects foreign government cross-border capital allocations by (U.S.) institutional investors. Political parties are inspired by ideologies (Bonaparte et al., Citation2017), and their inclination toward these individual ideologies – be it capitalist or socialist – influence their policies and governance (Mian et al., Citation2023) which impacts investment and returns (Kempf & Tsoutsoura, Citation2021). Bonaparte et al. (Citation2017) in their study documented that investor’s optimism toward macroeconomy conditions and financial markets is influenced dynamically not only by present political climate or ideologies but also by their political affiliation. Holding all other variables constant, investors generally perceive markets to be less risky and more optimistic when their favoured political party is in power (Kempf et al., Citation2023). Mian et al. (Citation2023) and Kempf and Tsoutsoura (Citation2021) observed that investors’ alignment with the ideologies of a political party in power significantly determines their perception of macroeconomic conditions and their allocation of domestic capital.
Macroeconomic environment and cycles impact investment returns. With the discovery of oil in commercial quantities in Ghana, the country had its highest gross domestic product (GDP) growth rate of 14.05% in 2011, and an average GDP of 6.65% (i.e. excluding the GDP for 2020 because of the impact of Covid’19) for the 2011–2020 decade compared with an average GDP growth rate of 5.78 and 4.27% for that of 2001–2010 and 1990–2000 decades, respectively. Changing macroeconomic environments and uncertainties associated with electoral outcomes and change in government have the potential of altering the characteristics of the data obtained, thereby introducing disturbances in the aggregated dada (Bogoev & Sergi, Citation2012; Creswell, Citation2014). In order to avoid selection bias, we conducted an ANOVA analysis for the three separate decades (1990–2000; 2001–2010; and 2011–2020) as well as for different regimes under NDC and NPP (i.e. 1990–2000; 2001–2008; 2009–2016; and 2017–2020) to determine if significant differences exist between the mean returns of the two instruments (stocks and T’Bills). We observed that while no significance difference was observed between the mean returns of stocks for all the two splitting criteria or holding periods (i.e. (1) the three decades and (2) the four different political regimes – i.e. NDC1, NPP1, NDC2, & NPP2) considered in segregating the 31-year period, significant difference was noted between the mean returns of T’Bills (Ref. ; ). Base on the above result we decided to use the three (3) decades segregated periods as the three main holding periods for the purpose of identifying possible aggregation bias.
We analyse risk and returns characteristics separately for the segregated periods and comparison was made among the three segregated periods and between the segregated periods and aggregated periods. The capital market instrument as represented by the GSE All-Share Index and the Composite Index and T’Bill are the two investment variables considered in the comparative analysis. The GSE-CI and the GSE All-Share Index were chosen because it does not only cover all listed stocks but also it tracks the performance of the capital market since its beginning.
Test for normality
Skewness, kurtosis and Jarque-Bera tests are essential measures of normality of a distribution and are important predictors of investment performance (Lee et al., Citation1990; Low et al., Citation2016; Pesaran et al., Citation1989; Pesaran & Pesaran, Citation2010; Rajesh, Citation2019). Skewness measures asymmetry or the distortion of symmetrical distribution in set of data (Low et al., Citation2016; Rajesh, Citation2019). Stock market returns most often demonstrates positive skewness (Di Maggio et al., Citation2020; Gupta & Sinha, Citation2016; Kamal (Citation2018). Hence, one can expect the distribution of stock market returns over the 31-year period to be right skewed. Kurtosis measures the tailedness of a distribution or the frequency or the probability of extreme low or high values (outliers) relative to the mean (Pesaran & Pesaran, Citation2010). It is also essential in measuring the inherent risk of an asset (Gupta, Citation1971). The Jarque-Bera test is a goodness-of-fit test that measures if sample data has skewness and kurtosis similar to a normal distribution (Charteris & Winchester, Citation2010; Gusni et al., Citation2018). The Jarque-Bera test statistic is always positive. A sample is said not to be normally distributed if the Jarque-Bera is farther from zero (Pesaran & Pesaran, Citation2010).
Model specification for risk and returns characteristics of stock and T’bill
The model adopted follows Mohamed and Ahmed (Citation2018) and Antwi et al. (Citation2012). In this model, two investors are considered: investor ‘A’ and investor ‘B’. Investor ‘A’ started the year 1990 by investing an amount (e.g. GHS1000.00) of money in a 91-day Treasury bill. Investor ‘B’ started the same year by purchasing one share each of the listed companies on the GSE with the same amount of money. Proceeds from the two investments are reinvested into their respective instruments over the various time frame (Aboagye et al., Citation2008; Antwi et al., Citation2012).
Testing for aggregation bias
One simple way to test the aggregation bias hypothesis in the view of Pesaran and Pesaran (Citation2010) is to test whether or not:
(1)
(1)
where
=the mean risk and return characteristics, correlation coefficient, and maximum drawdowns for the aggregated period (1990–2020)
=the mean risk and return characteristics, correlation coefficient, and maximum drawdowns for the first disaggregated period (1990–2000)
=the mean risk and return characteristics, correlation coefficient, and maximum drawdowns for the second disaggregated period (2001–2010)
=the mean risk and return characteristics, correlation coefficient, and maximum drawdowns for the third disaggregated period (2011–2020)
In the absence of aggregations bias, must be equal for each individual unit. This indicates homogeneous behaviour among the units of which the aggregated data is composed. If the opposite holds, then the units have heterogeneous behaviour (Pesaran & Pesaran, Citation2010), and for that matter, there is an aggregation bias.
Determination of aggregation bias in respect to present the study seeks to assess if the risk-return trade-off theory as confirmed by the results of earlier studies that used aggregated data is true for those of the segregated data. Thus, aggregation bias hypothesis in the view of Pesaran and Pesaran (Citation2010) is to test whether or not the mean returns (R) and risk and return (RR) characteristics of the two instruments differ significantly among the segregated periods. In testing for aggregation bias, the study investigates whether or not risk and return characteristics of the aggregated and segregated periods are the same as shown in the equation:
(2)
(2)
Where is the risk and return characteristics of the aggregated period while
is the risk and return characteristics of the segregated periods.
Bogoev and Sergi (Citation2012) investigated aggregation bias in regard to interest rate pass-through in the Republic of Macedonia and found the presence of aggregation bias, implying that the empirical studies based on aggregated data may provide biased results when compared with studies that used segregated data. Disaggregating the data takes into consideration changes in macroeconomic condition over the long-run and may provide more useful insight for policy decisions. This model was adopted in the present study in assessing the short-and long-term risk and return characteristics of stocks and treasury bills.
Correlation analysis
The result of correlation analysis is a remarkable ‘revealer’ of an aggregation bias by virtue differences that may be observed in the slope and magnitude of different individual datasets drawn from the aggregated data (Pesaran & Pesaran, Citation2010; Rajesh, Citation2019; Wade et al., Citation2019). In view of this, correlation analysis is carried out for the three individual segregated periods or decades (i.e. 1990–2000, 2001–2010 and 2011–2020) to ascertain possible differences slope and magnitude among the three individual datasets. This is helpful in establishing whether or not i (Pesaran & Pesaran, Citation2010). This is mathematically represented as:
(3)
(3)
Where is the slope of the correlation of the aggregated period while
is the slope of the correlation of the segregated period.
Maximum drawdowns
As indicated earlier, maximum drawdowns (MDD) measure is a measure of stand-alone risk that provides salient information to investors regarding investment volatility. In order to measure aggregation bias and to determine whether or not MDD for the aggregated period is similar to the segregated periods, we have:
(4)
(4)
Where is the Maximum drawdown of the aggregated period, and
is the maximum drawdown of the segregated period.
ANOVA analysis
The study aimed to determine whether or not aggregation bias exist in lumping the years together (1990 to 2020). In view of this, analysis of variance (ANOVA) for the three segregated periods (1990–2000, 2001–2010 and 2011–2020) is carried out to ascertain the significance difference among the means of the three segregated periods using E-views.
Mathematical models
Standard deviation, coefficient of variation and beta are the most popular measures of investment risk. Standard deviation measures total risk while Beta measures the systematic risk of an investment asset, by contrasting the return of the individual portfolio with that of the market (Macharia, Citation2018). Since the present study measure the entire market risk of stocks listed on the GSE, we use the standard deviation as proxy to measure total risk. The co-efficient of variation measures the risk per unit of returns (i.e. risk borne by investors relative to the returns) and is useful in comparing the risk-adjusted performance of two assets. For the measurement of returns, we use the arithmetic mean. For the purpose of comparison among the two instruments, the historical annual returns on stocks and T’bills were subjected to the following statistical measures: nominal and real mean returns, the risk premium, the coefficient of variation, standard deviations, the Sharpe ratio and maximum drawdowns (MDD). All figures are at the end of period calculations. The mathematical model for risk and returns are shown in .
Table 2. Variable measurement.
Results
This section presents the result on data analysis covering descriptive statistics, skewness, kurtosis, Jarque-Bera test, ANOVA, correlation, risk and return characteristics of stocks and T’bill returns for the segregated periods: 1990–2000, 2001–2010, 2011–2020, and 2001–2020 and the aggregated period 1990–2020 for the purpose of ascertaining aggregation bias in stocks and T’bill returns.
Trends in T’bill and stock returns
The pictorial view of the data is presented in , which projects the trends in stocks and T’bill returns from 1990 to 2020. The trends in stocks returns shows higher level of fluctuations depicting volatility in stock exchange returns compared with the trends in treasury bill returns ().
Descriptive statistics
Analysis of descriptive statistics was carried out with particular emphasis on skewness, Kurtosis, Jarque-Bera test, and their respective probabilities.
Stock returns ranged from a minimum of −46.58 to a maximum of 154.67. The mean stock returns over the aggregated period was 23.6682 and the standard deviation was 50.559. Skewness and kurtosis statistics is 1.0451 (), indicating a high and right skewed distribution. This confirms the general expectation regarding the distribution stock market returns (Low et al., Citation2016; Pesaran & Pesaran, Citation2010; Rajesh, Citation2019). It is observed that stock returns have a kurtosis of 3.2494 (), which relates to an excess kurtosis of 0.2494 (i.e. 3.2494–3). This means that the distribution is far from a normal distribution. Additionally, kurtosis greater than 3 implies a fatter tailed distribution. This means there is high probability or frequency of extreme low or high data values. Thus, the positive skewness and high kurtosis statistics implies the likelihood of extreme high or low values of stock returns distributed at the right (Pesaran & Pesaran, Citation2010). The distribution of extreme low or high values at the right implies stock investors can expect positive returns in the long-run. The t-statistics of the Jarque-Bera test is 5.169 with a p-value of 0.0754 (). We therefore reject the null hypothesis and conclude that stock market returns on GSE is not normally distributed.
Table 3. Descriptive statistics.
Inflation has a mean of 2.8275 and a range of 1.582 minimum and a maximum of 4.08533. The standard deviation computed is 0.6236. The observed skewness indicates a low and positive skewness of 0.2020, implying that the distribution of data in respect to inflation is skewed to the right. The kurtosis value is 2.3433, implying an excess kurtosis of −0.6587, signalling platykurtic. In other words, rate of inflation has lighter tail. This means that low frequency of extreme rate of inflation distributed on the left. The Jarque-Bera test shows a t-statistics of 0.6934 and a p-value of 0.7069 (). In view of this, we refuse to reject the null hypothesis. Hence, we conclude that rate of inflation follows a normal distribution.
Mean T’bills returns is 3.114, and ranges from a minimum of 2.297 and a maximum of 3.7558. The standard deviation observed is 0.4191. The skewness statistics shows a negative skewness of −0.2755, implying a left skewed distribution. The observed kurtosis is 2.0955, implying an excess kurtosis of −1.9045 (i.e.2.0955-3) a light tail distribution. The t-statistics of the Jarque-Bera test is 1.3066, with a p-value of 0.52033 (). Since the p-value is greater than 0.1 significant level, we refuse to reject the null hypothesis and therefore conclude that the distribution of T’bill returns is normal.
ANOVA analysis to determine possible aggregation bias
Differences in means provide evidence of aggregation bias. The ANOVA was carried out to examine significant differences in the mean returns of the two instruments for three segregated periods. The ANOVA analysis is shown .
Table 4. Results of ANOVA analysis.
The ANOVA analysis as shown in indicates a p-value of 0.000 for the mean treasury bill returns for the three segregated periods. The null hypothesis state that there is no significant difference among the means of treasury bill returns (i.e. there is no aggregation bias) for the three segregated periods. Using the 0.01 significant level, it is stated that the null hypothesis should be rejected if the p-value is less than or equal to 0.01. Therefore, since p-value is less than 0.01, we reject the null hypothesis. This means that there is a significant difference among the means treasury bill returns for the three segregated periods. This is confirmed by a higher F-statistics of 12.812. Thus, the mean return for 1990–2000, 2001–2010, and 2011-2020 differ significantly. This implies that there is an aggregation bias in lumping the years together with respect to treasury bill returns.
The ANOVA analysis for stock returns indicates a p-value of 0.482. We therefore refuse to accept the null hypothesis at 0.01 significant level. This means that there are no significant differences in the mean stock returns among the three segregated periods. This implies that there has not been a significant change in stock market performance (returns) since its inception in 1990. In other words, there is no aggregation bias in lumping the years together when assessing the risk and return characteristics of stock investments in respect to the Ghana Stock Exchange.
Correlation analysis
In order to assess the occurrence of aggregation bias in lumping the investment years together, correlation analysis is carried out for the three separate periods or decades (1990–2000; 2001–2010; and 2011–2020) as well as the aggregated period (1990–2020).
From , the first, second, and third decades (1990–2000; 2001–2010; and 2011–2020) of stock investment, the relationship between stock returns and T’bill returns in Ghana was positive, though not significant as shown by correlation coefficient of 0.103, 0.108, and 0.103 respectively for the 1990–2000, 2001–2010, and 2011–2020 investment periods (0.05 significant level). Similarly, for the aggregated period, the correlation between stocks and T’bill returns was positive but insignificant as indicated by correlation coefficient of 0.148. This observation differs from theoretically expected inverse relationship between stock and money market returns. Bessembinder et al. (Citation2019) and Chopra (Citation2019) indicated that risk in money stock and money markets co-varies. Bricker et al. (Citation2019) and Bessembinder (Citation2020) argued that although the unconditional correlation between T’bills and stocks are low, there exist a strong volatility between the two markets, a condition that is impacted by risk and return interaction that exist between two markets via trading activities. (Bessembinder, Citation2020; Jayech, Citation2016; Kamal, Citation2018), arguing for example, that unfavourable information in respect to stock market may result in ‘flight to quality’ as investors replace safer instruments for risky instruments (Jayech, Citation2016). And that the resulting outflow from stocks into Treasury bills may cause price pressures which may affect T’bill and stock returns (Abhishek et al., Citation2015; Menyah et al., Citation2014), and vice versa. Additionally, increase in T’bill rates is expected to affect capital flow into the stock market (Gupta, Citation1971; Kamal, Citation2018) and businesses as invest seek to maximise their return by investing in T’bill. However, due to lack of liquidity (Coffie, Citation2019; Menyah et al., Citation2014; Rajesh, Citation2019), investors are unable to react promptly to stock market information. Again, different investors invest in the two markets. It has been noted that while most Ghanaian prefer to invest in T’bills, majority of investors in the Ghana stock market are foreigners (Qureshi et al., Citation2019) who most often are unable to switch investment for lack of liquidity.
Table 5. Correlation matrix.
In respect to stock returns and inflation, the relationship between stock returns and rate of inflation was negative for two of the decades (1990–2000 and 2011–2020) as shown by correlation coefficient of −0.096 and −0.031, respectively. Similarly, studies by Zhang (Citation2021); Katz et al. (Citation2017) and Fama (Citation2014) have observed that nominal stock returns are negatively correlated with rate of inflation in the United States of America and other industrialized economies. Zhang (Citation2021) and Rajesh (Citation2019) indicated that the inverse relationship between stock returns and rate of inflation is due to negative relationship between rate of inflation and real activity, particularly when the general rise in price levels is caused by increasing cost of inputs such as increase in fuel prices, prices of raw material, etc. as well a fall in the purchasing power of households.
The 2001–2010 investment period shows a positive relationship between stock returns and rate of inflation as indicated by correlation coefficient of 0.193. This observation confirms theoretical expectations that stock performs better in the period of rising prices since revenue earnings rises with the rate of increase in inflation (El Abed & Zardoub, Citation2019). In theory, rising prices result in increase in real earnings due to the proposition of lead-lag (i.e. the assumption that, increase in the cost of production is less than the increase in the final price of finish products). Similarly, studies by Boamah (Citation2019) and Ndlovu et al. (Citation2018) have indicated a positive relationship between stocks and rate of inflation. For aggregated period (1990–2020), the relationship between stocks and rate of inflation was positive as indicated by correlation coefficient of 0.087. Similarly, using an aggregated period of 21 years, Antwi et al. (Citation2012) observed a positive relationship between stock returns and rate of inflation and argued that stocks are a better hedge against inflation especially when they are maintained over the long-run. The observed differences in the signs of the correlation coefficients of stock returns and rate of inflation provides evidence of aggregation bias.
A significant strong positive relationship was found between T’bill and rate of inflation for all the three decades as shown by a correlation coefficient of 0.605, 0.850, and 0.835, respectively for the 1990–2000, 2001–2010, and 2011–2020 segregated periods. Correlation fort the 1990–2020 aggregated period was 0.756, which is a strong positive relationship. Policy rate is key determinant of the T’bill rate, and since the bases of setting policy rate in Ghana is the rate of inflation, T’bill rate are expected to be co-move with the rate of inflation.
Analysis of risk and return characteristics for the aggregated period (1990–2020)
Risk and return characteristics of treasury bill and stocks are analysed and compared in respect to their nominal and real returns, the cumulative real value of investment (here after CRVI), standard deviation, and coefficient of variation for the aggregated period 1990–2020 are detailed in .
Table 6. Risk and return characteristics of treasury bill and stocks for the aggregated period (1990–2020).
shows that stocks and T’bills have a positive nominal return over the period. Stocks gave a slightly higher mean returns of 25.35% compared with that of T’bill (23.31%) over the 31-year period (1990–2020). This means that for the aggregated period, stocks have outperformed T’bills. This observation confirms the findings of earlier studies by Antwi et al. (Citation2012) and Aboagye et al. (Citation2008) who also used aggregated years of 21 and 14 years, respectively.
The positive real rate of returns for the two instruments () implies that T’bill and stocks have performed above the general rise in price levels. However, stocks have outperformed T. bill as shown by the real rate return of 6.4%% for stocks as compared with 4.40% for T. bill per annum. Thus, for the aggregated long-run period of 31 years, stocks have been observed as better hedge against inflation. This confirms earlier argument by Boamah (Citation2019) and Nasser et al. (Citation2018) that high rate of real returns of stock serves as hedge against inflation compared with T’bill. According to Nasser et al. (Citation2018), an increase in inflation may lead to increase in real earnings of firms due to the proposition of lead-lag effect– i.e. cost increments are most often less than the increase in the final price of finish products during time of inflation which results in high profits (Mohamed & Ahmed, Citation2018). This observation indicates that in a period of rising prices, investment in stock could yield higher returns in the long-run.
In terms of real value of investment, Investor ‘A’ (treasury bill investor) tend to have a higher cumulative real value of investment of GHS3457.72 at the end of the 31-year period compared with investor ‘B’ (Stock investor) who obtained a cumulative real investment value of GHS253.64. It was argued in the study of Antwi et al. (Citation2012), which covered 21 years period that 21 years is not enough for investors to make abnormal return. Assuming Antwi et al. (Citation2012) argument is true, the 31 year period covered by investors in stock should be enough to make returns that provide adequate compensation to investors in real terms.
In respect to risk, the GSE Index (SD = 48.39%) has turned out to be highly risky security compared with T’bill (SD = 9.77%). Comparing the risk and returns of the two instruments, it can be seen that the GSE index has a higher risk and higher return compared to T’bills. This observation is in sync with the general expectation of finance theory associated with risk and returns of an investment, that the higher the risk, the higher the returns.
One cannot be sure if the risk borne by a particular security is worth it or not. The Coefficient of Variation measures the relative risks of the two investments and indicates the extent to which the two investors have been compensated for the level of risk they bear by making adjustments. This adjustment is made by expressing the mean rate of return as a ratio of the SD. Therefore, the coefficient of variance shows the level of risk borne by the investor in realizing each unit of returns. The 91-Day T. bill shows a lower covariance of 0.42 while the GSE index had high covariance of 1.91. This means that for each unit of return obtained, T’bill bear relatively lower risk of 0.42% compared to stocks (1.91%). Hence, investors in T’bill were compensated more adequately for bearing risk compared with investors in stocks. Thus, though stock had higher returns compared with T’bill, that high return obtained does not match the level of risk borne.
Analysis of risk and return characteristics for the 1990–2000 segregated period
Risk and return characteristics of treasury bill and stocks are analysed separately for the first decade (1990–2000 segregated periods; see ).
Table 7. Analysis of risk and returns characteristics of T’Bill and GSE returns for the 1990–2000 segregated period.
From , T’bill had a nominal mean returns of 32.14%, while stocks had a mean return of 29.97% over the 11-year period. The results show that in nominal terms, both stocks and treasury bill gave a positive return during the period. However, GSE index posted a lesser mean return compared with stocks return over 11 the year period. Over the same period, stock had a negative real rate of return of (2.59%) while T’bill had a mean real rate of return of 4.75% over the same period. This means that, average T’bill returns are over and above the annual rate of inflation. Stock returns are however below the annual rate of inflation for the 1990–2000 period. This implies that for the 1990 to 2000 segregated period, stock returns were not a better hedge against inflation as was observed for the aggregated period (1990–2020). This observation again contradicts earlier findings by Boamah (Citation2019), Antwi et al. (Citation2012), Aboagye et al. (Citation2008). Thus, for the first decade (1990–2000), T’bill was a better hedge against inflation for the 1990–2000 segregated period. Consequently, the cumulative real value of investment for stocks dropped from GHS1000 initial money invested in 1990 to GHS239.90 in 2000. This means a loss of GHS760.10 (−76.01%) in the real terms. On the other hand, T’bill had a real investment value of GHS1,536.67 (Ref. ). This means that T’bill had a real return of GHS536.67 (GHS1,536.67. – GHS1,000.00) over the 11 year period, representing a proportional increase of 53.67% (4.87% annually) in real value of investment. It can be noted that 1990 to 2000 period was the initial stages of commencement of stock market in Ghana and this may have impacted its performance.
Stocks (SD = 51.79%) turned out to be by far riskier instruments compared with T’bill (SD = 6.84%). It is noted earlier that, the T’bill recorded higher returns both in nominal and real terms but had lower risk compared with stocks. This observation is at variance with the general expectations of finance theory that positively correlates high risk with high return; the higher the risk, the higher the return, and vice versa. Thus, risk and return characteristics of stocks and T’bill for the 1990–2000 period violates the theory risk-return trade-off theory (Chopra, Citation2019; El Abed & Zardoub, Citation2019). Thus, the higher risk borne by investors in stocks were not compensated by higher returns.
The coefficient of variation helps to standardize the risk and returns of the various instruments by measuring the relative risk borne by the investors in respect to the level of returns obtained. It measures the per unit risk of each unit of returns realized. In respect to the coefficient of variation, the 91–Day Treasury bill realized relatively lower CoV of 0.21 while the GSE Index reveals a very high CoV of 1.73. This means that for each unit of return obtained, investors in treasury bill borne a smaller unit 0.21% of risk, while investors in stock borne 1.73% unit of risk in order to gain a unit of return. Investors in T’bills were better rewarded bearing risk compared with stocks investors during the first decade (i.e. 1990–2000 segregated period) of its commencement.
Analysis of risk and return characteristics for the 2001–2010 segregated period
The annual returns realised by stocks and treasury bill investors for the second decade after commencement of the GSE (i.e. 2001–2010 segregated period) are analysed by making comparison between the instrument’s risk and return characteristics as indicated by the nominal returns, real returns, cumulative real value of investment (CRVI), standard deviation and co-efficient of variation of the instruments as shown in .
Table 8. Risk and return characteristics of T’bill and stocks for second decade (2001–2010).
The result indicates that both GSE returns and treasury bills obtained a positive nominal return for the 2001–2010 segregated period. However, the return of stocks was higher compared T’bill. While stock gave an average return of 35.41%, treasury bill gave a mean return of 19.84% over the same period. In real terms, the stocks (15.57%) outperformed T’bills (2.81%) Thus, returns on both stocks and Treasury bill are over and above the rate of inflation for 2001–2010 investment period. Compared with 1990–2000 investment period, stock recorded a higher real rate of return compared with treasury bill. The high mean real return rate realised by stocks during the period 2001–2010 indicates that stocks are a better hedge against inflation (Antwi et al., Citation2012; Chopra, Citation2019; Zhang, Citation2021) than T’bill during the second decades. This means in real value terms stock outperformed treasury bill over the same period. Contrary to the first decade where stocks had a negative real return, stock returns during the second decade (2001–2010 period) was impressive. Comparing the risks, stocks have higher risk as compared to T’bills. The risk and return characteristics of the 2001–2010 investment period confirms the general observation of the finance theory; the higher the risk, the higher the return.
The Coefficient of Variation, the 91–Day T’bill is lower (0.48) compared with stock (1.64). Thus, for each unit of return, 0.48 unit of risk was borne by T’bill investors, while for every unit of return realized by investors in stocks, 1.64 units of risk was borne by them. This indicates that Investor ‘A’ (i.e. treasury bill investors) was compensated more adequately for bearing risk compared with investor ‘B’ who invested in stocks over the same period (2001–2010). Therefore, though investors in stocks obtain higher returns compared with T'bill investors, investors in treasury bill have better risk-adjusted returns for their investment.
Analysis of risk and return characteristics for the third decade (2011–2020 segregated period)
shows that the mean nominal annual rate of returns for stocks and treasury bill for the period 2011–2020 indicate positive returns over the 10 year period.
Table 9. Risk and return characteristics of T’bill and stock for 2011–2020 segregated period.
T’bills (17.08%), compared to stocks (10.10%) has higher returns. The result further indicates that in reals terms, treasury bill has outperformed stock as it recorded a real rate of 5.59% compared with 1.39% obtained by stocks. The analysis of real money value of the two investments shows that while the real value of the GHS1000 invested in treasury bill gave real investment value of GHS1,716.06 for T’bill, the real value of the same amount invested in stocks at the end of 2020 was GHS561.33. This means that while T’bill gave a positive cumulative real value of investment of GHS1,716.06 over the 10-year period, stock yielded a negative cumulative real value of investment (GHS561.33-1000). In other words, there was a loss in real value of GHS1000 invested by GHS438.67. Thus, for the 2010–2020 investment period, stock have not been a better hedge against inflation (Aboagye et al., Citation2008; Bessembinder, Citation2018).
Analysis of risk shows that the GSE Index, i.e. stocks (SD = 32.30%) turned to be by far riskier instruments compared with T’bills (SD = 4.64%). The comparative return analysis shows that T’bill has higher returns and lower risk as compared with stocks. This observation contradicts the general expectation of the theory of finance that state that the higher the risk, the higher the return, and vice versa. The coefficient of variation for the 91-Day T’bill is lower (0.27), while stock has a very high coefficient of variation of 3.20. Thus, for each unit of return realized, 3.2units of risk was borne by investors in stock while only 0.27 units of risk was borne by investors in T’bill. This means that for the two instruments, investors in T’bill are being compensated more adequately for bearing risk compared with investors in stock.
Determination of aggregation bias in respect to risk-return trade-off
Aggregation bias has been analysed in respect to the extent to which risk and return characteristics of the three segregated periods and the aggregated periods confirm or contradict the risk-return trade-off theory. The result of the analysis is reported in . The result shows that in exception of 2001–2010 investment period, T’bills have outperformed stocks in all the segregated period.
Table 10. Determination of aggregation bias (risk-return trade-off).
The risk and return characteristics of the aggregated period (1990–2020) confirms the risk-return theory as shown by higher returns and higher risk of stocks, similar to the findings of Aboagye et al. (Citation2008) and Antwi et al. (Citation2012) who lump the years together. However, the risk and return characteristics of the two segregated periods (i.e. 1990–2000 and 2011–2020) out of the three decades (i.e. segregated periods) contradict the risk-return trade-off theory contrary to the findings of Aboagye et al. (Citation2008) and Antwi et al. (Citation2012). Risk and return characteristics of only one of the segregated periods (2001–2010) confirms the risk-return trade-off theory. In the absence of aggregation bias, we expect i (Pesaran & Pesaran, Citation2010). Therefore, the result of the aggregation bias tested indicated that
i, implying that risk and return characteristics of the segregated and aggregated periods vary. Differences in the risk and return characteristics of the segregated and aggregated period gives evidence of aggregation bias. Hence, we conclude there is aggregation bias in risk and return characteristics of stocks and T’bills. This means decision made only based on the aggregated data could be misleading.
Further analysis: maximum drawdown and likelihood of loss
Maximum drawdown as a measure of fall in the value investment over an investment period is used as our robustness check. The maximum drawdown as computed for the aggregated investment period as well as for the three segregated investment period are shown in . Given the significant effect of inflation on the cumulative real value of investment in most developing economies, both nominal and real maximum drawdowns were computed for both stocks and T'bills. It is observed that stock suffered a maximum drawdown of 29.53 and 71.08% (in nominal and real terms) real over the aggregated period with a recovery period ranging from 1 to 3 year after the drawdown. Nominal drawdown for the 1990–2000, 2001–2010, and 2011–2020 are 15.22, 38.21 and 25.30%, respectively. There is are remarkable differences in the nominal maximum drawdowns between the aggregated period and aggregated period. In real term, stocks suffered a high maximum drawdown of 68.48, 69.68, and 67.35%, respectively for the 1990–2000, 2001–2010 and 2011–2020 segregated period, suggesting high level of risk to investors. This indicates that the high maximum drawdowns suffered by stocks is worsen by inflation. Additionally, there was no remarkable difference in nominal maximum drawdowns between the aggregated and segregated period.
Table 11. Maximum drawdowns and likelihood of loss.
In nominal terms, T’bill suffered no maximum drawdowns for both the aggregated and segregated period. Maximum drawdowns of 27.82% was however suffer in real terms over the aggregated period. This implies that in real terms, the maximum fall in value of investment for investors who invested in T’bill was 27.82% at some point within the aggregated investment period. For the segregated period, real maximum drawdowns for 1990–2000; 2001–2010; and 2011–2020 segregated investment period were 27.82, 2.26 and 0, respectively. T’bill reported no nominal maximum drawdowns for all the three period. According to Riley and Yan (Citation2022), volatility is possible in a fund with non-constant increment in value, but no maximum drawdown. The differences in maximum drawdowns for the aggregated and segregated is an indication of aggregation of aggregation.
Hypothesis testing
First hypothesis: Investments in shares (stocks) have higher returns compared with T’bills
To test the hypothesis, we seek to find the significant differences in the mean returns of the two assets by examining the group statistics and then the independent sample tests are carried out. The results of the group statistics as shown in give the group comparison relating to the mean, standard error and standard deviation. The mean T’bills returns is 18.92% and the mean stocks returns is 25.32% over the 31-year period. The standard deviation of T’bills is 11.98% and stocks is 48.39%, corresponding to a variance of 2.15% for T’bills and 8.69% stocks.
Table 12. Group statistics.
The results of the variance analysis are indicated in . We would expect the entire length of the boxplot to be almost the same for the two groups (i.e. stocks and T’bills) if the variances are equal. We can hence expect the Independent Samples t Test and observe the Levene’s Test is significant.
The results of the independent sample test as shown in comprises two parts: (1) Levene’s Test for Equality of Variances and (2) t-test for Equality of Means. The p-value for the Levene’s test is 000. For this reason, we reject the null hypothesis and conclude that variance in T’bills is significantly differ from that of stock returns. The mean difference is −6.40% (18.9177 minus 25.3181) and a corresponding t value of −0.715. The negative t value is an indication that the mean returns for first group (T’bill) is significantly lower compared with the mean of the second group (stocks). Therefore, we conclude that there is a significant difference in the mean T’bills and Stocks returns and that stock has outperformed T’bills.
Table 13. Test for equality of means and variances between Stocks and T’bills.
Second hypothesis: Risk and return characteristics differ among segregated periods
To empirically establish the presence of aggregation bias, equality between the means and variances of the segregated periods (1990–2000, 2001–2010, and 2011–2020) and the aggregated period is tested. This is shown in . The differences in means as tested using the Anova F-test and Welch F-test shows no significance differences in mean return of stocks among the three segregated period as p-values for both Anova F-test and Welch F-test are greater than 0.05 and for that matter null hypothesis is reject. On the hand, p-values of Anova F-test and Welch F-test for T’bill returns are less than 0.05, hence we refuse to reject the null hypothesis. Thus, significant differences exist between T’bill mean returns among the segregated periods. This finding is further supported by an observed differences in skewness and kurtosis among the segregated periods of T’bill return (See and ). We therefore conclude that while there is aggregation bias in mean returns of T’bills, no aggregation bias is present with mean returns of stocks in Ghana. Test for equality between variances of stocks and T’bills shows p-values of Bartlett, Levene, and Brown–Forsythe tests to be greater than 0.05. For this reason, we reject the null hypothesis and conclude that no significance differences exist in the variances between stocks and T’bill returns among the segregated periods.
Table 14. Test for equality between means and variances of stocks and treasury bills.
Conclusion
The present study provides interesting insight into aggregation bias. Levene’s Test for Equality of Variances indicates stocks significantly have outperformed T’bills over the 31-year aggregated period. However, the performance of T’bill was above stocks for 1990–2000 and 2011–2020 segregated investment periods. The risk and return characteristics of the 1990–2020 aggregated period confirm the general expectation of the finance theory; the higher the risk, the higher the return. The risk-return characteristics for 1990–2000 and 2011–2020 segregated period contradict the general expectation of risk and return associated with investment as T’bill had higher return yet lower risk compared with stocks. The differences in the risk and return characteristics of the segregated periods and the aggregated period provided proves possible aggregation bias in lumping the years together. While aggregated period confirms the finance expectation of risk-return trade-off, two of the segregated period (1990–2000 and 2011–2020) contradict it. ANOVA analysis, Anova F-test and Welch F-test reveals that there is a significant difference between the mean T’bill returns for the segregated periods indicating the presence of aggregation bias only for T’bills and not for stock returns.
The presence of aggregation bias in risk and return characteristics of stock and T’bill returns has a grave implication for investors and policy decision. The present study shows investment decisions made using the aggregated period could be misleading. The study demonstrates that risk and return characteristics of stocks and T’bill have not followed same trends when compared with those of the aggregated period. Disaggregation could help solve problems of aggregation bias by bringing to the fore, the unique characteristics of the individual units or periods within the aggregated dataset. Therefore, investors need to consider not only investment performance over the aggregated period but also the segregated periods. Additionally, policy makers must give careful consideration to the disaggregated periods when formulating policy that guide and regulate investment in Ghana. This paper’s main contribution can be seen in its investigation into the possibility of aggregation bias in the results reported by earlier research based on aggregated data. For example, prior empirical studies indicate that the risk-return characteristics of stocks and T’Bills in Ghana confirms the trade-off between risk and return associated with investing, and that investors are rewarded for taking additional risk. We contend, however, that these results may have suffered aggregation bias because these studies were carried out using aggregated data. As a result, this could distort investor perception and affect policy strategy and decisions that should encouraged increased stock market investment and liquidity.
Our results imply that holding all other factors constant, stock investments’ risk and return characteristics changes over time. Our study’s results indicate that stock investments made in the second decade (2001–2010) can yield significant returns for investors if they are held for a reasonable amount of time. This increases investors’ confidence, participation and liquidity result in market efficiency.
When we consider risk alone without return adjustment, maximum drawdowns of stock exchange traded shares throughout the four separate investment period did not differ significantly (i.e. ranging from 67.35 to 71.08%), implying none existence of aggregation bias. This is supported by ANOVA results that shows that unlike T’bill, no significant difference was observed in the mean returns of stocks in Ghana. Thus, while aggregation bias was observed in respect to the risk of T’bill, stocks suffered no aggregation bias when risk alone is considered.
Though, earlier studies by Antwi et al. (Citation2012) concluded that investors in stocks were rewarded for bearing risk based on a positive risk premium, our study showed that the level returns obtained was not enough to compensate for the level of risk borne. Given that investors appreciate investment performance better based on increase in purchasing power, our study demonstrates that the cumulative real value of investment for stocks for example over the 31-year aggregated period was GHS 253.64 implying a 74.64% fall (i.e. of GHS 1000.00 initially invested) in the real value over the 31-year holding period. For the same period, the cumulative real value of investment for T’bill was GHS 3,457.72 representing 245.77% increase in the real value. On the other hand, stocks outperform T’bill in terms of real value in the 2001–2010 investment period (i.e. T’bill realised GHS 1,311.22 while stocks obtained GHS 1,545.70 cumulative real). These differences are clues of existence of aggregation bias with regard to risk and return characteristics. The presence of aggregation bias again implies that all things being equal, investment performance are not static for all times. There are political and macroeconomic conditions that impact investment performance. Thus, an investment strategy that was successful in one period may not be successful in another. It also implies that a policy might be useful in some periods and not in others, and therefore require a review to reflect existing conditions in order to be effective. Therefore, policy makers should carefully consider relevant or peculiar timelines of interest that responds to particular needs of individual periods.
Aggregation bias as demonstrated by the present study is an indication that there are variables that have caused a change in risk and return characteristics of stocks and T’bill over the past three decades. The present study does not cover these variables (factors). Further studies are needed to establish the determinants of stocks and investment performance in Ghana.
Closely related with the issue of aggregation bias is the question of whether not political party regime influence investment performance in Ghana as has been alleged among some elite Ghanaians and political commentators. At present, apart from the effect of political instability on investment performance, preliminary literature search indicates no empirical research on the subject matter. Further studies aimed at investigating the impact of a particular political party on investment performance in Ghana could erase prejudices that may impact investor behaviour, policy and investment decisions.
Disclosure statement
No potential conflict of interest was reported by the author(s).
Additional information
Notes on contributors

Dodzi K. Dunyo
Dodzi Dunyo ([email protected]) received his B.A degree in Publishing Studies, from the Kwame Nkrumah University of Science and Technology, Kumasi, Ghana in (2008), his Master of Business Administration (Finance) degree in (2014), and his MPhil in Business Administration from the same university. He currently works as a business consultant and researcher at Pointers Institute of Business Development and Research, Kumasi.
Ellis Kofi Akwaa-Sekyi
Ellis Kofi Akwaa-Sekyi ([email protected]) holds a Ph.D. in Law and Business Administration (Banking and Finance option) from the University of Lleida, Spain. He is a lecturer at Kwame Nkrumah University of Science and Technology (Dept. of Accounting and Finance at the KNUST School of Business). He is an associate researcher at the University of Lleida, Spain, University of Bari (Aldo Moro), Italy and University of Vaasa, Finland. Ellis Akwaa-Sekyi has graduated with MSc Micro Finance and MPhil Banking and Finance from the University of Cape Coast, Ghana and Kwame Nkrumah University of Science and Technology, Ghana respectively. He has presented at international conferences and published in the areas of Corporate Governance, Risk Management, Internal Controls, Micro Finance and Sports. Ellis is a certified peer reviewer for several journals including Cogent Economics and Finance, Journal of African Business, Cogent Business and Management, Journal of Financial Reporting and Intangible Capital among others.
Joseph Magnus Frimpong
Joseph Magnus Frimpong ([email protected]) is a Professor in Finance at the School of Business, KNUST. He is also an Economist and a Business Consultant. He is a Director of some successful businesses including Juaben Oil Mills Limited, Juaben-Ashanti and Sir Magnus Hotel in Kumasi. He was appointed a member of the governing board of the National Development Planning Commission (NDPC) of the Republic of Ghana in 2019. Prior to his appointment to serve on the NDPC board, Prof. Magnus Frimpong functioned as a Dean of KNUST School of Business in 2007. He also served as a Head of Department, Department of Accounting and Finance, KNUST School of Business between 2007 and 2015. He has rich international experience and in-depth knowledge in Mathematics, Economics, Finance, Econometrics, Research, Entrepreneurship and Business Consulting. He has attended several international conferences and published in reputable journals in the areas of Finance, Economics. Prof. Magnus holds a PhD in Finance from KNUST, an MSc. in Project Analysis, Finance and Investment from the University of York, England and a BA Hon. in Economics and Law from KNUST. He holds Teachers’ Diploma in Mathematics from the University of Education, Winneba and a Qualified Overseas Teachers’ Status in England.
Akua Peprah-Yeboah
Akua Peprah-Yeboah ([email protected]) holds PhD in Accounting and lectures at the Accounting and Finance Department of KNUST School of Business, KNUST-Kumasi, Ghana. She has published and presented in International conferences. She teaches Cost and Management Accounting and Financial Accounting. Her research focus includes but not limited to Corporate Social Responsibility Accounting, Climate Change Accounting and Finance, and Corporate Governance. She loves the quantitative approach to research.
References
- Abhishek, V., Hosanagar, K., & Fader, P. S. (2015). Aggregation bias in sponsored search data: The curse and the cure. Marketing Science, 34(1), 59–77. https://doi.org/10.1287/mksc.2014.0884
- Aboagye, Q. Q., Akoena, S. K., Antwi-Asare, T. O., & Gockel, F. A. (2008). Explaining interest rate spreads in Ghana. African Development Review, 20(3), 378–399. https://doi.org/10.1111/j.1467-8268.2008.00190.x
- Antwi, S., Atta Mills, E. F. E., & Zhao, X. (2012). Empirical analysis of the performance of the Ghana Stock Exchange and treasury bills. International Journal of Business and Social Science, 3(23), 97–108.
- Bellelah, M. A., Bellelah, M. O., Ben Ameur, H., & Ben Hafsia, R. (2017). Does the equity premium puzzle persist during financial crisis? The case of the French equity market. Research in International Business and Finance, 39(2017), 851–866. https://doi.org/10.1016/j.ribaf.2015.02.018
- Beshears, J., Choi, J. J., Laibson, D., & Madrian, B. C. (2017). Does aggregated returns disclosure increase portfolio risk taking? The Review of Financial Studies, 30(6), 1971–2005. https://doi.org/10.1093/rfs/hhw086
- Bessembinder, H. (2020). Wealth creation in the U.S. public stock markets 1926–2019. SSRN Scholarly Paper ID 3537838 Social Science Research Network, Rochester, NY. 3, 7
- Bessembinder, H. (2018). Do stocks outperform treasury bills? Journal of Financial Economics, 129(3), 440–457. https://doi.org/10.1016/j.jfineco.2018.06.004
- Bessembinder, H. H., Chen, T.-F., Choi, G., & Wei, K.-C J. (2019). Do global stocks outperform US treasury bills? SSRN Electronic Journal, 2, 3. https://doi.org/10.2139/ssrn.3415739
- Boamah, M. I. (2019). Inflation dynamics in a small developing economy: An empirical analysis for Ghana. The Journal of Developing Areas, 53(3), 229–237. https://doi.org/10.1353/jda.2019.0049
- Bonaparte, Y., Kumar, A., & Page J, K. (2017). Political climate, optimism, and investment decisions. Journal of Financial Markets, 34, 69–94. https://doi.org/10.1016/j.finmar.2017.05.002
- Bogoev, J., & Sergi, B. S. (2012). Investigating aggregation bias in the case of the interest rate pass-through, Intereconomics, 47(6), 361–367.
- Bricker, J., Moore, K. B., Thompson, J. P., Bricker, J., Moore, K. B., & Thompson, J. P. (2019). Trends in household portfolio composition. Finance and economics discussion series 2019-069. Board of Governors of the Federal Reserve System.
- Brockmeier, M., & Bektasoglu, B. (2014). Model structure or data aggregation level: Which leads to greater bias of results? Economic Modelling, 38, 238–245. https://doi.org/10.1016/j.econmod.2014.01.003
- Charteris, A., & Winchester, N. (2010). Dairy disaggregation and joint production in an economy-wide model. Australian Journal of Agricultural and Resource Economics, 54(4), 491–507. https://doi.org/10.1111/j.1467-8489.2010.00508.x
- Chopra, N. (2019). Macroeconomic analysis of capital good industry performance: Evidence from Indian stock market. SAMVAD, 17, 1–8.
- Coffie, M. S. (2019). An empirical analysis of the impact of macroeconomic factors on open-end mutual fund prices in Ghana. Ashesi University. http://hdl.handle.net/20.500.11988/501.
- Cremers, K. J. M., Fulkerson, J. A., & Riley, T. B. (2019). Challenging the conventional wisdom on active management: A review of the past 20 years of academic literature on actively managed mutual funds. Financial Analysts Journal, 75(4), 35. https://doi.org/10.2139/ssrn.3247356
- Creswell, J. W. (2014). Research design: Qualitative, quantitative and mixed methods approaches (4th ed.). Sage.
- Di Maggio, M., Kermani, A., & Majlesi, K. (2020). Stock market returns and consumption. The Journal of Finance, 75(6), 3175–3219.
- El Abed, R., & Zardoub, A. (2019). Exploring the nexus between macroeconomic variables and stock market returns in Germany: An ARDL co-integration approach. Theoretical & Applied Economics, 16(2), 139–148.
- Fama, E. F., & Gibbons, M. R. (1981). Inflation, real returns and capital investment. Journal of Monetary Economics, 9(3), 297–323. https://doi.org/10.1016/0304-3932(82)90021-6
- Fama, E. F. (2014). Two pillars of asset pricing. American Economic Review, 104(6), 1467–1485. https://doi.org/10.1257/aer.104.6.1467
- Foroni, C., & Marcellino, M. (2014). Mixed-frequency structural models: Identification, estimation, and policy analysis. Journal of Applied Econometrics, 29(7), 1118–1144. https://doi.org/10.1002/jae.2396
- Frimpong, J. M., & Oteng-Abayie, E. F. (2006). Bounds testing approach: An examination of foreign direct investment, trade, and growth relationships. MPRA Paper No. 352. http://mpra.ub.uni-muenchen.de/352/10.
- Ghana Stock Exchange – Investment & Capital. (2023). https://gse.com.gh/overview/#:∼:text=As%20a%20result%20of%20the%20work%20of%20the,Act%20of%201971%20%28Act%20384%29%20in%20October%201990.
- Greenwood, P. H., & Luloff, A. E. (1979). Inadvertent social theory: Aggregation and its effect son community research. Journal of the Northeastern Agricultural Economics Council, 8(1), 44–47. https://doi.org/10.1017/S0163548400004647
- Gupta, K. L. (1971). Aggregation bias in linear economic models. International Economic Review, 12(2), 293–305. https://doi.org/10.2307/2525689
- Gupta, S., & Sinha, A. K. (2016). Impacts of economic events on performance of mutual funds: Evidence from India. ELK Asia Pacific Journals – Special Issue (2016); ISBN: 978-93-855370-1-1. Available at SSRN: https://ssrn.com/abstract=3285682.
- Gusni, G., Silviana, S. & Hamdani, F. (2018). Factors affecting equity mutual fund performance: evidence from Indonesia. Investment Management and Financial Innovations, 15(1), 1–9. https://doi.org/10.21511/imfi.15(1)
- Gyimah, A. G., Addai, B., & Asamoah, G. K. (2021). Macroeconomic determinants of mutual funds performance in Ghana. Cogent Economics & Finance, 9(1), 1–20. https://doi.org/10.1080/23322039.2021.1913876
- Hilliard, J., & Zhang, H. R. (2015). Size and price-to-book effects: Evidence from the Chinese stock markets. Pacific-Basin Finance Journal, 32(2015), 40–55.
- Jayech, S. (2016). The contagion channels of July–August-2011 stock market crash: A DAG-copula based approach. European Journal of Operational Research, 249(2), 631–646. https://doi.org/10.1016/j.ejor.2015.08.061
- Jordà, Ò., Knoll, K., Kuvshinov, D., Schularick, M., & Taylor, A. M. (2019). The rate of return on everything, 1870–2015. The Quarterly Journal of Economics, 134(3), 1225–1298. https://doi.org/10.1093/qje/qjz012
- Kamal, A. L. M. (2018). The impact of treasury bill rate and interest rate on the stock market returns in Egypt. International Journal of Development and Sustainability, 7(2), 604–619.
- Katz, M., Lustig, H., & Nielsen, L. (2017). Are stocks real assets? Sticky discount rates in stock markets. Review of Financial Studies, 30(2), 539–587. https://doi.org/10.1093/rfs/hhw072
- Kolapo, F. T., Oke, M. O., & Olaniyan, T. O. (2018). Unravelling the Impact of macroeconomic fundamentals on stock market performance in Nigeria: An Ardl-bound testing approach. Journal of Economics, Management and Trade, 21(3), 1–15. https://doi.org/10.9734/JEMT/2018/40177
- Kempf, E., Luo, M., Schäfer, L., & Tsoutsoura, M. (2023). Political ideology and international capital allocation. Journal of Financial Economics, 148(2), 150–173. https://doi.org/10.1016/j.jfineco.2023.02.005
- Kempf, E., & Tsoutsoura, M. (2021). Partisan professionals: Evidence from credit rating analysts. The Journal of Finance, 76(6), 2805–2856. https://doi.org/10.1111/jofi.13083
- Lee, K. C., Pesaran, H., & Pierse, R. G. (1990). Testing for aggregation bias in linear models. The Economic Journal, 100(400), 137–150. https://doi.org/10.2307/2234191
- Liu, J., Stambaugh, R. F., & Yuan, Y. (2019). Size and value in China. Journal of Financial Economics, 134(1), 48–69. https://doi.org/10.1016/j.jfineco.2019.03.008
- Low, R. K. Y., Faff, R., & Aas, K. (2016). Enhancing mean-variance portfolio selection by modelling distributional asymmetries. Journal of Economics and Business, 85(2016), 49–72. https://doi.org/10.1016/j.jeconbus.2016.01.003
- Luloff, A., & Greenwood, P. H. (1980). Definitions of community: An illustration of aggregation bias. Station Bulletin 516, New Hampshire Agricultural Experiment Station, University of New Hampshire.
- Macharia, J. M. (2018). An analysis of the relationship between systematic risk and stakeholder’s return: a case of companies listed on Nairobi Securities Exchange [Doctoral dissertation]. Strathmore University.
- Markowitz, H. (1952). Portfolio selection. The Journal of Finance, 7(1), 77–91. https://doi.org/10.2307/2975974
- Menyah, K., Nazlioglu, S., & Wolde-Rufael, Y. (2014). Financial development, trade openness and economic growth in African countries: New insights from a panel causality approach. Economic Modelling, 37(C), 386–394. https://doi.org/10.1016/j.econmod.2013.11.044
- Mian, A., Sufi, A., & Khoshkhou, N. (2023). Partisan bias, economic expectations, and household spending. The Review of Economics and Statistics, 105(3), 493–510. https://doi.org/10.1162/rest_a_01056.
- Mohamed, I. A., & Ahmed, S. (2018). The effects of macroeconomic variables on stock returns in the Jordanian Stock Market. Global Journal of Management and Business, 5(2), 087–093.
- Nasser, F. A. M., Rosid, C. M. A. C., & Baharuddin, N. S. (2018). Relation between macroeconomic variables and ASEAN stock index. Terengganu International Finance and Economics Journal, 5(1). https://myjms.mohe.gov.my/index.php/tifej/article/view/3170
- Ndlovu, B., Faisa, F., Resatoglu, N. G., & Türsoy, T. (2018). The impact macroeconomic variables on stock returns: A case of the Johannesburg Stock Exchange. Romanian Statistical Review, nr. (2), 86–108.
- Ndubuaku, V. C., Ifeanyi, O., Nze, C., & Onyemere, S. (2017). Impact of monetary policy (interest rate) regimes on the performance of the banking sector in Nigeria. IOSR Journal of Economics and Finance, 08(04), 16–32. https://doi.org/10.9790/5933-0804011632
- Panigrahi, A., Karwa, P., & Joshi, P. (2020). Impact of macroeconomic variables on the performance of mutual funds: a selective study. Journal of Economic Policy and Research, 15(1), 29–43.
- Pesaran, M. H., Pierse, R. G., & Kumar, M. S. (1989). Econometric analysis of aggregation in the context of linear prediction models. Econometrica, 57(4), 861–888. https://doi.org/10.2307/1913775
- Pesaran, B., & Pesaran, M. H. (2010). Time series econometrics using Microfit 5.0: A user’s manual. Oxford University Press, Inc.
- Qureshi, F., Khan, H. H., Ur Rehman, I., Qureshi, S., & Ghafoor, A. (2019). The effect of monetary and fiscal policy on bond mutual funds and stock market: An international comparison. Emerging Markets Finance and Trade, 55(13), 3112–3130. https://doi.org/10.1080/1540496X.2018.1535432
- Rajesh, G. (2019). Relationship between Treasury bill rate and NEPSE index in Nepal. Silver Jubilee issue PRAVAHA, 25(1), 159–168.
- Riley, T. B., & Yan, Q. (2022). Maximum drawdown as predictor of mutual fund performance and flows. Financial Analysts Journal, 78(4), 59–76. https://doi.org/10.1080/0015198X.2022.2100232
- Wade, C. M., Baker, J. S., Latta, G., & Ohrel, S. B. (2019). Evaluating potential sources of aggregation bias with a structural optimization model of the U.S. forest sector. Journal of Forest Economics, 34(3–4), 337–366. https://doi.org/10.1561/112.00000503
- Zhang, Z. (2021). Stock returns and inflation redux: An explanation from monetary policy in advanced and emerging markets. International Monetary Fund Working Paper, European Department, 1–59. https://www.imf.org>wpiea2021219 WP/21/219.
Appendix
Table A1. Rate of inflation nominal interest rate, and real rate of returns, real money value of investment, standard deviation and co-efficient of variation of treasury bills for the period 1990–2020
Table A2. Nominal value of treasury bill and stocks at the end of each period.
Table A3. Rate of inflation nominal returns, real rate of returns, real money value of investment, risk premiums, standard deviation, co-efficient of variation of GSE stock (1990–2020).
Table A4. Real investment value of treasury bill and stocks (1990–2020)
Table A5. Inflation, treasury bill nominal rate of returns, and real rate of returns and real monetary returns of treasury bills for 1990–2000 segregated period.
Table A6. Rate of inflation, GSE all-share index returns (nominal), GSE all-share index real returns, GSE all-share index real money returns, value of money when no investment is made, and risk premium (1990–2000 segregated period)
Table A7. Nominal interest rates, rates of inflation and real rates of treasury bill returns (2001–2010 segregated period)
Table A8. Nominal returns, real rate of returns, rates of inflation for GSE shares in Ghana (2001–2010 segregated period).
Table A9. Investment value at the end of each period (in nominal terms) (2011–2020 segregated period).
Table A10. Inflation, treasury bill rates, real treasury bill return, and cumulative real value of investment (2011–2020 segregated period).
Table A11. Inflation, GSE all-share index returns, real returns, real value, risk premium (2011–2020 segregated period)
Table A12. Companies listed on the Ghana Stock Exchange and their stated capital, date listed, issued shares, and authorised shares.
Table A13. ANOVA analysis period split based on time specific regime ANOVA analysis (i.e. NDC1, NPP1, NDC2, NPP2).
Table A14. Descriptive statistics among the three segregated periods of T’bills returns.
Table A15. Descriptive statistics among the three segregated periods of stock returns stocks.