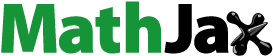
Abstract
Capital inflows, such as foreign aid, can serve as a means to enhance infrastructure development in developing countries. This suggests that foreign aid might have an impact on the level of industrialization in African nations. While existing studies indicate that foreign aid can affect the competitiveness of the manufacturing sector by appreciating the real exchange rate, the veracity of this claim relies on empirical evidence. This paper explores the influence of aid on manufacturing value added using time-series data spanning from 1990 to 2018 for 27 African countries. Employing a panel vector autoregression technique and generating associated impulse response functions, the study scrutinizes the interactions between foreign aid and manufacturing. The analysis is conducted on both the full dataset and subsamples disaggregated based on the income levels of countries. The results indicate that foreign aid acts as a stimulus for manufacturing, primarily through a sustained depreciation of the real exchange rate. This finding holds true for both the overall dataset and the subset of low-income countries. The study attributes this phenomenon to the strategic utilization of aid to enhance infrastructure, leading to a reduction in the price of non-tradables relative to tradables. Consequently, this enhances the profitability and output capacity of the manufacturing sector in African countries. In essence, the results suggest that foreign aid plays a role in influencing or stimulating industrialization in African countries. The study concludes with a discussion on the implications of these findings for industrial policy in African nations.
Impact statement
This paper determines the extent to which foreign aid influences the level of industrialization in African countries. By using time-series data spanning from 1990 to 2018 for 27 African countries, a panel vector autoregression technique and generating associated impulse response functions, the study scrutinizes the interactions between foreign aid and the level of industrialization in African countries. The analysis is conducted on both the full dataset and subsamples disaggregated based on the income levels of countries. Our results negate the general perception that foreign aid inflows harm industrialization in developing countries, which can affect the competitiveness and profitability of manufacturing and lead to deindustrialization. However, this perception has been one-sided because foreign aid can also be used to boost infrastructure in developing countries. The paper helps to unearth the dominant effect of foreign aid in this context and samples African countries because they are generally less developed and industrialized and stand to benefit from foreign aid.
REVIEW EDITOR:
1. Introduction
Africa values foreign aid because it helps the continent achieve its global development objectives. Its impacts on development, however, have been uneven. Data from the Organisation for Economic Cooperation and Development (OECD) show that African countries have been the highest receivers of foreign aid since the Sustainable Development Goals (SDGs) were ratified. For example in 2018, South Africa topped the list of African countries that received the most aid (US$ 4.2 billion), followed by Egypt (US$ 3.5 billion), Mozambique (US$ 3.2 billion), Kenya (US$ 2.4 billion), Ethiopia (US$ 2.3 billion) and Nigeria (US$ 2 billion) (Organisation for Economic Cooperation and Development [OECD], 2021). In addition, some scholars also point out aid’s contribution to achieving SDGs on poverty, hunger and gender inequality and improving access to social services in African countries (Dhahri & Omri, Citation2020; Ndikumana & Pickbourn, Citation2017; Pickbourn & Ndikumana, Citation2016).
Despite its seeming importance to developing countries, foreign aid can have some adverse effects—worsening resource governance, promoting corruption and weakening domestic export competitiveness (Cai et al., Citation2018; Mahembe & Mbaya Odhiambo, Citation2019). These negative effects of aid reduce its effectiveness and make it difficult to determine the effect of aid a priori. Some scholars have further argued that aid causes an appreciation of a economy’s real exchange rate, resulting in symptoms of the Dutch disease, which harms the manufacturing sector in developing countries and affects their export competitiveness (Magud & Sosa, Citation2013). This is an important issue for African countries because even though African countries receive several amounts in foreign aid, the growth and development of their manufacturing sector is crucial to their structural transformation and sustainable growth. In effect, there could be potential in supporting the sector using foreign aid. Hence, this paper’s re-examination of aid’s influence on manufacturing value added or the level of industrialization in African countries.
Typically, a productive sector, like manufacturing, must trade with the rest of the world and especially with developed economies, which usually influence the price of traded final consumption and service goods (generally referred to as tradables). Most African economies are known to be price takers, assuming a fixed world price for final goods and utilizing a relative exchange rate, known as the real exchange rate. This real exchange rate is defined as the ratio of the fixed foreign price of tradables (usually proxied by the price in the United States) to the domestic price of non-tradables (mainly considered to include infrastructure like power supply and the cost of capital investment). In this sense, a depreciation of the real exchange rate results from a decrease in the domestic price of non-tradables, relative to the domestic price of tradables, which increases the profitability of manufacturing. Foreign aid, on the other hand, may cause the real exchange rate to appreciate by increasing the demand/imports for non-tradable goods and services, which raises the price of non-tradables, and reduces the relative profitibality of manufacturing, a situation likened to the Dutch disease syndrome that results from overreliance on revenues from particular natural resources.
Indeed, several studies have examined the effect of aid in developing countries over time (Arndt et al., Citation2010; Boone, Citation1994; Easterly, Citation2003, Citation2006; Herzer et al., Citation2015; Herzer & Morrissey, Citation2009; Lof et al., Citation2015). Nonetheless, a small section of the literature has argued that aid can potentially crowd out manufacturing exports because of the loss of competitiveness caused by the appreciation of the real exchange rate (Addison & Baliamoune-Lutz, Citation2017; Magud & Sosa, Citation2013). This is an empirical matter because foreign aid can also be used to increase the supply of non-tradable and productive infrastructure like power supply, construction and transport. Aid used in this way causes the real exchange rate to depreciate, causes manufacturing exports to become more profitable and accelerates industrial development.
In any case, the non-tradable sector in African countries is underdeveloped and aid inflows can affect both the supply and demand for non-tradables, depending on its use. These different perspectives on aid effectiveness, therefore, give us a basis to empirically examine the effect of aid and the real exchange rate on manufacturing in African countries, which require structural transformation, despite recent growth and large receipts of aid (Akobeng, Citation2020; Rodrik, Citation2018).
Using a panel autoregressive technique, with data on 27 African countries from 1990 to 2018, the paper empirically examines the relationships between foreign aid, the real exchange rate and manufacturing. We analyze these relationships first at an aggregate level and later disaggregate the analysis according to the income levels of countries in the sample. This is the paper’s first contribution to the literature. By focusing on the income levels in our analysis, we control for the difference in development levels of sampled countries, which reflects the reality that the effect of aid is unlikely to be the same across all countries, since some African countries depend on aid for development more than others. The paper’s second contribution to the literature is hinged on the application of impulse response functions in the aid effectiveness literature. These response functions are generated from panel vector autoregression models and show the persistence of the effect of aid and the real exchange rate on manufacturing. To the best of our knowledge, previous studies that examine the effect of foreign aid and the real exchange rate in developing countries do not analyse the persistence of the effects of these variables using impulse response functions, leaving a gap in the literature that this paper contributes to filling.
The results first show that aid does not cause an appreciation of the real exchange rate in African countries. Instead, the results show that foreign aid causes a depreciation of the real exchange rate, which stimulates manufacturing in African countries. Also, the negative and independent effect of aid on manufacturing, is significant for the first few years and insignificant in later years, based on its impulse response function. Thus, this effect of aid does not persist as it is offset by aid’s stimulation of the real exchange rate (real exchange rate depreciation), which then stimulates manufacturing output relative to services. Therefore, the results suggest that foreign aid can influence manufacturing value added or the level of industrialization in African countries. In addition, the results show that the real exchange rate and manufacturing are inversely related. This means that a real exchange rate depreciation stimulates manufacturing in African countries. This finding suggests that competitive real exchange rates can boost manufacturing output in African countries. Also, we find that the effect of the real exchange rate persists over time, suggesting that a competitive real exchange rate is crucial for manufacturing development in African countries. We find similar results from some existing studies (Guzman et al., Citation2018; Rapetti et al., Citation2012; Rodrik, Citation2008) and discuss further the implications of the results for industrial policy in Africa.
The rest of this paper is structured as follows: Section two reviews existing literature on aid, the real exchange rate and manufacturing. Section three discusses the research methodology and section four presents the empirical results. Section five concludes.
2. Literature review
The dominant argument in the literature has been that undervaluation of the real exchange rate is good for growth and industrialization, especially in developing countries (Chukwu & Malikane, Citation2018; Rapetti et al., Citation2012; Rodrik, Citation2008).Footnote1 In fact, not many studies recommend overvaluation of the real exchange rate in developing countries.Footnote2
Several theoretical arguments supporting the undervaluation or depreciation of the real exchange rate have been put forth (Rodrik, Citation2008). The first theoretical argument in favour of undervaluation or depreciation of the real exchange rate is that it is indicative of international competitiveness and helps promote exports from the manufacturing sector. The rapid industrialization success of the East-Asian countries, in which export-led growth policies were pursued in conjunction with an exchange rate regime that supported undervaluation, bears testament to this argument. Indeed, as a result of undervalued exchange rates and positive externalities of the manufacturing sector, savings and investments were boosted by revenues from manufacturing exports in these economies (Dooley et al., Citation2004; Levy-Yeyati & Sturzenegger, Citation2007).
In addition, real exchange rate undervaluation is arguably a compensatory mechanism for the manufacturing sector, despite existing distortions that make it difficult for the sector to receive equilibrium allocation of resources, especially in developing countries (Rapetti et al., Citation2012; Rodrik, Citation2008; Schröder, Citation2013). These distortions are argued to result from bad institutions and market failures that affect the tradable sector, especially in developing countries (Ribeiro et al., Citation2020; Rodrik, Citation2008). Furthermore, scholars argue that real exchange rate undervaluation promotes economic diversification and technology-intensive structural change. This is plausible because a competitive exchange rate acts as a subsidy to the tradable sector that can generate learning spillovers across firms and other related sectors, thus making undervaluation a form of industrial policy (Guzman et al., Citation2018; Rodrik, Citation2008).
The empirical literature mostly shows support for an undervaluation or depreciation, and not an overvaluation or appreciation, of the real exchange rate (Guzman et al., Citation2018; Rapetti et al., Citation2012; Rodrik, Citation2008). However, these studies do not show whether a real exchange rate undervaluation or depreciation driven by aid affects manufacturing in developing countries like this paper does.
Another strand of the literature further argues that foreign aid, among many other capital inflows, leads to an appreciation of the real exchange rate, which then reflect some of the effects of the Dutch disease. This is because aid raises the demand for non-tradables and causes their price to rise. The increase in the price of non-tradables causes an appreciation of the real exchange rate, which reduces the profitability and, hence, amount of output manufacturing can produce at the given world price (Adam & Bevan, Citation2006; Addison & Baliamoune-Lutz, Citation2017; Issa & Ouattara, Citation2008; Li & Rowe, Citation2007; Magud & Sosa, Citation2013; Mongardini & Rayner, Citation2009). Thus, aid-induced Dutch disease harms a recipient country’s manufacturing sector by making its exports less profitable.Footnote3
However, the response of the real exchange rate determines whether or not foreign aid affects the profitability of the manufacturing sector. The real exchange rate is a relative price and can change or be misaligned based on consumer activity in the tradable (consumer goods) and non-tradable (fixed infrastructure) sectors (Magud & Sosa, Citation2013). Based on the way the real exchange rate responds to foreign aid inflows, one of the following effects may result from foreign aid inflows. First, when aid is spent to increase the availability of non-tradables services like education and health, higher wages may draw more skilled labour in these sectors. The wage demands of highly-skilled labour may cause the price of non-tradable services to go up and cause the real exchange rate to appreciate. The appreciation of the real exchange rate reduces the profitability of manufacturing and, because a developing economy is small and open, exports of tradable products decline. This raises the domestic price of non-tradables relative to the domestic price of tradables and affects the productivity of the tradable sector.
Secondly, aid used to invest in infrastructure (roads and ports) and power supply increases the supply of non-tradables and causes their price to fall. In this way, the real exchange rate depreciates and the tradable sector becomes profitable, which is good for growth. Thus when foreign aid is used to improve factor productivity by purchasing productive capital, intermediate goods and foreign consulting, the real exchange rate may depreciate and stimulate manufacturingbecause of the real exchange rate appreciation Again, higher wages paid to labour for non-tradable services leads to higher expenditure on non-tradables. The real exchange rate is likely to be appreciated if foreign aid is spent on imports to satisfy local demand, which the domestic supply sector cannot counter. This means that Therefore, the conclusions of earlier studies that aid is bad for manufacturing in developing countries because of its appreciation of the exchange rate cannot be fully accepted without more empirical investigation. This is because capital inflows, such as aid, are important for some developing countries, especially in Sub-Saharan Africa (Ouedraogo et al., Citation2021).
The dominance of any of the above-mentioned effects is therefore an empirical matter, since the effect of foreign aid cannot be determined beforehand and requires empirical testing. More so, in the case of African countries who receive so much in foreign aid and have not fully industrialized, the empirical test between foreign aid and manufacturing value added or the level of industrialization is crucial to guide industrial policy in the such countries.
3. Methodology
This section begins with a discussion on the vector autoregressive model and presents the model specification. It then presents the estimation technique and descriptive statistics of the sample data.
3.1. VAR & endogeneity concerns
We use a VAR model to determine whether aid causes the Dutch disease in African countries. Diagnostic tests for unit roots, optimal lag length and stability are conducted before estimating the VAR model, in order to ensure that meaningful inferences can be generated from the model. The panel VAR model allows one to examine the interactions between foreign aid, the real exchange rate and the manufacturing sector and determine whether there are reverse causalities between these variables. We also use impulse response functions, which are generated from the VAR model, to determine the dynamics in manufacturing and the persistence of innovations in aid and the real exchange rate.
Other methods, such as fixed effects panel regression analysis, co-integration regression techniques (DFGLS) and difference-in-differences analysis have been used in the literature to analyse the effect of aid (Cai et al., Citation2018; Dupuy et al., Citation2016; Minasyan et al., Citation2017; Nowak-Lehmann et al., Citation2012). Nevertheless, the VAR model is preferred because it can better control for simultaneity by endogenizing all the variables. By using an unrestricted VAR model, interactions between the variables are captured to allow for reverse causality in the model. In this way, it is possible to determine the influence of aid on manufacturing and vice versa, while analysing the interactions between the real exchange rate, aid and manufacturing.
More so, the measure of aid can affect the determination of aid’s effect. Lof et al. (Citation2015) use aid per capita but this is criticized by Herzer et al. (Citation2015), who argue that the use of aid per capita is affected by changes in aid in the numerator and the population in the denominator. As a result, aid, as a share of GDP is a better measure to use in empirical studies (Herzer et al., Citation2015). Therefore, aid’s share of Gross Domestic Product (GDP) is adopted to determine the effect of foreign aid.
Finally, impulse response functions can be derived from the panel VARs. According to Lof et al. (Citation2015), impulse response functions show the dynamic response of variables to innovations in other variables in the system over a given period. Also, we follow Abrigo and Love (Citation2016) because they use confidence intervals that are estimated with Monte Carlo simulations obtained from the distribution of a fitted reduced-form panel VAR model, which improves the reliability of the model estimates.
3.2. Model specification
Following the work of Abrigo and Love (Citation2016) and adapting their estimation codes, a panel VAR model is specified.Footnote4 The panel VAR codes of Abrigo and Love are relied on because they give one the option to control for exogenous variables, use GMM estimators, and conduct causality tests and optimal lag selection tests in line with Andrews and Lu (Citation2001) and Granger (Citation1969).
The panel VAR model examines interactions between the following variables: aid, measured as the share of foreign aid in total output (), manufacturing, measured as manufacturing value added share of total output (
) and the real exchange rate (
), which is measured as the domestic price level relative to the United States (US) price level, so that an increase indicates an appreciation. This RER is used as a proxy for the price of non-tradables relative to tradables and the domestic price level captures the price of non-tradables. An increase in the domestic price represents a rise in the price of non-tradables, which causes the RER to appreciate. In addition, the model controls for fixed capital investment, which is measured as the share of gross fixed capital formation in total output (
), in line with the HD and two-gap models earlier discussed. The variables and their sources are defined fully in the next section.
The causality prediction for the aid-induced Dutch disease is presented as:
(3.1)
(3.1)
Secondly, the causality prediction for the positive effect of AID working though investment is presented as:
(3.2)
(3.2)
Thirdly, the causality prediction for the positive/negative effect of aid operating though other forces, besides the Dutch Disease effect or investment effect is presented as:
(3.3)
(3.3)
To test the causality predictions of EquationEquations (3.1)(3.1)
(3.1) - Equation(3.3)
(3.3)
(3.3) , the following VAR models are specified:
(3.4)
(3.4)
(3.5)
(3.5)
(3.6)
(3.6)
(3.7)
(3.7)
Where
, c, d, e, f are coefficients to be estimated in the VAR models. Also, fixed effects are captured by
and
Furthermore, the stochastic error terms are represented by
and
From EquationEquation (3.4)(3.4)
(3.4) , the null hypothesis that aid does not Granger cause
is tested against the alternative that aid Granger causes
In addition, the null hypothesis that the real exchange rate does not Granger cause
is tested against the alternative that the real exchange rate Granger causes
Furthermore, the null hypothesis that gross fixed capital investment does not Granger cause
is tested against the alternative that gross fixed capital investment Granger causes
Hypotheses capturing reverse causality are also tested. Hence, from EquationEquation (3.5)(3.5)
(3.5) , the null hypothesis that
does not Granger cause aid,
is tested against the alternative that
Granger causes aid,
Again from EquationEquation (3.6)(3.6)
(3.6) , the null hypothesis that
does not Granger cause the real exchange rate,
is tested against the alternative that
Granger causes the real exchange rate,
Similarly, from EquationEquation (3.6)
(3.6)
(3.6) , the null hypothesis that
does not Granger cause the real exchange rate,
is tested against the alternative that
Granger causes the real exchange rate,
Finally from EquationEquation (3.7)(3.7)
(3.7) , the null hypothesis that
does not Granger cause gross fixed capital investment,
is tested against the alternative that
Granger causes gross fixed capital investment,
3.2.1. Estimation technique & specification tests
Consider now the estimation technique and specification tests used for the specified panel VAR model. The system GMM estimator is used to control for endogeneity, in line with Abrigo and Love (Citation2016). This estimation technique—developed by Arellano and Bover (Citation1995)—is used because it allows the variables to be estimated in levels, so that data loss is minimized. Using the Arellano and Bover GMM estimator, together with fixed effects and further specification tests, improves the reliability of the results from the panel VAR model. Anderson and Hsiao (Citation1982) developed an alternative method—the difference GMM technique—which involves the use of first differences. However, this approach can be problematic when the panel used has missing observations or is unbalanced.
Furthermore, the specification tests for the panel VAR model begin with panel unit root tests. The presence of unit roots reduces the efficiency of instruments used in the GMM estimator (Blundell & Bond, Citation1998). Also, moment conditions of the GMM estimator are nullified when unit roots are present (Abrigo & Love, Citation2016). We use both the Levin-Lin-Chu (LLC) and the Harris-Tzavalis (HT) tests, in line with the work of Levin et al. (Citation2002) and Harris and Tzavalis (Citation1999). Both tests use the null hypotheses that there are unit roots in all the panels. Also, the unit root tests, include and exclude the time trends as a robustness check for the results obtained. In addition, the z scores and adjusted t-statistics of the unit root tests are used to reject the null hypothesis of unit roots in the panels.
After unit root testing, the optimal lag length of the VAR model is required. The optimal lag length of the VAR model is determined using Moment Model Selection Criteria (MMSC) based on Hansen’s J statistic of overidentifying restrictions (Andrews & Lu, Citation2001; Hansen, Citation1982). These MMSC are similar to frequently used maximum likelihood-based criteria such as the Akaike, Bayesian and Hannan-Quinn information criteria (Abrigo & Love, Citation2016). The decision criteria, therefore, is to select the lag length that shows the lowest value of the Akaike, Bayesian or Hannan-Quinn MMSCs.
Again, the panel VAR model must be tested for stability. The VAR model is stable when each moduli of the companion matrix is lower than one (Hamilton, Citation1994; Lütkepohl, Citation2005). Stability of the VAR model is important because it proves that the VAR is invertible and its impulse response functions are useful for interpretation. This is an important pre-test to ensure that the VAR model is not misspecified.
In addition, the Granger causality tests are used to determine causality in the model. These tests are performed after the VAR estimates are obtained. Wald tests are used to test the null hypotheses in EquationEquations (3.4)–(3.7). In addition, orthogonalized impulse response functions are derived from the underlying VAR models. These functions show the response of one variable to innovations in another variable in the system. In this way, the effect of the variables on each other can be discussed.
Finally using this methodology, this paper is unique in the following way: a sub-sample analysis is conducted, where countries are grouped by their income levels to see the effect of aid, after the aggregate analysis. We use the World Bank’s income classification of countries over time, as this data is reliable. We anticipate that because low-income countries rely more on foreign aid, the effect of aid may be more noticeable in such countries, than in middle-income countries. In addition, by using impulse response functions generated from the panel VAR model, we are able to examine the dynamics of manufacturing over time and the persistence resulting from shocks in aid and the real exchange rate. Earlier studies examining the aid and the Dutch disease effect do not show the persistence of the effect of aid and the real exchange rate.
3.3. Data & descriptive statistics
3.3.1. Data
The analysis uses a balanced panel dataset, containing data for 27 African countries from 1990 to 2018. The sample size is selected based on data availability. The definitions of all variables in the dataset are shown in .
Table 1. Definition of variables.
Most of the data are obtained from the World Development Indicators (WDI) database of the World Bank,Footnote5 with the exception of data on the real exchange rate, which is obtained from the Penn World Tables (PWT) database version 9.1 (Feenstra et al., Citation2015). In addition, the list of countries sampled in the study are shown in of the Appendix A.
3.3.2. Descriptive statistics
The summary statistics for the main variables in our analysis are presented in . The results from the table show that the average output share of manufacturing value added in African countries sampled is 12% whereas the average share of foreign aid in total output is 6.5%. Also, the results from show that the average real exchange rate (relative price ratio) in African countries sampled is 0.3993 while the average share of gross capital formation in total output (fixed investment) is approximately 21.9%.
Table 2. Summary statistics using full sample.
Looking further at the distribution of manufacturing value added share and the share of foreign aid in total output across countries (see ), the results show that the average share of aid to GDP is higher in low-income countries (10.1%), as compared to lower-middle-income (2.2%) and upper-middle-income countries (1.3%). The difference in the share of foreign aid in GDP across income levels of countries in this sample suggests that the use and effect of foreign aid in African countries are unlikely to be the same.
Table 3. Country income group descriptive statistics.
These statistics align with earlier studies that have pointed out that aid takes on many forms and is used in different ways in developing countries, confirming the existence of a certain level of heterogeneity to foreign aid in developing countries (Addison & Baliamoune-Lutz, Citation2017; Park, Citation2019).
In addition, from , the results show that the average output share of manufacturing value added in low-income countries is quite close to the output share of manufacturing value added in total output in upper-middle-income countries (11.1%), although lower than the output shares of manufacturing value added in lower-middle-income countries (15.5%). The rise and fall in the output shares of manufacturing across the income levels of countries in our sample have also be identified and analyzed in previous studies such as Rodrik (Citation2016) and Tregenna (Citation2016), which acknowledge that manufacturing value added shares in total output have varied across African countries over time resulting from austere macroeconomic policies adopted by earlier African governments.
also shows that the average real exchange rate and capital investment are higher in upper-middle-income countries in our sample. This result suggests that on average, the real exchange rate (0.35) is more undervalued in low-income countries (more depreciated) relative to the 0.48 value in upper-middle income countries. This perhaps reflect the Balassa-Samuelson effect where RER is more undervalued/depreciated in poorer countries to attract foreign capital investments and grants. Previous studies such as Rodrik (Citation2008); Rapetti et al. (Citation2012) and Ribeiro et al. (Citation2020) find similar associations in non-African countries. Similarly, shows that there is relatively more capital investment in upper-middle-income countries as compared to lower-middle- and low-income African countries in the sample.
Correlation coefficient estimates for all variables are also presented in . From the table, it can bee seen that the output shares of foreign aid and manufacturing value added are negatively correlated. The correlation coefficient is statistically significant at the 1% level of significance. Previous studies such as Rajan and Subramanian also find a similar relationship in developing countries.
Table 4. Pairwise correlation estimates.
In addition, shows that there is a negative correlation between the real exchange rate and the output share of manufacturing value added. The correlation coefficient is statistically significant at the 5% level of significance. Previous studies such as Rapetti et al. (Citation2012) and Rodrik (Citation2008) find similar associations in developing countries. also shows a negative correlation between the real exchange rate and the output share of aid in African countries sampled. This correlation is statistically significant at the 1% level of significance. Previous studies such as Rajan and Subramanian and Magud and Sosa (Citation2013) do not find similar associations. Finally, shows that gross capital investment and the output shares of manufacturing and aid are negatively correlated. These correlations are significant at the 10% level of significance.
also shows a positive correlation between gross capital investment and the real exchange rate. This correlation is also statistically significant at the 1% level of significance. Previous studies such as Rajan and Subramanian and Magud and Sosa (Citation2013) find similar associations in non-African countries. Since only shows evidence of association, further using causal analysis is done in the next section in order to generate rigorous results.
4. Results & discussion
This section first presents results from the panel unit root tests. The results from the panel unit root tests are shown in . The LLC and HT tests confirm that the variables have no unit roots in levels and therefore require no differencing. That is, all the variables are integrated of order zero and are I(0). Similar results were obtained after including the time trend in the unit root tests, which confirmed the robustness of the results and suggested that our data would not produce spurious estimates.
Table 5. Panel VAR unit root tests.
After confirming the absence of unit roots, lag selection tests were conducted using pre-determined MMSC. The optimal lag length is important because it determines the structure of the VAR model. Following Abrigo and Love (Citation2016), the optimal lag length that minimizes the selection criteria was determined. Selection criteria include MMSC Bayesian Information Criteria (MBIC), MMSC Akaike Information Criteria (MAIC) and MMSC Hannan and Quinn Information Criteria (MQIC). The results obtained from the lag selection tests (see ) show that the lowest values of these criteria are associated with the first lag. Therefore, the VAR model was estimated using the optimal lag length of one. The choice of lag length is further supported by the coefficient of determination (CD) and the Hansen J statistic, whose large p-value indicates that the over-identifying restrictions are valid and that the model is well specified with one lag.
Table 6. Panel VAR lag selection order criteria.
In addition, the panel VAR model is found to be stable since all moduli are strictly less than one. The stability of the VAR also suggests that the panel VAR is invertible and its vector-order moving average (VMA) has an infinite order—see and in the Appendix A. Stability graphs of VARs from the subsamples are also presented in the Appendix A. Therefore, valid inferences and impulse response functions can be drawn from our VAR model, which is estimated and presented in the next section.
4.1. VAR model estimates
Consider now the causality test results obtained together the panel VAR model estimates. The results presented in show the influence of the variables in the VAR system on each other because of the use Wald p-values from Granger causality tests. The p-values from the Granger causality (Wald) test are reported in parentheses in . Hence, from the first column of , the estimates show that the one period lag of foreign aid as a share of GDP (henceforth, aid) and the real exchange rate (RER) have negative and statistically significant influences on the output share of manufacturing value added. Hence, the null hypotheses that aid and the RER do not Granger cause manufacturing, shown in EquationEquation (3.4)(3.4)
(3.4) , can be rejected.
Table 7. VAR model estimates using full sample.
The independent and negative effect of RER on manufacturing, shown in the first column of , suggests that increases in the RER cause the output share of manufacturing to decrease. This finding is similar to findings of earlier studies such as Rajan and Subramanian and Magud and Sosa (Citation2013). These studies find that the RER negatively influences the output share of manufacturing, even though they argue that the RER is the transmission channel of aid’s effect on manufacturing. Nevertheless, the independent effect of the RER on manufacturing is a strong finding. The results support arguments that depreciation of the RER promotes manufacturing growth. Previous empirical work on RER and growth show that currency depreciation is good for growth because it serves as a compensatory mechanism to redirect resources to the manufacturing sector due to market failures and weak institutions in developing countries (Rapetti et al., Citation2012; Rodrik, Citation2008). Other scholars like Chukwu and Malikane (Citation2018) show evidence of this relationship in African countries and estimate the effect of the RER on the growth of specific sectors.Footnote6
Also, the independent effect of aid on manufacturing value added is negative and statistically significant in the first column of . This finding suggests that increases in aid cause the output share of manufacturing value added to fall in African countries. A similar result is obtained by Rajan and Subramanian and Magud and Sosa (Citation2013). However, these studies suggest that aid’s negative effect is due to an appreciation of the RER.
Further results from show that increases in output share of manufacturing value added and foreign aid cause the RER to depreciate. This finding is enough evidence to reject the null hypothesis, based on the statistical significance of the Wald test statistic and from EquationEquation (3.6)(3.6)
(3.6) , that output share of manufacturing value added and foreign aid do not Granger cause the RER. The result suggests that foreign aid causes a depreciation of the RER in African countries and not an appreciation, as argued by Rajan and Subramanian and Magud and Sosa (Citation2013). As a result, foreign aid increases the competitiveness of the manufacturing sector in African countries and positively influences the level of industrialization in these countries. This is possible when aid is used to boost electricity supply and infrastructure. This increases the supply of non-tradables and causes the price of non-tradables to fall, which results in a depreciation of the RER. Secondly, the results also show causality from RER to manufacturing in African countries. This result suggests that real exchange rate depreciations lead to increases in the output share of manufacturing in African countries. This result is a strong finding, aligning with findings of earlier scholars who also find that the negative relationship between RER and manufacturing is evident in developing countries (Rapetti et al., Citation2012).
Our results are not consistent with the aid-induced Dutch disease hypothesis of previous studies like Rajan and Subramanian; Magud and Sosa (Citation2013). Our results show that the depreciation of the RER caused by aid increases the output share of manufacturing value added. which is more consistent with the literature supporting devaluation or depreciation of the RER (Guzman et al. Citation2018; Rapetti et al. Citation2012). Hence, our results provide evidence against the aid-induced Dutch disease hypothesis in African countries and does not support the argument that the RER is the transmission channel of any aid-induced Dutch disease hypothesis.
To examine the data further, a sub-sample analysis is conducted by grouping countries according their levels of income and estimating the VAR model for each category. Since African countries have different levels of income, the effect of aid is unlikely to be the same. Eventually, three sub-samples are used: low-income, lower-middle and upper-middle-income groups.Footnote7 The VAR estimates for each subsample are presented in .
Table 8. VAR model estimates for low-income groups.
First, the results in show that the negative effect of foreign aid and the RER on manufacturing value added is similar in low-income countries, relative to the full sample results presented earlier. Also, the results from the third column of the table show that foreign aid and manufacturing value added negatively influence or Granger cause the RER in low-income countries. The findings in are therefore similar to the results from . Therefore, these findings suggest that foreign aid can influence manufacturing value added or the level of industrialization by causing a depreciation of the real exchange rate in African countries, especially in low-income countries.
Furthermore, from the first column of , the results show that aid does not have any significant influence on the RER in lower-middle-income African countries. Instead, we find reverse causality, shown by the RER’s negative influence on manufacturing value added and positive influence on foreign aid (see the second column of ). The positive effect of the RER on aid suggests that shifts in the RER influence aid, so that an appreciation of the RER generates a current account deficit that aid fills. In this way, aid provides support to the balance of payment in recipient countries, in line with the two-gap model.
Table 9. VAR model estimates for lower-middle-income groups.
In addition, the results from the third column of show that increases in gross capital investment cause the RER in lower-middle-income African countries to depreciate. This is because more capital investments increase the supply of non-tradables and cause its price to reduce, relative to tradables. This then causes the RER to depreciate. Similar results on the relationship between investment and the RER were presented in the correlation analysis (see ). In addition, the negative influence of the RER on manufacturing value added in lower-middle-income African countries shown in is similar to results for low-income countries.
Also in column three of , it is important to note the result of no causality from aid to the RER in lower-middle-income African countries suggests that aid does not an appreciation of the real exchange rate in such countries. This result is not consistent with findings of earlier studies like Rajan and Subramanian; Magud and Sosa (Citation2013) and Addison and Baliamoune-Lutz (Citation2017). From , causality runs from the RER to foreign aid, which is not indicative of the Dutch disease. Typically, Dutch disease would be present where there is causality from aid to the RER and the relation is positive.
Consider now the results for the VAR model estimated for upper-middle-income countries, which is presented in . The results obtained show that foreign aid does not influence manufacturing value added or the RER in such countries. Previous studies such as Rajan and Subramanian; Magud and Sosa (Citation2013) and Addison and Baliamoune-Lutz (Citation2017) do not find this. The results from rather show causality from the RER and gross capital investment to foreign aid in these countries. Also, the results from show that gross capital investment negatively influences the RER in upper-middle-income African countries. The positive effect of the RER on foreign aid, as well as the negative influence of gross capital investment on the RER is similar to the results obtained for lower-middle-income countries. These results show that aid responds to changes in the RER in lower-middle-income and upper-middle-income countries.
Table 10. VAR model estimates for upper-middle-income groups.
4.2. Impulse response functions
Consider now the impulse response functions generated from the VAR models. Impulse response functions show the responses of selected variables over time when there are innovations or impulses in particular variables in the VAR system. This analysis in this section uses the impulse response functions to show the response of system variables to impulses in foreign aid and the RER. An impulse response function is significant when the horizontal zero line falls outside its confidence bands (Abrigo & Love, Citation2016).
First consider the impulse response function showing the response of manufacturing value added to impulses in foreign aid, using data from the full sample in this paper. From , the effect of aid on manufacturing value added is negative and statistically significant for the first few years (three to five), after which the effect becomes statistically insignificant. This impulse response function implies that aid’s independent effect on manufacturing, although negative, is marginal and not persistent.
Additional impulse response functions are generated, showing aid’s effect on manufacturing for each sub-sample. These are presented in . The results show that the independent effect of foreign aid on manufacturing is significant for the first few years and then fizzles out in low-income African countries. However, for lower-middle-income and upper-middle-income African countries, the results show that the effect of foreign aid on manufacturing is insignificant.
Therefore, the results from mean that aid has a negative, statistically significant and short-term influence on manufacturing in low-income countries, while in lower-middle and upper-middle income countries, aid shows no statistically significant effect on manufacturing.
Earlier results from the VAR system also show that aid has a negative influence on manufacturing, especially in low-income countries. Hence, from the VAR system and the impulse response functions, the negative effect of foreign aid is possible when foreign aid is increasingly used to expand non-tradable services, at the expense of the tradable sector. Thus, more aid expenditure on services increases the contribution of the services’ sector to GDP, relative to manufacturing. As a result, the share of manufacturing in total output decreases. Also, from and , the negative effect of foreign aid on manufacturing is not persistent. It is possible that the non-persistent influence of foreign aid on manufacturing may be due to influences of other variables like the RER—see next section on the influence of the real exchange rate.
Previous studies such as Rajan and Subramanian and Magud and Sosa (Citation2013) also find similar results. However, these studies do not examine the persistence of aid’s effect on manufacturing, as we do in this paper. Rajan and Subramanian give the impression that the negative effect is important, but without explicitly testing the persistence. But from this analysis, it is shown that it is important to qualify the negative effect; whether it is persistent or not. Thus, by using impulse response functions and determining the persistence of aid’s effect, a contribution is made to the literature on aid-induced Dutch disease in developing countries.
4.3. The influence of the real exchange rate
The impulse response functions showing the response of manufacturing to innovations or shocks from the real exchange rate are now examined in this section. Using the full sample, the impulse response function presented in shows that, at 5% level of significance, the RER has a negative and significant influence on manufacturing in African countries sampled. This is because the zero line falls outside the confidence intervals shown in . This finding suggests that innovations in the RER negatively and significantly influence manufacturing in the first few years and persists well into the tenth year. This means that an appreciation of the real exchange rate reduces the share of manufacturing value added in GDP. The independent effect of the RER on manufacturing in this paper is in line with the findings of earlier studies like Rajan and Subramanian, even though their paper argues that the RER is the transmission channel for aid’s effect on manufacturing. This major finding is also in line with the results obtained from the VAR model, presented in . Furthermore, it supports arguments in the literature on undervaluation and manufacturing promotion in developing countries (Rapetti et al., Citation2012; Rodrik Citation2008; Guzman et al. Citation2018; Missio et al. Citation2015; Schröder, Citation2013).
We further examine the response of manufacturing to innovations in the RER by analysing impulse response functions according to the income levels of countries in our dataset. These are presented in . The results from this sub-sample analysis show that the RER negatively influences manufacturing and this effect persists into the sixth year in low-income countries. After the sixth year, innovations in the RER have no significant influence on manufacturing in low-income countries. However, for lower-middle income and upper-middle-income countries, impulse response functions showing manufacturing’s response to innovations in the RER are insignificant. This is because the zero line passes through the confidence bands shown in the impluse response functions in .
4.4. Impulse responses from aid to RER
Finally, impulse response functions that show the response of the real exchange rate to impulses in aid are considered. From the VAR estimates presented earlier, we find that aid negatively influences the RER in African countries. This is also the case in low-income countries in our sample. We argue that this negative relationship is not consistent with the hypothesis of aid-induced Dutch disease, in which the RER would respond positively to aid inflows.
We find that the impulse response function showing the response of the RER to aid confirms the negative influence of aid on the RER, as seen in the VAR model. This impulse response function, shown in , indicates that aid’s influence on the RER does not persist over time. From , the negative influence of aid on the RER is statistically significant for the first four years, after which it becomes insignificant, because the zero line falls within the confidence bands of the impulse response function. Our finding differ from results of earlier studies like Rajan and Subramanian and Magud and Sosa (Citation2013), who argue that aid causes the Dutch disease because it has a positive influence on the RER.
Additional impulse response functions obtained from the subsample analysis also show that the effect of foreign aid on the RER is negative in low-income countries, albeit insignificant. Also for lower-middle-income and upper-middle-income countries, the impulse response functions obtained were insignificant.
5. Conclusion
The primary goal of this paper is to investigate the impact of foreign aid on the level of industrialization in African countries. Given the substantial significance of foreign aid to African economies, which are still in the process of catching up with the developed world, the study delves into the debate surrounding the growth effects of aid. While some scholars emphasize the positive role of aid in supporting infrastructure and manufacturing, others express skepticism due to the potential appreciation of the real exchange rate, reminiscent of the Dutch disease syndrome. The focus of this paper is to assess the influence of aid on manufacturing value added and the overall level of industrialization in African nations.
The paper employs a panel VAR estimation technique to model the dynamic interactions among manufacturing, aid, and the real exchange rate, while accounting for fixed investment in alignment with established aid theories. Two key contributions are made to the existing literature. Firstly, the study analyzes the impact of aid and the real exchange rate based on the income levels of the sampled countries, recognizing the variability of aid effects across different economic contexts. Secondly, it utilizes impulse response functions to gauge the persistence of shocks from aid and the real exchange rate on manufacturing in African countries. Through this approach, the paper empirically contributes to the aid effectiveness literature, offering insights not commonly explored in earlier studies examining aid-induced Dutch disease, particularly in developing countries.
In contrast to the conventional expectation that foreign aid inflows in developing economies lead to a real exchange rate appreciation, our findings do not align with this pattern. The outcomes derived from the VAR and impulse response functions indicate a contrary effect, wherein aid is associated with a real exchange rate depreciation. Remarkably, this depreciation, in turn, acts as a stimulus for manufacturing in African countries. As a result, our conclusion is that foreign aid can indeed exert an influence on the level of industrialization in African nations.
Our findings also show that the independent effect of foreign aid on manufacturing is negative. However, this effect is short-lived—it lingers for the first few years, after which it becomes insignificant. Previous studies such as Rajan and Subramanian and Magud and Sosa (Citation2013) find a similar result, even though they do not show any evidence of persistence as we do. We argue that it such short-term effects that convince other scholars that foreign aid negatively affects the growth. We suggest that there is merit in looking at the persistence of the aid’s effect on manufacturing in the long-term because of the lead time involved in establishing industries and manufacturing.
Also, we find that the real exchange rate negatively influences manufacturing in African countries. This means that a real exchange rate depreciation stimulates the output share of manufacturing in African countries. This result is in line with findings of earlier studies such as (Rapetti et al. Citation2012; Rodrik Citation2008; Guzman et al. Citation2018; and Missio et al. Citation2015). The effect of the real exchange rate on manufacturing also persists, all the way into the tenth year. This is an additional contribution to the literature as earlier studies do not look at persistence as we do in this paper.
There are potential policy implications arising from the obtained results. Policymakers in Africa might contemplate utilizing foreign aid to enhance the provision of non-tradable goods, such as power supply and infrastructure. This approach could effectively lower the cost of non-tradables relative to tradables, stimulate the exchange rate (resulting in real exchange rate depreciation), and render manufacturing more economically viable. The resultant stimulation of the real exchange rate could potentially foster growth in the manufacturing sector across African countries.
However, the utilization of foreign aid in this manner requires a cautious approach, aligning with each country’s existing industrial and trade policies. This ensures that each nation secures a competitive advantage in production, creating and sustaining employment in their productive sectors. By adopting such a strategy, donors can be assured of obtaining value for their funds, potentially motivating them to continue directing financial support to developing countries in Africa, independent of other contextual factors influencing foreign aid allocations.
Although Africa is recognized for its leadership in resource-based exports, it has not yet established itself as a hub for product-based exports due to the relatively high production costs faced by local entrepreneurs and manufacturers. Real exchange rate depreciations present an opportunity for economies to gain a competitive advantage, a strategy successfully employed by current global economic leaders. Encouraging real exchange depreciation can incentivize producers to boost output and exports. This strategy could be considered for regional and continental trade regimes in Africa, aiming to enhance productivity and output over time. Nevertheless, future research on aid and industrialization might explore the effects of aid at the sectoral level using input-output analysis, providing additional insights into the impact of aid and the real exchange rate on various sectors in African economies.
Aid_and_industrialization_paper_RKA_Nell.rar
Download (2.3 MB)Acknowledgments
The author expresses their gratitude to Professor Fiona Tregenna for her valuable reviews that have improved the quality of this paper.
Disclosure statement
No potential conflict of interest was reported by the author(s).
Data availability statement
Data is available upon request to the corresponding author.
Additional information
Notes on contributors
Rex Kweku Awuku Asiama
Dr Rex Kweku Awuku Asiama is an applied economist specializing in industrial policy in Africa, development economics and development studies.
Kevin Nell
Professor Kevin Nell is a professor of Economics, specializing in macroeconomics, with specific reference to developing countries.
Notes
1 The downside is that undervaluation of the exchange rate results in domestic inflation in small open economies who are mostly dependent on imports of final goods and services (Ribeiro et al., Citation2020).
2 See for instance Oreiro and D’Agostini (Citation2017); Cottani et al. (Citation1990); Ghura and Grennes (Citation1993).
3 The spending and resource movement effects, which arise from aid inflows into an economy, are fundamental to the theory of aid-induced Dutch disease (Rajan & Subramanian)
4 Abrigo and Love’s approach is an update of the methodology developed by Love and Zicchino (Citation2006), which investigates the relationship between firm financial conditions and investment in 36 countries for the period 1988–1998.
5 Although openly available, we find that current checks to update the dataset proved futile due to missing data. We provide a data availability statement at the end of the paper regarding access to the dataset used in this paper, as we have no control over updates to the WDI database.
6 Other scholars have argued against the benefits of undervaluation or depreciation of the RER for manufacturing and growth (Ribeiro et al., Citation2020).
7 The high-income category is omitted in this analysis due to insufficient country observations.
References
- Abrigo, M. R., & Love, I. (2016). Estimation of panel vector autoregression in Stata. The Stata Journal: Promoting Communications on Statistics and Stata, 16(3), 778–804. https://doi.org/10.1177/1536867X1601600314
- Adam, C. S., & Bevan, D. L. (2006). Aid and the supply side: Public investment, export performance, and Dutch disease in low-income countries. The World Bank Economic Review, 20(2), 261–290. https://doi.org/10.1093/wber/lhj011
- Addison, T., & Baliamoune-Lutz, M. (2017). Aid, the real exchange rate and why policy matters: The cases of Morocco and Tunisia. The Journal of Development Studies, 53(7), 1104–1121. https://doi.org/10.1080/00220388.2017.1303673
- Akobeng, E. (2020). Harnessing foreign aid for the poor: Role of institutional democracy. Journal of Economic Studies, 47(7), 1689–1710. https://doi.org/10.1108/JES-05-2019-0225
- Alves, J., Couto, C., & Coelho, J. C. (2023). The impact of foreign aid on aggregate welfare measures: a panel data analysis. Journal of the Knowledge Economy, 1–21. https://doi.org/10.1007/s13132-023-01119-w
- Anderson, T. W., & Hsiao, C. (1982). Formulation and estimation of dynamic models using panel data. Journal of Econometrics, 18(1), 47–82. https://doi.org/10.1016/0304-4076(82)90095-1
- Andrews, D. W., & Lu, B. (2001). Consistent model and moment selection procedures for GMM estimation with application to dynamic panel data models. Journal of Econometrics, 101(1), 123–164. https://doi.org/10.1016/S0304-4076(00)00077-4
- Arellano, M., & Bover, O. (1995). Another look at the instrumental variable estimation of error-components models. Journal of Econometrics, 68(1), 29–51. https://doi.org/10.1016/0304-4076(94)01642-D
- Arndt, C., Jones, S., & Tarp, F. (2010). Aid, growth, and development: Have we come full circle? Journal of Globalization and Development, 1(2), 1–27. https://doi.org/10.2202/1948-1837.1121
- Blundell, R., & Bond, S. (1998). Initial conditions and moment restrictions in dynamic panel data models. Journal of Econometrics, 87(1), 115–143. https://doi.org/10.1016/S0304-4076(98)00009-8
- Boone, P. (1994). The impact of foreign aid on savings and growth. Centre for Economic Performance.
- Cai, J., Zheng, Z., Hu, R., Pray, C. E., & Shao, Q. (2018). Has international aid promoted economic growth in Africa? African Development Review, 30(3), 239–251. https://doi.org/10.1111/1467-8268.12333
- Chukwu, A. B., & Malikane, C. (2018). Real exchange rate (RER) as a policy tool for industrial diversification and growth in Africa. The Journal of International Trade & Economic Development, 27(5), 504–520. https://doi.org/10.1080/09638199.2017.1397729
- Cottani, J. A., Cavallo, D. F., & Khan, M. S. (1990). Real exchange rate behavior and economic performance in LDCs. Economic Development and Cultural Change, 39(1), 61–76. https://doi.org/10.1086/451853
- Dhahri, S., & Omri, A. (2020). Foreign capital towards SDGs 1 & 2–Ending Poverty and hunger: The role of agricultural production. Structural Change and Economic Dynamics, 53, 208–221. https://doi.org/10.1016/j.strueco.2020.02.004
- Dooley, M. P., Folkerts-Landau, D., & Garber, P. (2004). The revived Bretton woods system. International Journal of Finance & Economics, 9(4), 307–313. https://doi.org/10.1002/ijfe.250
- Dupuy, K., Ron, J., & Prakash, A. (2016). Hands off my regime! Governments’ restrictions on foreign aid to non-governmental organizations in poor and middle-income countries. World Development, 84, 299–311. https://doi.org/10.1016/j.worlddev.2016.02.001
- Easterly, W. (2003). Can foreign aid buy growth? Journal of Economic Perspectives, 17(3), 23–48. https://doi.org/10.1257/089533003769204344
- Easterly, W. (2006). The white man’s burden: Why the West’s efforts to aid the rest have done so much ill and so little good. Penguin Press.
- Feenstra, R. C., Inklaar, R., & Timmer, M. P. (2015). The next generation of the Penn World Table. American Economic Review, 105(10), 3150–3182. https://doi.org/10.1257/aer.20130954
- Ghura, D., & Grennes, T. J. (1993). The real exchange rate and macroeconomic performance in Sub-Saharan Africa. Journal of Development Economics, 42(1), 155–174. https://doi.org/10.1016/0304-3878(93)90077-Z
- Granger, C. W. (1969). Investigating causal relations by econometric models and cross-spectral methods. Econometrica: Journal of the Econometric Society, 37(3), 424–438. https://doi.org/10.2307/1912791
- Guzman, M., Ocampo, J. A., & Stiglitz, J. E. (2018). Real exchange rate policies for economic development. World Development, 110, 51–62. https://doi.org/10.1016/j.worlddev.2018.05.017
- Hamilton, J. (1994). Time series analysis. Princeton University Press.
- Hansen, L. P. (1982). Large sample properties of generalized method of moments estimators. Econometrica: Journal of the Econometric Society, 50(4), 1029–1054. https://doi.org/10.2307/1912775
- Harris, R. D., & Tzavalis, E. (1999). Inference for unit roots in dynamic panels where the time dimension is fixed. Journal of Econometrics, 91(2), 201–226. https://doi.org/10.1016/S0304-4076(98)00076-1
- Herzer, D., & Morrissey, O. (2009). The long-run effect of aid on domestic output. CREDIT Research Paper. University of Nottingham.
- Herzer, D., Nowak-Lehmann, F., Dreher, A., Klasen, S., & Martinez-Zarzoso, I. (2015). Comment on Lof, Mekasha, and Tarp (2014). World Development, 70, 389–396. https://doi.org/10.1016/j.worlddev.2014.06.012
- Issa, H., & Ouattara, B. (2008). Foreign aid flows and real exchange rate: Evidence from Syria. Journal of Economic Development, 33(1), 133–146.
- Levin, A., Lin, C.-F., & Chu, C.-S J. (2002). Unit root tests in panel data: Asymptotic and finite-sample properties. Journal of Econometrics, 108(1), 1–24. https://doi.org/10.1016/S0304-4076(01)00098-7
- Levy-Yeyati, E., & Sturzenegger, F. (2007). Fear of floating in reverse: Exchange rate policy in the 2000s. In LAMES-LACEA Annual Meetings (pp. 8–22). Citeseer.
- Li, Y., & Rowe, F. (2007). Aid inflows and the real effective exchange rate in Tanzania. Policy Research Working Paper 4456. The World Bank Open Knowledge Repository.
- Lof, M., Mekasha, T. J., & Tarp, F. (2015). Aid and income: Another time-series perspective. World Development, 69, 19–30. https://doi.org/10.1016/j.worlddev.2013.12.015
- Love, I., & Zicchino, L. (2006). Financial development and dynamic investment behavior: Evidence from panel VAR. The Quarterly Review of Economics and Finance, 46(2), 190–210. https://doi.org/10.1016/j.qref.2005.11.007
- Lütkepohl, H. (2005). New introduction to multiple time series analysis. Springer-Verlag.
- Magud, N., & Sosa, S. (2013). When and why worry about real exchange rate appreciation? The missing link between Dutch disease and growth. Journal of International Commerce, Economics and Policy, 04(02), 1350009. https://doi.org/10.1142/S1793993313500099
- Mahembe, E., & Mbaya Odhiambo, N. (2019). Foreign aid, poverty and economic growth in developing countries: A dynamic panel data causality analysis. Cogent Economics & Finance, 7(1), 1626321. https://doi.org/10.1080/23322039.2019.1626321
- Minasyan, A., Nunnenkamp, P., & Richert, K. (2017). Does aid effectiveness depend on the quality of donors? World Development, 100, 16–30. https://doi.org/10.1016/j.worlddev.2017.07.023
- Missio, F. J., Jayme, F. G., Jr., Britto, G., & Luis Oreiro, J. (2015). Real exchange rate and economic growth: New empirical evidence. Metroeconomica, 66(4), 686–714. https://doi.org/10.1111/meca.12087
- Mongardini, M. J., & Rayner, B. (2009). Grants, remittances, and the equilibrium real exchange rate in Sub-Saharan African countries. IMF Working Papers no. 075. International Monetary Fund. https://doi.org/10.5089/9781451872224.001
- Ndikumana, L., & Pickbourn, L. (2017). The impact of foreign aid allocation on access to social services in sub-Saharan Africa: The case of water and sanitation. World Development, 90, 104–114. https://doi.org/10.1016/j.worlddev.2016.09.001
- Nowak-Lehmann, F., Dreher, A., Herzer, D., Klasen, S., & Martínez-Zarzoso, I. (2012). Does foreign aid really raise per capita income? A time series perspective. Canadian Journal of Economics/Revue canadienne d'économique, 45(1), 288–313. https://doi.org/10.1111/j.1540-5982.2011.01696.x
- Organisation for Economic Co-operation and Development (OECD). (2021). Detailed aid statistics: Total receipts (Edition 2020). OECD International Development Statistics, OECD ilibrary.
- Oreiro, J. L., & D’Agostini, L. (2017). Macroeconomic policy regimes, real exchange rate overvaluation, and performance of the Brazilian economy (2003–2015). Journal of Post Keynesian Economics, 40(1), 27–42. https://doi.org/10.1080/01603477.2016.1273070
- Ouedraogo, R., Sourouema, W. S., & Sawadogo, H. (2021). Aid, growth, and institutions in sub-saharan africa: New insights using a multiple growth regime approach. The World Economy, 44(1), 107–142. https://doi.org/10.1111/twec.12968
- Park, J.-D. (2019). Assessing the role of foreign aid, donors and recipients. In Re-inventing Africa’s development (pp. 37–60). Palgrave Macmillan.
- Pickbourn, L., & Ndikumana, L. (2016). The impact of the sectoral allocation of foreign aid on gender inequality. Journal of International Development, 28(3), 396–411. https://doi.org/10.1002/jid.3213
- Rajan, R., & Subramanian, A. (2007). Does aid affect governance? American Economic Review, 97(2), 322–327. https://doi.org/10.1257/aer.97.2.322
- Rapetti, M., Skott, P., & Razmi, A. (2012). The real exchange rate and economic growth: Are developing countries different? International Review of Applied Economics, 26(6), 735–753. https://doi.org/10.1080/02692171.2012.686483
- Ribeiro, R. S., McCombie, J. S., & Lima, G. T. (2020). Does real exchange rate undervaluation really promote economic growth? Structural Change and Economic Dynamics, 52, 408–417. https://doi.org/10.1016/j.strueco.2019.02.005
- Rodrik, D. (2008). The real exchange rate and economic growth. Brookings Papers on Economic Activity, 2008(2), 365–412. https://doi.org/10.1353/eca.0.0020
- Rodrik, D. (2016). Premature deindustrialization. Journal of Economic Growth, 21(1), 1–33. https://doi.org/10.1007/s10887-015-9122-3
- Rodrik, D. (2018). An African growth miracle? Journal of African Economies, 27(1), 10–27.
- Schröder, M. (2013). Should developing countries undervalue their currencies? Journal of Development Economics, 105, 140–151. https://doi.org/10.1016/j.jdeveco.2013.07.015
- Tregenna, F. (2016). Deindustrialization and premature deindustrialization: Chapter 38. In Handbook of alternative theories of economic development (pp. 710–728). Edward Elgar Publishing.
- Xu, Z., Zhang, Y., & Li, D. (2023). Chinese aid and nutrition improvement in Sub-Saharan Africa. Applied Economics, 1–19. https://doi.org/10.1080/00036846.2023.2204216
Appendix A.
Income classifications of sampled countries
Table 11. Income classifications of sampled countries.
Table 12. Panel VAR stability stats.