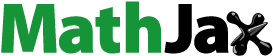
Abstract
Understanding the relationship between health capital and economic performance is crucial for policymakers in Southern African Development Community (SADC) countries. This study explores this relationship in 14 SADC countries over a 14-year period (2005-2019), with GDP per capita as a measure of economic performance and health capital’s impact analysed alongside labour force participation rate, institutional quality, and trade openness. To address potential outliers, the data was transformed using logarithms, and two panel unit root tests, Levin, Lien, and Chu (LLC), and Im, Pesaran, and Shin (IPS), were employed to test for stationarity of the series. The findings indicate a combination of integrated orders, including I(0) and I (1), but not I (2). Panel cointegration tests by Pedroni and Kao reveal cointegration, suggesting a long-run relationship between the variables. The study utilized the panel auto regressive distributed lag (ARDL) model with Pooled Mean Group analysis as the best estimator to investigate both long run and short run relationships. For robustness, the study employed the fully modified least squares (FMOLS) method and the fixed effects (FE) model. The empirical findings establish a significant and negative relationship between health capital and economic performance in the selected SADC countries. Based on these results, the study recommends that governments in the SADC region prioritize addressing issues related to leakages in the health sector to improve economic performance.
Reviewing Editor:
1. Introduction and background
The link between health capital and economic performance is of paramount importance for policymakers in the Southern African Development Community (SADC) countries. Health capital, representing the overall health and well-being of a population, plays a vital role in shaping a nation’s productivity and economic growth (Bloom et al., Citation2004; Raghupathi & Raghupathi, Citation2020; Ridhwan et al., Citation2022). Understanding this relationship is particularly pertinent in the context of SADC countries, where healthcare systems face challenges, and sustainable economic development remains a top priority.
The interplay between health capital and economic performance has garnered significant attention in global research (Achoki et al., Citation2022; Raghupathi & Raghupathi, Citation2020). A healthy workforce is more productive, and reduced healthcare expenses contribute to economic prosperity. The significance and conceptualization of health capital in a nation have been highlighted in studies conducted by Schneider-Kamp (Citation2021), Xu and Jiang (Citation2020) which emphasise the importance of health capital, which represents the overall health and well-being of the population, in shaping a nation’s development and economic performance. The studies contribute to the understanding of how a healthy population can lead to increased productivity, reduced healthcare costs, and ultimately foster economic growth. By acknowledging the role of health capital in national development, policymakers can devise effective strategies to enhance healthcare systems and promote sustainable economic progress.
Within the SADC countries, the specific implications of health capital on economic performance remain underexplored, and the available studies mostly focus on individual country analyses, overlooking the comprehensive regional perspective (Ndaguba & Hlotywa, Citation2021). Moreover, despite improvements in economic performance, healthcare systems in the SADC countries continue to lag behind other regions, indicating the need for targeted research to address the unique challenges and opportunities in this context (Achoki et al., Citation2022). To address this gap, this study employs a panel data regression approach to investigate the relationship between health capital and economic performance in 14 selected SADC countries over a 14-year period (2005–2019). By utilizing advanced methodologies, such as panel auto regressive distributed lags (ARDL) and robust techniques (Affoh et al., Citation2022), this research aims to provide valuable insights that can inform policymakers and foster regional development.
Health capital is regarded as one of the leading indicators of economic performance, attracting the attention of a wide range of stakeholders, including scholars and policymakers in a variety of nations (World Health Organisation (WHO), Citation2017). According to Aboubacar and Xu (Citation2017), one of the most crucial factors in determining a country’s economic success is the amount of money spent on health care. This is because efficient health capital results in improved health outcomes (Piabuo & Tieguhong, Citation2017; Yazdi & Khanalizadeh, Citation2017). Thus, investing in health improves the physical and psychological health of the population, especially the workforce, which is predicted to result in improved health outcomes, which translates into improved human capital and, consequently, increased productivity for the economy (Chandra Das & Chatterjee, Citation2020). Therefore, efficient health expenditure is linked to health outcomes and consequently economic performance.
Previous studies have found a correlation between health capital and economic performance (Arthur & Hassan, Citation2017; Mehrara & Musai, Citation2011; Ndaguba & Hlotywa, Citation2021; Piabuo & Tieguhong, Citation2017). However, establishing the efficacy of health capital in Southern African Development Community (SADC) remains challenging owing to the fact that the majority of African countries, especially those classified as low- and middle-income, severely lack the resources necessary to deliver better health care (Piabuo & Tieguhong, Citation2017). This issue may be a consequence of developing countries’ lower public spending on health in comparison to industrialised countries, which results in poor management practices in the health sector, a lack of medical facilities, and low family incomes (Aboubacar & Xu, Citation2017). This is an alarming trend, since enough and effective health capital is required to enhance health care outcomes, which in turn results in better economic performance across countries.
Few studies, like Hasanshahi (Citation2017), Davlasheridze et al. (Citation2018), and Erçelik (Citation2018), looked at the relationship between health capital and economic performance and came to the conclusion that there is a positive and significant relationship between economic performance and health capital. On the other hand, Khan et al. (Citation2016) and Edeme and Olisakwe (Citation2019) claim that there is a negative relationship between health capital and economic performance, although other researchers established no relationship at all between health capital and economic performance (Khembo & Tchereni, Citation2013). In light of the fact that the relationship between health capital and economic performance is controversial across the globe and has not received a great deal of attention, the purpose of this study was to investigate the relationship between health capital and economic performance in selected SADC countries.
Extensive study has been conducted, including some that was carried out in countries outside of Africa, on the subject of the relationship that exists between health capital and economic performance (Zhao et al., Citation2019; Li et al., Citation2017; Raghupathi & Raghupathi, Citation2020). However, it was noticed that throughout the search, only a few studies (Piabuo & Tieguhong, Citation2017; Yazdi & Khanalizadeh, Citation2017; Zaidi & Saidi, Citation2018) have looked at this issue in the Southern African Development Community. Piabuo and Tieguhong (Citation2017), Yazdi and Khanalizadeh (Citation2017), and Zaidi and Saidi (Citation2018) are three examples of these studies (SADC) ().
As far as this study is aware, none of the studies conducted in the SADC adjusted for the quality of the institutions, which is a variable that is very relevant. Due to the fact that many African nations struggle with weak institutions, there is a pressing need to regulate for institutions. This investigation made use of the panel cointegration technique because, in comparison to other methods, it offers a number of benefits. One of these benefits is the fact that it does not call for the variables to be cointegrated in the conventional sense of being a linear combination of stationary variables. Additionally, the method is reliable because the common unit root components, which in a setting that was more typical, would cause a disruption in the cointegration connection between the dependent variables and the explanatory variables, have been omitted (Pedroni, Citation2018). The enhancement and improvement of econometric models for health capital and economic performance in selected Southern African Developing Countries is the goal of this effort.
This research fills a significant gap in the existing body of knowledge on the topic of health capital and economic performance in the Southern African Development Community (SADC) countries. It contributes by providing empirical evidence on the relationship between health capital and economic performance using a panel data regression approach, which includes 14 selected SADC nations. This approach allows for a comprehensive analysis covering multiple countries, which is more robust than single-country studies. Additionally, the study makes use of panel auto regressive distributed lags (ARDL) methodology, which addresses issues of group analytics and heteroscedasticity. By employing two robust techniques in the panel ARDL analysis, the study overcomes different problems faced with this method, enhancing the reliability of the findings. The significance of this study lies in its potential impact on policymakers and governing bodies. It sheds light on the relationship between health capital and economic performance in the SADC, enabling policymakers to design more effective health financing systems and better understand the drivers of economic growth in the region. The research’s fourfold contribution includes addressing a theoretical gap with limited studies on this topic in the SADC region during the study period (2005–2019). Additionally, the inclusion of institutional quality as a driver of economic performance is a valuable addition missing in previous literature. Moreover, the study’s methodological contribution lies in the use of panel data analysis, incorporating multiple countries to offer a more comprehensive perspective, and applying panel ARDL to examine both short-run and long run impacts of health spending on economic performance. In conclusion, this research significantly contributes to the understanding of health capital and economic performance in the SADC region by providing empirical evidence, methodological advancements, and insights that can guide policymakers in enhancing both healthcare systems and economic growth strategies. By tackling data scarcity and addressing theoretical gaps, this study paves the way for future research to build upon these findings, fostering regional development and progress.
2. Literature review
In this section, both theoretical and empirical literature on the relationship between health expenditures and economic performance are assessed and discussed. The literature is divided into two categories: theoretical and empirical. The first portion deals with theoretical literature, while the subsequent section covers empirical literature.
2.1. Theoretical literature
The theoretical literature on health capital and economic performance encompasses various models and mechanisms that attempt to explain the relationship between the two. Grossman’s theory (Grossman, Citation1999) posits that money spent on health is an investment in human capital, contributing to sustained medium- and long-term economic performance. It emphasizes that health goes beyond a long life and encompasses a life free of ailments, benefiting both individuals and the economy (Demirci et al., Citation2018; Yıldırım et al., Citation2020). Furthermore, endogenous growth theories proposed by Romer (Citation1986), Lucas (Citation1988), and Rebelo (Citation1991) emphasize the importance of human capital formation and the health care system in improving economic performance (Halıcı-Tülüce et al., Citation2016; Piabuo & Tieguhong, Citation2017).
One such endogenous growth model, developed by Romer (Citation1986), highlights the significance of human capital in enhancing productivity. Similarly, Lucas (Citation1988) and Rebelo (Citation1991) acknowledge the role of health care in determining workers’ productivity. However, while these theories suggest a positive correlation between health expenditure and economic performance, it is essential to approach these findings critically. Some scholars argue that a comprehensive understanding of the complex relationship between health capital and economic performance requires accounting for other factors such as the quality of health care services and the efficiency of health spending (Demirci et al., Citation2018).
The Total Factor Productivity (TFP) mechanism is another perspective linking the health expenditure system to economic performance. It posits that an increase in health expenditure leads to a reduction in the burden of diseases and illnesses, thereby boosting labour productivity. However, it is crucial to consider the limitations of this mechanism, as it may not fully account for the broader socio-economic factors that influence economic performance. Additionally, it assumes a linear relationship between health expenditure and productivity, which may oversimplify the complex interactions involved (Halıcı-Tülüce et al., Citation2016).
As researchers, we recognize the significance of health expenditure in fostering economic growth, as emphasized by the theoretical literature. However, we approach these models critically, considering the complexities and interactions present in real-world settings. By doing so, we aim to ensure a nuanced and robust analysis that accounts for the broader dynamics shaping economic performance. This research will contribute to the field by offering a thorough examination of the relationship between health capital and economic performance. By integrating, various factors that influence economic growth, our study seeks to provide a more comprehensive understanding of the link between health expenditure and economic performance. Through this approach, we aim to shed light on the complexities involved and offer valuable insights for policymakers and stakeholders in designing effective strategies to enhance both health capital and economic development.
2.2. Empirical review
The existing literature on health capital and economic performance spans various methodologies, including ARDL, Production Function Based Approach, Structural Equation Model, among others. The literature reveals an established relationship between health capital and economic performance at the global, regional, and country levels. While most studies from developed countries and developing countries demonstrate a positive and significant association between economic success and health capital (Davlasheridze et al., Citation2018; Erçelik, Citation2018; Hasanshahi, Citation2017), there are some exceptions. For instance, Khan et al. (Citation2016) using a structural equation model found a negative relationship between health care spending and economic performance. Similarly, research from developing countries demonstrates mostly consistent positive findings between health capital and economic performance (Chaabouni et al., Citation2016; Umezinwa, Citation2016; Piabuo & Tieguhong, Citation2017). However, there are studies like Edeme and Olisakwe (Citation2019) and Eggoh et al. (Citation2015) that found a negative relationship between health capital and economic performance in Nigeria and developing countries, respectively. These discrepancies in findings might be attributed to differences in methodologies used and the complexities involved in the relationship between health capital and economic performance.
In the context of the Southern African Development Community (SADC) region, research on health capital and economic performance is relatively limited but shows a positive and substantial association (Kouassi et al., Citation2018; Nyamuranga & Shin, Citation2019; Sinha, Citation2021). While Khembo and Tchereni (Citation2013) found a positive correlation between health capital and economic performance in the SADC, the relationship was not statistically significant. Nonetheless, they concluded that the connection is real, highlighting the need for further investigation to fill the knowledge gap in the SADC region.
The reviewed studies on health capital and economic performance offer valuable insights into the relationship between individual health behaviors and overall economic growth (Xu & Jiang, Citation2020). While some studies focus on specific countries or regions, such as the United States (Raghupathi & Raghupathi, Citation2020) and South Africa (Achoki et al., Citation2022), their findings can provide useful theoretical frameworks and contextual information for understanding the broader impact of health capital on economic outcomes. The meta-regression analysis by Ridhwan et al. (Citation2022) highlights the need to consider variations in findings across different studies. The production function approach by Bloom et al. (Citation2004) offers insights into how health capital contributes to economic productivity. The impact of social capital, as highlighted by Boischio et al. (Citation2009), holds particular significance. Social capital refers to the networks, relationships, and norms within a community that facilitate cooperation and mutual support. Boischio et al. (Citation2009) emphasized that social capital plays a crucial role in shaping health outcomes and economic performance. Strong social networks and community cohesion can enhance access to healthcare, encourage healthier behaviours, and promote economic cooperation and productivity.
Building upon the existing body of knowledge, our study aims to conduct a comprehensive analysis using multiple methodologies to gain a deeper understanding of the relationship between health capital and economic performance. By incorporating a range of factors influencing economic growth, including institutional quality, labour force participation and trade openness, we seek to provide more robust and nuanced insights. The objectives of this research are to aid policymakers and governing bodies in developing efficient health care systems to enhance economic output and to offer guidance on the direction of the causality relationship between health capital and economic performance. While the literature supports the connection between health capital and economic performance, there are inconsistencies that necessitate further exploration. Our study in the SADC region aims to contribute valuable insights to fill this knowledge gap and offer evidence-based guidance to foster both health capital and economic development in the region.
3. Methodology
3.1. Model specification
The model developed by Piabuo and Tieguhong (Citation2017), which is based on endogenous growth models, was adopted and modified in this study. One of the primary objectives of their research was to examine the relationship between health expenditure and economic performance across two groups of countries. Their model was formally specified as presented below as Equationequation 1(1)
(1) :
(1)
(1)
Where represents the individual country component including African countries (Botswana, Rwanda, Zambia Madagascar, and Togo) and CEMAC countries (Cameroon, Equatorial Guinea, Gabon, Chad, Central African Republic, and Democratic republic of Congo),
represents time component from 1995 to 2015,
represents the coefficients for the different variables and
is an error term.
The model above was adopted and modified into Equationequation 2(2)
(2) below to achieve the purpose of this study. The model can be presented as follows, in accordance with the study’s aim:
(2)
(2)
Where represents the individual country component (Democratic Republic of Congo, Madagascar, Botswana, Mozambique, Comoros, Tanzania, South Africa, Namibia, Mauritius, Angola, ESwatini, Lesotho, Zambia, and Zimbabwe),
represents time component from 2005 to 2019,
represents the coefficients for the study’s different variables and
is an error term.
3.2. Data sources
This study examined 14 SADCFootnote1 countries excluding Seychelles and Malawi due to data availability (N = 14). For a period of 15 years (2005 to 2019), the research utilised annual data for all variables, including economic performance as defined by GDP and health capital measured by health expenditure as a percentage of GDP as evaluated by Global Data. The data was extracted from the global economy database for GDP per capita, quality of institutions (measured by control of corruption), labour force (measured by labour force participation rate as a %), and trade openness (measured by exports plus imports as a %GDP).
3.3. Estimation techniques
When utilised in individual countries with a limited time, there were flaws in the usage of time series, such as a reduction in the power of unit-root tests and cointegration tests (Maddala & Wu, Citation1999). To solve these flaws, the study employed the panel data approach, which has the ability to increase the degree of freedom while reducing the likelihood of multi-collinearity among independent variables, hence improving the efficiency of econometric estimate (Gujarati, Citation2011).
3.3.1. The panel unit root test and cointegration
In order to evaluate the sequence of integration of variables during the course of this research, two panel unit root tests were carried out based on the information that had been gathered. Both the LLC test developed by Levin et al. (Citation2002) and the IPS test developed by Im et al. (Citation2003) were used in this research. The LLC test is more well recognised. Nevertheless, after the order of integration had been figured out, the research proceeded to investigate the cointegration, also known as the long-term relationship. Many different tests were developed in a multivariate framework to explore the presence of long-term relationships (Kao, Citation1999; Pedroni, Citation1997; Citation1999). These studies included a statistical test in their models that opposed the null hypothesis of no cointegration against the likelihood of cointegration. This study employed seven residual tests, four of which were used inside the dimension panel and the other three across dimension groups. The majority-based decision approach was comparable to Pedroni’s, and this research used a majority-based decision technique similar to Pedroni’s (Citation1997). The Kao (Citation1999) test was utilised to confirm the accuracy of the Pedroni findings. The panel ARDL model was performed in the last stage to examine the short-run and long-run coefficients. Lastly, tests for robustness of the model were done including fixed effects (FE) model, Fully Modified Ordinary Least Square (FMOLS) model, and normality test. Furthermore, for stability of parameters Ramsey Reset test was performed.
3.3.2. Pooled mean group (PMG) estimator
The PMG estimator was used to identify important insights on the long-term relationship between health capital and economic performance in the SADC region. For each country examined, the PMG estimator model was used to examine the long- and short-term relationships between health capital and economic performance in the SADC region. As an intermediate estimator, PMG outperforms mean group and dynamic fixed effects (Piabuo & Tieguhong, Citation2017). This is because PMG provides important judgments in the presence of long-run homogeneity (Piabuo & Tieguhong, Citation2017). The PMG estimator produces very varied short-run coefficients and error variances, but when simple averages of individual unit coefficients are constructed, the estimates of mean short-run coefficients are consistent. This study used a panel ARDL approach. The ARDL (p, q) model is specified by the following equation:
(4.3)
(4.3)
With = 1, 2,………, N is the number of countries;
= 1, …,
is the time;
is the number of lags;
is the vector of the variables relating to economic performance and
is the specific fixed effect of the countries. To consider the adjustment coefficient and the long-run dynamics, Equation (1) is reparametrized as follows:
(4.4)
(4.4)
Where is the adjustment coefficient of the long run dynamics.
indicates the long-run equilibrium relationship between
and
represents the short run coefficient linking the economic performance of the current period with its past values and the variables of interest
When there is long-run relationship between health capital and economic performance the
will be negative and significant (Mlambo et al., Citation2022). To estimate the ARDL model, PMG was employed as the appropriate estimator because of its consistency under the assumption of long slope homogeneity and other advantages discussed above.
3.3.3. Robust estimators (fully Modified least squares (FMOLS) and fixed effects (FE) methods
In our study on health capital and economic performance in the SADC region, we employ robust estimators, including Fully Modified Least Squares (FMOLS) and Fixed Effects (FE) methods, to ensure the accuracy and reliability of our results. The use of panel ARDL models is appropriate for our research as they allow us to analyse the long-run relationship between health capital and economic performance while controlling for the potential heterogeneity and dynamics within the panel data. FMOLS is preferred in our study as it efficiently addresses endogeneity issues and provides consistent estimates even when the explanatory variables are endogenous (Phillips & Hansen, Citation1990). This is crucial in the context of health capital and economic performance, where the relationship might be subject to reverse causality or omitted variable bias. Additionally, FE estimation allows us to account for time-invariant unobservable factors at the country level, reducing potential bias and improving the precision of our estimates (Nickell, Citation1981). By using robust estimators, we can obtain more reliable and unbiased results, thus enhancing the robustness and credibility of our findings (Beck & Katz, Citation2011; Pesaran & Yamagata, Citation2008).
4. Empirical results
4.1. Correlation
The estimate procedure started with preliminary tests to assess the correlation and guarantee that the explanatory variables are correlated. This test is very important in ascertaining that the model is to avoid the issues of multicollinearity or collinearity. According to Gujarati (Citation2011), multicollinearity means that two or more explanatory variables explain each other rather than explaining the dependent variable and therefore should not be on the right side of the equation to avoid this problem of multicollinearity or collinearity. The results are presented in .
Table 1. Correlations.
Given the range of absolute values in the table above (0.019816 – −0.509512), it is safe to assume that there is no problem with multicollinearity among the explanatory variables. The study by Ologunde et al. (Citation2020) revealed that there is no specific rule or rules in the modern econometric analyses to indicate that there can be perfect or exact values that mean perfect relationship or whatsoever exists, and hence moving away from the initial definition of perfect value or exact value. However, it would appear that all the explanatory variables in this study were not linearly dependent on one another. This is evidenced in showing that the highest correlation value in the model is −0.509512 which is health expenditure (HE) to labour force participation rate (LF). This is closely followed by the value −0.398916 which is health expenditure (HE) to institutional quality (HC_INST), followed by −0.360031 which is trade openness (TR) to labour force participation rate (LF), followed by health expenditure (HE) and trade openness (TR) at −0.319171, followed by institutional quality (HC_INST) to trade openness (TR) sitting at −0.295880, and lastly institutional quality (HC_INST) to labour force participation rate (LF) sitting at 0.019816. There was no sign of multicollinearity between the explanatory variable in any of the values, thus all these values are acceptable based on the existing literature (Ologunde et al., Citation2020). Therefore, the model was deemed safe to proceed with the variables to the estimation of the model specified earlier because it exhibited the acceptable correlation amongst explanatory variables against multicollinearity or collinearity challenge.
4.2. Descriptive statistics
The table contained 198 observations and descriptive statistics (). TR had the highest mean (80.77410) and the lowest GDP per capita (2.113442). The standard deviations explain variances in each variable list of observation across the 14 chosen SADC nations. The lowest standard deviation is 2.172696, followed by GDP per capita at 3.366668. The table’s 3rd and 4th rows recorded the variables’ maximum and minimum values. The table’s 5th row showed the data’s skewness, followed by the 6th row’s kurtosis.
Table 2. Descriptive statistics.
4.3. Panel unit root tests
The formal panel unit root tests presented below were done to ascertain the order of integration of variables in this study. Levin et al. (Citation2002), commonly known as LLC, Im et al. (Citation2003), popularly known as IPS, are among the tests that were undertaken and presented below ().
Table 3. Unit root tests.
Results show that under the Levin test, 3 variables had unit root at levels (LF, HC_INT and TR). These variables became stationary after being differenced once. HE and GDP_PC were stationary at levels. Under the Lm and Pesaran test, TR and LF had unit root. They became stationary after first differencing. The rest of the variables were stationary at levels. This shows that the series is a combination of I(0) and I(1) variables which necessitates the use of panel cointegration techniques. Having established the sequence of integration, the study determined the long-term relationship, or cointegration.
4.4. Panel cointegration test
The Johansen cointegration test is often used to evaluate long-term relationships. In multivariate systems with small sample numbers, the power of the Johansen test may be deceiving, according to Piabuo and Tieguhong (Citation2017). Using a multivariate framework, long-term relationships were identified (Kao, Citation1999; Pedroni, Citation1997; Citation1999). In the absence of a null hypothesis, these researchers used statistical analyses to evaluate the probability of cointegration. This study employed seven residual tests, four inside the dimension panel and three across dimensions. There was also a combination of Pedroni’s (Citation1997) and this study’s methods for majority-based selection. In order to improve Pedroni’s results, the Kao (Citation1999) test was also used. The results are presented in the below.
Table 4. Cointegration test (Pedroni).
The results show that there is cointegration amongst the variables. Of all the 11 test statistics, eight tests found evidence of cointegration amongst the variables. This is seen by the p-values which are below 0.05. The Kao test was also performed to confirm the Pedroni test results. Results are shown in the below.
Table 5. Kao residual cointegration test.
Results from the Kao test also show that there is cointegration amongst the variables. The p-value turned out to be 0.0122 and this is below the 5% significance level which means that the results are probably statistically significant, and the t-statistic value is above 2 which means the results are statistically significant. This confirms the presence of cointegration amongst variables. Therefore, this study could run both short-run and long-run regressions.
4.5. Regression results
The primary objective of the study was to examine the relationship between health capital and economic performance, using 14 Southern African Development Community member states for the period of 15 years from 2005 to 2019. The study included other explanatory variables such as institutional quality (HC_INST), labour force participation rate (LF), and trade openness (TR). To achieve this objective this study took advantage of different estimation techniques that were identified and explained in Chapter 4 above including the three basic panel regression estimators of which this study had chosen one based on the advantages identified in Chapter 4. The diagnostic tests were also performed in this study to assist the study in establishing the correct robust standard error estimators for the study.
4.5.1. Long-run results
After detecting the presence of cointegration, the study determined its estimates using the PMG. According to Pesaran et al. (Citation1999), the PMG is more informative and has an increased efficiency compared to the MG. The results are presented and discussed in the next two subsections.
4.5.1.1. Panel long-run ARDL results
This study made use of the PMG estimators. The long-run results are displayed in .
Table 6. Pooled mean group (PMG) results.
As can be seen in the table above, there is a negative relationship between the health expenditure (HE) and the GDP per capita in the long run. Although the findings are significant at a significance level of 10%, they do not correspond to what was anticipated before doing this research. This demonstrates that health spending has a negative effect on GDP per capita. This indicates that a one unit increase in total health spending will result in a 0.2068 percentage point decline in overall economic growth. The findings of this study are consistent with the findings of Ogundipe and Lawal, (Citation2011) in Nigeria, where it was found that there is a negative relationship between health expenditure and economic growth.
These finding by Ogundipe and Lawal (Citation2011) was later contradicted by Piabuo and Tieguhong (Citation2017), who found that there is a positive and significant relationship between health expenditure and economic growth, which encourages investment in health care. One of the reasons why there is a negative relationship is the fact that most African nations do not allocate their health expenditures to productive areas of the economy which could contribute to the negative impact that health expenditures have on the performance of the economy. Other variables may also be at play. For instance, the majority of African nations are struggling under the weight of HIV and other non-communicable illnesses. Although the larger budgets of African nations are often allocated to the fight against these illnesses, the fact that these funds are being spent does not immediately have a favourable impact on the economic performance of the country; rather, it enhances the welfare of the population.
Another reason noted in literature (for example Hlafa et al., Citation2019) is the problem of corruption in the health sector. This makes economic sense given the fact that during the time of COVID-19 alone there were allegations of corruption in some of the member states of the SADC region. According to an examination of literature and media reports undertaken between February and December 2020, corruption in service delivery was as prevalent as ever during the COVID-19 pandemic, resulting in the exploitation of especially vulnerable people (Cuadrado, Citation2022).
South Africa’s inadequate accountability in the health sector, which decreases health system efficacy, and the country’s overall high levels of corruption are acknowledged in the NDP as major obstacles to the country’s progress toward its many social and developmental objectives (Rispel, De Jager & Fonn, Citation2016). Even in the instance of Zimbabwe, where the poor are disproportionately impacted by corruption in the health sector, it was found that bribery, nepotism, embezzlement, theft, mismanagement, and absenteeism are among the most common kinds of corruption in the country (Muchena, Citation2019). Corrupt practices are commonplace in the health care industry of many developing countries, which has serious consequences for people’s health and quality of life (Vian, Citation2008). Given these realities, it stands to reason that health care spending drags down economic performance in the selected SADC countries. Corruption causes a large loss of funds, as seen by the system’s leakage.
above also shows that there is a positive relationship between institutional quality and GDP per capita, which is denoted by the positive coefficient (0.0754). This implies that 1 unit improvement in institutional quality (HC_INST) will result in 0.0754% increase in GDP per capita. The results are statistically significant at 10% significance level and consistent with the expected priori of this study that there is a positive relationship between the quality of institution and economic performance, consistent with the literature (Asghar et al., Citation2020; Mokdad et al., Citation2018; Radulović, Citation2020).
According to the findings, there is a negative relationship between the labour force (LF) and the GDP per capita. At a significance level of 5%, the findings are statistically significant. This demonstrates that LF has a detrimental impact on GDP per capita. In contrast to the findings of Piabuo and Tieguhong, (Citation2017), who found that labour force has a significantly positive impact on GDP per capita, this argument contends that investing in human capital results in a healthy labour force, which in turn improves economic performance. Piabuo and Tieguhong, (Citation2017) found that labour force has a significantly positive impact on GDP per capita. The results of this study do not support the hypothesis that an increase in the labour force participation rate would lead to a rise in productivity, which would result in higher earnings. Higher earnings would lead to increased spending and savings, which would improve people’s well-being and happiness, which in turn would improve the economy’s performance. However, the findings of this study do not support this hypothesis (Aboubacar & Xu, Citation2017). However, these findings are consistent with Motsatsi’s (Citation2019) findings that the high tendency of poor skilled labour employment and high rate of unemployment in the SADC region can explain the negative relationship between the labour force participation rate and economic growth in that region.
In countries like Botswana, the Democratic Republic of the Congo, Lesotho, Mauritius, Mozambique, Namibia, South Africa, Swaziland, and Tanzania, a weak education system that has not been able to provide job seekers with the requisite skills needed in the labour force has received much of the blame for contributing to a high labour force participation rate with no improvement in the economic performance. Low levels of productivity are the direct outcome of a lack of skills, which in turn has a negative impact on the overall functioning of the economy. It is likely that a lack of vocational training opportunities and restricted access to skill development have made it more difficult for the school system to assist the development of practical skills necessary in the labour market (Motsatsi, Citation2019). On the basis of all this data, it is clear that one of the challenges that the SADC area is experiencing is the fact that individuals who are employed most likely earn poor incomes and do not have suitable employment, which is detrimental to the region’s economic progress.
According to the findings, there is a relationship between trade openness (TR) and GDP per capita that is in the positive direction. At a significance level of 5%, the findings are statistically significant. This demonstrates that TR has a beneficial effect on GDP per capita. An improvement of 0.021 percentage points in economic performance may be expected in the SADC area for every one unit that trade openness is increased. These results are in line with what was anticipated before to doing this research, and they have been supported by Keho (Citation2017). The research conducted by Keho (Citation2017) revealed that there is a positive and powerfully complementary relationship between economic openness and the formation of capital in the process of fostering economic growth. This relationship is beneficial to economic development both in the short run and in the long run. To put it another way, this suggests that there is a positive and substantial link between the openness of trade and the success of the economy in the SADC area. Within the context of regional economic integration within the SADC area, one of the major pillars is the liberalisation of trade. The value of goods exported inside the SADC in 2019 was about $34.8 billion, while the value of goods imported within the SADC was around $32.6 billion.
4.5.1.2. Short-run results
According to the findings, the coefficient of the error correction term (ECT) is found to be significant at a significance level of 1% even though it is negative (-.08748). This suggests that the disequilibrium is restored to its original state each year to the extent of 87%.
According to the findings, there is an inverse relationship between the health expenditure and the GDP per capita. At a significance level of 5%, the findings are statistically significant. This demonstrates that health spending has a negative effect on GDP per capita. In other words, the change in GDP per capita would be 0.8847 units lower if there was a unit change in health expenditure (HE) in the short run ().
Table 7. Panel short-run ARDL results.
According to the findings, there is a connection between HC and GDP per capita, but it is one that is neither positive nor statistically significant. This shows that a unit improvement in institutional quality (HC INST) would result in enhanced economic performance by 0.0181%; nevertheless, the findings are statistically insignificant due to the p-value surpassing the accepted 10% significance threshold by about 75.24%. This suggests that institutions do not significantly affect GDP per capita over the near term.
According to the findings, there is an inverse correlation that cannot be considered statistically significant between the GDP per capita and the labour force participation rate (LF). This indicates that a one-unit change in the labour force participation rate (LF) would result in a drop in GDP per capita of −0.0679 dollars. Due to the fact that the p-value (17.12%) is higher than the accepted significance limit of 10%, the data cannot be considered statistically significant. This demonstrates that in the near term, the LF does not have a detrimental effect on GDP per capita.
Also, according to the findings, there is a correlation between trade openness (TR) and GDP per capita that is in the positive direction. This suggests that a one-unit increase in trade openness (TR) would result in a shift of 0.0910 percentage points in GDP per capita. Because the p-value is 1.40%, which is lower than the 5% significance threshold, the findings are statistically significant when evaluated using a 5% significance level. This demonstrates that TR has a beneficial effect on GDP per capita both in the long run and in the near term.
Two different methodologies for secondary panel testing were used so that the effectiveness and robustness of the PMG could be evaluated. These models were referred to as FMOLS and random effects respectively. The PMG model was put through several tests so that researchers may evaluate how resilient it is. The outcomes are summarised in and below.
Table 8. FMOLS results.
Table 9. Fixed effects.
The findings from both the Fully Modified Least Squares (FMOLS) and fixed effects models align with those obtained from the Panel ARDL (Auto Regressive Distributed Lag) analysis. However, a notable difference arises with FMOLS, where the health capital (HC) variable was found to be statistically insignificant in affecting GDP per capita. This implies that according to the FMOLS results, health capital does not play a significant role in explaining the variations in GDP per capita. Conversely, in the fixed effects model, the labour force (LF) variable was also found to be statistically insignificant in explaining changes in GDP per capita. Thus, under the fixed effects approach, the labour force does not appear to be a significant determinant of the variations in GDP per capita.
4.6. Diagnostic tests
To provide robustness of the estimated coefficients, this study performed diagnostic tests. below presents the results of the diagnostic tests that this study adopted.
Table 10. Diagnostic tests.
The residuals were found to have a normal distribution, as shown by the normality test. This is shown by the fact that the p-value of the Jarque-Bera test was more than 0.05. (0.1390). In addition, the value of skewness was quite near to zero (0.3095), while the value of kurtosis was relatively close to 3. (3.3085). It may be deduced from this that the residuals followed a normal distribution.
5. Policy implications and recommendations
The findings of an inverse relationship between health expenditure (HE) and GDP per capita in both the short run and long run have important policy implications. At a significance level of 5%, the negative effect of health spending on GDP per capita indicates that a unit increase in health expenditure is associated with a decrease of 0.8847 units in GDP per capita in the short run. This suggests that while investing in health may improve population well-being and reduce the burden of diseases, it could have short-term implications for economic performance. In the long run, the negative relationship between health expenditure and GDP per capita remains, with the findings being significant at a 10% significance level. This indicates that over the long term, excessive health spending might have adverse effects on economic growth. Policymakers need to carefully balance health investments with other development priorities to ensure sustainable economic growth.
The study also highlights the importance of institutional quality in improving GDP per capita in the long run. Institutional quality plays a crucial role in creating an enabling environment for economic growth by fostering investor confidence, promoting efficient resource allocation, and reducing corruption. Therefore, policymakers should focus on strengthening institutions, enhancing governance, and implementing reforms to create a conducive business environment that encourages investment and economic development.
From a theoretical perspective, the findings underscore the need for a comprehensive understanding of the relationship between health expenditure and economic performance. While investing in health is crucial for human development and overall well-being, excessive health spending without proper planning and efficient resource allocation could have unintended consequences on economic growth.
In practice, the study suggests that policymakers should adopt a prudent and evidence-based approach to health spending, focusing on cost-effective interventions and promoting preventive measures. Efficient allocation of resources in the health sector, along with measures to improve healthcare management and delivery, can help maximise the benefits of health investments while minimizing the potential negative impact on GDP per capita.
On a policy level, the findings call for a holistic approach that considers the interplay between health investments, economic development, and institutional quality. Policymakers need to foster collaboration between the health sector and other economic sectors to ensure coherence and synergy in development policies. Moreover, policies aimed at improving institutional quality and governance are essential for creating an environment conducive to sustained economic growth.
The study’s findings suggest that while health spending is essential for human development, an overly high level of health expenditure might have negative implications for GDP per capita. Policymakers should carefully balance health investments with other development priorities and focus on improving institutional quality to foster sustainable economic growth and well-being in the long run. By adopting a prudent and evidence-based approach to health spending and governance, countries within the SADC region can promote both health and economic development effectively.
6. Recommendations for future research
For future research, it is recommended to study deeper into the underlying factors that contribute to the observed inverse relationship between health expenditure and GDP per capita. Exploring the specific mechanisms through which health spending affects economic performance can provide a more nuanced understanding of the relationship. Additionally, conducting comparative studies across different countries within the SADC region can help identify variations in the impact of health expenditure on economic growth, considering the diverse economic, social, and institutional contexts. Furthermore, it would be valuable to investigate the role of healthcare quality and efficiency in mediating the relationship between health spending and GDP per capita. Longitudinal studies that track the effects of health investments over an extended period can shed light on the dynamic nature of this relationship. It is essential for future research to also further explore the impact of social capital on health capital and economic performance. Future studies could investigate the role of social capital in shaping health behaviors, access to healthcare services, and resource mobilization for health investments. Lastly, given the significance of institutional quality in influencing economic performance, future research should focus on the interplay between institutional reforms, health investments, and economic development, providing insights into how policy interventions can optimise the benefits of health spending while ensuring sustained and inclusive economic growth in the SADC region.
Disclosure statement
No potential conflict of interest was reported by the author(s).
Additional information
Notes on contributors
Alungile Qoko
Alungile Qoko recently graduated with a Master’s degree from Walter Sisulu University and is currently a dedicated PhD student registered for a doctoral program at the University of Fort Hare. His research interests primarily focus on development economics, health economics, and environmental economics. Alungile is passionate about economic development, offering valuable insights into policy formulation and societal progress.
Kin Sibanda
Professor Kin Sibanda is a distinguished researcher in economics, holding a PhD in the field. He is an NRF (National Research Foundation) rated scholar and currently serves as a professor at Walter Sisulu University. Prof. Sibanda’s expertise encompasses development economics, macroeconomics, health economics, and environmental economics. Through his academic pursuits, Prof. Sibanda aims to contribute valuable insights to these fields, addressing critical issues related to economic development, healthcare systems, and environmental sustainability.
Phakama Senzangakhona
Phakhama Senzangakhona is a dedicated lecturer at Walter Sisulu University and a PhD candidate at Nelson Mandela University. With a particular interest in development economics, macroeconomics, and international trade, Phakhama’s research pursuits aim to expand knowledge and offer fresh insights into these pivotal areas of economic study.
Notes
1 Democratic Republic of Congo, Madagascar, Botswana, Mozambique, Comoros, Tanzania, South Africa, Namibia, Mauritius, Angola, ESwatini, Lesotho, Zambia, and Zimbabwe.
References
- Aboubacar, B., & Xu, D. Y. (2017). The impact of health expenditure on the economic growth in sub-Saharan Africa. Theoretical Economics Letters, 07(03), 615–622. https://doi.org/10.4236/tel.2017.73046
- Achoki, T., Sartorius, B., Watkins, D., Glenn, S. D., Kengne, A. P., Oni, T., Wiysonge, C. S., Walker, A., Adetokunboh, O. O., Babalola, T. K., Bolarinwa, O. A., Claassens, M. M., Cowden, R. G., Day, C. T., Ezekannagha, O., Ginindza, T. G., Iwu, C. C. D., Iwu, C. J., Karangwa, I., … Naghavi, M. (2022). Health trends, inequalities and opportunities in South Africa’s provinces, 1990–2019: findings from the Global Burden of Disease 2019 Study. Journal of Epidemiology and Community Health, 76(5), 471–481. https://doi.org/10.1136/jech-2021-217480
- Affoh, R., Zheng, H., Dangui, K., & Dissani, B. M. (2022). The impact of climate variability and change on food security in sub-saharan africa: Perspective from panel data analysis. Sustainability, 14(2), 759. https://doi.org/10.3390/su14020759
- Arthur, E., & Hassan, O. (2017). The effects of health expenditure on health outcomes in Sub‐Saharan Africa (SSA). African Development Review, 29(3), 524–536. https://doi.org/10.1111/1467-8268.12287
- Asghar, N., Qureshi, S., & Nadeem, M. (2020). Institutional quality and economic growth: Panel ARDL analysis for selected developing economies of Asia. South Asian Studies, 30(2).
- Beck, N., & Katz, J. N. (2011). Modeling dynamics in time-series-cross-section political economy data. Annual Review of Political Science, 14(1), 331–352. https://doi.org/10.1146/annurev-polisci-071510-103222
- Bloom, D. E., Canning, D., & Sevilla, J. (2004). The effect of health on economic growth: a production function approach. World Development, 32(1), 1–13. https://doi.org/10.1016/j.worlddev.2003.07.002
- Boischio, A., Sánchez, A., Orosz, Z., & Charron, D. (2009). Health and sustainable development: Challenges and opportunities of ecosystem approaches in the prevention and control of dengue and Chagas disease. International Development Research Centre, 25, pp.149–154.
- Chaabouni, S., Zghidi, N., & Mbarek, M. B. (2016). On the causal dynamics between CO2 emissions, health expenditures and economic growth. Sustainable Cities and Society, 22, 184–191. https://doi.org/10.1016/j.scs.2016.02.001
- Chandra Das, R., & Chatterjee, A. (2020). Depression rate, GDP growth rate, health expenditure, and voice and accountability: Are there any co-movements? Multidimensional Perspectives and Global Analysis of Universal Health Coverage, pp. 21.
- Cuadrado, D. C. (2022). The ignored pandemic behind COVID-19: The impact of corruption on healthcare service delivery. Transparency International Global Health, June 03. https://ti-health.org/content/corruption-covid-19-coronavirus-health-delivery/
- Davlasheridze, M., Goetz, S. J., & Han, Y. (2018). The effect of mental health on US county economic growth. Review of Regional Studies, 48(2), 155–171. https://doi.org/10.52324/001c.7997
- Demirci, N., Yıldırım, İ., Toptaş Demirci, P., & Ersöz, Y. (2018). Why should we do physical activity? More active people for a healthier world. International Journal of Disabilities Sports and Health Sciences, 1(2), 1–14. https://doi.org/10.33438/ijdshs.488292
- Edeme, R. K., & Olisakwe, O. (2019). Public health expenditure, economic growth and health outcomes in Nigeria. International Journal of Public Policy and Administration Research, 6(1), 23–32. https://doi.org/10.18488/journal.74.2019.61.23.32
- Eggoh, J., Houeninvo, H., & Sossou, G.-A. (2015). Education, health and economic growth in African countries. Journal of Economic Development, 40(1), 93.
- Erçelik, G. (2018). The relationship between health expenditure and economic growth in Turkey from 1980 to 2015. Journal of Politics, Economy and Management, 1(1), 1–8.
- Grossman, M. (1999). The human capital model of the demand for health. Working Paper No. 7078. Nation Bureau of Economic Research. https://doi.org/10.3386/w7078
- Gujarati, D. (2011). Econometrics by example. Macmillan.
- Halıcı-Tülüce, N. S., Doğan, İ., & Dumrul, C. (2016). Is income relevant for health expenditure and economic growth Nexus? International Journal of Health Economics and Management, 16(1), 23–49. https://doi.org/10.1007/s10754-015-9179-8
- Hasanshahi, M. (2017). Measuring the impact of health on economic growth. Journal of Health Administration (JHA), 20(69), 7–17.
- Hlafa, B., Sibanda, K., & Hompashe, D. M. (2019). The impact of public health expenditure on health outcomes in South Africa. International Journal of Environmental Research and Public Health, 16(16), 1–13. https://doi.org/10.3390/ijerph16162993
- Im, K. S., Pesaran, H., & Shin, Y. (2003). Testing for unit roots in heterogeneous panels. Journal of Econometrics, 115(1), 53–74. https://doi.org/10.1016/S0304-4076(03)00092-7
- Kao, C. (1999). Spurious regression and residual-based tests for cointegration in panel data. Journal of Econometrics, 90(1), 1–44. https://doi.org/10.1016/S0304-4076(98)00023-2
- Keho, Y. (2017). The impact of trade openness on economic growth: The case of Cote d’Ivoire. Cogent Economics & Finance, 5(1), 1332820. https://doi.org/10.1080/23322039.2017.1332820
- Khan, H. N., Khan, M. A., Razli, R. B., Sahfie, A. B., Shehzada, G., Krebs, K. L., & Sarvghad, N. (2016). Health care expenditure and economic growth in SAARC countries (1995–2012): A panel causality analysis. Applied Research in Quality of Life, 11(3), 639–661. https://doi.org/10.1007/s11482-015-9385-z
- Khembo, F., & Tchereni, B. (2013). The impact of human capital on economic growth in the SADC region. Developing Country Studies, 3, 144–152.
- Kouassi, E., Akinkugbe, O., Kutlo, N. O., & Brou, J. M. (2018). Health expenditure and growth dynamics in the SADC region: Evidence from non-stationary panel data with cross section dependence and unobserved heterogeneity. International Journal of Health Economics and Management, 18(1), 47–66. https://doi.org/10.1007/s10754-017-9223-y
- Levin, A., Lin, C.-F., & James Chu, C.-S. (2002). Unit root tests in panel data: asymptotic and finite-sample properties. Journal of Econometrics, 108(1), 1–24. https://doi.org/10.1016/S0304-4076(01)00098-7
- Li, L., Wu, M., & Wu, Z. (2017). The impact of public health expenditure on economic development –Evidence from prefecture-level panel data of Shandong Province. Research in World Economy, 8(2), 59. [Online]. Available at: (accessed 23 September 2022) https://doi.org/10.5430/rwe.v8n2p59
- Lucas, R. E. Jr., (1988). On the mechanics of economic development. Journal of Monetary Economics, 22(1), 3–42. https://doi.org/10.1016/0304-3932(88)90168-7
- Maddala, G., & Wu, S. (1999). A comparative study of unit root tests with panel data and a new simple test. Oxford Bulletin of Economics and Statistics, 61(S1), 631–652. https://doi.org/10.1111/1468-0084.61.s1.13
- Mahmoud A. (2015). Quantitative Research Design. https://doi.org/10.13140/RG.2.1.3539.6001
- Mehrara, M., & Musai, M. (2011). Granger causality between health and economic growth in oil exporting countries. Interdisciplinary Journal of Research in Business, 1(8), 103–108.
- Mlambo, C., Sibanda, K., Ntshangase, B., & Mvuyana, B. (2022). ICT and women’s health: An examination of the impact of ICT on maternal health in SADC states. Healthcare, 10(5), 802. https://doi.org/10.3390/healthcare10050802
- Mokdad, A. K., Ballestros, K., Echko, M., Glenn, S., Olsen, H., Mullany, E., & Kasaeian, A. (2018). The state of US health, 1990–2016: Burden of diseases, injuries, and risk factors among US states. JAMA, 319(14), 1444–1472.
- Motsatsi, J. M. (2019). Unemployment in the SADC region. Botswana Institute for Development Policy Analysis.
- Muchena, R. I. (2019). An exploration of corruption in the health sector: A case of Zimbabwe. Stellenbosch University, Stellenbosch. https://scholar.sun.ac.za
- Ndaguba, E., & Hlotywa, A. (2021). Public health expenditure and economic development: The case of South Africa between 1996 and 2016. Cogent Economics & Finance, 9(1), 1905932. https://doi.org/10.1080/23322039.2021.1905932
- Nickell, S. (1981). Biases in dynamic models with fixed effects. Econometrica, 49(6), 1417–1426. https://doi.org/10.2307/1911408
- Nyamuranga, C., & Shin, J. (2019). Public health expenditure and child mortality in Southern Africa. International Journal of Social Economics, 46(9), 1137–1154. https://doi.org/10.1108/IJSE-12-2018-0643
- Ogundipe, & Lawal, A. (2011). Health expenditure and Nigerian economic growth. European Journal of Economics, Finance and Administrative Sciences, 30(4), 125–129.
- Ologunde, I., Kapingura, F., & Sibanda, K. (2020). Sustainable development and crude oil revenue: A case of selected crude oil-producing African countries. International Journal of Environmental Research and Public Health, 17(18), 6799. https://doi.org/10.3390/ijerph17186799
- Pedroni, P. (2018). Panel co-integration techniques and open challenges. Panel Data Econometric Volume 1: Theory – forthcoming. Elsevier. https://web.williams.edu/Economics/wp/Pedroni_PanelCointegration.pdf
- Pedroni, P. (1997). Asymptotic and finite sample properties of pooled time series. Bloomington, Indiana University.
- Pedroni, P. (1999). Critical values for cointegration tests in heterogeneous panels with multiple regressors. Oxford Bulletin of Economics and Statistics, 61(s1), 653–670. https://doi.org/10.1111/1468-0084.61.s1.14
- Pesaran, H. M., & Pesaran, B. (1997). Working with Microfit 4.0: Interactive econometric analysis. Oxford University Press.
- Pesaran, H. M., Shin, Y., & Smith, R. P. (1999). Pooled mean group estimation of dynamic heterogeneous panels. Journal of the American Statistical Association, 94(446), 621–634. https://doi.org/10.2307/2670182
- Pesaran, M. H., & Yamagata, T. (2008). Testing slope homogeneity in large panels. Journal of Econometrics, 142(1), 50–93. https://doi.org/10.1016/j.jeconom.2007.05.010
- Phillips, P. C., & Hansen, B. E. (1990). Statistical inference in instrumental variables regression with I(1) processes. The Review of Economic Studies, 57(1), 99–125. https://doi.org/10.2307/2297545
- Piabuo, S. M., & Tieguhong, J. C. (2017). Health expenditure and economic growth: A review of the literature and an analysis between the economic community for central African states (CEMAC) and selected African countries. Health Economics Review, 7(1), 23. https://doi.org/10.1186/s13561-017-0159-1
- Radulović, M. (2020). The impact of institutional quality on economic growth: A comparative analysis of the EU and non-EU countries of Southeast Europe. Economic Annals, 65(225), 163–181.
- Raghupathi, V., & Raghupathi, W. (2020). Healthcare expenditure and economic performance: Insights from the United States data. Frontiers in Public Health, 8, 156. https://doi.org/10.3389/fpubh.2020.00156
- Rebelo, S. (1991). Long-run policy analysis and long-run growth. Journal of Political Economy, 99(3), 500–521. https://doi.org/10.1086/261764
- Ridhwan, M. M., Nijkamp, P., Ismail, A., M., & Irsyad, L. (2022). The effect of health on economic growth: A meta-regression analysis. Empirical Economics, 63(6), 3211–3251. https://doi.org/10.1007/s00181-022-02226-4
- Rispel, L. C., De Jager, P., & Fonn, S. (2016). Exploring corruption in the South African health sector. Health Policy and Planning, 31(2), 239–249. https://doi.org/10.1093/heapol/czv047
- Romer, P. (1986). Increasing returns and long-run growth. Journal of Political Economy, 94(5), 1002–1037. https://doi.org/10.1086/261420
- Schneider-Kamp, A. (2021). Health capital: Toward a conceptual framework for understanding the construction of individual health. Social Theory & Health: STH, 19(3), 205–219. https://doi.org/10.1057/s41285-020-00145-x
- Sinha, N, Professor of Economics, University of Botswana, Gaborone, Botswana. (2021). Health expenditure and GDP Growth in Botswana. SDMIMD Journal of Management, 12(1), 1–17. https://doi.org/10.18311/sdmimd/2021/26140
- Southern African Development Community (SADC). (2022). Towards the common future. [Online]. https://www.sadc.int/sadc-statistics/statistics-database
- Umezinwa, C., 2016. Health and economic growth in South East, Nigeria. African Research Review, 10(3), pp.111–123.
- Vian, T. (2008). Review of corruption in the health sector: theory, methods and interventions. Health Policy and Planning, 23(2), 83–94. https://doi.org/10.1093/heapol/czm048
- World Health Organisation (WHO). (2017). Health expenditure and economic performance in African countries. World Health Statistics.
- Xu, P., & Jiang, J. (2020). Individual capital structure and health behaviours among Chinese middle-aged and older adults: A cross-sectional analysis using Bourdieu’s theory of capitals. International Journal of Environmental Research and Public Health, 17(20), 7369. https://doi.org/10.3390/ijerph17207369
- Yazdi, S. K., & Khanalizadeh, B. (2017). Air pollution, economic growth and health care expenditure. Economic Research-Ekonomska Istraživanja, 30(1), 1181–1190. https://doi.org/10.1080/1331677X.2017.1314823
- Yıldırım, S., Yildirim, D. C., & Calıskan, H. (2020). The influence of health on economic growth from the perspective of sustainable development: A case of OECD countries. World Journal of Entrepreneurship, Management and Sustainable Development, 16(3), 181–194. https://doi.org/10.1108/WJEMSD-09-2019-0071
- Zaidi, S., & Saidi, S. (2018). Environmental pollution, health expenditure and economic growth in the sub-Saharan Africa countries: Panel ARDL Approach. Sustainable Cities and Society, 41, 833–840. https://doi.org/10.1016/j.scs.2018.04.034
- Zhao, Y., Oldenburg, B., Mahal, A., Lin, Y., Tang, S., & Liu, X. (2019). Trends and socio‐economic disparities in catastrophic health expenditure and health impoverishment in China: 2010 to 2016. Tropical Medicine & International Health, 25(2), 236–247.